Automated Candidate Screening in Recruitment
Approximately 72% of resumes never make it to human eyes in the current recruitment process. The flood of applications has made traditional candidate screening nearly impossible to manage effectively. Automated candidate screening harnesses AI and machine learning to transform how organizations evaluate talent.
Imagine a recruitment process where artificial intelligence sifts through thousands of applications in seconds, identifying the most promising candidates while eliminating human bias. This is not science fiction; it’s the reality of modern recruitment technology already embraced by 79% of organizations worldwide, according to recent studies.
Manually reviewing resumes and matching qualifications is rapidly becoming obsolete. Today’s AI-powered screening systems can dramatically improve recruitment results by automating arduous tasks, predicting outcomes, and analyzing data with unprecedented accuracy and speed.
This comprehensive guide explores how automated candidate screening is changing the recruitment landscape. You’ll discover the remarkable benefits this technology brings to hiring teams, from reducing time-to-hire by up to 70% to eliminating unconscious bias in the selection process. We’ll also address the key challenges organizations face when implementing these systems and provide practical insights on leveraging this technology effectively.
Whether you’re a seasoned recruiter looking to streamline your hiring process or an HR professional seeking to understand the future of talent acquisition, this article will equip you with the knowledge you need to navigate the exciting world of AI-powered candidate screening.
Benefits of Automated Candidate Screening
The traditional manual approach to candidate screening has become increasingly unsustainable. HR teams spend an average of 23 hours vetting candidates for a single position, time that could be better spent on strategic hiring decisions. Automated screening systems are transforming this reality, offering significant efficiency gains for modern recruitment.
One of the most compelling advantages is the dramatic reduction in time-to-hire. According to research, organizations utilizing AI-powered screening can process thousands of applications in mere minutes, compared to the days or weeks required for manual review. This acceleration enables recruiters to identify and engage top talent before competitors quickly.
Equally important is automated screening’s ability to enhance objectivity in the hiring process. By evaluating candidates based on predetermined criteria and qualifications, these systems help eliminate the unconscious biases that often creep into human decision-making. Rather than being influenced by factors like a candidate’s name, gender, or educational background, automated tools focus purely on skills and experience relevant to the role.
The consistency achieved through automation is remarkable. Every application receives the same thorough evaluation against standardized criteria, creating a truly level playing field. This standardization not only promotes fairness but also improves the quality of hiring decisions by ensuring no qualified candidates slip through the cracks due to reviewer fatigue or oversight.
These automated systems can handle large volumes of applications consistently, ensuring that no potential candidate is overlooked. This scalability is particularly beneficial for organizations that receive a high volume of applications or are in the midst of rapid growth.
Elizabeth Parker, HireBee
Beyond efficiency and fairness, automated screening frees up valuable recruiter time for high-value activities like conducting in-depth interviews, building relationships with top candidates, and focusing on strategic hiring initiatives. Rather than getting bogged down in administrative tasks, talent acquisition teams can dedicate their expertise to making nuanced assessments of cultural fit and potential, elements that truly require human judgment.
The data-driven insights provided by automated screening systems also enable continuous improvement of the hiring process. Organizations can track key metrics, identify bottlenecks, and refine their screening criteria based on actual hiring outcomes. This analytical capability helps companies build increasingly sophisticated and effective recruitment strategies over time.
Challenges Addressed by Automated Candidate Screening
Modern recruiters face a relentless avalanche of resumes. Online job platforms and one-click applications often lead to hundreds, sometimes thousands, of applications for a single position. HR professionals spend an average of 23 hours screening candidates for just one role, time that could be better spent on strategic hiring decisions.
The most pressing challenge automated screening tackles is the sheer volume of applications. Traditional manual screening methods can’t keep pace with today’s application volumes. Overwhelmed recruiters often resort to quick-scanning techniques that can overlook qualified candidates. Studies show that up to 75% of applicants may be unqualified for a given position, making the task of finding the right candidate even more daunting.
Unconscious bias presents another significant hurdle in the recruitment process. Even well-intentioned hiring managers can be influenced by subtle prejudices about age, gender, ethnicity, or educational background. These biases often manifest in split-second decisions during resume screening, potentially excluding talented candidates before they ever get a chance to prove their worth.
Time management poses yet another critical challenge. The repetitive nature of manual screening consumes valuable hours and leads to mental fatigue. As fatigue sets in, the quality of screening decisions tends to deteriorate, potentially allowing excellent candidates to slip through the cracks while advancing less qualified ones.
Consistency in evaluation criteria is a persistent challenge in traditional screening methods. Different recruiters may apply varying standards when reviewing applications, leading to inconsistent outcomes. This lack of standardization can result in qualified candidates being evaluated differently depending on who reviews their application and when.
Automated screening systems address these challenges by processing applications systematically and objectively. Using advanced algorithms, these systems can evaluate thousands of resumes against standardized criteria in a fraction of the time it would take a human recruiter. They maintain consistent evaluation standards throughout the process, ensuring every candidate receives fair consideration regardless of when their application is reviewed.
How to Implement Automated Candidate Screening
Implementing AI-powered candidate screening transforms your recruitment process from a manual bottleneck into a streamlined operation. Automated screening can dramatically reduce time-to-hire while surfacing the most qualified candidates. The first critical step is selecting the right screening software for your organization’s needs. Look for solutions that offer seamless integration capabilities with your existing applicant tracking system (ATS) and other recruitment tools. As research shows, successful implementation requires careful integration of AI screening models into your existing recruitment workflow.
Once you’ve selected your software, focus on properly formatting job descriptions to optimize screening accuracy. Each job posting should clearly outline required skills, qualifications, and experience using consistent terminology. This standardization helps the AI algorithms better match candidates to opportunities. Consider breaking down complex requirements into discrete, measurable criteria that the system can evaluate objectively.
The next phase involves training the AI models with high-quality historical data. Feed the system examples of successful past hires and their resumes, along with information about candidates who weren’t a good fit. This helps the AI learn your organization’s specific needs and preferences.
Source | Training Data Usage | Benefits | Challenges |
---|---|---|---|
Obviously AI | 80% of data for training, 20% for testing | Improves model accuracy | Requires large amounts of data |
Shaip | Annotated data for supervised learning | Ensures high-quality training data | Time-consuming data labeling process |
G2 | Training data for model fitting | Helps models learn patterns | Quality of data is crucial for success |
CloudFactory | Labeled data for supervised learning | Improves prediction accuracy | Requires continuous data quality checks |
Remember that the quality of the training data directly impacts the effectiveness of the screening process. Setting up automated workflows is another crucial implementation step. Configure the system to automatically screen incoming applications against your predetermined criteria. Define clear rules for how candidates should be scored and ranked, and establish thresholds for automatic advancement or rejection. However, maintain human oversight of these decisions, especially in the early stages. Regular monitoring and refinement of the screening parameters ensures optimal performance. Track key metrics like time-to-hire, quality of candidates advanced, and hiring manager satisfaction. Use these insights to continuously fine-tune the screening criteria and algorithms. Implementation is an iterative process – expect to make adjustments as you learn what works best for your organization.
Case Studies: Success of Automated Candidate Screening
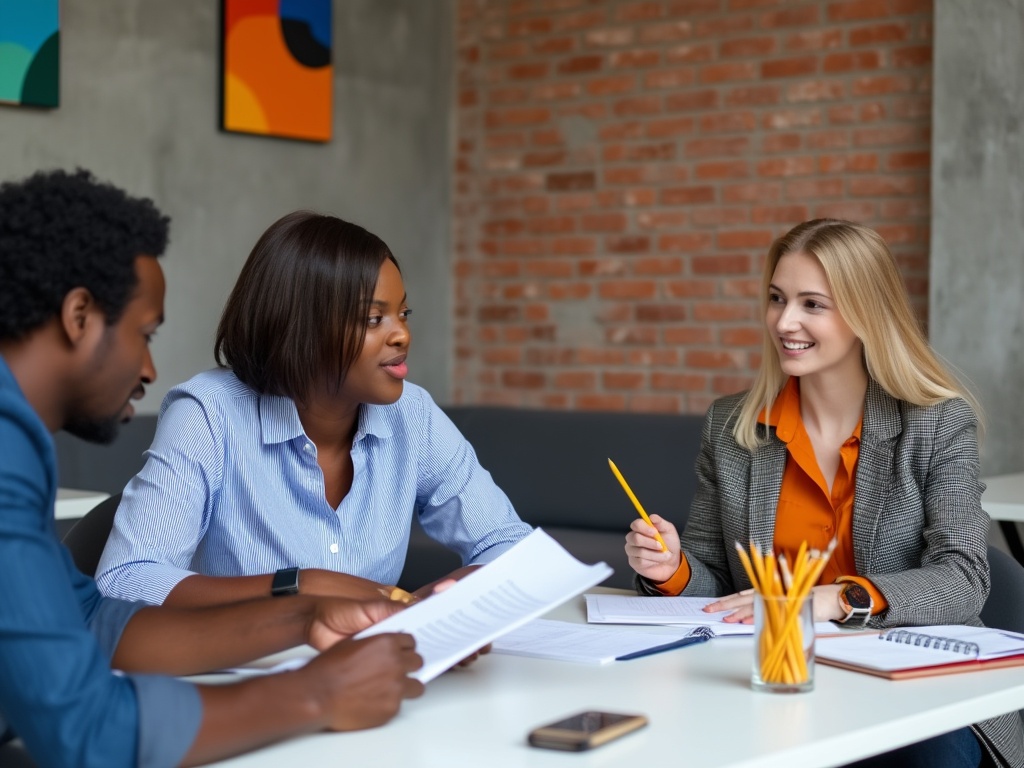
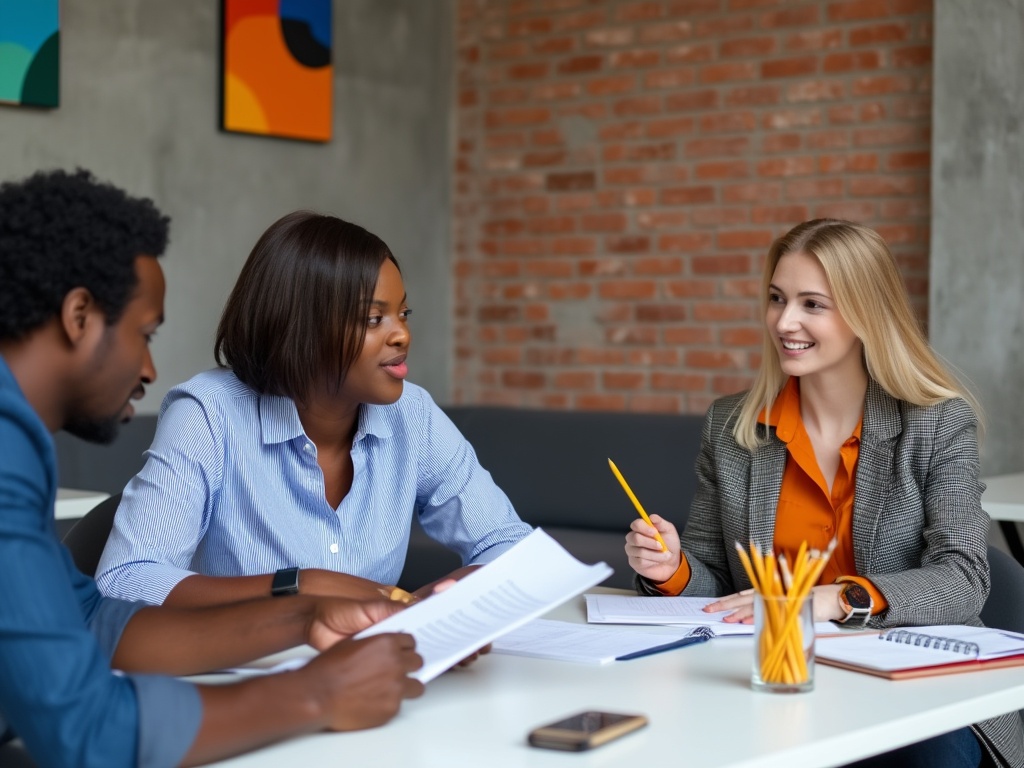
Leading organizations have transformed their hiring processes through AI-powered candidate screening, achieving remarkable results in efficiency and talent quality. Here are some compelling success stories that demonstrate the real-world impact of automated screening solutions.
One standout example comes from a major healthcare system that slashed their interview scheduling time by 86%, reducing it from 7 days to just 24 hours through automated screening and scheduling tools. This dramatic improvement allowed them to secure top candidates before competitors could act.
Scaling Success for High-Growth Companies
A rapidly expanding startup faced the challenge of doubling their workforce while maintaining rigorous hiring standards. By implementing AI-powered screening tools, they automated resume analysis and candidate ranking, enabling their recruitment team to focus on evaluating the most promising applicants.
The results proved transformative. Within six months, they successfully doubled their headcount while reducing time-to-hire by 40%. Most importantly, the automated screening maintained consistent quality standards despite the increased hiring volume.
Their automated platform screened resumes against custom criteria, ranked candidates based on fit, and even predicted potential success using machine learning algorithms trained on historical hiring data. This data-driven approach empowered recruiters to make faster, more informed decisions.
Metric | Definition | Industry Benchmark |
---|---|---|
Time to Screen Resumes | Measures the duration it takes for recruiters to review and evaluate resumes from the moment they are received. | 30 to 60 minutes per resume; reduced to 10 to 15 minutes with AI |
Percentage of Automated Screens | Measures the proportion of resumes screened through automation compared to the total number of resumes submitted. | 70% or higher |
Candidate Quality Score | Evaluates the overall suitability of candidates based on their resumes and application materials. | Varies by sector |
Interview-to-Hire Ratio | Represents the number of candidates interviewed compared to the number of hires made. | 3:1; can drop to 5:1 in specialized fields |
Diversity of Candidates | Refers to the representation of various demographic groups within the applicant pool. | 30% representation of underrepresented groups within five years |
Cost per Hire | Quantifies the total expenses incurred in the recruitment process for filling a job vacancy. | $3,000 to $5,000; can exceed $10,000 in specialized fields |
User Satisfaction Rate | Measures the satisfaction level of users with the automated screening process. | 75% or higher; 80% to 90% for high-performing organizations |
Accuracy of Screening Algorithm | Refers to how accurately the AI-powered resume screening system identifies suitable candidates. | 85% to 95% |
Time Saved by Automation | Refers to the reduction in hours spent on reviewing resumes manually, achieved through automated processes. | 20-50% time saved |
Enhancing Diversity Through Unbiased Screening
A multinational corporation leveraged AI screening to tackle unconscious bias in their hiring process. Their automated solution anonymized candidate profiles by removing identifying information like names, photos, and educational institutions, ensuring evaluation focused purely on skills and experience.
The impact was significant. They achieved a 25% increase in workforce diversity among new hires. The AI screening tool also analyzed job descriptions to eliminate potentially biased language that could deter diverse candidates from applying.
The integration of AI has transformed our ability to identify top talent efficiently while ensuring a fair and consistent evaluation process for all candidates.
HR Director at a Fortune 500 Company
Beyond the numbers, the automated screening led to higher quality hires by standardizing evaluation criteria and ensuring no promising candidates were overlooked due to human oversight or bias. The system’s ability to process applications 24/7 also improved the candidate experience by providing faster responses and feedback.
These success stories demonstrate how automated candidate screening, when thoughtfully implemented, can deliver measurable improvements in hiring efficiency, quality, and diversity. As AI technology continues advancing, we can expect even more innovative applications that further enhance the recruitment process.
Conclusion and Future of Automated Candidate Screening
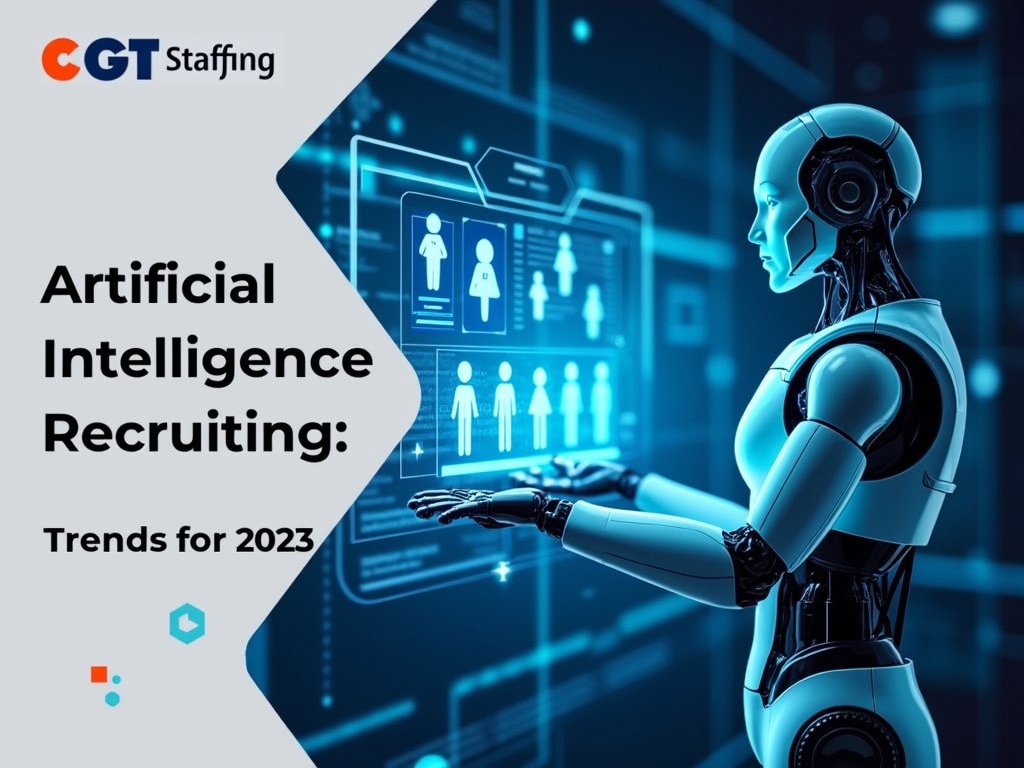
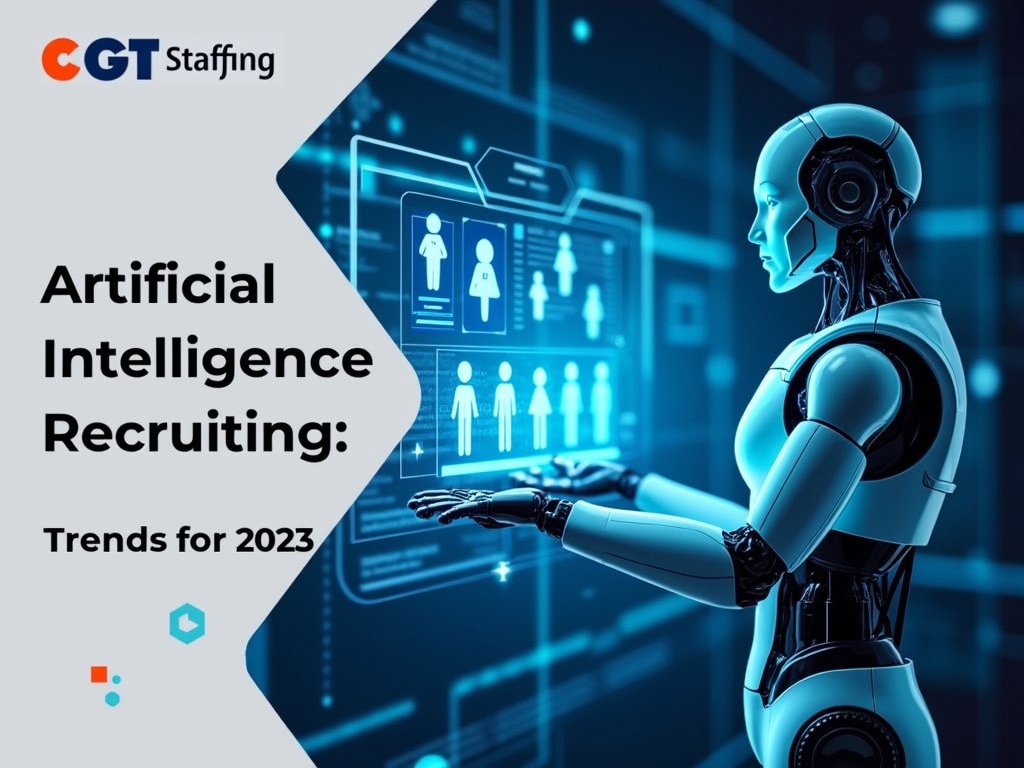
Futuristic AI recruitment trends for 2023.
The landscape of recruitment has dramatically transformed through AI-powered candidate screening, delivering unprecedented efficiency and objectivity to hiring processes. As highlighted by recent industry studies, organizations implementing AI screening tools have seen their time-to-hire reduced by up to 35%, while improving the quality of their candidate selections.
The future of automated screening looks promising as AI technology evolves. Advanced algorithms are becoming increasingly sophisticated at analyzing candidate data, moving beyond basic keyword matching to understand complex patterns in candidate behaviors and qualifications. These improvements make it possible to identify high-potential candidates with greater accuracy than ever before.
We can expect to see more nuanced applications of AI in candidate screening, particularly in areas like behavioral assessment and cultural fit analysis. Machine learning models are being refined to better understand subtle indicators of candidate success, while natural language processing capabilities advance to provide deeper insights into communication styles and soft skills.
It’s crucial to acknowledge that the future of automated screening isn’t just about technological advancement—it’s about balancing AI efficiency with human insight. While AI excels at processing vast amounts of data and identifying patterns, the human element remains vital for making nuanced hiring decisions that consider factors like team dynamics and organizational culture.
As we move forward, the integration of advanced AI capabilities with human expertise will become increasingly seamless, creating a more holistic and effective hiring process. This evolution will enable organizations to screen candidates more efficiently and make more informed decisions that lead to better hiring outcomes and stronger teams.
Last updated:
Disclaimer: The information presented in this article is for general informational purposes only and is provided as is. While we strive to keep the content up-to-date and accurate, we make no representations or warranties of any kind, express or implied, about the completeness, accuracy, reliability, suitability, or availability of the information contained in this article.
Any reliance you place on such information is strictly at your own risk. We reserve the right to make additions, deletions, or modifications to the contents of this article at any time without prior notice.
In no event will we be liable for any loss or damage including without limitation, indirect or consequential loss or damage, or any loss or damage whatsoever arising from loss of data, profits, or any other loss not specified herein arising out of, or in connection with, the use of this article.
Despite our best efforts, this article may contain oversights, errors, or omissions. If you notice any inaccuracies or have concerns about the content, please report them through our content feedback form. Your input helps us maintain the quality and reliability of our information.