Future Trends in Symbolic AI: What to Expect in the Next Wave of AI Innovation
Artificial intelligence stands at a pivotal moment as symbolic AI, once overshadowed by neural networks, experiences a remarkable renaissance through groundbreaking integration with modern deep learning approaches. This fusion has opened new frontiers in AI’s ability to reason, learn, and solve complex real-world problems with greater sophistication than ever before.
The convergence of symbolic reasoning with neural networks has led to significant advances across multiple domains, from healthcare systems that combine medical knowledge with pattern recognition to financial models that merge market data analysis with rule-based decision making. These hybrid approaches deliver both the adaptability of deep learning and the logical transparency of symbolic systems.
In autonomous vehicles, for instance, symbolic AI’s rule-based reasoning works in concert with neural networks to create more robust and interpretable decision-making systems. Rather than relying solely on pattern recognition, these systems can explain their choices through logical steps while still maintaining the flexibility to learn from new situations.
Perhaps most exciting is the emergence of large language model-powered autonomous agents that seamlessly blend symbolic and neural capabilities. These systems demonstrate unprecedented abilities in handling complex tasks by breaking them down into logical sequences while maintaining the adaptability to learn from examples, a trademark of human-like intelligence.
Looking ahead, the integration of symbolic AI with other emerging technologies promises even more transformative applications. From enhancing the explainability of AI decisions in critical systems to enabling more sophisticated reasoning in robotics, symbolic AI’s evolution represents not just a technological advancement, but a fundamental shift in how we approach artificial intelligence.
Historical Context of Symbolic AI
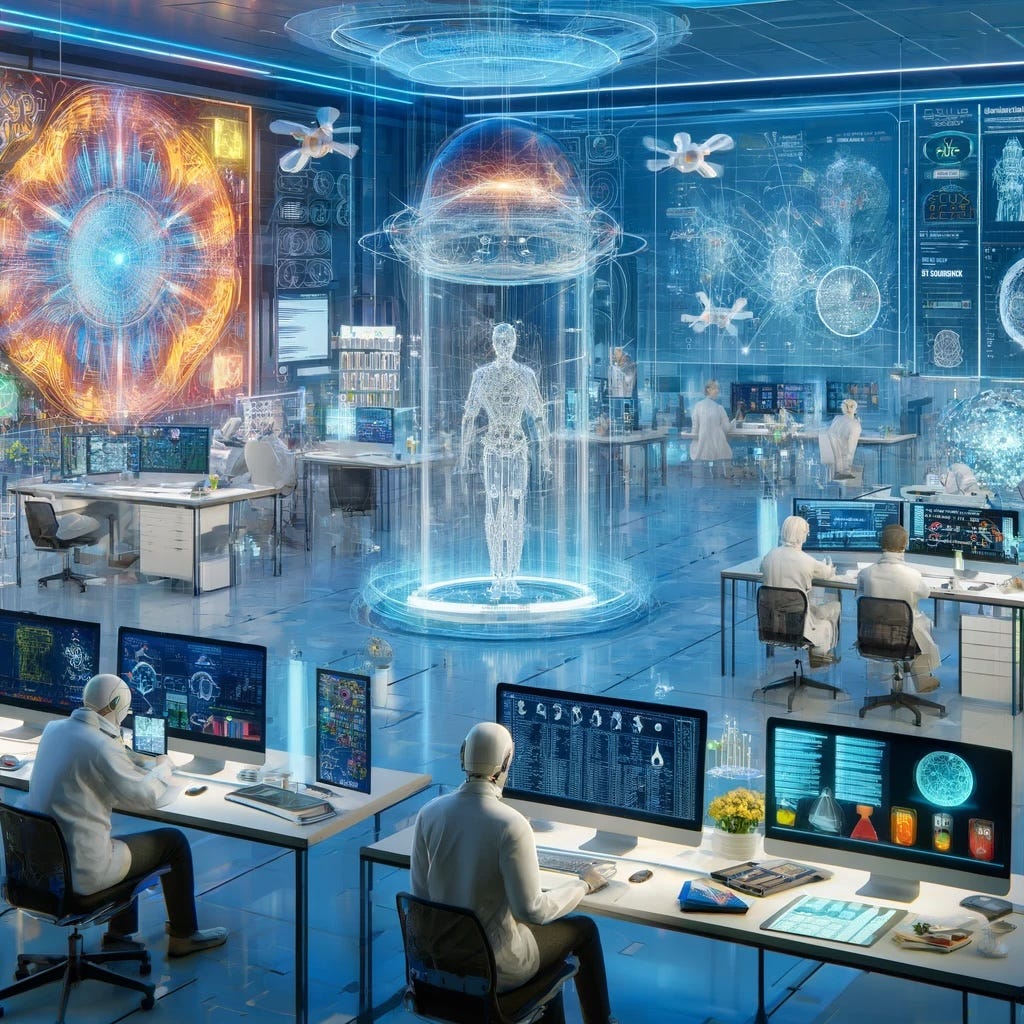
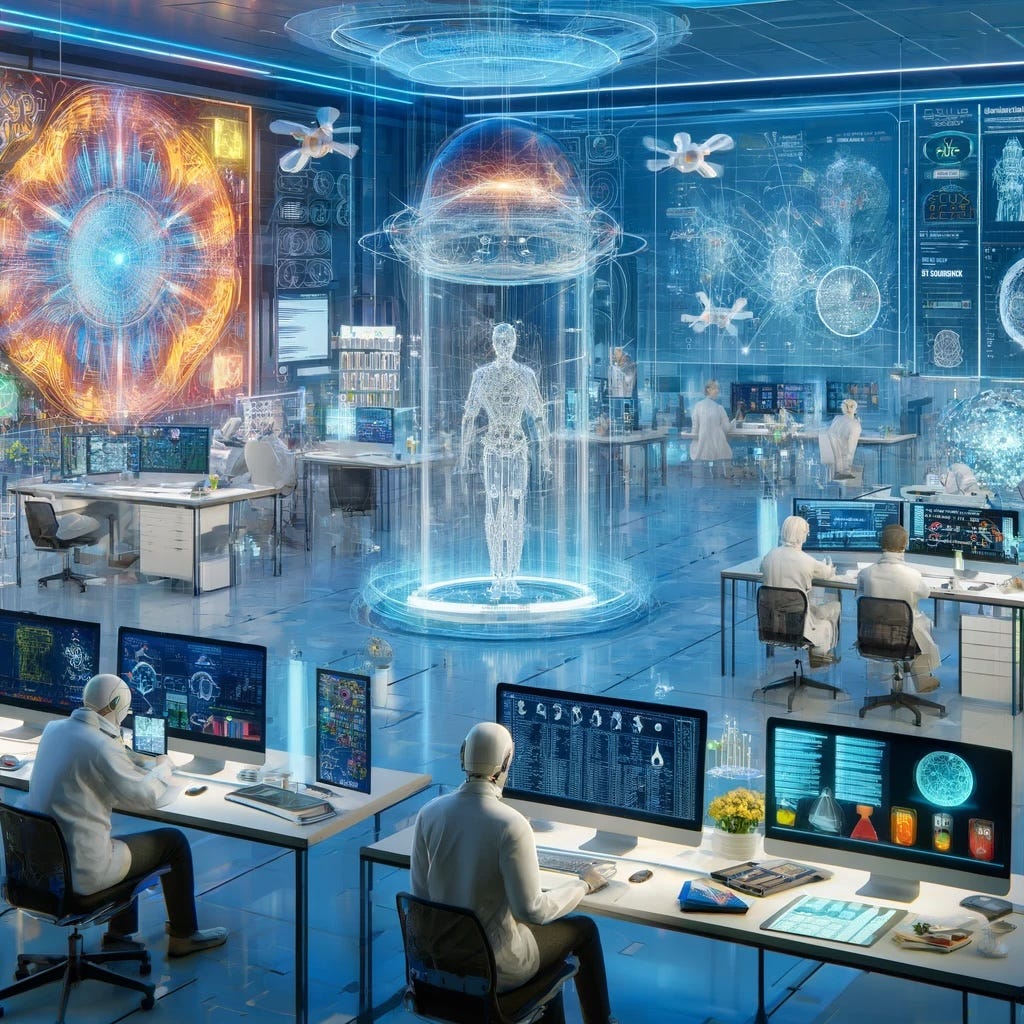
Symbolic AI emerged in the mid-1950s as a groundbreaking approach to artificial intelligence, launched by pioneering researchers like Allen Newell and Herbert A. Simon. Their creation of the Logic Theorist in 1956 marked the first program designed to mimic human problem-solving skills, establishing the foundations of rule-based computing.
Throughout the 1960s and 1970s, symbolic AI flourished with the development of expert systems – specialized programs that solved complex problems by applying logical rules and knowledge representation. MYCIN, a landmark system for diagnosing blood infections, demonstrated how symbolic AI could match human expert performance in specialized domains through carefully crafted rules and logical inference.
The 1980s saw symbolic AI reach new heights with the widespread adoption of knowledge representation frameworks and reasoning engines. Researchers developed sophisticated methods for encoding human expertise into computer-readable formats, allowing machines to perform logical deductions and make informed decisions. However, this era also exposed key limitations – the systems struggled with uncertainty, required extensive manual knowledge engineering, and couldn’t easily learn from experience.
A significant transition began in the 1990s as researchers explored ways to overcome these constraints. The emergence of probabilistic approaches and early neural networks sparked interest in hybrid systems that could combine symbolic reasoning with statistical learning. This shift laid the groundwork for modern neuro-symbolic AI, which aims to merge the logical clarity of symbolic systems with the adaptability of machine learning.
Today’s symbolic AI has evolved far beyond its rigid rule-based origins. Contemporary hybrid models integrate symbolic knowledge with deep learning capabilities, enabling more flexible reasoning while maintaining interpretability. Systems can now learn symbolic rules from data, adapt to new scenarios, and handle uncertainty – addressing many of the limitations that challenged early implementations while preserving the core strengths of logical reasoning.
Integration of Neural and Symbolic AI
The marriage of neural networks and symbolic AI represents one of the most promising developments in artificial intelligence. This fusion aims to combine their strengths—the learning capabilities of neural networks with the logical reasoning of symbolic systems—while overcoming their limitations.
Neural networks excel at pattern recognition and learning from large datasets, enabling them to handle complex tasks like image recognition and natural language processing. However, they often operate as black boxes, making their decision-making processes difficult to interpret. In contrast, symbolic AI systems use explicit rules and logic, making them highly interpretable but less adaptable to new situations.
When integrated effectively, these approaches create systems that can both learn from data and reason logically about their decisions. For example, in medical diagnosis, a neuro-symbolic system could use neural networks to analyze patient symptoms while applying symbolic reasoning to explain its diagnostic recommendations using established medical knowledge and protocols.
To build a robust, knowledge-driven approach to AI we must have the machinery of symbol manipulation in our toolkit. Too much useful knowledge is abstract to proceed without tools that represent and manipulate abstraction.
Researchers face several key challenges in developing these hybrid systems. One major hurdle involves reconciling the different ways neural and symbolic systems represent information—neural networks use continuous numerical values, while symbolic systems work with discrete symbols and rules. Additionally, maintaining interpretability while preserving the learning capabilities of neural networks requires careful architectural design.
Despite these challenges, recent advances in neural-symbolic integration have shown promising results across various domains, including computer vision and natural language processing. These systems demonstrate superior performance while providing clearer insights into their decision-making processes.
Applications of Symbolic AI in Various Fields
Symbolic AI is a powerful tool for solving complex problems across various industries by leveraging formal logic and structured knowledge representation. This approach is particularly valuable in healthcare, where systems aid in diagnostic reasoning and treatment planning by interpreting medical data through established knowledge bases and logical rules.
In the financial sector, symbolic AI excels at portfolio management and risk assessment tasks. Its ability to follow explicit regulatory frameworks while making decisions that can be clearly traced and audited makes it especially suitable for finance. Unlike black-box AI systems, symbolic AI’s reasoning process remains transparent—a critical requirement for financial compliance.
The manufacturing industry has seen significant improvements in efficiency through symbolic AI implementation. The technology optimizes production workflows by modeling complex processes and resource allocation scenarios. Factory managers can now identify bottlenecks and streamline operations with unprecedented precision, thanks to symbolic AI’s structured approach to problem-solving.
Industry | Application |
---|---|
Healthcare | Expert systems for medical diagnosis, treatment planning |
Finance | Financial fraud detection, portfolio management, risk assessment |
Legal | Automated legal document analysis, contract review, legal advisory systems |
Industrial Automation | Precise rule-based processes, predictive maintenance |
Customer Service | Chatbots for customer inquiries |
Cybersecurity | Threat detection and intelligence |
Education | Personalized education platforms |
One of the most fascinating applications lies in robotics, where symbolic AI enables machines to interpret their environment and make informed decisions. Unlike purely neural network approaches, symbolic AI allows robots to reason about their actions using explicit rules and knowledge representation. This proves invaluable in settings requiring careful deliberation, such as robotic surgery or automated assembly lines.
What sets symbolic AI apart in these applications is its ability to provide clear explanations for its decisions. When a medical diagnostic system suggests a treatment plan, it can walk healthcare professionals through its reasoning process step by step. This transparency builds trust and allows for effective collaboration between human experts and AI systems.
The future looks promising for symbolic AI in hybrid systems that combine rule-based reasoning with modern machine learning techniques. Organizations increasingly recognize that while data-driven approaches excel at pattern recognition, symbolic AI’s logical framework remains essential for handling complex decision-making scenarios that require explicit reasoning and accountability.
Challenges and Future Directions
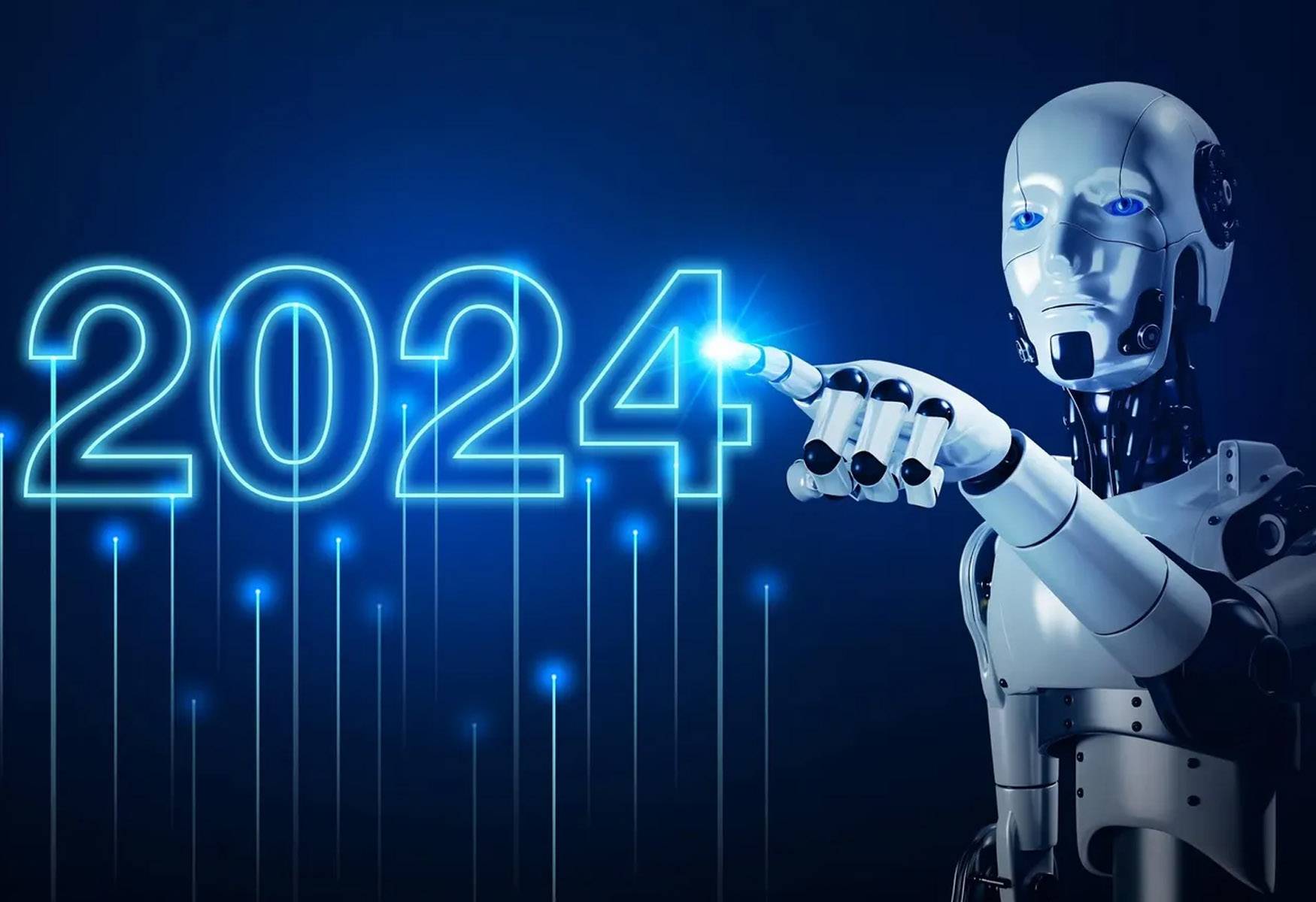
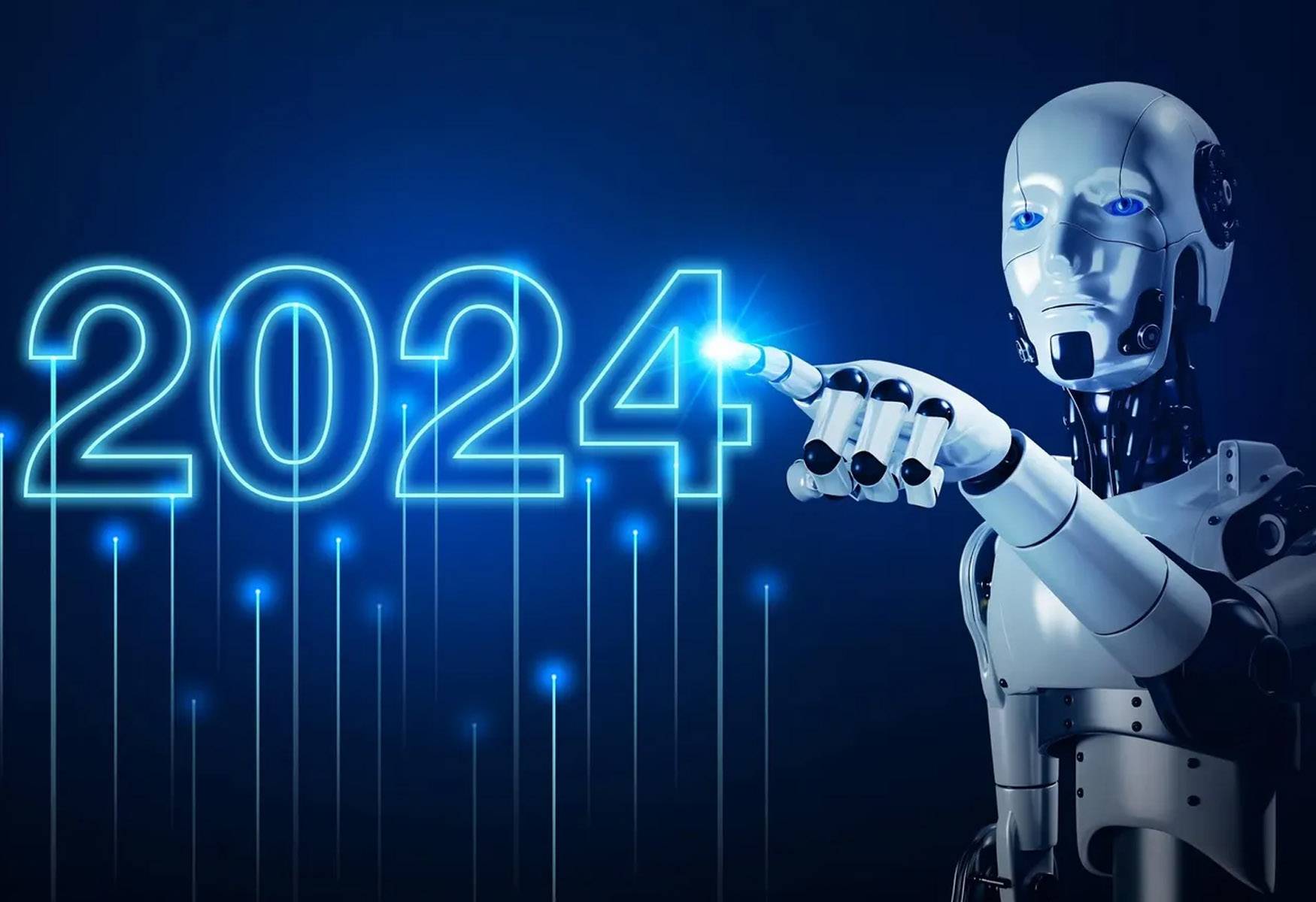
A robot interacts with luminous blue digits in a digital world. – Via robots.net
Symbolic AI, despite its theoretical elegance and interpretable nature, faces critical hurdles that must be addressed as the field evolves. The remarkable advancements in artificial intelligence have exposed challenges surrounding unsustainable computational trajectories, limited robustness, and a pervasive lack of explainability that demand innovative solutions.
One of the most pressing concerns is scalability. As research indicates, traditional symbolic systems struggle to handle the massive datasets and complex reasoning chains required for real-world applications. The computational demands grow exponentially with the size of the knowledge base, making it difficult to deploy these systems at scale without significant performance degradation.
Explainability presents another significant challenge. While symbolic AI’s rule-based nature theoretically offers transparency, integrating these systems with modern neural approaches often creates a ‘black box’ effect. This opacity becomes particularly problematic in critical applications like healthcare or autonomous vehicles, where understanding the reasoning behind decisions is crucial for trust and accountability.
The integration of symbolic and neural approaches, known as neuro-symbolic AI, offers promising directions for addressing these limitations. Recent developments show that combining logical reasoning with probabilistic models can enhance both scalability and interpretability. For instance, frameworks like Logic Tensor Networks demonstrate how symbolic rules can be embedded within neural architectures to maintain explainability while improving performance.
Future research directions are increasingly focused on developing hybrid architectures that can leverage the strengths of both symbolic and neural approaches. This includes exploring meta-cognitive frameworks that enable systems to monitor and adjust their reasoning strategies in real-time, improving their ability to handle complex, dynamic environments while maintaining transparency.
The remarkable advancements in artificial intelligence are facing challenges surrounding unsustainable computational trajectories, limited robustness, and a lack of explainability. To develop next-generation cognitive AI systems, neuro-symbolic AI emerges as a promising paradigm.
IEEE Xplore Digital Library
Open research questions remain around developing scalable frameworks that integrate traditional logic programming with neural networks for complex reasoning tasks. Additionally, there’s a growing need to incorporate commonsense knowledge and advanced language understanding to enhance multi-hop reasoning capabilities while ensuring reliable and trustworthy decision-making processes.
Leveraging SmythOS for Symbolic AI Development
SmythOS revolutionizes symbolic AI development through its intuitive visual debugging environment and seamless integration capabilities. The platform’s comprehensive toolset empowers developers to create sophisticated rule-based systems without getting bogged down in implementation complexities.
At the core of SmythOS’s offering is its groundbreaking visual debugging environment, which transforms the traditionally opaque process of symbolic AI development into an interactive and transparent experience. Developers can observe their AI systems in real-time, tracking decision paths and rule executions with unprecedented clarity. This visibility dramatically reduces the time spent identifying and resolving logical inconsistencies.
The platform’s integration framework stands out for its ability to seamlessly connect with existing rule systems. Rather than requiring a complete overhaul of established workflows, SmythOS adapts to current infrastructure, allowing organizations to preserve their investments while advancing their AI capabilities. This practical approach to integration minimizes disruption and accelerates adoption.
A standout feature of SmythOS is its scalability architecture. The platform efficiently handles growing workloads and increasing complexity without sacrificing performance. Whether processing thousands of rules or managing intricate decision trees, SmythOS maintains consistent performance while keeping infrastructure costs manageable. According to platform metrics, users have reported a 70% reduction in debugging time compared to traditional development approaches.
Beyond its technical capabilities, SmythOS democratizes symbolic AI development through its user-friendly interface. The platform’s visual workflow builder enables both experienced developers and domain experts to contribute to AI system development, fostering collaboration and accelerating project timelines. This accessibility doesn’t compromise sophistication – advanced users can still leverage the platform’s full range of features for complex implementations.
Conclusion and Future Outlook
The landscape of symbolic AI is evolving, where traditional rule-based approaches are merging with advanced machine learning capabilities. These hybrid systems, known as neural-symbolic models, combine the logical reasoning strengths of symbolic AI with the pattern recognition capabilities of deep learning.
While symbolic AI has faced challenges with scalability and adaptability, innovative solutions are emerging. Integrating symbolic methods with data-driven approaches is proving promising, enabling AI systems to reason more effectively while maintaining the transparency and interpretability that make symbolic AI valuable in critical applications.
Platforms like SmythOS are playing a pivotal role by democratizing access to sophisticated AI tools. Through intuitive visual development environments and robust deployment options, organizations can harness the power of symbolic AI without complex implementation details. This accessibility is crucial for accelerating innovation across industries.
The future of symbolic AI appears bright as researchers explore new frontiers in neural-symbolic integration. These advances are opening possibilities for AI systems that can process information logically and learn and adapt from experience. As experts note, this integration is more than a technical achievement – it’s a step toward creating AI systems that can reason, learn, and adapt in ways more aligned with human cognition.
Looking ahead, the focus will likely remain on developing more robust hybrid approaches that leverage the strengths of both symbolic and statistical methods. By addressing current limitations while building on established successes, the industry is moving toward more capable, flexible, and practical AI solutions that can tackle increasingly complex real-world challenges.
Last updated:
Disclaimer: The information presented in this article is for general informational purposes only and is provided as is. While we strive to keep the content up-to-date and accurate, we make no representations or warranties of any kind, express or implied, about the completeness, accuracy, reliability, suitability, or availability of the information contained in this article.
Any reliance you place on such information is strictly at your own risk. We reserve the right to make additions, deletions, or modifications to the contents of this article at any time without prior notice.
In no event will we be liable for any loss or damage including without limitation, indirect or consequential loss or damage, or any loss or damage whatsoever arising from loss of data, profits, or any other loss not specified herein arising out of, or in connection with, the use of this article.
Despite our best efforts, this article may contain oversights, errors, or omissions. If you notice any inaccuracies or have concerns about the content, please report them through our content feedback form. Your input helps us maintain the quality and reliability of our information.