Semantic AI: The Future of Intelligent Systems
Ever wondered how machines could truly understand us, not just process our words? Welcome to the world of Semantic AI, where artificial intelligence leaps beyond mere data crunching to grasp the essence of information.
Imagine a computer that doesn’t just read your email but comprehends the urgency, emotion, and context behind your words. That’s the promise of Semantic AI—a groundbreaking approach reshaping how machines interact with our complex, nuanced world.
But what exactly is Semantic AI, and why should you care? At its core, Semantic AI focuses on understanding the meaning and context of data, much like a human would. It’s not just about recognizing words or patterns; it’s about comprehending the intricate web of relationships and implications that humans navigate effortlessly in everyday communication.
This isn’t just another tech buzzword. Semantic AI is already transforming industries, from healthcare diagnostics to financial fraud detection. It’s the technology that allows virtual assistants to engage in more natural conversations and helps autonomous vehicles make split-second decisions based on complex environmental cues.
We’ll explore the groundbreaking techniques powering Semantic AI, its real-world applications that are changing the game, and the exciting future directions that promise to revolutionize intelligent systems. Whether you’re a tech enthusiast, a business leader, or simply curious about the future of AI, understanding Semantic AI is crucial in our increasingly data-driven world.
Discover how Semantic AI is bridging the gap between human intuition and machine intelligence, paving the way for smarter, more intuitive technologies that could redefine our relationship with artificial intelligence.
Understanding Semantic AI and Its Importance
Imagine a world where machines understand what we mean. That’s the promise of Semantic AI, a technology transforming our interaction with computers and various industries.
At its core, Semantic AI combines Natural Language Processing (NLP) and Natural Language Understanding (NLU). These technologies enable machines to grasp the context and meaning behind human language.
NLP acts as the ears and eyes, breaking down text and speech into structured data for analysis. NLU serves as the brain, interpreting that data to understand intent, emotions, and nuances.
Why does this matter? Consider this example: You ask a virtual assistant, “What’s the weather like for my beach trip tomorrow?” A traditional system might provide a weather forecast. A Semantic AI-powered assistant could respond, “The weather looks perfect for the beach tomorrow – sunny and 80 degrees. Don’t forget your sunscreen!” It understands the context of your question and provides a more helpful response.
This level of understanding opens up possibilities across various industries:
- In healthcare, Semantic AI can analyze medical records and research papers to assist doctors in making accurate diagnoses and treatment plans.
- For e-commerce, it enables personalized product recommendations based on a deep understanding of customer preferences and behavior.
- In customer service, Semantic AI powers chatbots and virtual assistants that handle complex queries with accuracy and empathy.
The importance of Semantic AI lies in its ability to bridge the gap between human communication and machine processing. By enabling more natural interactions with technology, it makes our digital experiences more efficient and personalized.
Semantic AI is not just about understanding words, but about comprehending the rich tapestry of meaning, context, and relationships that underpin human communication.
As this technology evolves, we can expect more sophisticated applications that blur the line between human and machine understanding. From natural conversational interfaces to advanced decision support systems, Semantic AI promises to enhance our use of artificial intelligence in daily life.
The future of human-machine interaction is here, and it speaks our language. Are you ready to embrace the semantic revolution?
Applications of Semantic AI Across Various Domains
Semantic AI is transforming how machines understand and process information across multiple fields. By structuring and organizing data to capture meaning and context, Semantic AI enables sophisticated AI systems to tackle complex real-world tasks. Here are some key domains where Semantic AI is making a significant impact.
In natural language processing (NLP), Semantic AI powers major advances in machine comprehension of human language. By mapping words and phrases to concepts and relationships, NLP systems can grasp nuanced meanings and contextual subtleties. For example, large language models like GPT-3 leverage semantic knowledge to engage in human-like dialogue, answer questions, and generate creative text. In machine translation, Semantic AI helps capture the intended meaning rather than just literal word-for-word translations, resulting in more natural and accurate translations across languages.
Semantic AI is also transforming robotics, enabling machines to better understand their environment and interact more intelligently. Autonomous robots can recognize objects, navigate complex spaces, and collaborate with humans more effectively thanks to improved semantic understanding. In the automotive industry, Semantic AI drives the development of safer and more capable self-driving vehicles. These systems use semantic segmentation to interpret road scenes, identifying the presence and relevance of objects to driving decisions.
Semantic AI is revitalizing expert systems, which emulate human decision-making. By incorporating domain-specific knowledge and reasoning capabilities, these systems provide sophisticated advice and support in fields like medicine, finance, and engineering. For example, in healthcare, semantic AI-powered systems can analyze patient data, medical literature, and clinical guidelines to assist doctors in diagnosis and treatment planning. This improves the accuracy of medical decisions and helps standardize care across different healthcare providers.
In cybersecurity, Semantic AI enhances threat detection and response capabilities. By understanding semantic relationships between different network events and behaviors, AI systems can more accurately identify potential security breaches and sophisticated attacks. Semantic AI enables cybersecurity tools to understand the context and intent behind network activities, resulting in fewer false positives and more effective threat mitigation. For instance, AI-powered security systems can now detect novel malware variants by understanding their semantic similarities to known threats, even if their code is substantially different.
As Semantic AI continues to evolve, its applications across these domains and others will grow more sophisticated and impactful. By enabling machines to understand and reason about the world in ways more similar to humans, Semantic AI paves the way for more intelligent, capable, and trustworthy AI systems across industries.
Challenges in Implementing Semantic AI
Researchers face several critical hurdles in bringing Semantic AI to practical applications. Let’s explore key challenges and innovative solutions being developed to overcome them.
Scalability Issues
One of the most pressing issues in Semantic AI is scalability. As knowledge bases expand, processing and reasoning over massive amounts of interconnected information becomes increasingly resource-intensive, particularly in large-scale semantic networks and enterprise knowledge graphs.
Researchers are exploring advanced techniques in distributed computing. For example, some teams are developing methods to partition knowledge graphs across multiple systems, allowing for parallel processing. Others are implementing more efficient storage systems optimized for semantic data structures. These approaches aim to maintain performance even as data volumes grow.
Ambiguity Challenges
Ambiguity presents another obstacle. Natural language and real-world information often contain inherent uncertainties and multiple interpretations. Consider the word “bank”—an AI system must determine whether it refers to a financial institution, a river bank, or something else based on context.
Innovative solutions include hybrid approaches that combine symbolic and neural methods. These systems leverage rule-based reasoning while incorporating the pattern recognition capabilities of neural networks, allowing for more nuanced interpretation of ambiguous information.
Computational Complexity
As Semantic AI systems become more sophisticated, they often require significant computational resources, especially for real-time reasoning tasks. This creates a delicate balancing act between the expressiveness of knowledge representation and computational efficiency.
Researchers are developing optimized algorithms and leveraging advances in hardware acceleration. Some teams are exploring quantum computing for complex semantic reasoning tasks. Additionally, there is growing interest in edge computing architectures that can perform semantic processing closer to data sources, reducing latency and computational burden on centralized systems.
Integrating Diverse Knowledge Representations
Modern AI systems often need to combine various types of knowledge, from structured databases to unstructured text and multimedia content. Creating unified representation schemes that can effectively handle this diversity while maintaining consistency and accuracy remains an ongoing challenge.
Researchers are addressing this through the development of more flexible knowledge representation formats and improved ontology mapping techniques. Some innovative approaches involve using machine learning to automatically detect and reconcile inconsistencies between different knowledge sources, creating a more cohesive semantic understanding.
Despite these challenges, the future of Semantic AI looks bright. As one researcher noted, “Recent results show that efficient and practical implementations of expressive languages are feasible, despite their theoretical complexity.” By continuing to innovate and refine these technologies, we’re moving closer to AI systems that can truly understand and reason about the world in ways that mirror human cognition.
The key to unlocking Semantic AI’s potential lies in finding the right balance between expressiveness, efficiency, and practical applicability. It’s not just about raw computational power, but about representing and reasoning with knowledge in truly intelligent ways.
As we push the boundaries of what’s possible in Semantic AI, these challenges drive innovation and bring us closer to AI systems that can tackle complex real-world problems with human-like understanding. The journey ahead is exciting, and the potential applications of mature Semantic AI technologies are truly transformative across industries.
SmythOS: Enhancing Knowledge Representation with Visual Tools
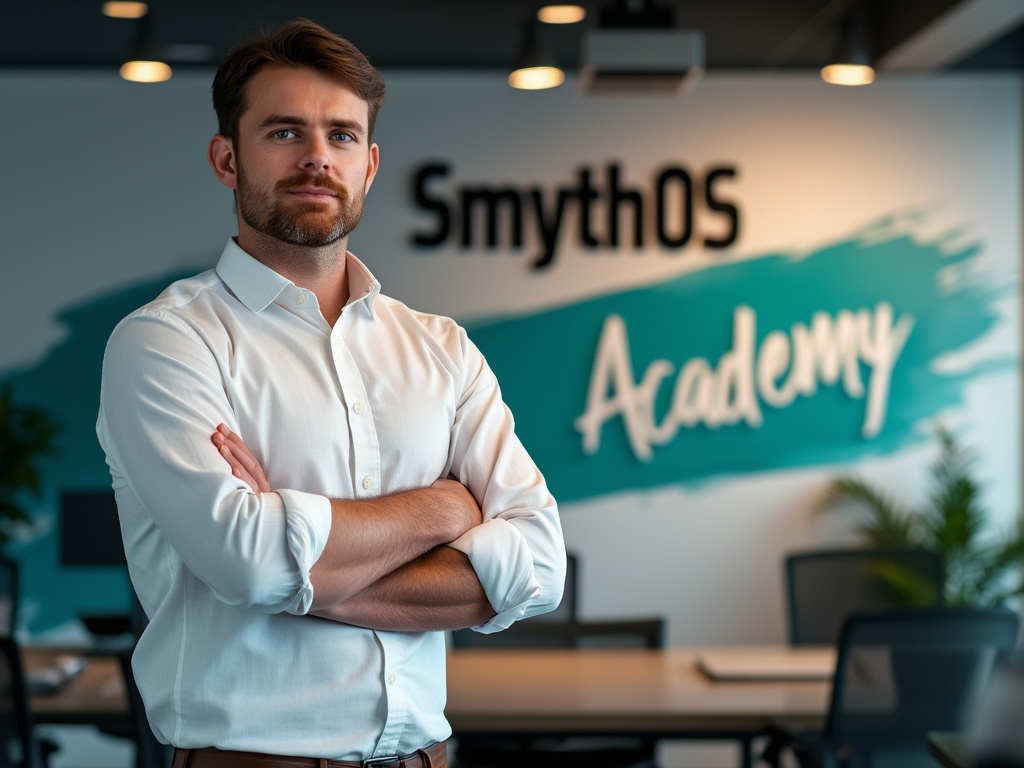
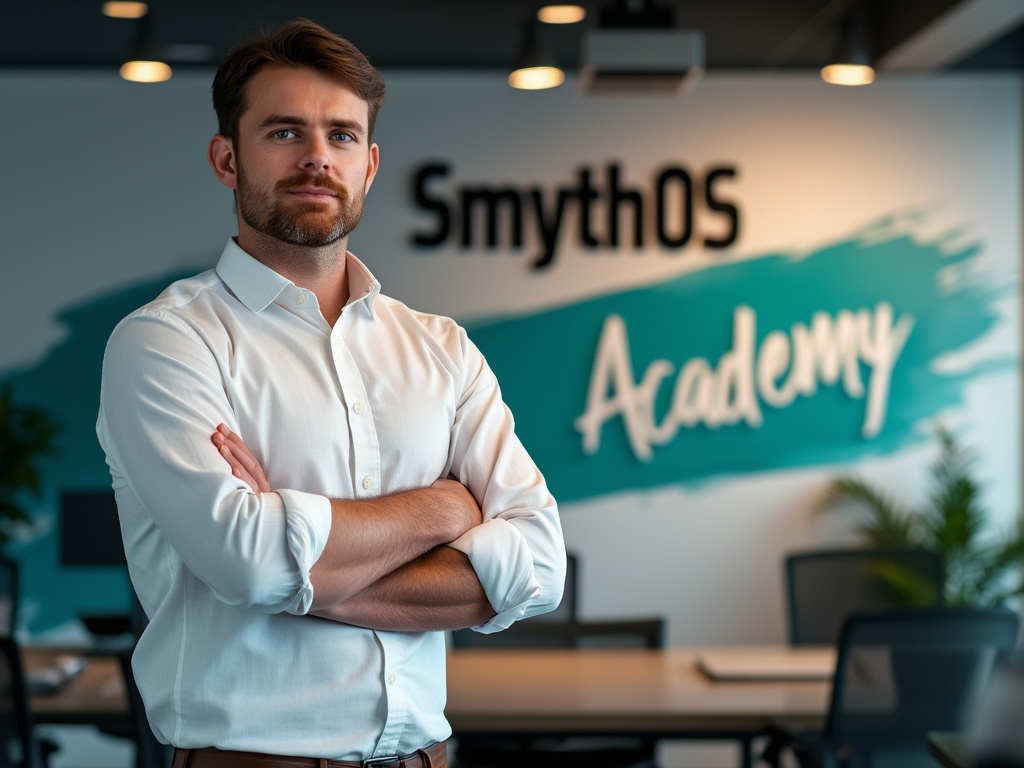
SmythOS emerges as a game-changer for organizations grappling with complex knowledge structures. This innovative platform offers advanced tools designed to transform the way teams build and manage knowledge graphs, addressing the need for more intuitive and powerful AI systems.
At the heart of SmythOS lies its visual workflow builder—a feature transforming how developers and data scientists approach knowledge representation. Teams can now visualize and manipulate data connections with ease, reducing the learning curve and accelerating development cycles.
SmythOS also excels in maintenance. The platform’s integrated debugging environment provides clear, visual insights into the inner workings of knowledge graphs, empowering developers to identify and resolve issues with precision, ensuring robust AI performance.
Interoperability is another advantage of SmythOS. The platform integrates seamlessly with major graph databases, allowing organizations to leverage their existing data infrastructure. This compatibility makes SmythOS a versatile choice for businesses of all sizes and sectors.
In an era of frequent data breaches, SmythOS takes security seriously. The platform offers enterprise-grade security features, providing peace of mind for organizations handling sensitive information. It balances robust protection with user-friendly operation, a rare feat in enterprise software.
Furthermore, SmythOS does not require teams to be coding experts. Its intuitive interface and visual tools democratize the process of building sophisticated AI systems, allowing a broader range of professionals to contribute to knowledge graph development. This accessibility saves time and resources, opening up new possibilities for innovation across departments.
SmythOS is not just a tool; it’s a catalyst for AI innovation. By visualizing the complex, we make it comprehensible. By simplifying the difficult, we make it achievable.
Alexander De Ridder, Co-Founder and CTO of SmythOS
As organizations continue to handle increasing volumes of data, tools like SmythOS become essential. By providing a visual, intuitive approach to knowledge representation, seamless integration capabilities, and robust security, SmythOS is positioning itself as a crucial ally in harnessing the full power of AI. For teams looking to stay ahead in the evolving world of artificial intelligence, SmythOS offers a compelling vision of a more visual, accessible, and powerful approach to knowledge representation.
Conclusion: The Evolving Landscape of Semantic AI
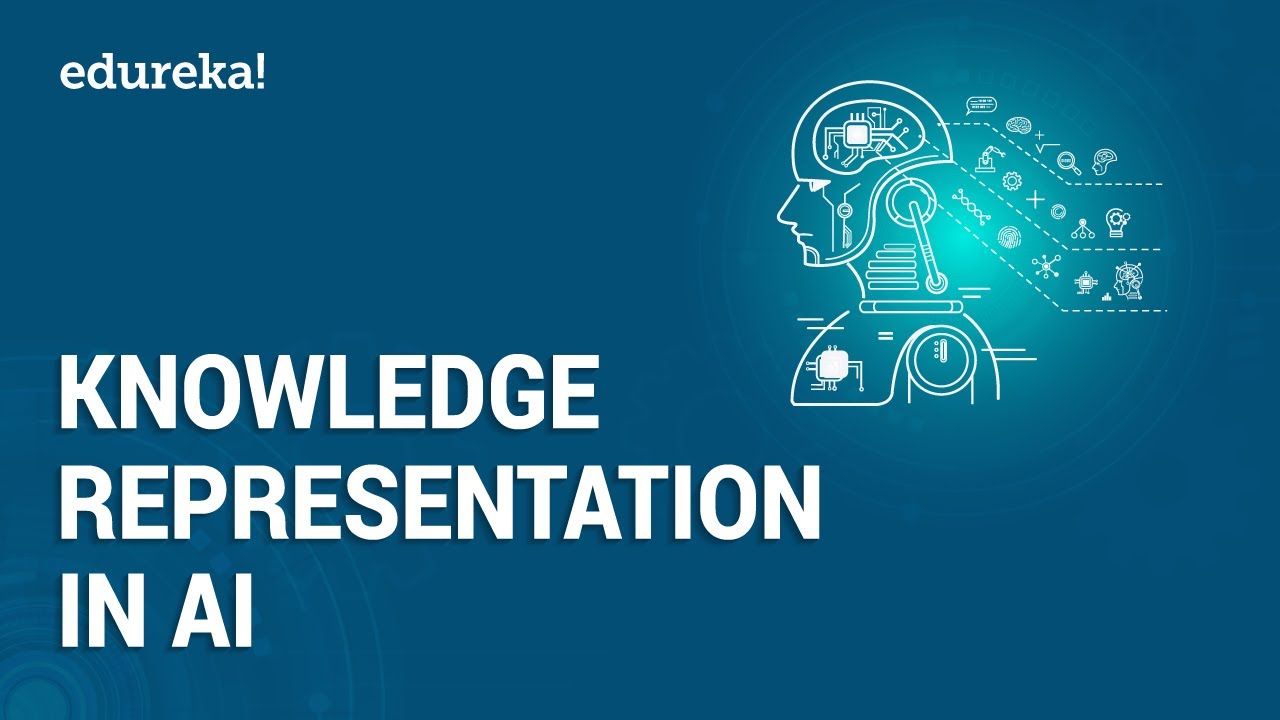
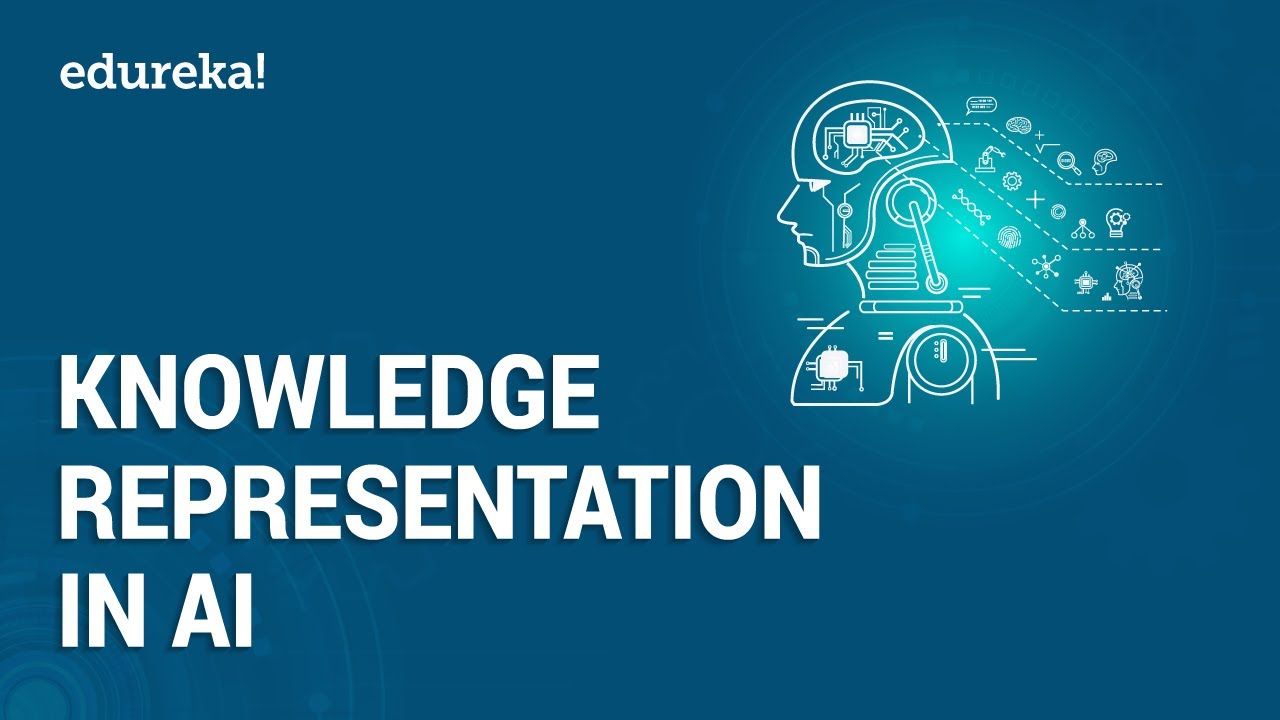
Knowledge Representation in AI illustrated in a unique design. – Via edisonlabs.net
Semantic AI is emerging as a transformative force, reshaping intelligent systems by harnessing the power of context and meaning. This technology is not just improving existing systems—it’s redefining possibilities in machine intelligence.
Significant advancements in knowledge representation and reasoning mark the journey toward sophisticated AI systems. Techniques like ontologies, knowledge graphs, and semantic networks are becoming the backbone of AI systems that can understand and interact with the world meaningfully. These developments pave the way for AI to process and comprehend information similarly to human understanding.
However, challenges such as scalability, ambiguity in natural language, and integrating diverse knowledge sources must be addressed. Innovative approaches and collaborative efforts across the AI community are essential to overcome these hurdles.
Platforms like SmythOS play a pivotal role in this evolving landscape. By providing robust tools for advanced knowledge representation, SmythOS empowers developers and researchers to build AI systems that are more contextually aware and capable of nuanced reasoning. This development is not just about creating smarter machines—it’s about developing AI that can augment human intelligence and decision-making processes.
The potential applications of semantic AI are vast and transformative. From enhancing natural language processing to revolutionizing data analytics, semantic AI brings us closer to the dream of artificial general intelligence. This evolving landscape is reshaping our understanding of intelligence and opening new frontiers in human-machine collaboration.
Semantic AI stands at the forefront of the next great leap in artificial intelligence. By continuing to innovate and address current challenges, we are building better AI systems and laying the groundwork for a future where machines can understand, reason, and interact with the world in ways once considered science fiction. The journey ahead is complex but filled with immense potential, promising to usher in an era of AI that is more intuitive, capable, and aligned with human cognition.
Last updated:
Disclaimer: The information presented in this article is for general informational purposes only and is provided as is. While we strive to keep the content up-to-date and accurate, we make no representations or warranties of any kind, express or implied, about the completeness, accuracy, reliability, suitability, or availability of the information contained in this article.
Any reliance you place on such information is strictly at your own risk. We reserve the right to make additions, deletions, or modifications to the contents of this article at any time without prior notice.
In no event will we be liable for any loss or damage including without limitation, indirect or consequential loss or damage, or any loss or damage whatsoever arising from loss of data, profits, or any other loss not specified herein arising out of, or in connection with, the use of this article.
Despite our best efforts, this article may contain oversights, errors, or omissions. If you notice any inaccuracies or have concerns about the content, please report them through our content feedback form. Your input helps us maintain the quality and reliability of our information.