Symbolic AI in Healthcare
Healthcare decisions can mean the difference between life and death. Leading medical institutions are increasingly turning to symbolic AI—a powerful form of artificial intelligence that mimics human expertise through explicit rules and logic—to transform how they diagnose, treat, and care for patients.
Unlike ‘black box’ machine learning systems, symbolic AI provides transparent reasoning that doctors can verify and trust. By encoding medical knowledge and protocols into rule-based frameworks, these systems act as tireless digital assistants, helping clinicians analyze symptoms, recommend treatments, and catch potential errors that busy human doctors might miss.
The impact is already evident across healthcare facilities. At major hospitals, symbolic AI analyzes thousands of patient records to identify disease patterns. In research labs, it helps develop personalized treatment plans by logically evaluating drug interactions. In doctors’ offices, it provides explainable, evidence-based suggestions that physicians can confidently incorporate into their decision-making.
As recent research in neurosymbolic approaches demonstrates, symbolic AI brings unique advantages to healthcare through its ability to handle complex medical rules while maintaining transparency in its reasoning process. For technical leaders and developers implementing these systems, understanding how to effectively deploy symbolic reasoning in healthcare AI has become crucial.
This article will explore how symbolic AI is enhancing diagnosis accuracy, optimizing treatment planning, and improving data management. Whether you’re building clinical decision support tools or managing healthcare AI infrastructure, you’ll discover practical insights for leveraging rule-based AI to create more reliable and explainable healthcare solutions.
Enhancing Medical Diagnosis with Symbolic AI
Symbolic AI is transforming how doctors diagnose diseases by combining the power of logical reasoning with advanced data analysis. Unlike traditional AI that works like a black box, symbolic AI makes decisions we can actually understand and trace, much like how a doctor thinks through a diagnosis step by step. One of the most impressive capabilities of symbolic AI is its ability to process vast amounts of medical data while following clear diagnostic rules.
For example, when analyzing blood test results, symbolic AI can identify patterns and relationships between different markers, similar to how an experienced doctor connects various symptoms to reach a diagnosis.
The power of symbolic AI lies in its ability to mimic expert medical reasoning. Just as doctors use their knowledge of symptoms, test results, and patient history to make diagnoses, symbolic AI systems use predefined rules and logical connections to analyze patient data. This structured approach helps reduce diagnostic errors by ensuring important indicators aren’t overlooked. Perhaps most importantly, symbolic AI provides clear explanations for its diagnostic suggestions.
When the system flags a potential diagnosis, it can show exactly which factors led to that conclusion. This transparency helps doctors validate the AI’s reasoning and make more informed decisions about patient care.
The impact of symbolic AI extends beyond individual diagnoses. By processing data from thousands of patient cases, these systems can identify subtle patterns that might escape even experienced physicians. These insights can lead to earlier disease detection and more accurate diagnoses, ultimately improving patient outcomes. The combination of symbolic AI with medical expertise creates a powerful tool for enhancing diagnostic accuracy while maintaining the critical element of human oversight in healthcare decisions.
As medical knowledge continues to expand, symbolic AI helps doctors stay current with the latest diagnostic criteria while ensuring decisions remain grounded in established medical principles. This balance of innovation and reliability makes symbolic AI an invaluable partner in modern healthcare delivery.
Applications in Drug Repurposing
Drug repurposing has emerged as an innovative approach in pharmaceutical development, where existing medications find new therapeutic purposes through symbolic AI systems. This strategy leverages advanced knowledge graphs and symbolic reasoning to accelerate the traditionally lengthy and costly drug discovery process.
A recent pilot study at Vanderbilt University Medical Center demonstrated the potential of AI-driven drug repurposing, particularly in addressing complex conditions like Alzheimer’s disease. The research revealed that medications like metformin, simvastatin, and losartan—originally developed for different conditions—showed promising potential for Alzheimer’s treatment when identified through AI analysis.
Knowledge graphs serve as the backbone of successful AI-assisted drug repurposing by creating intricate networks of relationships between drugs, diseases, genes, and biological pathways. These complex webs of information enable symbolic AI systems to identify hidden patterns and potential therapeutic applications that might otherwise remain undiscovered through traditional research methods.
Symbolic AI’s strength in drug repurposing lies in its ability to provide explainable reasoning paths—a crucial feature for healthcare applications. Unlike black-box AI models, symbolic systems can trace and justify their suggestions through logical steps, making their recommendations more trustworthy and actionable for medical researchers.
Drug repurposing represents an attractive alternative to the costly and time-consuming process of new drug development, particularly for serious, widespread conditions with limited effective treatments
Wei-Qi Wei, MD, PhD, Associate Professor of Biomedical Informatics
The integration of symbolic AI with knowledge management strategies has proven particularly effective in addressing data-related challenges in drug repurposing. By combining structured biomedical knowledge with symbolic reasoning capabilities, researchers can more efficiently navigate through vast amounts of scientific literature and clinical data to identify promising drug candidates.
Original Drug | Initial Use | Repurposed Use |
---|---|---|
Metformin | Type 2 Diabetes | Various Cancers |
Sildenafil Citrate | Angina | Erectile Dysfunction |
Thalidomide | Morning Sickness | Erythema Nodosum Leprosum (ENL), Multiple Myeloma |
Simvastatin | High Cholesterol | Alzheimer’s Disease |
Losartan | Hypertension | Alzheimer’s Disease |
Explainable AI in Clinical Settings
Doctors and medical professionals increasingly rely on artificial intelligence to make critical decisions about patient care. For AI systems to be valuable and trustworthy in clinical settings, healthcare providers need to understand how these systems arrive at their recommendations. This is where explainable AI (XAI) plays a vital role.
When doctors use AI to help diagnose conditions or recommend treatments, they need more than just a final answer. They need to understand the reasoning behind it. According to research published in BMC Medical Informatics and Decision Making, explainable AI enables healthcare providers to evaluate AI recommendations based on their clinical experience and judgment, allowing them to make informed decisions about whether to rely on the system’s suggestions.
Symbolic AI offers a particularly promising approach for achieving transparency in healthcare AI systems. Unlike “black box” AI models that obscure their decision-making process, symbolic AI uses clear rules and logic that mirror human reasoning. This makes it easier for healthcare professionals to follow the AI’s chain of reasoning and verify that it aligns with established medical knowledge and practices.
The benefits of explainable AI in clinical settings extend beyond just building trust. When doctors can understand how an AI system reaches its conclusions, they can better identify potential biases or errors in the system’s reasoning. This is especially crucial in healthcare, where decisions can have life-or-death consequences. Additionally, explainability helps healthcare providers explain AI-assisted decisions to their patients, maintaining transparency in the doctor-patient relationship.
If used appropriately, explainable AI decision support systems may not only contribute to patients feeling more knowledgeable and better informed but could also promote more accurate risk perceptions.
BMC Medical Informatics and Decision Making
The continued development of explainable AI solutions in healthcare promises to create more collaborative relationships between medical professionals and AI systems. Rather than replacing human judgment, these transparent AI tools serve as valuable aids that augment and support clinical decision-making while maintaining the critical role of human expertise and oversight in patient care.
Integration Challenges and Solutions
Healthcare organizations face significant hurdles when implementing symbolic AI systems into their existing infrastructure. As recent research shows, successful AI integration requires more than just advanced algorithms; it demands careful attention to system compatibility, data quality, and organizational processes.
Data interoperability presents one of the biggest obstacles. Healthcare systems often use different data formats and structures, making it difficult to share information seamlessly between departments and facilities. Organizations must establish standardized data protocols and ensure their existing systems can effectively communicate with the new AI components.
System compatibility issues also create significant challenges. Many healthcare facilities rely on legacy systems that weren’t designed with AI integration in mind. Technical leaders need to carefully evaluate their current infrastructure and potentially upgrade key components before implementing symbolic AI solutions. This may require investing in new hardware, updating software systems, or developing custom integration layers.
Concern | Required Action |
---|---|
Data Interoperability | Establish standardized data protocols |
System Compatibility | Upgrade hardware and software systems |
Staff Training | Develop comprehensive training programs |
Security and Privacy | Implement strong encryption, access controls, and audit trails |
Continuous Monitoring | Set up continuous monitoring systems to track performance |
Staff training and change management represent another crucial consideration. Healthcare professionals need proper education on how to work with symbolic AI systems effectively. Organizations should develop comprehensive training programs that help staff understand both the technical aspects and practical applications of the technology in their daily workflows.
To address these challenges, healthcare organizations should follow a structured implementation approach. This includes:
- Conducting thorough system compatibility assessments before implementation
- Developing clear data standardization protocols
- Creating detailed integration timelines with specific milestones
- Establishing robust testing procedures to ensure system reliability
- Implementing continuous monitoring systems to track performance
Security and privacy concerns must also be carefully managed during integration. Healthcare organizations need to ensure their symbolic AI implementations comply with relevant regulations while maintaining the confidentiality and integrity of patient data. This requires implementing strong encryption, access controls, and audit trails.
Successful integration also depends on strong collaboration between technical teams and healthcare professionals. Regular communication and feedback loops help ensure the symbolic AI system meets actual clinical needs and workflows. Organizations should establish clear channels for staff to report issues and suggest improvements during the integration process.
While these challenges may seem daunting, they can be overcome with proper planning and execution. By taking a methodical approach to integration and actively addressing potential obstacles, healthcare organizations can successfully implement symbolic AI systems that enhance their capabilities while maintaining operational efficiency.
Future Directions in Symbolic AI and Healthcare
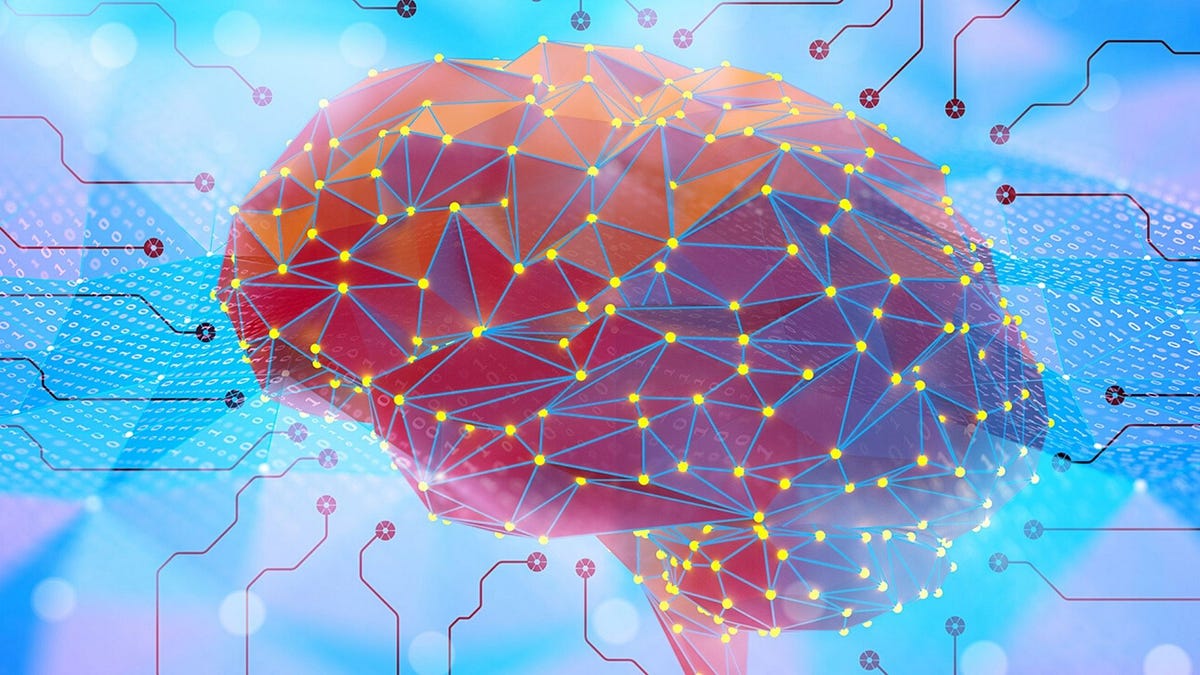
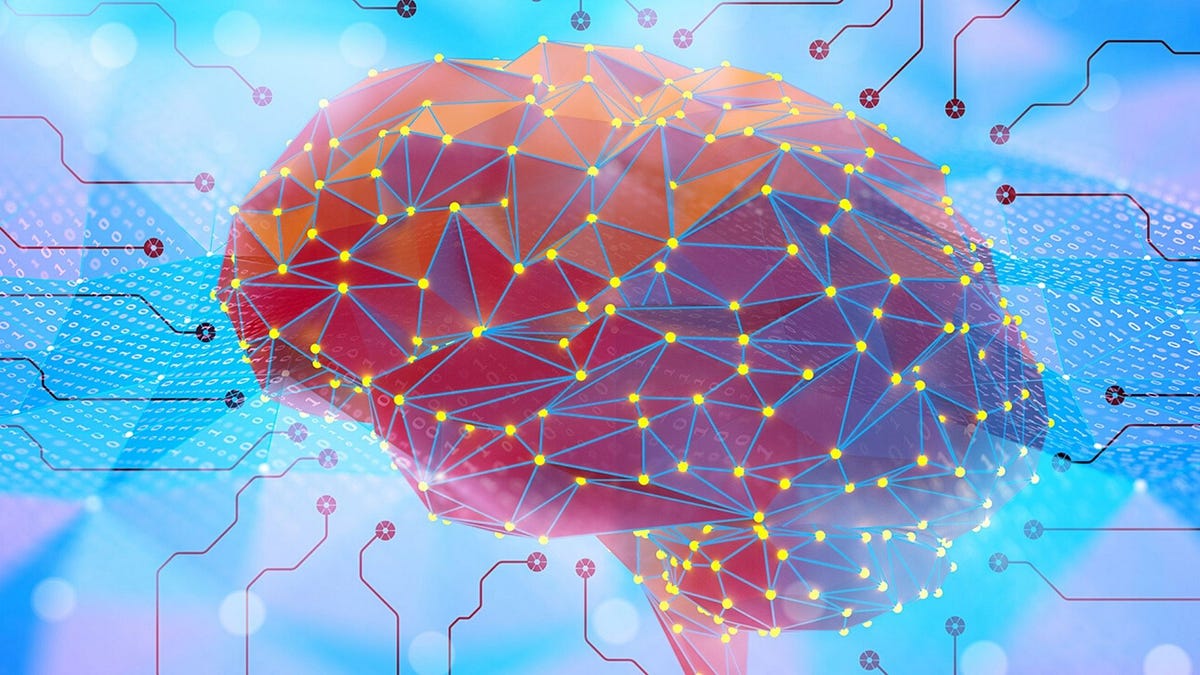
The integration of neuro-symbolic AI frameworks in healthcare stands at a pivotal moment, promising to transform how we approach medical care and decision-making. Recent advancements in combining neural networks’ pattern recognition capabilities with symbolic reasoning’s logical framework are opening new frontiers in personalized medicine and predictive analytics.
In personalized medicine, neuro-symbolic approaches are enhancing diagnostic accuracy and treatment customization. By leveraging vast amounts of patient data while maintaining interpretable decision pathways, these systems can identify subtle patterns in medical histories and imaging studies to suggest highly individualized treatment plans. Research indicates that this hybrid approach will be particularly transformative in drug discovery and genomic medicine by 2025.
Predictive analytics in healthcare is experiencing a significant boost through neuro-symbolic integration. These systems excel at combining historical patient data with medical knowledge bases to forecast potential health issues before they become critical. The ability to process unstructured medical data while applying rule-based reasoning leads to more accurate and explainable predictions, crucial for preventive healthcare strategies.
SmythOS’s hybrid AI capabilities align well with these emerging healthcare applications, offering a platform that can handle both the complexity of medical data analysis and the precision required for healthcare decision-making. The platform’s ability to combine symbolic and neural approaches provides the necessary framework for developing sophisticated healthcare solutions that maintain both accuracy and interpretability.
Looking ahead, we can expect to see increased emphasis on developing interpretable AI systems that can handle the complexity of healthcare decision-making while maintaining transparency. The focus will likely shift toward creating more robust frameworks that can integrate real-time patient data with established medical knowledge, leading to more dynamic and responsive healthcare systems.
The future of healthcare lies not just in the power of AI, but in its ability to reason and explain its decisions in ways that build trust with both healthcare providers and patients.
The integration of meta-cognitive abilities in healthcare AI systems represents another crucial frontier. These capabilities will enable systems to monitor and adjust their reasoning strategies in real-time, leading to more adaptable and reliable healthcare solutions that can better serve diverse patient populations.
Last updated:
Disclaimer: The information presented in this article is for general informational purposes only and is provided as is. While we strive to keep the content up-to-date and accurate, we make no representations or warranties of any kind, express or implied, about the completeness, accuracy, reliability, suitability, or availability of the information contained in this article.
Any reliance you place on such information is strictly at your own risk. We reserve the right to make additions, deletions, or modifications to the contents of this article at any time without prior notice.
In no event will we be liable for any loss or damage including without limitation, indirect or consequential loss or damage, or any loss or damage whatsoever arising from loss of data, profits, or any other loss not specified herein arising out of, or in connection with, the use of this article.
Despite our best efforts, this article may contain oversights, errors, or omissions. If you notice any inaccuracies or have concerns about the content, please report them through our content feedback form. Your input helps us maintain the quality and reliability of our information.