Semantic AI and AI Governance: Shaping the Future of Ethical and Intelligent Systems
Semantic AI is transforming how artificial intelligence understands and interacts with data. By enabling AI systems to interpret information in a more human-like manner, semantic AI adds nuance and context to data analysis. However, as these AI capabilities advance, robust governance frameworks become increasingly critical.
AI governance refers to the structures and processes that ensure AI technologies are developed and used ethically and responsibly. This involves addressing challenges like algorithmic bias, data privacy, and transparency in AI decision-making. Organizations across various sectors are grappling with how to effectively implement and manage AI while upholding ethical standards.
This article will explore the intersection of semantic AI and AI governance, examining:
- The key principles of responsible AI development
- Best practices for implementing AI governance frameworks
- Real-world examples of effective AI governance in action
- Emerging challenges and future considerations for ethical AI
By understanding these critical issues, organizations can harness the power of semantic AI while mitigating risks and building trust with stakeholders. Let’s explore AI ethics and governance to uncover insights that can guide the responsible development of this transformative technology.
The convergence of semantic AI capabilities and robust governance practices will be essential for realizing AI’s full potential to benefit society.
Challenges in Implementing AI Governance
As artificial intelligence (AI) advances and becomes more integrated into society, implementing effective governance frameworks faces several complex challenges. The rapid pace of AI innovation, combined with the lack of standardized guidelines across regions, creates significant hurdles for policymakers and regulators aiming to harness AI’s benefits while mitigating potential risks.
One of the primary challenges is keeping up with the speed of technological progress. By the time regulators draft policies for a particular AI application, the technology may have already evolved, potentially rendering those policies obsolete. For example, when OpenAI released GPT-3 in 2020, it was considered a major breakthrough. Yet just three years later, GPT-4 has vastly surpassed its capabilities, highlighting how rapidly the AI landscape can shift.
The lack of standardized, global guidelines for AI governance further complicates implementation efforts. Different countries and regions are taking varied approaches, leading to a fragmented regulatory environment. While the European Union pushes for strict AI regulations through its proposed AI Act, countries like the United States have opted for a more hands-off approach focused on voluntary guidelines. This lack of international alignment makes it difficult for companies developing AI systems to navigate compliance across borders.
Another significant challenge is the inherent complexity and opacity of many AI systems. The ‘black box’ nature of advanced machine learning models can make it extremely difficult for regulators to audit these systems and ensure they are operating as intended. This lack of transparency and explainability poses major obstacles for governance frameworks aiming to ensure AI systems are safe, ethical, and unbiased.
The multifaceted nature of AI applications across various sectors also necessitates adaptive and flexible governance mechanisms. A one-size-fits-all approach is unlikely to be effective. For instance, the governance needs for AI in healthcare will differ greatly from those in finance or autonomous vehicles. Regulators must develop frameworks that can be tailored to specific use cases while still maintaining consistent overarching principles.
Additionally, there is often a knowledge and expertise gap between those developing AI systems and those tasked with regulating them. Government agencies may struggle to attract and retain the technical talent needed to effectively oversee rapidly evolving AI technologies. This imbalance can lead to regulatory blind spots or overly broad policies that stifle innovation.
To address these challenges, governance frameworks must be robust yet flexible enough to evolve alongside the technology. International cooperation and knowledge sharing will be crucial to develop more standardized guidelines. Engaging diverse stakeholders—including AI developers, ethicists, legal experts, and civil society—in the governance process can help bridge knowledge gaps and create more comprehensive frameworks.
Ultimately, implementing effective AI governance is a complex, ongoing process rather than a one-time solution. As the technology continues to advance, our governance approaches must become equally dynamic and adaptive to ensure AI benefits society while minimizing potential harms.
Best Practices for AI Governance
As artificial intelligence (AI) reshapes industries and decision-making processes, organizations must implement robust governance practices to ensure responsible and ethical use of these powerful technologies. This section explores key best practices for AI governance, drawing insights from leading organizations that have successfully navigated the complexities of AI implementation.
Regular Auditing of AI Systems
One of the cornerstones of effective AI governance is establishing a rigorous auditing process. Regular audits help organizations identify potential biases, errors, or unintended consequences in AI systems before they cause harm. For instance, Tata Consultancy Services recommends that companies allocate approximately 5-10% of their AI budget specifically for governance, including auditing processes.
To implement an effective AI auditing practice:
- Develop clear audit criteria and schedules for each AI system
- Assemble cross-functional teams to conduct audits, including data scientists, ethicists, and domain experts
- Document findings thoroughly and create action plans to address any issues discovered
- Continuously refine audit processes based on lessons learned and emerging best practices
Transparent Use of Data
Transparency in data usage is crucial for building trust with stakeholders and ensuring compliance with evolving regulations. Organizations must be clear about how they collect, process, and utilize data in their AI systems. This transparency extends to both internal stakeholders and external users affected by AI-driven decisions.
Best practices for data transparency include:
- Clearly communicating data collection and usage policies to all stakeholders
- Implementing robust data governance frameworks to ensure data quality and integrity
- Providing mechanisms for individuals to access, correct, or delete their personal data used in AI systems
- Regularly publishing transparency reports detailing data usage and AI system performance
Continuous Stakeholder Engagement
Effective AI governance requires ongoing dialogue with a diverse range of stakeholders, including employees, customers, partners, and regulatory bodies. This engagement helps organizations stay attuned to concerns, gather valuable feedback, and adapt their AI practices accordingly.
To foster meaningful stakeholder engagement:
- Establish regular forums for discussion and feedback on AI initiatives
- Involve stakeholders in the design and testing phases of AI systems
- Provide clear channels for reporting concerns or issues related to AI systems
- Regularly update stakeholders on changes and improvements to AI governance practices
Establishing a Cross-Functional AI Governance Committee
A critical step in implementing these best practices is forming a dedicated AI governance committee. This cross-functional team should include representatives from various departments, including IT, legal, ethics, and business units. The committee’s role is to oversee AI initiatives, ensure alignment with organizational values, and make critical decisions regarding AI deployment and use.
Role | Responsibilities |
---|---|
Risk Managers | Ensure quality and safety, align innovation with business objectives, control risks, provide assurances to the C-suite, board, and regulators. |
Model Builders | Develop AI models, seek budgetary support, collaborate with internal partners for deployment, add value to the business as problem-solvers. |
Business Leaders | Drive innovation, bring new products to market, reduce costs, increase profit, ensure cross-functional alignment for effective AI investments. |
Legal Representatives | Ensure compliance with regulations, address legal risks, provide guidance on ethical use of AI. |
IT Representatives | Oversee technical implementation, ensure data security and integrity, support AI system maintenance and updates. |
Human Resources | Manage the impact of AI on employees, oversee training and development for AI literacy, address workforce-related ethical concerns. |
The International Association of Privacy Professionals (IAPP) emphasizes the importance of such committees in their AI Governance in Practice Report, noting that they play a crucial role in defining policies and guidelines for AI development and deployment.
Continuous Learning and Adaptation
The field of AI is rapidly evolving, and governance practices must keep pace. Organizations should foster a culture of continuous learning and adaptation in their AI governance approach. This includes:
- Staying informed about the latest developments in AI technology and governance best practices
- Regularly updating governance policies and procedures based on new insights and experiences
- Investing in ongoing training and education for employees involved in AI development and deployment
- Participating in industry forums and collaborations to share knowledge and learn from peers
By adopting these best practices, organizations can create a robust framework for AI governance that balances innovation with responsibility. Regular auditing, transparent data use, continuous stakeholder engagement, cross-functional oversight, and a commitment to ongoing learning form the foundation of ethical and effective AI implementation. As AI continues to transform business landscapes, these governance practices will be essential in building trust, mitigating risks, and realizing the full potential of AI technologies.
The Role of International Cooperation in AI Governance
As artificial intelligence reshapes our world, the need for global collaboration in AI governance has never been more pressing. The rapid advancement of AI technologies across borders demands a unified approach to regulation and ethical standards. Without international cooperation, we risk a fragmented landscape where AI’s benefits—and risks—are unevenly distributed.
The challenges of AI governance are inherently global. From data privacy concerns to the potential for AI-driven warfare, these issues transcend national boundaries. As Seán S. ÓhÉigeartaigh, a researcher at the University of Cambridge, points out, “Cross-cultural cooperation will be essential for the success of these ethics and governance initiatives.” This sentiment underscores the urgency of creating cohesive frameworks that can guide AI development worldwide.
International bodies are recognizing the critical nature of this task. The Organization for Economic Co-operation and Development (OECD) has established an expert group on AI, bringing together diverse voices from across the globe. Similarly, UNESCO has taken strides in this direction, adopting a Recommendation on the Ethics of Artificial Intelligence in 2021. These efforts signal a growing awareness of the need for global standards in AI ethics and governance.
Bridging Cultural Divides in AI Ethics
One of the most significant challenges in international AI cooperation is navigating cultural differences in ethical perspectives. What might be considered an acceptable use of AI in one country could be viewed as a violation of privacy or human rights in another. This diversity of viewpoints is not a roadblock but an opportunity to create more robust and universally applicable frameworks.
Efforts are underway to find common ground. The Beijing AI Principles, for instance, echo many of the ethical concerns raised in Western AI ethics guidelines. This convergence suggests that despite cultural differences, there is a shared recognition of AI’s potential impacts and the need for responsible development.
However, we must be cautious not to oversimplify these agreements. As Jess Whittlestone and colleagues note, “nations with different cultures may interpret and prioritize the same principles differently in practice.” This nuance highlights the need for ongoing dialogue and collaboration to ensure that global AI governance frameworks are truly inclusive and effective.
The Path Forward: From Principles to Practice
While establishing ethical principles is a crucial first step, the real challenge lies in translating these ideals into practical governance measures. International cooperation must extend beyond academia and policy circles to include industry leaders, civil society organizations, and governments.
Initiatives like the Partnership on AI are paving the way, bringing together diverse stakeholders to address AI challenges collectively. These collaborative efforts are essential for developing governance frameworks that are both comprehensive and adaptable to the rapid pace of AI innovation.
Moreover, we must recognize that effective AI governance requires more than just regulation. It demands a commitment to shared values, transparent communication, and a willingness to learn from one another. As AI continues to evolve, so too must our approaches to governing it.
The best way to arrive at more robustly justified norms, standards, and regulation for AI will be to find those that can be supported by a plurality of different value systems.
The journey towards cohesive global AI governance is complex and ongoing. It requires us to bridge cultural divides, find common ethical ground, and work tirelessly to implement practical solutions. As we navigate this challenging terrain, one thing is clear: the future of AI governance depends on our ability to cooperate across borders, cultures, and disciplines. Only through sustained international collaboration can we hope to harness the full potential of AI while safeguarding against its risks.
The Future of AI Governance
As artificial intelligence advances rapidly, AI governance is set for significant evolution. Two key trends are emerging: the integration of ethical frameworks and an increased role for industry self-regulation.
Embedding Ethics into AI Frameworks
Ethical considerations are becoming a core part of AI development processes. As Alalawi et al. note in a recent study, ‘Trust AI regulation? Discerning users are vital to build trust and effective AI regulation.’ This shift reflects a growing awareness that public trust is essential for AI’s continued adoption and development.
What might ethical AI frameworks look like in practice? We are already seeing early examples emerge:
- Explainable AI systems that can articulate the reasoning behind their decisions
- Bias detection and mitigation tools built into machine learning pipelines
- Privacy-preserving techniques like federated learning becoming standard practice
Framework | Principles | Organization | Source |
---|---|---|---|
Ethics Guidelines for Trustworthy AI | Human agency and oversight, Technical robustness and safety, Privacy and data governance, Transparency, Diversity, non-discrimination, and fairness, Societal and environmental wellbeing, Accountability | European Commission | Springer |
OECD Ethical AI Principles | Interpretability, Reliability, Accountability, Data privacy, Human agency | Organization for Economic Co-operation and Development (OECD) | World Economic Forum |
IBM Principles of Trust and Transparency | Fairness, Explainability, Robustness, Transparency, Accountability | IBM | IBM |
The challenge lies in operationalizing ethical principles in a way that doesn’t stifle innovation. As one AI ethics researcher put it, ‘It’s a delicate balance between guardrails and golden handcuffs.’
The Rise of Industry Self-Regulation
The increasing role of industry in governing itself when it comes to AI is driven partly by the rapid speed of AI progress outpacing traditional regulatory approaches. Companies are realizing that proactive self-regulation may be preferable to reactive government intervention.
This is evident in several ways:
- Industry consortiums developing best practices and standards
- Internal ethics boards at major tech companies
- Voluntary commitments to responsible AI development
For instance, the Avanade Institute reports a growing trend of industry-led AI governance initiatives, noting that ‘Industry self-regulation of AI is rising due to diverse use-cases and fast innovation.’
However, skeptics rightly point out potential conflicts of interest in letting the fox guard the henhouse. Effective industry self-regulation will require robust accountability mechanisms and transparency to maintain public trust.
Implications for the Future
As these trends converge, we are likely to see a hybrid model of AI governance emerge. Government regulations may set broad guardrails, while industry self-regulation fills in the details with more agile, context-specific guidelines.
This evolving landscape presents both opportunities and challenges:
- More nimble, adaptive governance frameworks
- Potential for global harmonization of AI standards
- Risk of regulatory capture by powerful tech interests
- Balancing innovation with adequate safeguards
Ultimately, the success of future AI governance will hinge on genuine collaboration between technologists, ethicists, policymakers, and the public. As AI becomes ever more woven into the fabric of our lives, ensuring its responsible development is not just a technical challenge but a profoundly human one.
Leveraging SmythOS
As artificial intelligence integrates more deeply into enterprise operations, effective governance has become a critical challenge. SmythOS offers a powerful solution, providing a comprehensive platform that enhances transparency, accountability, and control in AI systems. Organizations can leverage SmythOS to elevate their AI governance practices.
At the core of SmythOS’s governance capabilities is its innovative visual debugging environment. This feature transforms the typically opaque process of AI decision-making into a clear, traceable workflow. Data scientists and compliance officers can visualize how AI models process information, make decisions, and generate outputs in real-time. This unprecedented level of transparency enables teams to quickly identify and address potential biases, errors, or unintended behaviors before they impact business operations or customer experiences.
SmythOS’s support for major graph databases further amplifies its governance prowess. By seamlessly integrating with popular graph database technologies, the platform allows organizations to create rich, interconnected representations of their AI ecosystems. This approach enables a holistic view of data lineage, model dependencies, and decision pathways, crucial for maintaining regulatory compliance and ethical AI practices.
SmythOS isn’t just another AI tool. It’s transforming how we approach AI debugging. The future of AI development is here, and it’s visual, intuitive, and incredibly powerful.
G2 Reviews
Enterprise architects will appreciate SmythOS’s robust audit logging capabilities. The platform maintains detailed records of all AI operations, decisions, and modifications, creating an unbroken chain of accountability. This comprehensive audit trail not only satisfies regulatory requirements but also provides valuable insights for continuous improvement of AI governance practices.
One of the most significant advantages of SmythOS in AI governance is its ability to democratize oversight. The platform’s intuitive visual interface allows non-technical stakeholders, such as legal and ethics teams, to actively participate in the governance process. This inclusive approach ensures that AI systems align not just with technical specifications, but also with broader organizational values and ethical guidelines.
Security-conscious organizations will find SmythOS’s enterprise-grade security features particularly valuable. The platform implements stringent measures to protect sensitive data and AI models, ensuring that governance efforts don’t compromise data integrity or intellectual property. This robust security framework makes SmythOS an ideal choice for industries handling sensitive information, such as healthcare or finance.
By leveraging SmythOS, enterprises can create a culture of responsible AI development and deployment. The platform’s comprehensive approach to governance empowers organizations to build AI systems that are not only powerful and efficient but also transparent, accountable, and aligned with ethical standards. As AI continues to shape the future of business, SmythOS stands out as an essential tool for organizations committed to leading the way in responsible AI innovation.
Conclusion: Enhancing AI Governance with SmythOS
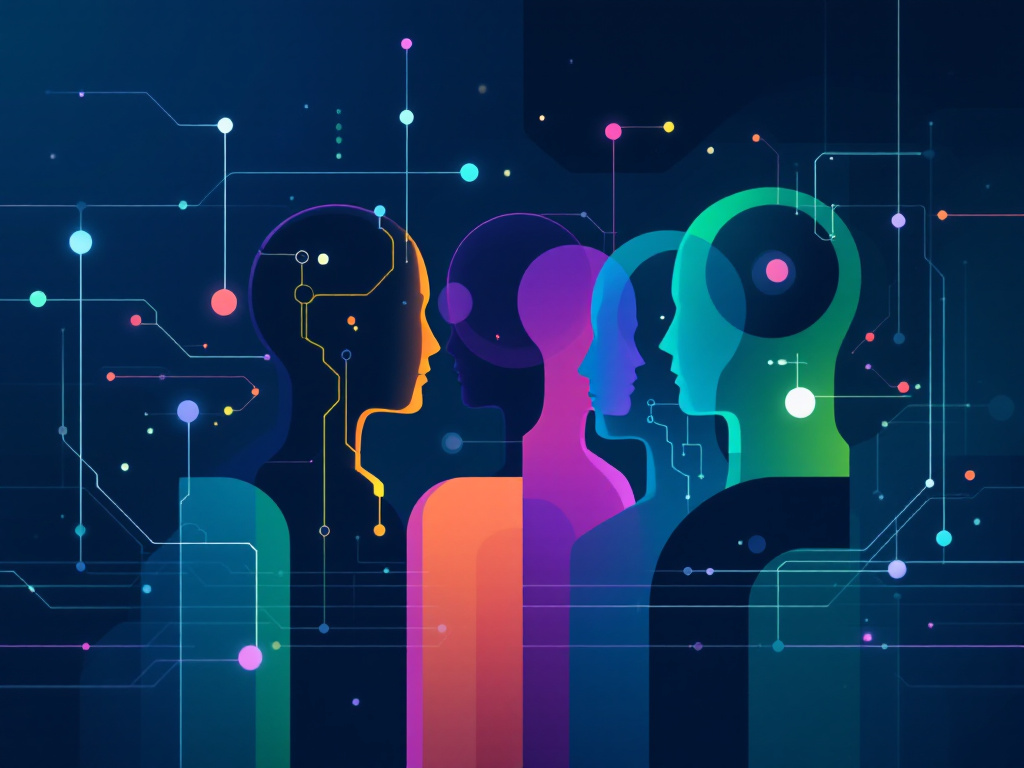
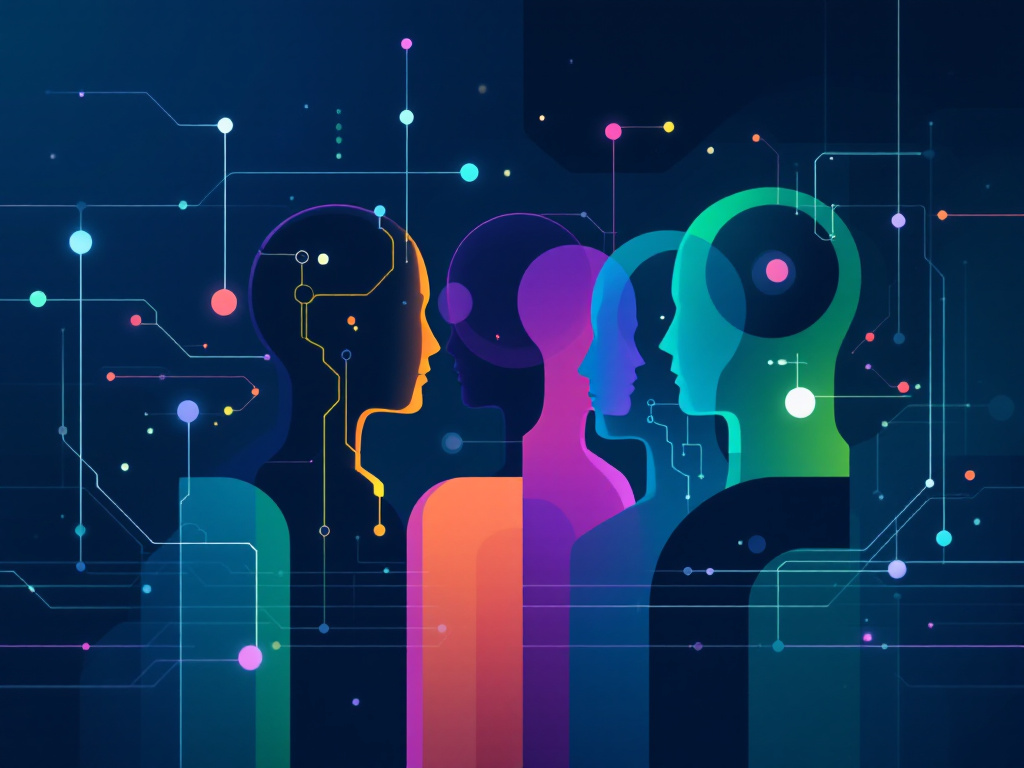
As artificial intelligence continues to reshape our world, the need for effective AI governance has never been more crucial. The ethical and responsible deployment of AI technologies demands a robust framework that can adapt to the rapidly evolving landscape of machine learning and data science. In this context, SmythOS emerges as a vital tool for organizations striving to implement best practices in AI governance.
SmythOS aligns with international standards and provides a comprehensive suite of tools designed to address the multifaceted challenges of AI oversight. By offering visual builders for creating agents that reason over knowledge graphs and supporting major graph databases, SmythOS enables enterprises to maintain granular control over their AI systems. This integration fosters transparency and accountability—cornerstones of responsible AI deployment.
The platform’s built-in debugging tools for knowledge graph interactions ensure the reliability and safety of AI applications. The ability to query and update knowledge graphs through visual workflows enhances efficiency and promotes a deeper understanding of AI decision-making processes among stakeholders. This transparency is critical in building trust and mitigating the risks associated with ‘black box’ AI systems.
Looking ahead, the future of AI governance promises even more sophisticated frameworks and tools. As regulatory bodies worldwide refine their approaches—exemplified by initiatives like the EU AI Act and the NIST AI Risk Management Framework—platforms like SmythOS will play an increasingly pivotal role in helping organizations navigate this complex terrain. The integration of enterprise-grade security features for sensitive knowledge bases demonstrates SmythOS’s commitment to addressing growing concerns around data privacy and protection in AI applications.
SmythOS stands at the forefront of the evolution towards truly responsible AI. By providing a platform that emphasizes ethical considerations, transparency, and robust governance tools, SmythOS is not just facilitating compliance—it is actively shaping the future of AI governance. The continued refinement and adoption of such comprehensive governance solutions will be essential in realizing the full potential of AI while safeguarding the values and rights that underpin our society.
Last updated:
Disclaimer: The information presented in this article is for general informational purposes only and is provided as is. While we strive to keep the content up-to-date and accurate, we make no representations or warranties of any kind, express or implied, about the completeness, accuracy, reliability, suitability, or availability of the information contained in this article.
Any reliance you place on such information is strictly at your own risk. We reserve the right to make additions, deletions, or modifications to the contents of this article at any time without prior notice.
In no event will we be liable for any loss or damage including without limitation, indirect or consequential loss or damage, or any loss or damage whatsoever arising from loss of data, profits, or any other loss not specified herein arising out of, or in connection with, the use of this article.
Despite our best efforts, this article may contain oversights, errors, or omissions. If you notice any inaccuracies or have concerns about the content, please report them through our content feedback form. Your input helps us maintain the quality and reliability of our information.