Top Examples of Semantic AI in Action
Imagine a computer that understands not just your words, but their actual meaning. That’s the power of Semantic AI. Using techniques like natural language processing (NLP) and machine learning (ML), these systems can comprehend human communication with remarkable accuracy.
Semantic AI is transforming our daily interactions with technology. Whether it’s personalized Netflix recommendations, chatting with a bank’s virtual assistant, or finding exactly what you need on Google, Semantic AI works quietly behind the scenes to make it all possible.
What sets Semantic AI apart is its ability to grasp context and nuance, much like a human. When you ask Semantic AI-powered virtual assistants a question, they understand your intent and provide relevant, contextual responses.
From healthcare to retail, organizations are finding innovative ways to use Semantic AI. Doctors analyze medical literature and patient records quickly. Online stores create shopping experiences tailored to individual preferences. Customer service teams better understand and address customer needs.
This article will explore how Semantic AI is revolutionizing various industries. You’ll learn how this technology processes vast amounts of information, learns from patterns, and delivers insights that were once impossible to obtain. Discover how Semantic AI is shaping the future of human-machine interaction.
Enhancing Search Recommendations with Semantic AI
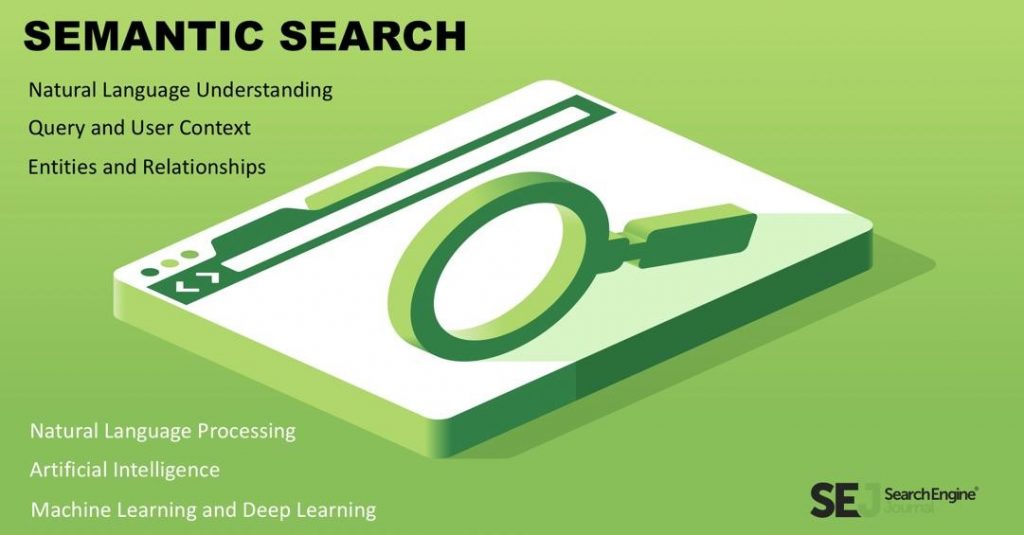
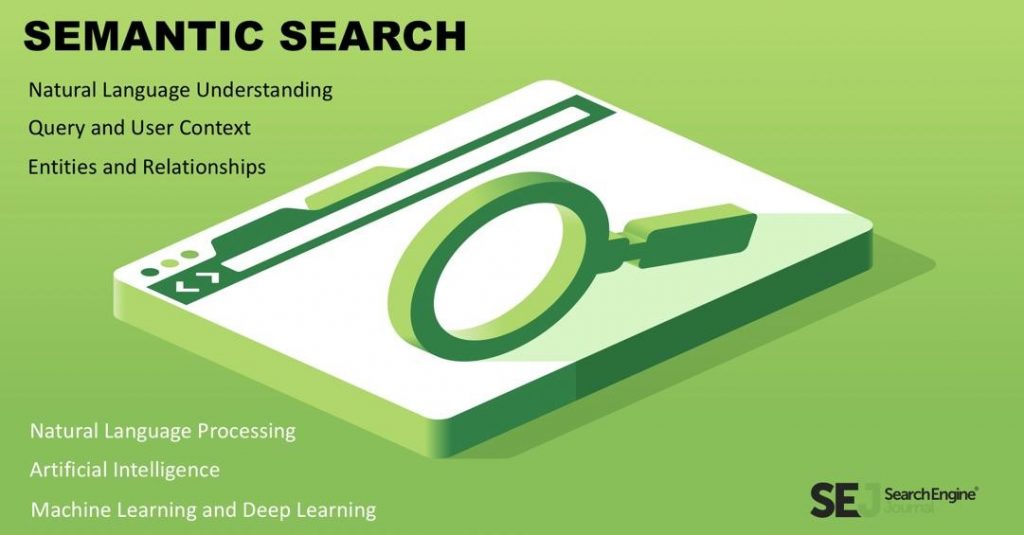
Modern search engines are transcending basic keyword matching by harnessing the power of semantic AI. Unlike traditional search methods that rely solely on exact word matches, semantic AI understands language more like humans do – grasping context, meaning, and intent behind queries.
When you search for apple
, semantic AI analyzes surrounding context to determine whether you’re interested in the fruit or the technology company. By examining factors like your previous searches, device type, and search patterns, the AI can distinguish between someone researching nutrition versus shopping for electronics. As reported by Shaped.ai, major platforms like Amazon, Spotify, and TikTok have already shifted from traditional keyword search to AI-powered semantic search to deliver more personalized results.
The technology works by converting search queries into mathematical representations called embeddings, which capture the meaning and relationships between words in a multi-dimensional space. This allows search engines to identify conceptually related content even when exact keywords don’t match. For instance, a search for workout gear
might return results for fitness equipment
or exercise accessories
because the AI understands these terms are semantically related.
Personalization plays a crucial role in semantic search effectiveness. The AI continuously learns from user interactions, analyzing click patterns, time spent on results, and engagement behaviors. This creates a feedback loop that helps refine and improve future search recommendations. When you frequently click on tech news after searching python
, the system learns to prioritize programming-related results over snake-related content for your future searches.
Semantic search enables shoppers to find products more easily and delivers personalized recommendations, enhancing the overall shopping experience.
Shaped.ai Research Report
For businesses and content creators, semantic AI’s ability to understand user intent translates into higher engagement rates and improved customer satisfaction. By delivering more relevant results that align with what users are actually seeking, semantic search reduces friction in the discovery process and helps connect people with the information they need more efficiently.
Revolutionizing Customer Support with Virtual Assistants
Modern virtual assistants, powered by semantic AI technology, are transforming how businesses interact with customers. Unlike traditional chatbots that rely on simple keyword matching, these AI-powered assistants leverage advanced natural language processing to truly comprehend customer inquiries and provide contextually relevant responses.
The sophistication of these virtual assistants lies in their ability to understand conversational nuances. When customers reach out with questions, these AI systems can recognize synonyms, interpret misspelled words, and even grasp informal language patterns, much like a human agent would. This deep language comprehension enables them to provide accurate, helpful responses even when customers phrase their questions in unexpected ways.
Take IBM’s Watson Assistant, for example, which demonstrates how semantic AI enhances customer support through personalized interactions. The system analyzes customer history and preferences to deliver tailored responses that feel natural and contextually appropriate. This level of personalization helps businesses build stronger connections with their customers while maintaining efficiency in their support operations.
The impact on operational efficiency is particularly noteworthy. Virtual assistants can handle multiple customer inquiries simultaneously, providing instant responses 24/7 without the limitations of traditional support hours. This continuous availability ensures customers receive timely assistance regardless of when they reach out, significantly improving the overall support experience.
Beyond just answering questions, these AI assistants contribute to a more streamlined support process. They can automatically categorize and route complex queries to appropriate human agents, complete with relevant context and background information. This intelligent handoff ensures that when human intervention is needed, agents can pick up conversations seamlessly and resolve issues more quickly.
We’ve observed that AI-powered virtual assistants can address customer inquiries approximately 70% of the time, leading to projected cost savings of $11 billion by 2023 across various industries.
Zartis Industry Research
Example | Cost Savings |
---|---|
Initial Cost | $100,000 |
Projected Cost | $80,000 |
Annual Savings | $20,000 |
Efficiency Improvement Example | $18 savings with minimal changes |
Targeted Savings Year 1 | $18 |
Targeted Savings Year 2 | $27 |
Targeted Savings Year 3 | $13 |
For businesses, the implementation of semantic AI-powered virtual assistants represents more than just a technological upgrade—it’s a strategic investment in customer satisfaction. By combining the efficiency of automation with the nuance of human-like understanding, these systems help create more engaging, effective, and satisfying customer support experiences.
Semantic AI in Natural Language Processing Applications
Natural Language Processing has entered an exciting new era with Semantic AI technologies revolutionizing how machines interpret and process human language. Unlike traditional keyword-based systems, Semantic AI brings nuanced understanding and contextual awareness to NLP applications, making human-machine interactions more natural and effective.
At the forefront of this evolution is language translation, where Semantic AI’s contextual understanding enables more accurate and culturally-aware translations. By using natural language processing and machine learning techniques, these systems can now grasp idioms, cultural references, and context-dependent meanings that once confused automated translators.
Text summarization represents another breakthrough application, where Semantic AI excels at distilling lengthy documents into concise, meaningful summaries while preserving key information and context. Rather than simply extracting sentences based on statistical patterns, these systems understand the relationships between ideas and can generate coherent summaries that capture the essence of the original text.
Sentiment analysis has also been transformed by Semantic AI’s ability to detect subtle emotional nuances in text. These systems now recognize sarcasm, implied meanings, and complex emotional states that traditional keyword-based approaches often missed. For instance, they can differentiate between “This movie was killer!” (positive) and “This movie was a killer of my time” (negative) by understanding the contextual usage.
The practical applications of these technologies extend across industries. Customer service centers use sentiment analysis to gauge customer satisfaction in real-time, while global businesses rely on enhanced language translation for seamless international communication. News organizations and researchers benefit from intelligent text summarization to process vast amounts of information efficiently.
The network is known to enhance the ability of semantic analysis in artificial intelligence to connect disparate pieces of information, enabling more accurate and contextually relevant insights.
Appinventiv
Looking ahead, these applications continue to evolve as Semantic AI becomes more sophisticated. The technology’s ability to understand context, recognize patterns, and learn from interactions promises even more intuitive and capable NLP systems in the future, further bridging the gap between human and machine communication.
Integrating Semantic AI in Knowledge Management
Modern enterprises face an overwhelming challenge: managing vast repositories of information while ensuring quick, relevant access for decision-makers. Semantic AI transforms how organizations process, organize, and retrieve their institutional knowledge by understanding the context and relationships between different pieces of information. Unlike traditional systems that rely on exact keyword matches, semantic AI comprehends the intent behind queries, much like a human would interpret a question. For example, when an employee searches for “latest compliance requirements,” the system understands to include recent regulatory updates, related policy changes, and implementation guidelines.
In enterprise settings, semantic AI excels at organizing unstructured data into meaningful, interconnected formats. Tools powered by semantic AI can automatically extract key entities from documents, apply consistent tags, and build enterprise knowledge graphs, reducing information retrieval time from days to minutes. This automated organization ensures that valuable insights don’t get buried in departmental silos.
The technology’s impact on decision-making is particularly noteworthy in large organizations. When leaders need to make strategic choices, semantic AI helps surface relevant past experiences, market analyses, and internal expertise. Consider a product development team evaluating a new feature. Semantic AI can quickly connect customer feedback, technical documentation, and market research to support informed decisions.
Knowledge sharing across departments becomes more efficient with semantic AI’s natural language processing capabilities. The system can identify connections between seemingly unrelated documents, helping teams discover relevant insights from other departments. For instance, marketing strategies that worked in one region can be easily surfaced when another regional team searches for successful campaign examples.
Natural language processing capabilities enable semantic AI to understand and process information the way humans do, making knowledge retrieval more intuitive and efficient. Enterprise-wide collaboration improves as semantic AI helps bridge terminology gaps between departments. What the IT team calls “system architecture” might be termed “technical infrastructure” by operations, but semantic AI understands these variations, ensuring relevant information reaches the right people regardless of their specific terminology.
Most importantly, semantic AI continuously learns from user interactions, improving its understanding of organizational context over time. This adaptive capability means the system becomes more valuable as it processes more queries and content, leading to increasingly precise and relevant results for users across the enterprise.
Addressing Challenges in Semantic AI Implementation
Semantic AI has great potential for transforming how organizations process and use data, but implementing these systems presents several significant hurdles that must be carefully managed. Data quality is a fundamental challenge, with organizations struggling to maintain consistent, accurate, and comprehensive datasets that fuel their AI systems.
According to implementation studies, poor data quality can significantly undermine AI initiatives, requiring organizations to establish robust data governance frameworks and standardization practices. This includes implementing comprehensive data validation protocols and regular auditing processes to ensure data integrity.
Scalability is another critical challenge as organizations expand their semantic AI deployments. Systems must be designed to handle growing data volumes and increasing computational demands without compromising performance. Companies frequently encounter bottlenecks when their infrastructure fails to keep pace with expanding semantic processing requirements, necessitating careful capacity planning and architecture optimization.
Integration with existing systems poses perhaps the most complex challenge. Legacy systems, often built without semantic capabilities in mind, require significant adaptation to work seamlessly with new AI implementations. Organizations must carefully orchestrate the integration process to prevent disruptions to ongoing operations while ensuring that semantic AI capabilities enhance rather than hinder existing workflows.
To overcome these challenges, successful organizations typically adopt a phased approach to implementation. This includes establishing clear data quality standards from the outset, investing in scalable infrastructure that can grow with demand, and developing comprehensive integration strategies that account for both technical and organizational requirements. Regular monitoring and optimization of these systems ensure they continue to deliver value as the organization’s needs evolve.
The success of semantic AI implementation hinges on three critical factors: maintaining pristine data quality, ensuring robust scalability, and achieving seamless integration with existing systems. Organizations that excel in these areas position themselves to unlock the full potential of semantic AI.
Dr. Prashanth Southekal, Managing Principal of DBP Institute
Additionally, organizations must invest in proper training and change management initiatives to ensure team members can effectively utilize semantic AI systems. This human element often proves crucial for successful implementation, as even the most sophisticated systems require skilled operators to maximize their potential.
Looking ahead, organizations that successfully address these implementation challenges position themselves to leverage semantic AI’s full capabilities, from enhanced data understanding to more sophisticated decision-making processes. The key lies in maintaining a balanced approach that addresses technical requirements while ensuring the system remains accessible and valuable to end users.
Future Prospects of Semantic AI
Semantic AI’s trajectory points toward unprecedented capabilities in understanding and processing complex data. Recent industry analyses reveal that organizations are increasingly embracing this technology, with adoption rates surging to 72% across sectors—a dramatic increase from previous years according to McKinsey’s latest research.
The future landscape of Semantic AI promises more sophisticated real-time data analysis capabilities. These advancements will enable organizations to process vast amounts of unstructured information with unprecedented speed and accuracy, transforming how businesses make decisions and respond to market changes. From healthcare diagnostics to financial risk assessment, the applications continue to expand into new territories.
Industry adoption is accelerating across sectors, particularly in healthcare, finance, and manufacturing. This broader integration signals a shift from experimental implementations to mission-critical applications. We are witnessing the emergence of more nuanced applications that can understand context, intent, and subtle linguistic variations with remarkable precision.
What makes this evolution particularly exciting is the convergence of Semantic AI with other cutting-edge technologies. The integration with knowledge graphs and natural language processing is creating systems that can not only understand complex queries but also provide contextually relevant insights that mirror human-like reasoning patterns.
Looking ahead, we can expect Semantic AI to become increasingly embedded in enterprise operations. The technology’s ability to enhance automation, improve decision-making accuracy, and deliver personalized experiences positions it as a cornerstone of future business infrastructure. As organizations continue to recognize its potential, we are likely to see even more innovative applications emerge that push the boundaries of what’s possible in artificial intelligence.
Last updated:
Disclaimer: The information presented in this article is for general informational purposes only and is provided as is. While we strive to keep the content up-to-date and accurate, we make no representations or warranties of any kind, express or implied, about the completeness, accuracy, reliability, suitability, or availability of the information contained in this article.
Any reliance you place on such information is strictly at your own risk. We reserve the right to make additions, deletions, or modifications to the contents of this article at any time without prior notice.
In no event will we be liable for any loss or damage including without limitation, indirect or consequential loss or damage, or any loss or damage whatsoever arising from loss of data, profits, or any other loss not specified herein arising out of, or in connection with, the use of this article.
Despite our best efforts, this article may contain oversights, errors, or omissions. If you notice any inaccuracies or have concerns about the content, please report them through our content feedback form. Your input helps us maintain the quality and reliability of our information.