Streamlining AI Integration with Semantic AI
Imagine a world where machines not only process data but truly understand it. Semantic AI is transforming enterprises by adding a crucial element to artificial intelligence: context. Through sophisticated knowledge graphs and natural language understanding, organizations can now extract deeper meaning from their vast data resources in unprecedented ways.
At its core, Semantic AI represents a fundamental shift in how AI systems interpret information. Unlike traditional AI that relies on simple pattern matching, semantic technologies grasp subtle nuances and relationships within data, enabling more intelligent and contextually aware processing. This advanced understanding allows organizations to break down data silos, enhance search capabilities, and automate complex analysis tasks that once required extensive manual effort.
The business impact of this semantic revolution extends far beyond basic data management. Companies implementing semantic AI are witnessing transformative changes in their decision-making processes. By creating sophisticated knowledge graphs, semantic AI connects information across various sources, making it easier for employees to find relevant insights when needed and enabling more accurate, context-aware business decisions.
What makes semantic AI particularly powerful is its ability to bridge the gap between human communication and machine processing. Through advanced natural language processing capabilities, these systems can understand the intent behind queries, grasp contextual meanings, and deliver more relevant results. This enhanced comprehension leads to more natural interactions between users and AI systems, fundamentally changing how organizations leverage their data assets.
This comprehensive exploration examines how semantic AI is revolutionizing data integration, its practical applications across industries, and future developments that promise even greater capabilities. From enhancing customer experiences to streamlining operations, semantic AI is setting new standards for intelligent data processing and business automation.
Key Features of Semantic AI
Semantic AI represents a significant advancement in artificial intelligence, transforming how machines comprehend and process information. Unlike traditional AI systems that rely on simple pattern matching, semantic AI understands the actual meaning and context behind data.
Natural Language Understanding (NLU) is a cornerstone feature of semantic AI. This capability enables machines to grasp the nuances of human communication, going beyond mere keyword recognition. When analyzing text data, semantic AI doesn’t just look at keywords – it understands the relationships between words, phrases, and concepts, helping to identify the true meaning behind what is being said.
Knowledge graphs are another pivotal component of semantic AI systems. These structures act as vast networks of interconnected information, allowing machines to establish relationships between different pieces of data. Think of knowledge graphs as digital maps of information where concepts, entities, and their relationships are all connected, enabling AI to make more informed decisions and provide more accurate responses to complex queries.
Contextual search capabilities represent the third major pillar of semantic AI. This feature elevates traditional search functionality by understanding the intent and context behind queries. Rather than matching exact keywords, contextual search considers the broader meaning and relationships between concepts, delivering more relevant and precise results.
The integration of these features enables intelligent data processing that mirrors human-like understanding. In healthcare settings, for instance, semantic AI can comprehend medical terminologies in context, connecting symptoms with potential diagnoses while considering patient history and current medical research. This level of sophisticated processing makes semantic AI particularly valuable in complex fields where precise interpretation of information is crucial.
Semantic AI goes beyond the form of data to interpret nuances and deliver more meaningful insights, revolutionizing how machines assist in decision-making processes.
The practical implications of these features extend across industries. In finance, semantic AI can analyze market reports while understanding complex financial terminology and market contexts. In legal settings, it can process vast amounts of case law while grasping subtle legal precedents and their relationships to current cases. This versatility and depth of understanding make semantic AI an increasingly indispensable tool in our data-driven world.
Challenges in Implementing Semantic AI
Organizations adopting semantic AI face significant hurdles that extend beyond typical technical implementations. Data integration is at the core of these challenges, involving the complex process of combining information from diverse sources while preserving meaning and context. For example, a healthcare provider implementing semantic AI must carefully integrate patient records, lab results, and medical literature in ways that maintain critical relationships between different types of medical data.
Data integrity poses another crucial challenge in semantic AI deployment. Unlike traditional AI systems, semantic AI requires not just accurate data, but also reliable connections between data points. Financial institutions, for instance, must ensure that customer transaction data remains accurate while simultaneously maintaining the integrity of relationships between accounts, users, and financial products. Any break in these semantic connections can lead to flawed insights and decision-making.
Managing bias represents perhaps the most nuanced challenge in semantic AI implementation. Research shows that AI systems can perpetuate and amplify existing biases, particularly when processing complex semantic relationships. A semantic AI system analyzing job applications might unconsciously reinforce gender stereotypes by making assumptions about career paths based on historical data patterns.
Transparency emerges as another critical consideration, as organizations must be able to explain how their semantic AI systems arrive at conclusions. This becomes especially challenging when dealing with complex knowledge graphs and inference chains. For example, a semantic AI system used in criminal justice needs to provide clear explanations for its recommendations to ensure accountability and fairness.
The scale of data processing adds another layer of complexity. Semantic AI systems must handle vast amounts of interconnected information while maintaining performance and accuracy. Organizations often struggle to balance the need for comprehensive semantic analysis with practical limitations on computing resources and processing time.
Technical integration challenges also arise when implementing semantic AI alongside existing systems. Legacy databases and applications may not be designed to support the rich semantic relationships required for effective AI operation. Organizations must carefully plan how to bridge these technological gaps without disrupting critical business operations.
Challenge | Description | Solution |
---|---|---|
Data Integration | Combining information from diverse sources while preserving meaning and context. | Careful integration planning and use of advanced data integration tools. |
Data Integrity | Ensuring accurate data and reliable connections between data points. | Implement robust data validation and maintenance processes. |
Managing Bias | AI systems can perpetuate and amplify existing biases. | Implement bias detection and mitigation strategies during AI development. |
Transparency | Explaining how AI systems arrive at conclusions, especially with complex knowledge graphs. | Develop clear documentation and provide explainability tools. |
Scale of Data Processing | Handling vast amounts of interconnected information while maintaining performance and accuracy. | Optimize computational resources and use scalable AI infrastructure. |
Technical Integration | Integrating semantic AI with legacy databases and applications. | Bridge technological gaps with middleware and integration platforms. |
Practical Applications of Semantic AI
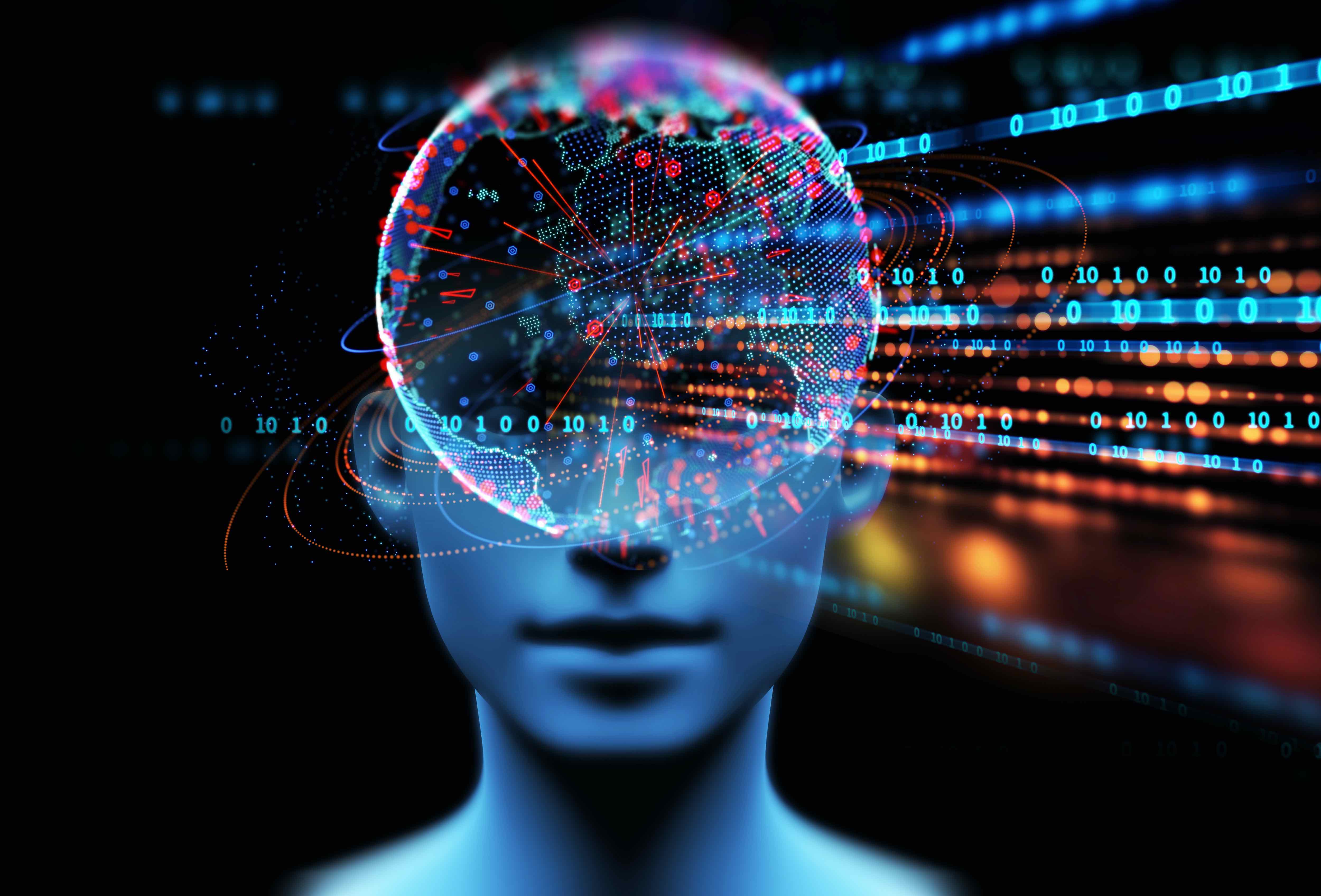
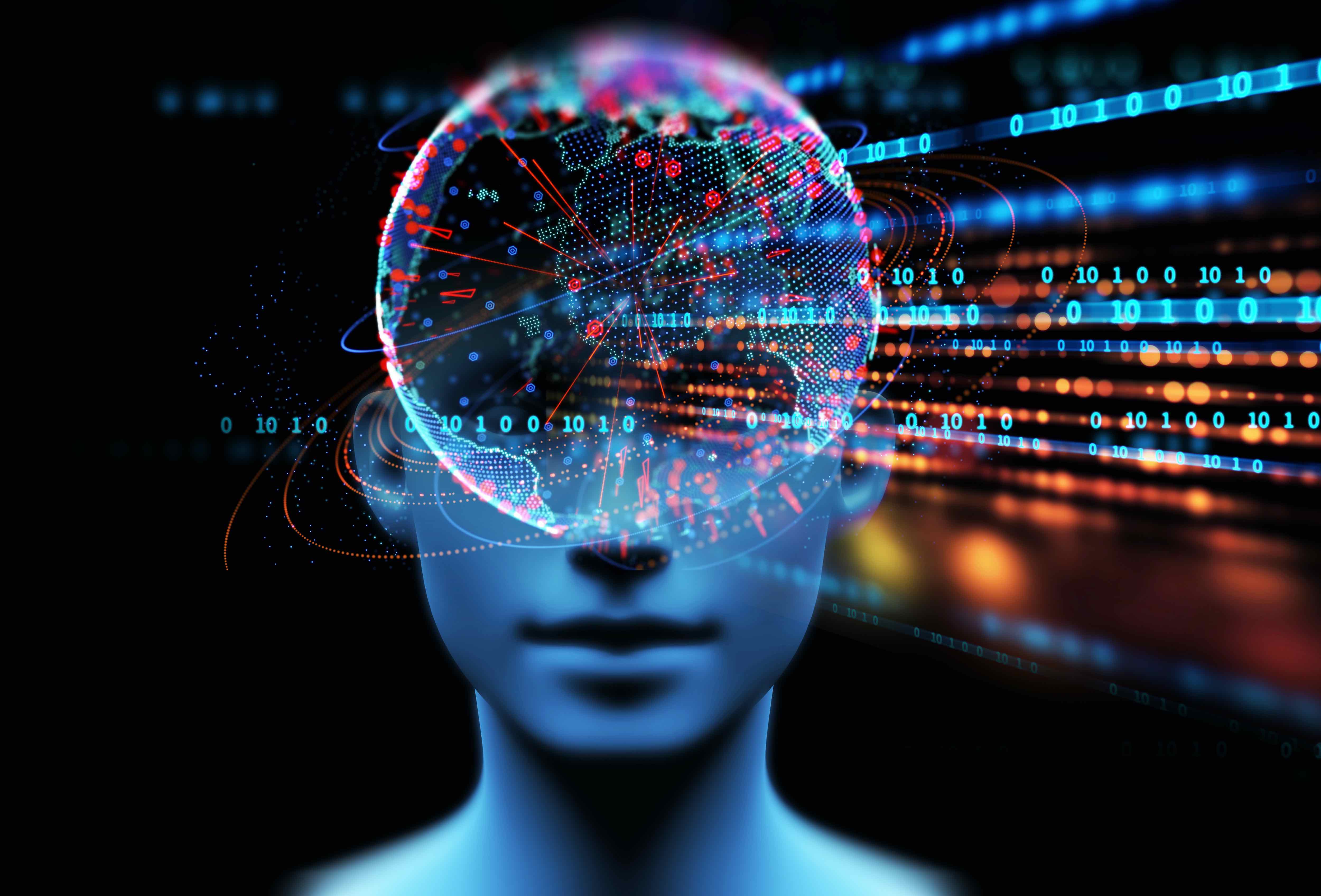
Semantic AI is transforming how organizations process information across sectors. By leveraging advanced natural language processing and machine learning, this technology enhances contextual understanding and decision-making. Studies show that Semantic AI significantly improves the accuracy of data analysis and classification, leading to more reliable business insights.
In healthcare, Semantic AI powers systems that interpret complex medical terminology and relationships between symptoms, diagnoses, and treatments. For example, when analyzing patient records, Semantic AI can understand subtle connections between health indicators, enabling more accurate diagnoses and personalized treatment recommendations. This helps healthcare providers make informed decisions while reducing the risk of medical errors.
The technology’s impact on customer service has been equally transformative. Semantic AI-powered virtual assistants can grasp the nuanced meaning behind customer queries, moving beyond simple keyword matching. These systems maintain context throughout conversations, remembering previous interactions and applying that understanding to new queries, much like a skilled human customer service representative. This leads to more natural, efficient, and satisfactory customer experiences.
In financial services, Semantic AI enhances risk assessment and decision-making processes. The technology can analyze vast amounts of financial data while understanding complex relationships between market indicators, company performance metrics, and economic factors. This deep contextual understanding enables more accurate predictions and better-informed investment strategies.
Sector | Key Applications | Benefits |
---|---|---|
Technology | Software development, cloud computing, hardware innovations | Enhanced efficiency and innovation |
Financial Services | Fraud detection, risk management, customer service chatbots, algorithmic trading | Improved accuracy, security, and user experience |
Healthcare | Diagnostics, patient care, drug discovery, genome sequencing | More accurate diagnoses, personalized treatment, reduced medical errors |
Retail | Targeted marketing, search engine optimization | Enhanced customer experience, increased sales |
Education | Personalized learning, automated grading | Improved learning outcomes, reduced workload for educators |
Construction | Predictive maintenance, optimization of operations | Increased efficiency, reduced costs |
Recommendation systems have seen dramatic improvements through Semantic AI. Unlike traditional systems that rely on basic pattern matching, Semantic AI-powered recommendations consider multiple contextual factors simultaneously. For instance, when suggesting products to customers, these systems analyze not just purchase history but also seasonal context, current trends, and individual user preferences to provide highly relevant suggestions.
The efficiency gains from Semantic AI implementations are particularly noteworthy in operational contexts. Organizations report significant reductions in processing time for complex tasks like document analysis and data classification. These improvements stem from the technology’s ability to understand context and relationships within information, eliminating many manual review steps that were previously necessary.
Future Directions of Semantic AI
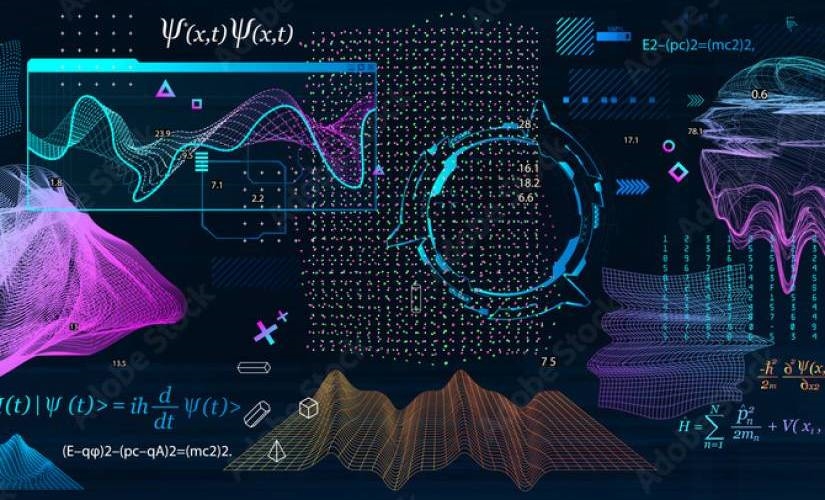
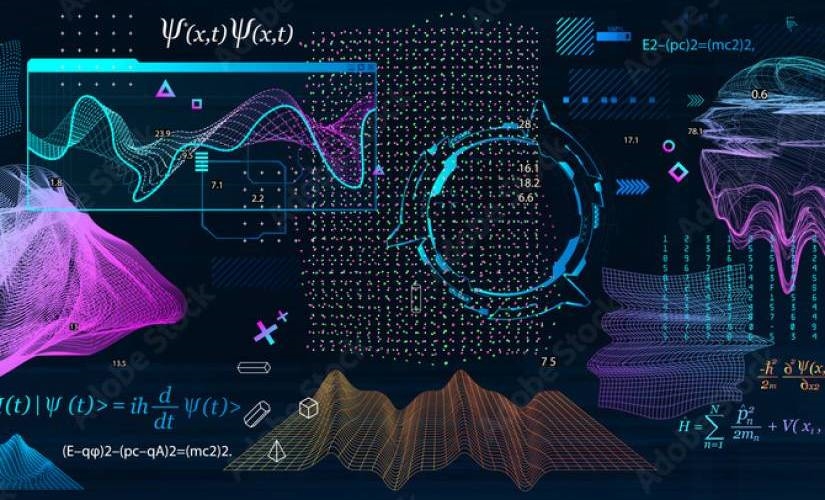
Graphs illustrating quantum mechanics concepts and AI. – Via devicedaily.com
Semantic AI stands at a transformative threshold, with quantum computing emerging as a game-changing catalyst for its evolution. Recent research demonstrates how quantum-enhanced semantic networks could revolutionize our ability to process and interpret complex data relationships at unprecedented scales.
The integration of quantum computing promises to overcome current computational limitations in semantic analysis. Quantum systems can analyze multiple semantic relationships simultaneously through quantum superposition. This breakthrough capability means semantic AI could soon tackle vastly more complex knowledge representations and contextual understanding tasks.
Interdisciplinary collaboration stands as another crucial driver of semantic AI’s future. The convergence of expertise from fields like linguistics, neuroscience, and quantum physics is creating novel approaches to knowledge representation. These cross-pollinated insights are helping semantic AI systems better mirror human-like understanding and reasoning patterns.
One of the most promising developments lies in quantum-enhanced natural language processing. Quantum algorithms show potential for parsing semantic nuances and contextual relationships that traditional computing struggles to capture. This advancement could lead to AI systems that truly grasp the subtle meanings and implications in human communication.
The quantum computing impact on AI will enable processing capabilities a thousand times faster and more potent than conventional computers, particularly in analyzing complex semantic relationships.
Parangat Technologies Report
As these technologies mature, we can expect semantic AI to evolve beyond simple pattern recognition toward genuine contextual understanding. The combination of quantum computing’s raw processing power with sophisticated semantic networks points toward AI systems that can navigate the complexities of human knowledge with unprecedented depth and nuance.
Leveraging SmythOS for Semantic AI Solutions
Enterprise-scale semantic AI development traditionally demands extensive expertise in both knowledge representation and software engineering, creating significant barriers to entry. SmythOS transforms this landscape through an innovative drag-and-drop interface that changes how organizations approach AI development.
At the heart of SmythOS lies a comprehensive visual development environment that sets it apart from conventional platforms. Rather than requiring deep coding knowledge, teams can construct sophisticated AI agents through an intuitive visual builder. This approach reduces the learning curve while accelerating development cycles, enabling organizations to solve business problems without getting lost in technical complexities.
The platform’s robust integration capabilities serve as a particular strength for enterprise deployments. SmythOS seamlessly connects with various AI models, APIs, and tools, allowing organizations to leverage their existing technology investments while expanding capabilities through advanced semantic features. This flexibility ensures that teams can build and deploy AI solutions that align perfectly with their specific needs.
Perhaps most impressively, SmythOS’s built-in debugging environment provides unprecedented visibility into semantic operations. Developers can examine workflows in real-time, quickly identifying and resolving issues that might otherwise slow development. This transparency reduces development cycles and ensures higher-quality implementations that stand up to enterprise demands.
Security considerations are woven throughout the platform’s architecture, acknowledging the sensitive nature of organizational knowledge bases. SmythOS implements comprehensive measures to protect data integrity while enabling authorized access and collaboration. These enterprise-grade security features ensure that sensitive information remains protected without compromising the platform’s rich functionality.
SmythOS’s process agents handle much of the heavy lifting in semantic AI development, automatically managing data ingestion from various sources and organizing information into meaningful connections. This automation reduces manual effort and potential human error in knowledge graph construction and maintenance, allowing teams to focus on strategic objectives rather than technical implementation details.
Conclusion and Future Directions
As organizations explore the potential of Semantic AI, its transformative capabilities are becoming clear. According to IBM’s latest analysis, these AI applications could contribute between USD 2.6 trillion and 4.4 trillion annually to the global economy through enhanced productivity and innovation.
The journey ahead requires careful consideration of both challenges and opportunities. Data quality remains a critical hurdle, with recent studies showing that 70% of organizations with low trust in their data cite quality issues as their biggest obstacle to confident decision-making. Addressing these fundamental challenges will be crucial for unlocking the full potential of Semantic AI implementations.
Looking toward the future, we are witnessing the emergence of more sophisticated and specialized AI models. Purpose-built solutions are becoming commonplace, offering greater precision and efficiency for specific organizational needs. This shift from general-purpose to tailored implementations marks a significant evolution in how enterprises leverage AI technology.
The integration of multimodal capabilities presents another frontier for Semantic AI advancement. These systems will increasingly process and analyze various forms of data—from text and images to complex organizational knowledge graphs—creating a more comprehensive and nuanced understanding of business contexts.
To maximize the benefits of these technological advances, organizations must prioritize responsible AI development and deployment. This includes establishing robust governance frameworks, ensuring data quality, and maintaining transparency in AI operations. Success in the coming years will depend not just on adopting these technologies, but on implementing them thoughtfully and strategically to drive genuine organizational value.
Last updated:
Disclaimer: The information presented in this article is for general informational purposes only and is provided as is. While we strive to keep the content up-to-date and accurate, we make no representations or warranties of any kind, express or implied, about the completeness, accuracy, reliability, suitability, or availability of the information contained in this article.
Any reliance you place on such information is strictly at your own risk. We reserve the right to make additions, deletions, or modifications to the contents of this article at any time without prior notice.
In no event will we be liable for any loss or damage including without limitation, indirect or consequential loss or damage, or any loss or damage whatsoever arising from loss of data, profits, or any other loss not specified herein arising out of, or in connection with, the use of this article.
Despite our best efforts, this article may contain oversights, errors, or omissions. If you notice any inaccuracies or have concerns about the content, please report them through our content feedback form. Your input helps us maintain the quality and reliability of our information.