Revolutionizing E-Commerce with Semantic AI
Picture walking into a virtual store where the shelves rearrange themselves based on your exact needs, preferences, and shopping history. This is the transformative power of Semantic AI reshaping online shopping in 2024.
Today’s e-commerce landscape faces a critical challenge: helping customers find exactly what they want amid millions of products. Research shows that traditional web mining often fails to meet user needs, creating frustrating shopping experiences. Enter Semantic AI – an innovative approach that combines natural language processing and machine learning to understand not just what customers search for, but the actual meaning and context behind their queries.
Think of Semantic AI as your brilliantly intuitive personal shopping assistant. Rather than just matching keywords, it grasps nuanced search intentions – the difference between searching for “light summer dresses” versus “evening gowns for summer weddings.” This technological breakthrough is transforming how we discover and purchase products online.
The impact extends far beyond basic product searches. By processing vast amounts of data about customer behaviors, preferences, and interactions, Semantic AI creates hyper-personalized shopping experiences that feel remarkably human. Whether you’re browsing fashion items or hunting for technical equipment, the AI understands your unique context and guides you to the most relevant options.
This comprehensive guide will explore how Semantic AI is transforming key aspects of e-commerce – from intelligent product recommendations to dynamic pricing strategies. We’ll examine real-world applications that are already changing how millions shop online and look into the future of AI-powered retail. Discover how this technology is creating shopping experiences that are not just smarter, but fundamentally more satisfying for consumers worldwide.
Understanding Semantic Search
Search engines have evolved from simple keyword matching to sophisticated semantic search technology, which acts more like a smart assistant. By leveraging Natural Language Processing (NLP), semantic search decodes the meaning and context behind your words to deliver accurate results.
Consider this example: When you search for “feel good movies for a rainy day,” traditional keyword search would hunt for exact matches of those terms. In contrast, semantic search understands you’re seeking uplifting, heartwarming films – even if those specific words aren’t used in the movie descriptions. As research shows, this contextual understanding significantly improves the accuracy and relevance of search results.
Semantic search transforms both your query and potential search results into mathematical representations called embeddings. These embeddings capture the deeper meaning of words and phrases, allowing the search engine to identify conceptually similar content even when the exact keywords don’t match. For instance, it recognizes that ‘automobile’ and ‘car’ refer to the same concept or that someone searching for ‘headache remedies’ might also benefit from results about ‘pain relief’.
This intelligent interpretation of user intent has revolutionized product discovery in e-commerce. When you search for ‘comfortable office chair,’ semantic search understands you’re likely interested in ergonomic features, lumbar support, and adjustable settings – not just listings that happen to contain those exact words. The technology considers your previous searches, current trends, and even regional preferences to surface the most relevant products.
What makes semantic search particularly powerful is its ability to learn and adapt. Through continuous analysis of user interactions and search patterns, these systems become increasingly adept at understanding nuanced queries. The more people use semantic search, the better it becomes at predicting and fulfilling their true search intent, creating a more intuitive and satisfying search experience for everyone.
Benefits of Semantic AI in E-Commerce
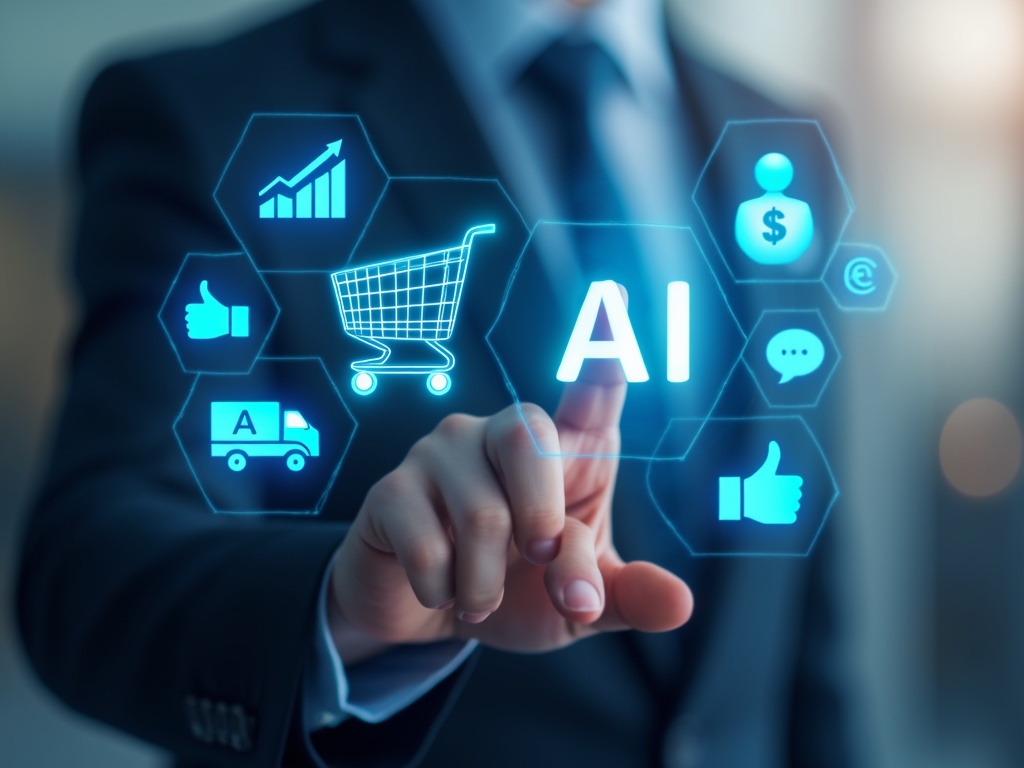
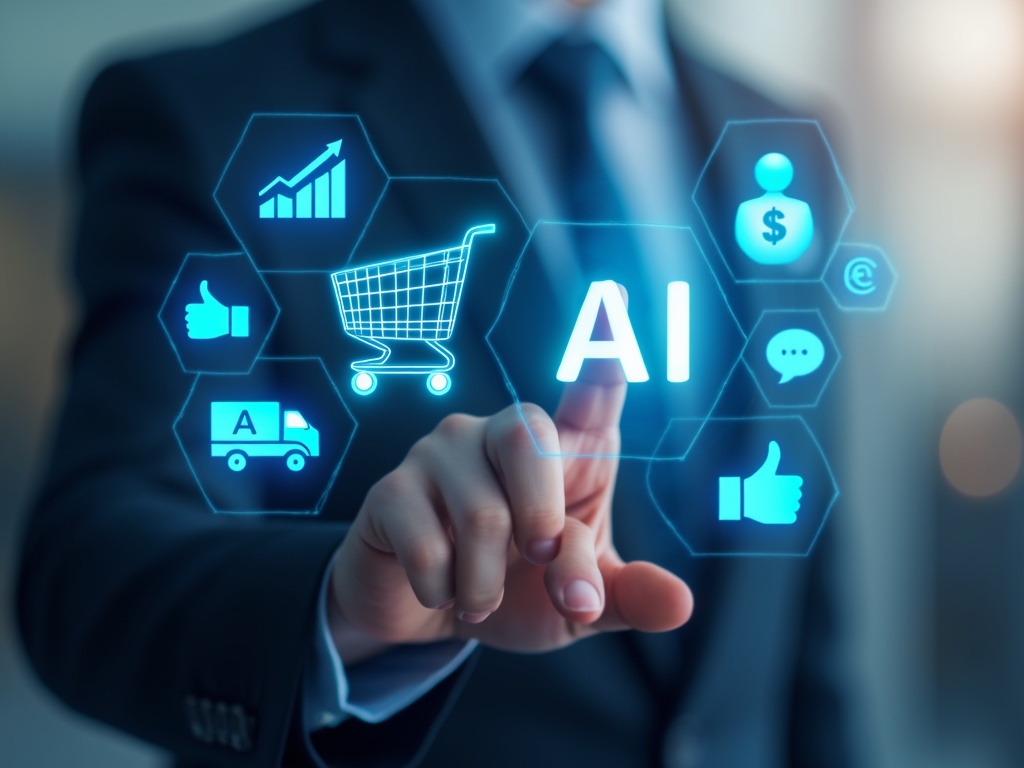
The traditional keyword-based search approach in e-commerce has long frustrated shoppers trying to find exactly what they want. Enter semantic AI—a technology transforming how customers discover and purchase products online. Research shows that implementing semantic AI can significantly boost conversion rates and average order values by enhancing product discovery.
Enhanced Product Discovery and Search Accuracy
Semantic AI excels at understanding natural language and user intent, going far beyond simple keyword matching. When a customer searches for “comfy running shoes for long distances,” the AI comprehends the underlying need for cushioning, support, and durability—even if those exact terms aren’t used.
This contextual understanding enables the system to surface highly relevant products that truly match what customers are seeking. Even with spelling mistakes or conversational language, semantic AI interprets the intent and delivers accurate results.
Through continuous machine learning, these systems become increasingly adept at recognizing patterns in how customers express their needs. The more interactions that occur, the better the AI becomes at matching products to search queries.
For example, when someone searches for “business casual outfits for summer,” semantic AI understands seasonality, dress code requirements, and style preferences to recommend appropriate clothing combinations.
This enhanced search precision dramatically reduces the frustration of scrolling through irrelevant items, helping customers find what they want quickly and efficiently.
Personalized Shopping Experiences
Beyond improving search, semantic AI powers sophisticated personalization by analyzing customer behavior patterns and preferences. The technology tracks interactions across the shopping journey to build detailed customer profiles.
This deep understanding allows the system to tailor product recommendations to each individual shopper. Rather than generic “bestseller” suggestions, customers see items specifically matched to their tastes and needs.
The personalization extends to how products are presented and merchandised. Semantic AI can automatically adjust the ordering of search results and category pages based on a customer’s demonstrated preferences.
70% of e-commerce decision-makers believe AI-driven technology can help their business improve personalization, while 54% said it can help with their site search
Statista Research
With each interaction, the system refines its understanding of customer preferences, leading to increasingly relevant recommendations that boost engagement and sales.
Streamlined Operations and Efficiency
The benefits of semantic AI extend beyond the customer experience to internal operations. The technology dramatically reduces the manual effort required to optimize product data and search results.
Instead of spending countless hours manually tagging products and managing synonyms, businesses can rely on semantic AI to automatically understand product attributes and relationships. This frees up valuable time for staff to focus on strategic initiatives.
The system’s ability to learn and adapt also means that optimization happens continuously without human intervention. As shopping patterns and preferences evolve, semantic AI automatically adjusts to maintain optimal performance.
This automation of tedious manual tasks not only improves operational efficiency but also ensures more consistent and accurate product discovery across the entire catalog.
By combining enhanced customer experiences with operational improvements, semantic AI is transforming how e-commerce businesses serve their customers while streamlining internal processes. The technology’s ability to understand context, learn from interactions, and automate optimization makes it an essential tool for modern online retail.
Case Studies of Successful Implementation
Major e-commerce giants have transformed their platforms through innovative applications of semantic AI, delivering remarkable improvements in user experience and operational efficiency. Amazon’s implementation of convolutional neural networks (CNNs) has achieved up to 30% higher accuracy in image recognition and search functionality, transforming how customers discover products on their platform.
This technology extends beyond simple image matching. Amazon’s CNN-powered systems can now interpret subtle product features, understand context, and deliver increasingly relevant search results. This enhancement has improved the customer experience and led to more efficient product categorization and inventory management across millions of items.
Meanwhile, Alibaba has taken semantic AI implementation in a different direction through its groundbreaking ‘New Retail’ initiative. By seamlessly merging online and offline shopping experiences, Alibaba has created an ecosystem where AI powers everything from inventory management to personalized recommendations. Their Hema supermarket chain exemplifies this innovation, where customers can shop both digitally and physically while AI systems analyze shopping patterns to optimize stock levels and predict demand.
The success of these implementations is evident in the numbers. Alibaba’s New Retail strategy has enabled them to process over 400 million product predictions daily, while their smart stores have seen customer engagement increase significantly. The integration of semantic AI has allowed them to offer 30-minute delivery services within a 3km radius of their physical locations, a feat made possible by AI-powered logistics and demand forecasting.
These case studies demonstrate how semantic AI has evolved from a theoretical concept to a practical tool driving real business value. The technology’s ability to understand context, learn from user behavior, and adapt to changing patterns has made it an indispensable component of modern e-commerce infrastructure. As these systems continue to evolve, we can expect even more sophisticated applications that further blur the lines between digital and physical retail experiences.
Challenges and Future Directions
Despite the potential of semantic AI in e-commerce, several significant hurdles remain. Data privacy is a critical concern as systems process increasingly personal shopping behaviors and preferences to deliver tailored experiences. According to recent studies highlighted by Fast Simon, businesses must balance personalization with robust data protection measures.
Maintaining and updating semantic AI algorithms presents another substantial challenge. As consumer behaviors evolve and new shopping patterns emerge, these systems require constant refinement to remain effective. This ongoing need for updates demands significant resources and technical expertise, often stretching beyond what many e-commerce platforms can sustainably manage.
Looking toward the future, the industry appears poised for several breakthrough developments. Automated algorithm updates stand out as a particularly promising advancement, potentially solving one of the field’s most persistent challenges. By implementing self-learning systems, e-commerce platforms could maintain optimal performance while reducing the manual oversight currently required.
Another exciting frontier lies in semantic AI’s ability to process increasingly complex queries. Future systems will likely bridge the gap between natural language understanding and user intent more seamlessly, enabling more nuanced and accurate product recommendations. This evolution could fundamentally transform how consumers interact with e-commerce platforms, making product discovery more intuitive and personalized than ever before.
Moving forward, the success of semantic AI in e-commerce will largely depend on balancing innovation and responsibility. While the technology promises unprecedented levels of personalization and efficiency, its implementation must prioritize user privacy and data security. This careful equilibrium will be crucial in shaping the next generation of e-commerce experiences.
Last updated:
Disclaimer: The information presented in this article is for general informational purposes only and is provided as is. While we strive to keep the content up-to-date and accurate, we make no representations or warranties of any kind, express or implied, about the completeness, accuracy, reliability, suitability, or availability of the information contained in this article.
Any reliance you place on such information is strictly at your own risk. We reserve the right to make additions, deletions, or modifications to the contents of this article at any time without prior notice.
In no event will we be liable for any loss or damage including without limitation, indirect or consequential loss or damage, or any loss or damage whatsoever arising from loss of data, profits, or any other loss not specified herein arising out of, or in connection with, the use of this article.
Despite our best efforts, this article may contain oversights, errors, or omissions. If you notice any inaccuracies or have concerns about the content, please report them through our content feedback form. Your input helps us maintain the quality and reliability of our information.