Semantic AI in Healthcare: Advancing Patient Care
Did you know that 4.5 billion people worldwide lack access to essential healthcare services? With an expected shortage of 10 million healthcare workers by 2030, Semantic AI is transforming modern medicine. This technology revolutionizes how healthcare professionals access, interpret, and use vast amounts of medical data.
Imagine a healthcare system where patient records seamlessly flow between providers, diagnostic decisions are enhanced by intelligent systems that understand medical context, and administrative burdens no longer overshadow patient care. This is the reality that Semantic AI is creating right now.
According to recent findings, semantic interoperability is a top health IT priority, with AI transforming unstructured medical data into standardized, actionable insights. Healthcare organizations are using this technology to convert complex medical documents into structured formats, improving data accessibility and clinical decision-making.
This comprehensive exploration delves into how Semantic AI is reshaping healthcare data management, enhancing diagnostic processes, and solving interoperability challenges. From streamlining clinical workflows to improving patient outcomes, we examine the practical applications, challenges, and future directions of this technology.
What awaits you in this article? Gain insights into how Semantic AI is bridging gaps in healthcare delivery, making sense of complex medical data, and contributing to more efficient, accurate, and accessible healthcare for all.
Challenges in Healthcare Data Interoperability
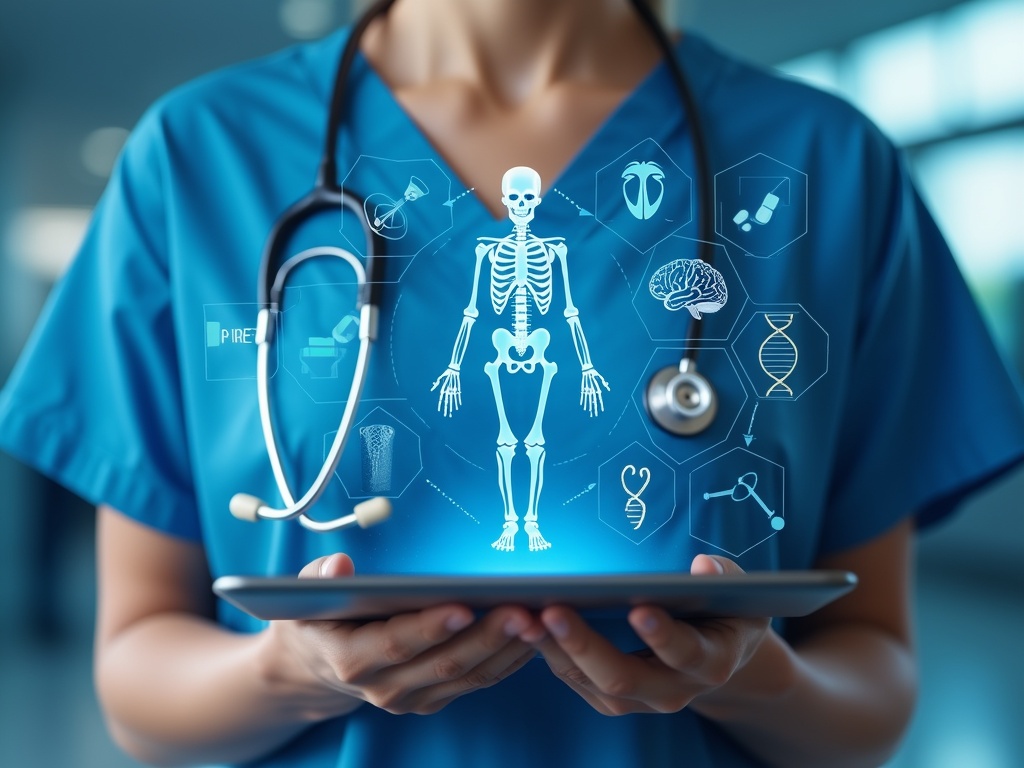
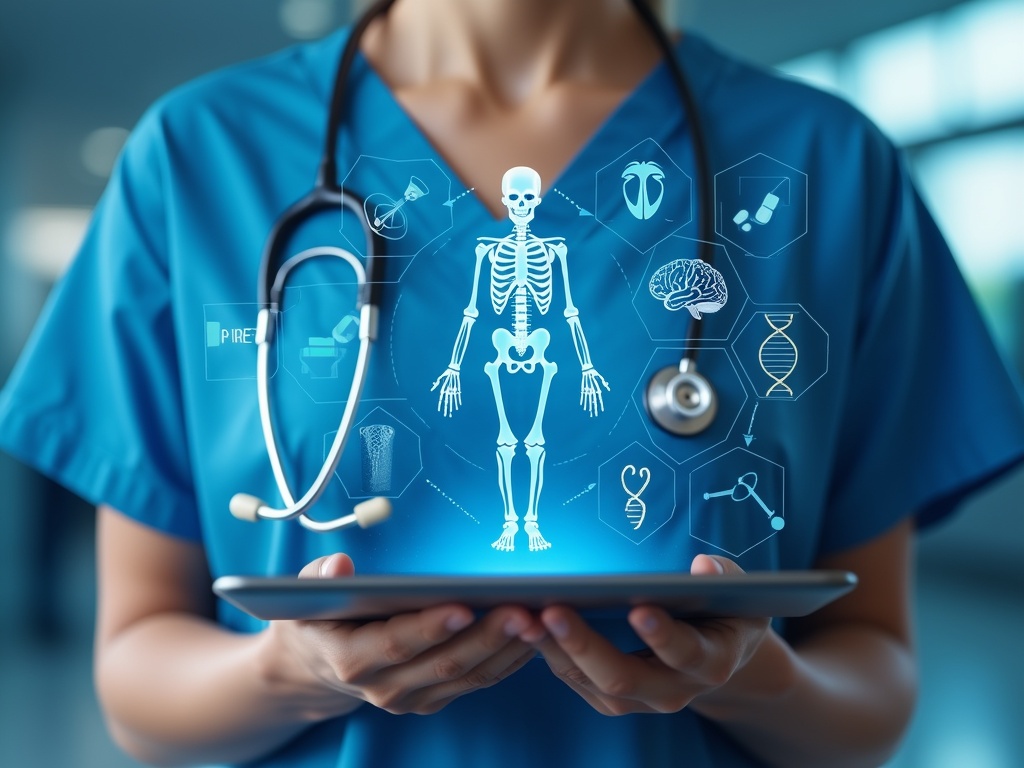
Healthcare organizations face a fundamental challenge: the inability to effectively share and understand patient data across different systems. When a patient moves between providers or facilities, their critical health information often gets trapped in isolated data silos, creating potential gaps in care quality.
The root of this interoperability challenge lies in how different healthcare systems speak essentially different languages. A primary care clinic may code a diagnosis one way, while a specialist’s office uses entirely different terminology for the same condition. As one hospital representative noted, “There’s been an explosion of health IT applications, vendors and products over the past decade, many of whom overlap and intersect in ways that don’t really allow for great interoperability.”
This lack of standardization creates significant obstacles for healthcare organizations trying to build complete patient records. For example, when a hospital needs to access a patient’s medication history from an outside pharmacy system, incompatible data formats can make it impossible to automatically import that critical information into the hospital’s electronic health record.
Semantic AI technology offers a promising solution by acting as an intelligent translator between these disparate systems. Rather than requiring every healthcare organization to adopt the exact same terminology, semantic AI can map different terms to their shared meanings. For instance, it can recognize that “myocardial infarction” and “heart attack” refer to the same condition, allowing systems to exchange data meaningfully even when using different terms.
More advanced semantic interoperability tools powered by artificial intelligence can also help systems interpret clinical context and relationships between data points. As noted by a healthcare technology expert, these tools focus on the meaning of information and its context, establishing a common framework for accurate data interpretation across platforms.
The impact of improved semantic interoperability extends beyond individual patient care. Healthcare organizations can more effectively aggregate population health data, identify trends, and develop insights that benefit entire communities. Additionally, semantic AI supports the growing adoption of precision medicine by enabling researchers to analyze standardized data across multiple institutions and studies.
Applications of Semantic AI in Clinical Settings
Healthcare institutions are increasingly leveraging semantic AI technologies to transform patient care delivery and clinical operations. This sophisticated technology interprets complex medical data with human-like understanding, enabling more nuanced and effective healthcare solutions.
Semantic AI analyzes vast amounts of patient data to forecast potential health outcomes with remarkable precision. As highlighted in a comprehensive study, these AI systems can identify high-risk populations and predict treatment responses, allowing healthcare providers to intervene proactively before conditions worsen. For instance, semantic AI models can analyze patterns in patient vital signs and lab results to predict complications days before they become clinically apparent.
One of the most significant applications is in clinical coding accuracy. AI-powered systems now parse through medical records using natural language processing to suggest appropriate diagnostic codes with greater precision. Traditional manual coding processes often result in errors and backlogs, but semantic AI platforms can analyze clinical documentation in real-time, improving both speed and accuracy. These systems can process complex medical narratives and automatically suggest relevant codes while maintaining compliance with ever-changing healthcare regulations.
Perhaps most importantly, semantic AI enables truly personalized patient care by processing individual health histories, genetic information, and treatment responses to craft tailored therapeutic approaches. The technology can identify subtle patterns in patient data that might escape human observation, leading to more precise treatment recommendations. This level of personalization helps clinicians make more informed decisions about patient care, ultimately improving treatment outcomes.
The impact extends beyond direct patient care into operational efficiency. By automating routine tasks and providing real-time insights, semantic AI allows healthcare professionals to focus more on patient interaction and complex decision-making. This shift not only improves the quality of care but also helps address the growing challenges of healthcare workforce shortages and increasing patient demands.
Semantic AI in Medical Coding and Auditing
Medical coding has transformed with the emergence of Semantic AI technologies. These systems tackle what was once an overwhelmingly manual process, where human coders typically processed around 60 cases daily with each case requiring 7-8 minutes of focused attention.
Platforms like Semantic Health leverage advanced artificial intelligence to analyze complex clinical documentation, automatically suggesting appropriate medical codes while maintaining high accuracy standards. This AI-driven approach doesn’t just speed up the coding process; it fundamentally enhances its precision and consistency.
Semantic AI’s impact on coding efficiency is particularly noteworthy in handling lengthy medical records. While human coders previously had to manually comb through hundreds or thousands of pages of clinical documentation, AI systems can now instantly process this information, identifying relevant diagnoses and procedures with remarkable accuracy. This advancement helps address significant backlog issues that many healthcare facilities face.
Modern Semantic AI platforms go beyond simple code matching by incorporating sophisticated Natural Language Processing (NLP) capabilities. These systems understand clinical context, recognize medical terminology variations, and account for complex coding guidelines. This comprehensive approach maintains coding accuracy while significantly reducing the manual effort required from human coders.
One of the most significant advantages of Semantic AI in medical coding is its ability to learn and improve over time. As these systems process more cases and receive feedback from human coders, their accuracy and efficiency continue to increase. This creates a cycle where the technology becomes increasingly reliable, helping healthcare facilities optimize their revenue cycles and ensure compliance with coding standards.
Future Directions of Semantic AI in Healthcare
Real-time data processing is at the forefront of healthcare’s AI revolution. Wearable devices with AI sensors now continuously monitor patients’ vitals, enabling healthcare providers to detect anomalies instantly and respond quickly. This technological leap transforms traditional reactive healthcare into a proactive model where intervention occurs before conditions worsen.
Clinical decision support systems are undergoing a remarkable transformation through Semantic AI. According to healthcare technology experts, AI’s ability to process and analyze patient data in real-time is revolutionizing how medical professionals detect anomalies and assist in surgical procedures.
These advancements create a more efficient and accurate healthcare delivery system. The integration of AI into broader clinical workflows represents another significant advancement. Future systems will automate tasks like clinical note-taking and patient registration while summarizing vast amounts of medical data. This evolution streamlines administrative work and allows healthcare professionals to dedicate more time to patient care. Interdisciplinary collaboration is a crucial driver of innovation in this space.
Medical professionals, data scientists, and AI researchers are joining forces to develop more sophisticated healthcare solutions. This collaborative approach ensures that technological developments remain grounded in practical clinical needs while pushing the boundaries of patient care.
Looking ahead, the fusion of Semantic AI with Internet of Things (IoT) technology promises to revolutionize patient monitoring and care delivery. Smart systems will not only collect and analyze patient data but also predict potential health issues before they become critical. This predictive capability, combined with enhanced decision support tools, will enable healthcare providers to deliver more personalized and effective treatment plans.
Conclusion and Role of SmythOS in Semantic AI
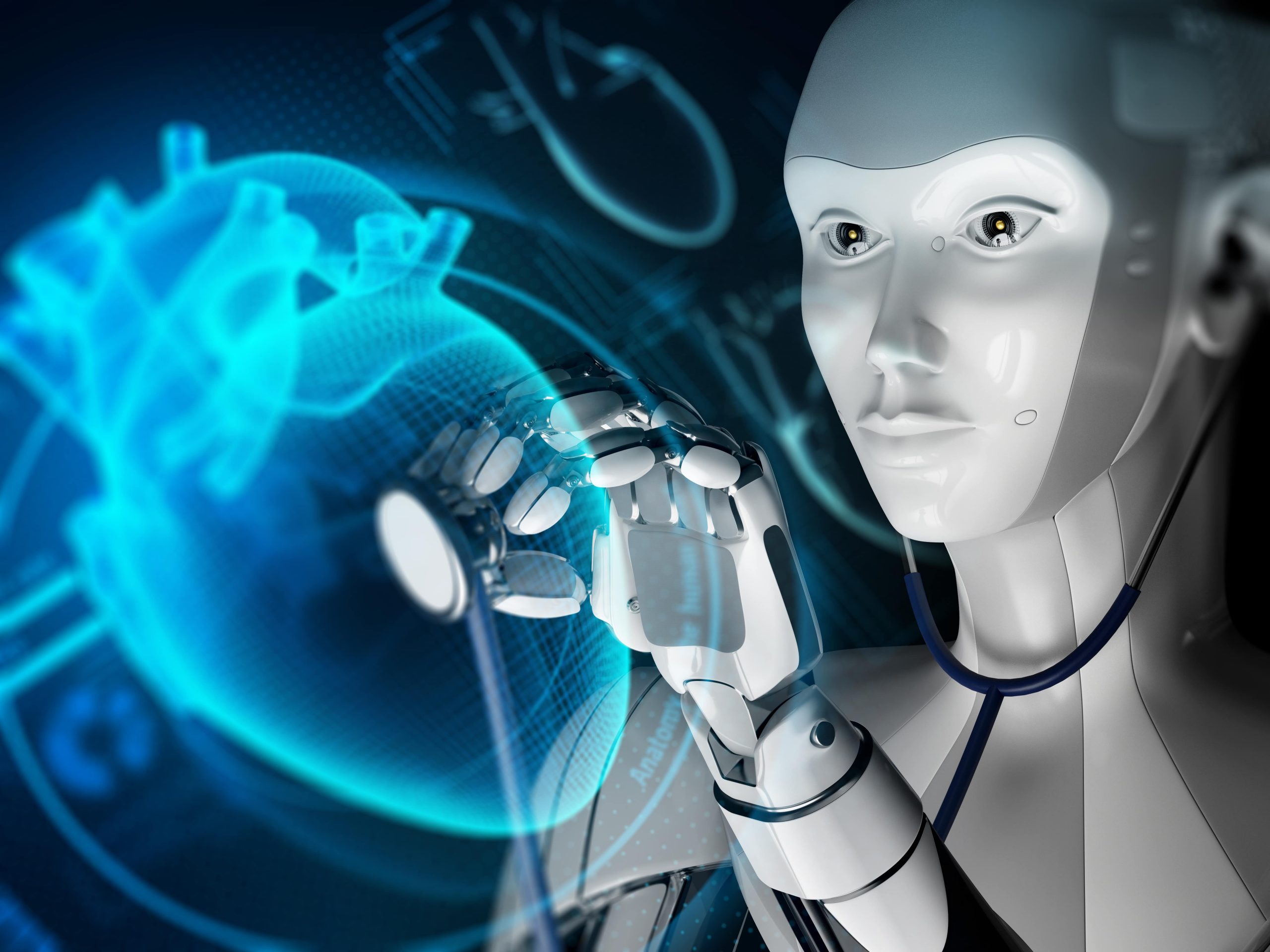
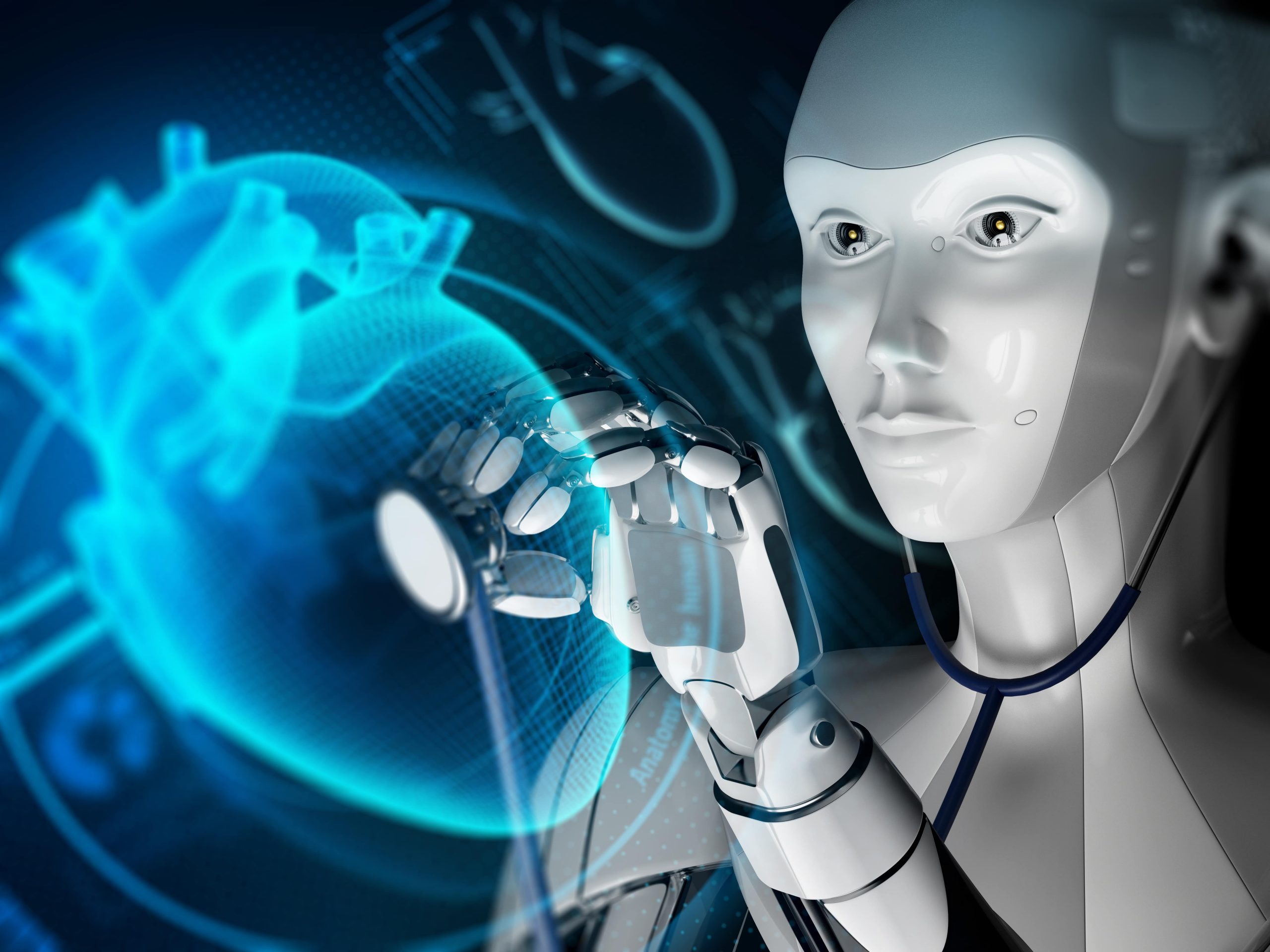
AI advancing healthcare through innovative solutions. – Via capestart.com
Artificial intelligence is transforming healthcare delivery, with semantic AI playing a key role in improving clinical decision-making and patient outcomes. Advances in natural language processing and knowledge representation allow healthcare systems to extract deeper meaning from complex medical data, leading to more accurate diagnoses and personalized treatment plans.
The integration of semantic technologies in healthcare presents unique challenges, such as ensuring data privacy and maintaining transparency in AI decision-making. Platforms like SmythOS provide enterprise-grade tools that make semantic AI implementation more accessible and manageable.
SmythOS’s visual builder offers an intuitive interface for creating and deploying semantic AI applications, eliminating the complexity traditionally associated with these systems. The platform’s built-in debugging capabilities enable healthcare organizations to monitor and validate their AI systems in real-time, ensuring accuracy and reliability in clinical settings.
Importantly, SmythOS’s comprehensive approach to semantic AI integration helps healthcare providers maintain compliance with regulatory requirements while advancing their technological capabilities. By streamlining the development and deployment of semantic AI applications, SmythOS enables healthcare organizations to focus on delivering exceptional patient care.
Looking to the future, the continued evolution of semantic AI in healthcare will bring new challenges and opportunities. With robust platforms like SmythOS supporting this transformation, healthcare organizations can confidently embrace these innovations, knowing they have the tools needed to harness the full potential of semantic AI while maintaining the highest standards of patient care and data security.
Last updated:
Disclaimer: The information presented in this article is for general informational purposes only and is provided as is. While we strive to keep the content up-to-date and accurate, we make no representations or warranties of any kind, express or implied, about the completeness, accuracy, reliability, suitability, or availability of the information contained in this article.
Any reliance you place on such information is strictly at your own risk. We reserve the right to make additions, deletions, or modifications to the contents of this article at any time without prior notice.
In no event will we be liable for any loss or damage including without limitation, indirect or consequential loss or damage, or any loss or damage whatsoever arising from loss of data, profits, or any other loss not specified herein arising out of, or in connection with, the use of this article.
Despite our best efforts, this article may contain oversights, errors, or omissions. If you notice any inaccuracies or have concerns about the content, please report them through our content feedback form. Your input helps us maintain the quality and reliability of our information.