Semantic AI in Business Intelligence: Transforming Data into Actionable Insights
Imagine having a business analyst who understands your needs instantly and delivers precise insights from your company’s vast data. That’s the power of Semantic AI in business intelligence.
According to recent industry studies, Semantic AI is changing how businesses interact with their data by understanding context and relationships. Unlike traditional tools that just process numbers, Semantic AI grasps the meaning behind your data, much like a human expert would.
Think of Semantic AI as your organization’s cognitive bridge, connecting scattered data points into a coherent story that drives smarter decisions. When a sales manager asks about customer churn in Q4, Semantic AI understands the need to know about lost customers, related factors, and potential preventive actions, not just raw numbers.
Most importantly, this technology transforms how teams access and utilize business intelligence. By understanding context and natural language, it empowers everyone from executives to analysts to extract meaningful insights without needing deep technical expertise. The struggle with complex query languages or waiting for IT support is becoming history.
This article explores how Semantic AI is reshaping business intelligence in three critical areas: enhancing data analysis capabilities, streamlining decision-making processes, and creating sustainable competitive advantages in today’s data-driven marketplace. Whether you’re looking to improve operational efficiency or uncover hidden market opportunities, Semantic AI offers the tools to turn your data into actionable intelligence.
How Semantic AI Works
Semantic AI represents a fusion of natural language processing (NLP), knowledge graphs, and machine learning. Unlike traditional AI systems that process data in isolation, Semantic AI creates an intricate web of interconnected information that mirrors how humans understand and relate concepts.
At its core, Semantic AI uses knowledge graphs to establish a structured collection of real-world entities and their relationships. These graphs function like a digital brain, storing information as interconnected nodes and edges that capture the nuanced connections between different pieces of data. Just as humans understand that a “mouse” could refer to either a computer peripheral or a rodent based on context, knowledge graphs enable AI systems to grasp these semantic distinctions.
Natural language processing serves as the interpretive layer, allowing Semantic AI to parse and comprehend human language in its natural form. Through sophisticated NLP techniques, the system can extract meaning from unstructured text, identify entities, and understand the relationships between concepts, much like how we process conversations and written content.
Machine learning algorithms then work in concert with these components to continuously improve the system’s understanding. They analyze patterns in data, refine semantic connections, and enhance the AI’s ability to make context-aware decisions. This creates a virtuous cycle where the system becomes increasingly adept at understanding nuanced meanings and relationships.
The practical impact of this integration is remarkable. When conducting data searches, Semantic AI doesn’t just match keywords; it comprehends the underlying intent and context of queries. For instance, if you’re researching “Java,” the system understands whether you’re interested in the programming language, the island, or the coffee based on the surrounding context and your previous interactions.
By creating this rich, interconnected web of knowledge, Semantic AI facilitates more intuitive and accurate data processing. It can identify subtle connections that might escape traditional systems, leading to more informed and contextually relevant business decisions. Think of it as having a highly knowledgeable assistant who not only remembers facts but truly understands their relationships and implications.
Enhancing Search Accuracy with Semantic AI
Search engines have evolved from merely matching keywords to understanding user intent. Traditional methods often miss the mark by focusing on exact word matches rather than the query’s meaning. For example, a search for ‘apple pie recipe without dairy’ might return articles about Apple computers or pie shops in New York City, which are irrelevant to the user’s intent.
Semantic AI changes this by analyzing the context and relationships between words. When searching for a dairy-free apple pie recipe, the AI understands you want cooking instructions, not technology or businesses. It recognizes ‘without dairy’ as a dietary restriction and adjusts results to prioritize lactose-free and vegan options.
Research has demonstrated that semantic search algorithms enhance result accuracy by interpreting the nuanced meaning behind queries. Rather than just matching terms, these systems create knowledge graphs that map relationships between concepts, ensuring more precise and relevant results.
The ability to grasp context and intent is what differentiates semantic search from traditional keyword-based search. This understanding is crucial for discerning the intent behind search terms that may have multiple meanings depending on their usage.
Dimensions AI Research Team
Semantic AI also learns and adapts from user interactions. When users searching for ‘python basics’ click on programming tutorials rather than snake care guides, the system adjusts its understanding. This continuous refinement ensures increasingly accurate search results, creating a more intuitive and efficient experience.
For businesses, this accuracy translates to reduced search time, improved customer satisfaction, and more efficient knowledge management. Users spend less time reformulating queries or sifting through irrelevant results, allowing them to focus on finding and using the information they need.
Applications of Semantic AI in Business
Semantic AI is transforming how businesses operate across multiple sectors. Unlike traditional keyword-based systems, Semantic AI brings nuanced understanding and contextual awareness to enterprise applications, making business operations more intelligent and efficient.
In customer service, Semantic AI powers sophisticated virtual assistants capable of understanding customer intent and delivering contextually relevant responses. According to Gartner, 80% of customer service organizations will adopt AI by 2025, with semantic technologies playing a crucial role in improving response accuracy and customer satisfaction. These AI-powered systems can analyze customer sentiment, interpret requests across different channels, and provide personalized support 24/7.
The healthcare sector has seen remarkable transformations through Semantic AI applications. The technology assists medical professionals by analyzing vast amounts of clinical data, research papers, and patient records to aid in diagnosis and treatment planning. For instance, hospitals using knowledge graph-backed semantic systems report significant improvements in diagnostic accuracy and patient outcomes, with AI helping to identify patterns and relationships in medical data that might otherwise go unnoticed.
In the financial sector, Semantic AI enhances decision-making processes through advanced data analysis and risk assessment. Banks and investment firms leverage this technology to analyze market trends, assess credit risks, and detect fraudulent activities in real-time. The ability to process and understand complex financial documents, regulations, and market data helps institutions make more informed decisions while ensuring compliance with regulatory requirements.
Marketing strategies have become more sophisticated with Semantic AI’s ability to understand consumer behavior and preferences at a deeper level. The technology enables hyper-personalization by analyzing customer interactions across multiple touchpoints, social media sentiment, and purchase patterns. This leads to more targeted campaigns and improved customer engagement rates, with some businesses reporting up to 25% increase in conversion rates through semantically-enhanced personalization.
What makes Semantic AI particularly powerful is its ability to learn and adapt from interactions, continuously improving its understanding of business contexts and user needs. This self-improving capability ensures that businesses can maintain competitive advantages while delivering increasingly sophisticated and personalized experiences to their customers.
Beyond direct customer interactions, Semantic AI transforms how organizations process and utilize their internal knowledge. By creating intelligent knowledge graphs, businesses can better connect disparate data sources, uncovering valuable insights that inform strategic decisions. This capability is particularly valuable in large enterprises where information silos often hinder effective decision-making.
Sector | Application | Example |
---|---|---|
Customer Service | Virtual Assistants | AI-powered chatbots providing personalized responses |
Healthcare | Clinical Documentation | NLP extracting insights from patient records |
Finance | Fraud Detection | AI algorithms identifying suspicious transactions |
Retail | Recommendation Systems | eCommerce platforms suggesting products based on user behavior |
Knowledge Management | Intelligent Search | AI understanding context to improve information retrieval |
The technology also excels at predictive analytics, helping businesses anticipate market trends and customer needs. By analyzing historical data patterns and current market conditions, Semantic AI can forecast potential outcomes with greater accuracy, enabling proactive rather than reactive business strategies.
Importantly, Semantic AI’s natural language processing capabilities enable business users to query complex data sets using conversational language, making advanced analytics accessible to non-technical staff. This democratization of data analysis empowers employees across all levels to make data-driven decisions.
As businesses continue to generate more data, Semantic AI’s role in extracting meaningful insights becomes increasingly crucial. Organizations that effectively implement these technologies are better positioned to respond to market changes, meet customer expectations, and drive innovation in their respective industries.
Benefits of Semantic AI for Enterprises
Modern enterprises grapple with an overwhelming volume of unstructured data scattered across disparate systems. Semantic AI emerges as a transformative solution, offering capabilities that change how organizations process, understand, and leverage their information assets.
One of the most compelling benefits is Semantic AI’s ability to dramatically enhance search accuracy. Unlike traditional keyword-based systems, semantic technology interprets context and meaning, enabling employees to find relevant information quickly and efficiently. For instance, when a sales representative searches for “customer churn prevention strategies,” the system understands the intent and returns contextually relevant results, even if the exact phrase isn’t present in the documents.
Data insights represent another crucial advantage of implementing Semantic AI. By understanding relationships between different data points, these systems uncover patterns and connections that might otherwise remain hidden. Financial institutions have particularly benefited from this capability, using semantic analysis to identify market trends and risk factors that traditional analytics might miss.
Business Benefit | Description |
---|---|
Improved Customer Service | Semantic AI can implement chatbots that provide fast and personalized customer service, reducing wait times and improving customer satisfaction. |
Enhanced Search Results | By analyzing the meaning and context of search queries, Semantic AI provides more accurate and relevant results. |
Improved Text Analysis and Summarization | Semantic AI can analyze and summarize large amounts of text, helping businesses quickly understand the main points and key ideas. |
Enhanced Translation | Semantic AI provides more accurate translations by understanding the meaning and context of words and phrases. |
Increased Efficiency | By automating routine tasks and improving decision-making processes, Semantic AI helps businesses streamline workflows and save time. |
Better Insights | Semantic AI extracts relevant information from unstructured data, providing better insights for informed decision-making. |
Reliability | Semantic AI improves data reliability by leveraging natural language processing and machine learning algorithms, reducing errors and bias. |
Improved Data Quality | Semantic AI enhances data quality by improving predictions and classification, resulting in more organized and accurate data. |
Increase Productivity | Semantic AI automates content tagging and classification, reducing the need for manual operations and increasing productivity. |
Enhanced Decision-Making | By analyzing data and identifying patterns, Semantic AI assists with decision-making, leading to more informed and accurate decisions. |
The technology’s impact on decision-making speed and quality cannot be overstated. When presented with complex business scenarios, Semantic AI processes vast amounts of data in real-time, providing executives with comprehensive insights for faster, more informed decisions. A manufacturing company recently reported reducing their strategic planning cycle by 40% after implementing semantic analysis tools for market and operational data.
Scalability stands out as a particularly valuable benefit for growing enterprises. As organizations expand and data volumes increase, Semantic AI systems adapt seamlessly, maintaining performance without requiring significant additional resources. This scalability ensures that investments in semantic technology continue to deliver value as businesses evolve.
Competitive advantage emerges naturally from these combined benefits. Organizations leveraging Semantic AI consistently outperform their peers in key metrics like customer satisfaction and operational efficiency. For example, an e-commerce giant attributes their 25% improvement in customer service response accuracy to their semantic AI implementation.
Semantic AI transcends traditional computing by understanding not just what words mean, but how they relate to each other and the broader world of knowledge – it’s the difference between a system that can read and one that can truly comprehend.
SmythOS Research Team
The transformative potential of Semantic AI extends beyond immediate operational improvements. By enabling more natural interactions between humans and machines, it paves the way for innovative business models and services that weren’t previously possible. This forward-looking aspect ensures that enterprises investing in semantic technology today are better positioned for tomorrow’s challenges.
Challenges and Considerations in Implementing Semantic AI
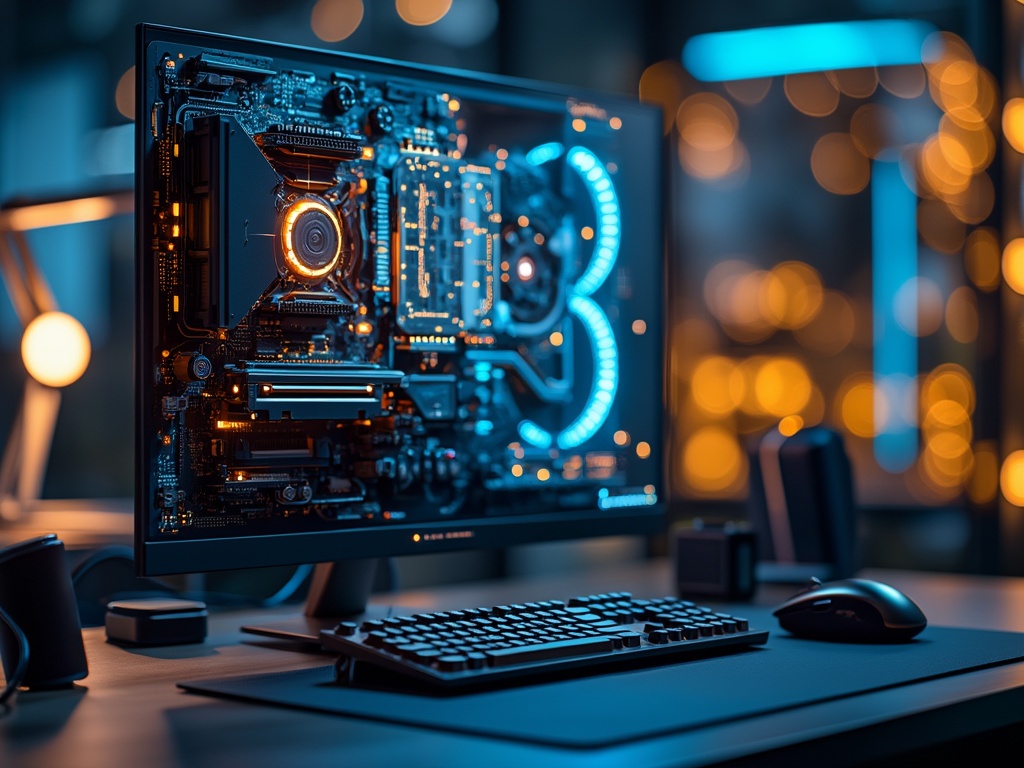
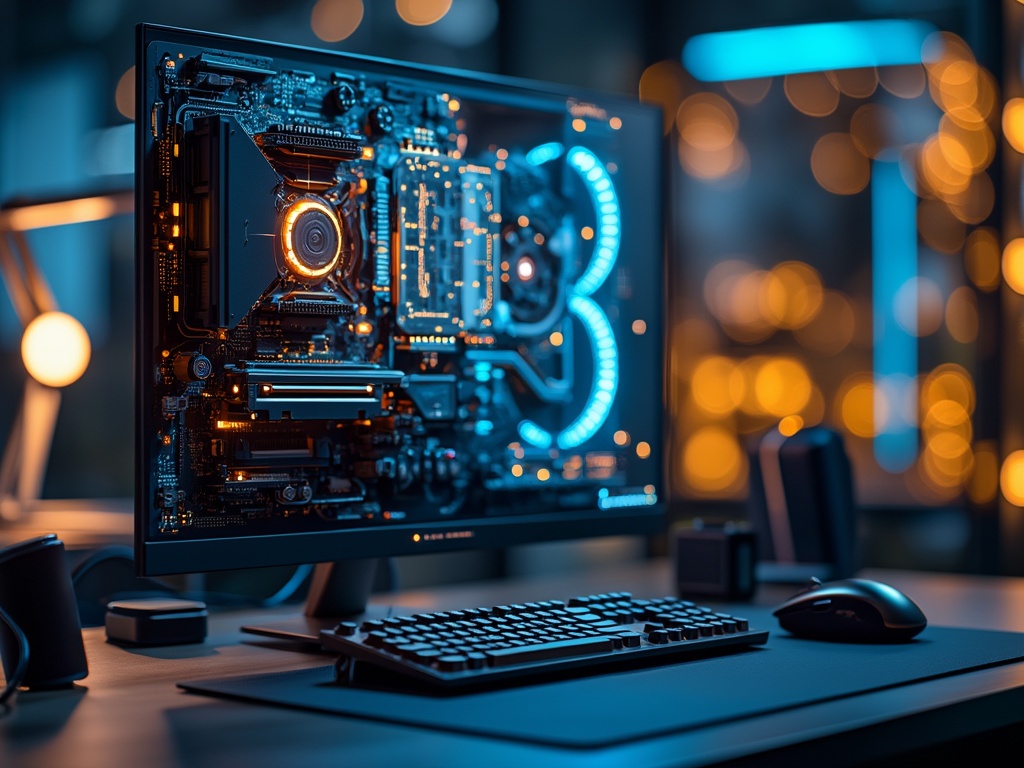
Implementing semantic AI offers transformative benefits but comes with significant challenges. Organizations face multiple hurdles when integrating semantic AI solutions into their existing infrastructure and processes.
Data quality is a fundamental challenge. Semantic AI systems require vast amounts of clean, well-structured data to function effectively. Research from the National Science Open highlights that inconsistent or incomplete data can severely impact the system’s ability to extract meaningful semantic information. Organizations need to invest in data preparation, cleaning, and ongoing quality maintenance.
Integration complexity is another significant hurdle. Existing IT infrastructures often consist of multiple legacy systems not designed with semantic technologies in mind. Connecting these systems while maintaining semantic consistency across different data sources requires sophisticated engineering work. Organizations often underestimate the technical debt and architectural changes needed to enable seamless semantic processing.
The high development costs associated with semantic AI implementation can be prohibitive. Beyond the initial investment in infrastructure and tools, organizations must account for ongoing costs related to specialized talent, system maintenance, and continuous model training. The need for semantic AI experts, who command premium salaries, adds significantly to the total cost of ownership.
Knowledge base construction and maintenance are also crucial challenges. Creating comprehensive, accurate semantic knowledge bases requires deep domain expertise and considerable time investment. These knowledge bases must be continuously updated to reflect new information and changing relationships while maintaining consistency and avoiding contradictions.
The success of semantic AI implementations heavily depends on the quality and comprehensiveness of their underlying knowledge bases. Without proper maintenance, these systems can quickly become outdated or inconsistent.
Zhang et al., Engineering 2022
Organizations can overcome these challenges through several strategies. First, adopt an incremental implementation approach, starting with well-defined use cases that demonstrate clear value. This allows for gradual scaling while managing costs and complexity. Second, invest in automated data quality tools and processes to ensure consistent, high-quality input data. Third, consider cloud-based semantic AI solutions that can reduce initial infrastructure costs and provide greater flexibility.
Success requires building cross-functional teams that combine technical expertise with domain knowledge. These teams should include data scientists, domain experts, and business analysts working collaboratively to ensure semantic AI solutions align with business objectives while maintaining technical feasibility. Regular evaluation and optimization of semantic models ensure continued accuracy and relevance as business needs evolve.
Conclusion and Future Directions
Semantic AI is at a pivotal point, set to transform how enterprises process and derive meaning from their extensive data resources. As organizations face increasingly complex decision-making scenarios, the merging of semantic technologies with quantum computing offers unprecedented potential for processing speed and analytical depth. The integration of advanced knowledge graphs with natural language processing capabilities signifies a major advancement in contextual understanding. According to recent industry analysis, two key forces are shaping the evolution of decision intelligence platforms: the fusion of natural language processing with semantic layers, and the convergence of composite AI with smart business processes.
Looking ahead, semantic AI’s impact on business intelligence will grow through enhanced multimodal capabilities. Systems will not only process text and data but also analyze complex relationships across various information types simultaneously, enabling more nuanced and accurate decision support. The trend toward smaller, specialized models democratizes access to these powerful tools, allowing organizations of all sizes to leverage semantic AI’s potential. Enterprise platforms like SmythOS are leading this transformation through visual debugging environments and robust integration capabilities. Through automated process agents and sophisticated knowledge graph management, organizations can now deploy semantic AI solutions that scale with their needs while maintaining enterprise-grade security and performance monitoring.
The future of semantic technologies promises even greater advancements in interpretability and accuracy. As these systems mature, their ability to provide transparent, contextually aware insights will become increasingly crucial for organizations seeking to maintain competitive advantages in data-driven markets. Success in this evolving landscape will depend on thoughtful implementation strategies that balance technological innovation with practical business value.
Last updated:
Disclaimer: The information presented in this article is for general informational purposes only and is provided as is. While we strive to keep the content up-to-date and accurate, we make no representations or warranties of any kind, express or implied, about the completeness, accuracy, reliability, suitability, or availability of the information contained in this article.
Any reliance you place on such information is strictly at your own risk. We reserve the right to make additions, deletions, or modifications to the contents of this article at any time without prior notice.
In no event will we be liable for any loss or damage including without limitation, indirect or consequential loss or damage, or any loss or damage whatsoever arising from loss of data, profits, or any other loss not specified herein arising out of, or in connection with, the use of this article.
Despite our best efforts, this article may contain oversights, errors, or omissions. If you notice any inaccuracies or have concerns about the content, please report them through our content feedback form. Your input helps us maintain the quality and reliability of our information.