How Symbolic AI is Transforming Legal Practice: A Guide for Modern Law Firms
Symbolic AI is transforming how legal practitioners approach their work. This sophisticated technology, blending rule-based reasoning with neural networks, promises to transform everything from contract analysis to case research.
Nearly 92% of impoverished Americans cannot afford legal representation for civil suits. Yet as reported by Forbes, symbolic AI’s ability to combine traditional logic-based systems with advanced neural networks is opening new frontiers in legal accessibility and efficiency.
Much like a seasoned attorney who draws upon both established precedents and nuanced interpretation, symbolic AI brings together the best of both worlds. It harnesses the structured, rule-following nature of traditional legal reasoning while incorporating the pattern-recognition capabilities that make modern AI so powerful.
Through this powerful combination, legal professionals can now tackle complex challenges with unprecedented accuracy and speed. Whether analyzing vast databases of case law or identifying subtle patterns in legal documents, symbolic AI serves as an invaluable partner in the pursuit of justice.
This article explores how symbolic AI is reshaping legal practice, from streamlining routine tasks to enhancing decision-making processes. We examine its practical applications, benefits, and the transformative impact it’s having on legal efficiency and accuracy.
Understanding Symbolic AI Components
Symbolic AI represents a fascinating marriage between traditional rule-based systems and modern neural approaches. At its core, this technology operates like a highly sophisticated legal mind, processing both concrete rules and nuanced interpretations simultaneously.
Rule-based systems form the logical backbone of symbolic AI, excelling at handling well-structured knowledge. These systems operate much like a meticulous lawyer consulting a legal codebook, systematically applying predefined rules, laws, and statutes to reach clear conclusions. For instance, when analyzing contractual requirements or regulatory compliance, rule-based components apply explicit logical frameworks to make precise determinations.
However, the real power of symbolic AI emerges through its integration with neural networks. These neural components act as the intuitive counterpart, processing unstructured data with remarkable flexibility. Much like how experienced legal professionals develop an intuition for complex cases, neural networks excel at recognizing patterns in messy, real-world data that doesn’t fit neatly into predefined categories.
Consider how a symbolic AI system processes information in a legal context: while the rule-based component might strictly interpret statutory requirements, the neural network component can analyze contextual factors like case precedents, judicial opinions, and evolving legal interpretations. This dual approach enables more comprehensive and nuanced decision-making.
The synergy between these components creates a system greater than the sum of its parts. Rule-based systems provide the necessary structure and logical rigor, while neural networks add adaptability and pattern recognition capabilities. This combination proves particularly valuable in complex scenarios where both strict rule adherence and flexible interpretation are crucial.
To build a robust, knowledge-driven approach to AI we must have the machinery of symbol-manipulation in our toolkit. Too much useful knowledge is abstract to proceed without tools that represent and manipulate abstraction.
Gary Marcus, AI Researcher
This hybrid approach allows symbolic AI to handle both the black-and-white aspects of structured data and the numerous shades of gray present in real-world applications. By leveraging both rule-based precision and neural network adaptability, symbolic AI creates more robust and practical solutions for complex decision-making scenarios.
Applications of Symbolic AI in Law
Symbolic AI is transforming the legal profession by bringing precision and efficiency to complex legal tasks. Unlike traditional keyword-based systems, symbolic AI employs sophisticated logical reasoning to understand and analyze legal documents with remarkable accuracy.
In contract analysis, symbolic AI shows particular prowess. Recent developments indicate that these systems can dissect complex contract structures, identify potential legal conflicts, and suggest strategic optimizations by understanding the underlying logic and interdependencies between different clauses. This ensures that contracts remain compliant with legal requirements and aligned with business objectives.
Legal research, traditionally a time-intensive process, has been transformed by symbolic AI’s ability to parse vast databases of case law and statutes. The technology goes beyond simple pattern matching, employing logical reasoning to understand the context and nuances of legal precedents. This helps lawyers identify relevant cases and legal principles more efficiently while maintaining the high level of accuracy required in legal work.
Symbolic AI excels in precedent analysis by combining rule-based reasoning with deep learning capabilities. This hybrid approach allows the system to grasp subtle legal principles within case law and predict potential outcomes based on historical patterns. For instance, when analyzing past rulings, the AI can identify not just similar cases, but also understand the underlying legal reasoning that led to specific decisions.
Application | Traditional AI | Neuro-Symbolic AI |
---|---|---|
Contract Analysis | Limited to keyword searches and static-rule applications | Dissects complex structures, identifies conflicts, and suggests optimizations |
Legal Research | Time-intensive, relies on keyword matching | Efficiently parses databases, understands context and nuances |
Precedent Analysis | Basic pattern matching | Combines rule-based reasoning with deep learning for nuanced predictions |
Decision-Making | Rigid, rule-based | Integrates logical consistency with flexible interpretation |
The integration of logical reasoning with deep learning technologies has proven valuable in ensuring consistency across legal interpretations. While human lawyers provide essential expertise and judgment, symbolic AI enhances their capabilities, enabling them to work more efficiently while maintaining high standards of accuracy.
Challenges in Implementing Symbolic AI
Implementing symbolic AI systems presents several formidable challenges for organizations. Data biases are a critical concern, as symbolic AI systems can inadvertently perpetuate or amplify existing prejudices in training data. According to recent research from Neural Computing and Applications, these biases can lead to unfair outcomes in areas like hiring, lending, and healthcare decisions.
Integration with existing technology infrastructure is another significant hurdle. Legacy systems often lack the flexibility and architectural components needed to support symbolic reasoning engines. This integration challenge frequently requires organizations to undertake expensive and time-consuming system modernization efforts while maintaining operational continuity.
The complexity of symbolic AI implementations demands extensive interdisciplinary collaboration. Computer scientists must work alongside domain specialists, ethicists, and business stakeholders to ensure these systems are both technically sound and practically applicable. This collaboration, while essential, often introduces additional coordination challenges and can extend development timelines.
Resource constraints present yet another obstacle. Organizations frequently underestimate the computational power required for effective symbolic reasoning, particularly with large-scale knowledge bases. The processing demands can strain existing infrastructure and require significant investments in hardware and optimization techniques.
While symbolic AI holds immense potential for enhancing machine reasoning capabilities, its successful implementation requires careful consideration of these challenges and a commitment to addressing them systematically.
Neural Computing and Applications, 2024
Data quality and knowledge representation also emerge as persistent challenges. Creating and maintaining accurate, comprehensive knowledge bases requires significant human expertise and ongoing curation. Organizations must establish robust processes for validating and updating symbolic representations while ensuring consistency across different components of the system.
The Future of Symbolic AI in Legal Fields
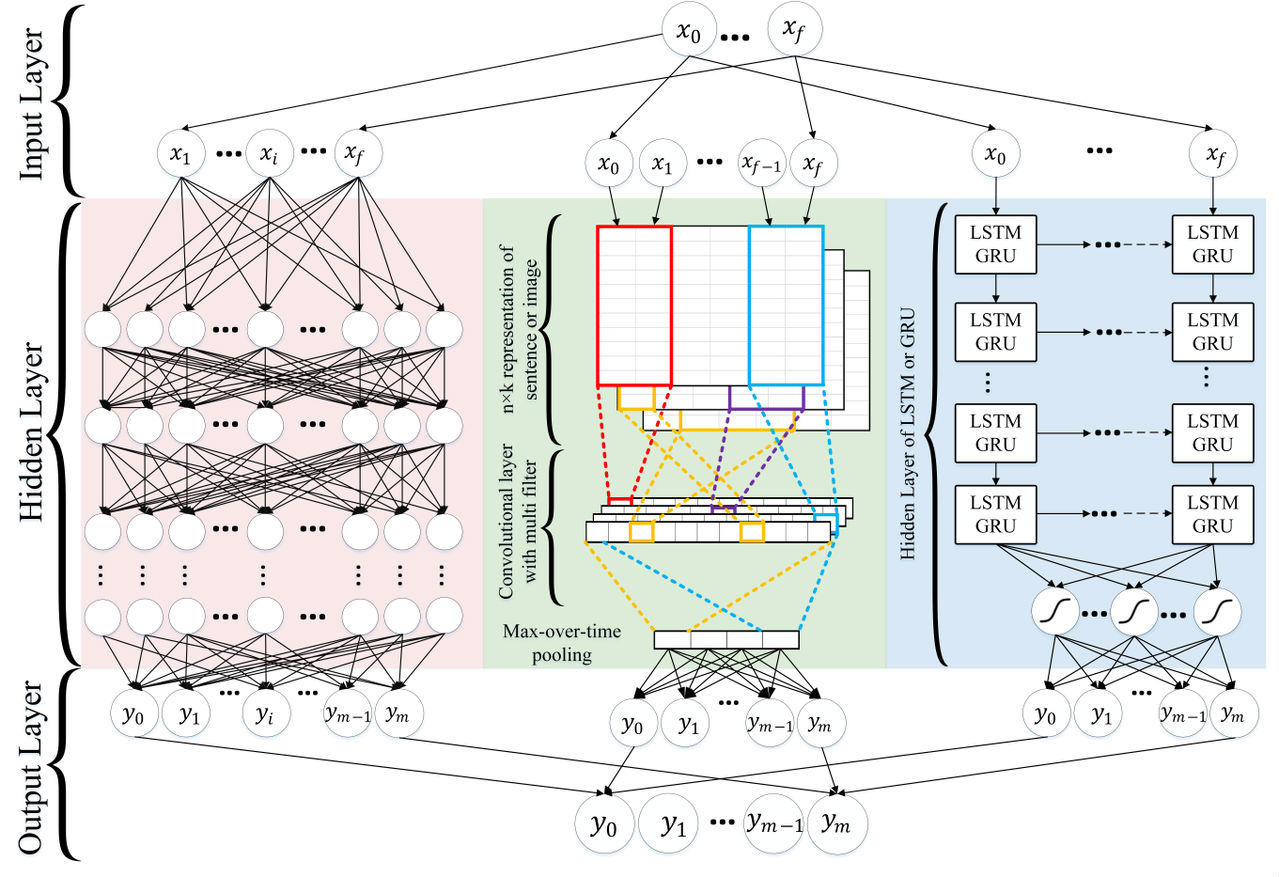
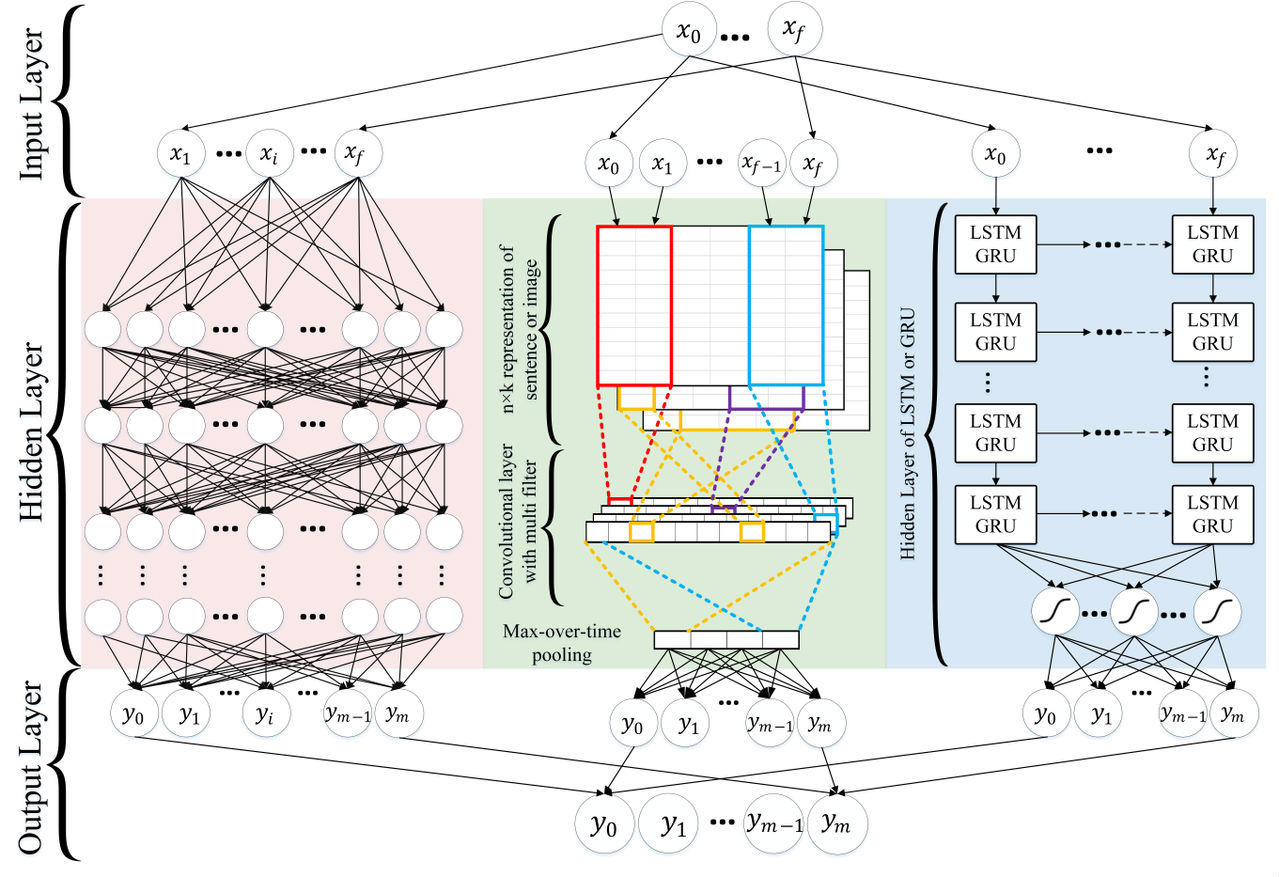
The legal landscape is on the verge of transformation as neuro-symbolic AI emerges as a groundbreaking force, combining the precision of traditional rule-based systems with the adaptability of neural networks. This hybrid approach promises to change how legal professionals analyze cases and develop strategies.
Unlike conventional legal AI tools that rely solely on keyword matching, neuro-symbolic systems can grasp the nuanced context of legal arguments. These advanced systems excel at dissecting complex contract structures, identifying potential conflicts, and suggesting strategic optimizations by understanding the underlying logic and interdependencies of legal documents. The technology’s ability to navigate intricate legal spaces while maintaining logical consistency sets it apart from previous innovations.
The impact on legal strategy development is particularly noteworthy. Drawing on techniques refined in geometric reasoning, these systems can analyze vast amounts of case law with unprecedented depth and precision. They don’t just process information; they understand legal principles and their contextual applications, enabling lawyers to develop more sophisticated and nuanced legal arguments.
Legal precedent analysis stands to gain significantly from this technological evolution. Modern neuro-symbolic systems can delve beyond surface-level text analysis, employing a deductive approach that mirrors human legal reasoning. This capability allows for a deeper understanding of case law contexts and subtleties, leading to more accurate outcome predictions and strategic insights.
Aspect | Traditional AI | Neuro-Symbolic AI |
---|---|---|
Reasoning Approach | Relies on predefined rules or learned patterns from data | Combines rule-based reasoning with neural network learning |
Adaptability | Limited adaptability in ambiguous scenarios | High adaptability due to integration of neural networks |
Performance in Legal Tasks | Effective in structured environments with clear rules | More nuanced approach, capable of understanding complex legal structures |
Data Requirements | Requires large amounts of labeled data | Can work with limited data due to symbolic reasoning |
Explainability | Often seen as a ‘black box’ | More transparent due to logical reasoning components |
Applications | Basic contract analysis, legal research | Advanced contract analysis, precedent analysis, strategic legal planning |
The transformation extends beyond just analytical capabilities. As these systems become more sophisticated, they’re beginning to demonstrate an ability to assist in crafting legal strategies that are both technically sound and contextually aware. This advancement suggests a future where legal professionals can leverage AI not just for research, but as a collaborative tool in developing comprehensive legal strategies.
Leveraging SmythOS for Advanced Legal AI Solutions
The legal profession demands sophisticated AI tools that can handle both intuitive pattern recognition and rigorous logical analysis. SmythOS rises to this challenge by seamlessly integrating hybrid symbolic-neural approaches, offering legal professionals a powerful platform for developing advanced AI solutions.
At the core of SmythOS’s legal capabilities is its innovative visual representation system for logic rules. Legal professionals can map out complex legal reasoning patterns and decision frameworks through an intuitive drag-and-drop interface. This visual approach makes it easier for law firms to translate intricate legal principles into actionable AI workflows without requiring deep technical expertise.
The platform’s built-in debugging capabilities for logic flows set it apart in the legal technology landscape. Unlike traditional “black box” AI systems that obscure their decision-making processes, SmythOS provides transparent insight into how its AI agents process legal information and arrive at conclusions. This transparency is crucial for maintaining accountability and ensuring compliance with legal ethics requirements.
Recent developments in neuro-symbolic AI have shown that combining symbolic reasoning with neural networks can dramatically improve legal analysis. SmythOS leverages this hybrid approach, allowing legal AI systems to handle both structured rule-based reasoning for clear-cut legal principles and adaptive learning for complex case analysis.
The platform’s hybrid architecture enables legal teams to develop AI solutions that can tackle diverse tasks – from automated contract analysis and compliance monitoring to legal research and case prediction. By integrating both symbolic and neural capabilities, SmythOS-powered legal AI can identify subtle patterns in case law while strictly adhering to established legal frameworks and precedents.
For law firms concerned about accuracy and reliability, SmythOS offers comprehensive monitoring tools that provide continuous visibility into AI operations. This allows legal teams to track performance metrics and decision outputs in real-time, ensuring their AI solutions maintain the high standards of precision required in legal practice.
Conclusion: The Synergy of Human and AI in Law
The integration of AI and human expertise in legal practice represents a significant moment for the profession. According to a recent Thomson Reuters report, nearly 80% of law firm leaders recognize AI’s transformative potential in the next five years, highlighting a shift in how legal services will be delivered. This technological evolution enhances rather than replaces human capabilities, creating a powerful synergy that drives more precise and efficient outcomes.
Legal professionals who combine their expertise with AI tools consistently demonstrate improved accuracy and productivity. In document review processes, while AI systems maintain unwavering attention across thousands of pages, human lawyers provide the crucial judgment and contextual understanding needed for complex legal interpretation. This partnership has shown remarkable results, with some firms reporting up to 50% reduction in document preparation time while maintaining high accuracy standards.
The role of platforms like SmythOS in developing transparent, robust AI solutions cannot be overstated. By providing visual debugging environments and enterprise-grade security controls, SmythOS enables legal teams to create and deploy AI tools that augment their capabilities while maintaining high standards of data protection and ethical compliance.
The success of legal practice will increasingly depend on this harmonious blend of human insight and artificial intelligence. The firms that thrive will be those that effectively leverage AI’s computational power while preserving the irreplaceable elements of human legal expertise—emotional intelligence, ethical reasoning, and strategic thinking. This synergy promises not just increased efficiency, but a fundamental enhancement in how justice is served and legal services are delivered.
The future of law lies not in choosing between human expertise and artificial intelligence, but in their thoughtful integration. As this symbiotic relationship continues to evolve, it paves the way for a more accessible, efficient, and equitable legal system that serves both practitioners and clients better than ever before.
Last updated:
Disclaimer: The information presented in this article is for general informational purposes only and is provided as is. While we strive to keep the content up-to-date and accurate, we make no representations or warranties of any kind, express or implied, about the completeness, accuracy, reliability, suitability, or availability of the information contained in this article.
Any reliance you place on such information is strictly at your own risk. We reserve the right to make additions, deletions, or modifications to the contents of this article at any time without prior notice.
In no event will we be liable for any loss or damage including without limitation, indirect or consequential loss or damage, or any loss or damage whatsoever arising from loss of data, profits, or any other loss not specified herein arising out of, or in connection with, the use of this article.
Despite our best efforts, this article may contain oversights, errors, or omissions. If you notice any inaccuracies or have concerns about the content, please report them through our content feedback form. Your input helps us maintain the quality and reliability of our information.