Understanding Semantic AI and AI Assistants
Imagine a world where computers truly grasp the meaning of your words. That’s the transformative reality of Semantic AI, a breakthrough technology that revolutionizes how machines understand and interact with human language. By combining natural language processing, knowledge graphs, and machine learning, Semantic AI brings us closer to genuinely intelligent digital assistants that think and reason more like humans.
Unlike traditional AI systems that rely on rigid rules and pattern matching, Semantic AI can interpret nuances, understand context, and make intelligent connections much like our own cognitive processes. This sophisticated technology enables AI assistants to move beyond simple keyword responses to deliver truly contextual and meaningful interactions.
Today’s AI assistants leverage this semantic understanding to transform how we interact with technology across industries. From enhancing customer service with contextually aware chatbots to powering sophisticated recommendation systems that truly understand user preferences, these AI assistants are becoming increasingly adept at handling complex tasks that once required human intelligence.
This comprehensive guide explores how organizations can effectively integrate these powerful technologies, examining crucial aspects like bias detection and mitigation, and practical implementation strategies. We also investigate how modern platforms make these advanced capabilities more accessible, allowing businesses to harness the full potential of semantically-aware AI assistants.
The implications of this technology are profound. We’re not just building smarter machines; we’re creating more intuitive and natural ways for humans and computers to collaborate. As we delve deeper into this topic, you’ll discover how Semantic AI and AI assistants are reshaping our approach to problem-solving, decision-making, and human-computer interaction in ways that were once confined to science fiction.
Key Components of Semantic AI
Semantic AI represents a sophisticated fusion of technologies that enables machines to understand human language and derive meaning from data. Like a skilled linguist who grasps not just words but their deeper implications, Semantic AI combines several essential components to achieve contextual understanding.
Natural Language Processing (NLP) forms the foundation of Semantic AI by enabling computers to interpret and analyze human language with remarkable accuracy. According to expert.ai, semantic analysis in NLP determines word meanings based on context and relationships, helping machines understand language the way humans naturally communicate. This goes beyond simple keyword matching to grasp nuances, detect emotional undertones, and comprehend complex linguistic patterns.
Knowledge representation serves as the cognitive framework, organizing information in ways that mirror human understanding. Using sophisticated knowledge graphs, this component creates a web of interconnected concepts and relationships. Think of it as building a detailed map of ideas where each concept is linked to related information, much like how our brains connect different pieces of knowledge to form deeper understanding.
Machine learning algorithms power the system’s ability to learn and improve from experience. Rather than following rigid rules, these algorithms analyze patterns in data to make intelligent decisions about meaning and context. This capability allows Semantic AI to handle complex queries with increasing accuracy over time, adapting to new information and scenarios much like a student becoming more proficient through practice.
The integration of these components creates powerful synergies. For example, when you ask a virtual assistant about local restaurants, NLP processes your query, knowledge graphs provide context about different cuisines and locations, and machine learning algorithms personalize recommendations based on your preferences and past behavior. This orchestrated approach enables more natural and effective human-machine interactions.
Semantic AI is not just another machine learning algorithm. It’s a comprehensive AI strategy that combines carefully selected methods and tools to solve complex problems with high precision.
SEMANTiCS Conference 2021
By enhancing data intelligence and manipulation, these core components empower Semantic AI to provide more intelligent and context-aware solutions, ultimately transforming how we interact with technology across diverse applications and domains. The result is a system that doesn’t just process information, but truly understands it in ways that mirror human cognition.
Building Effective AI Assistants
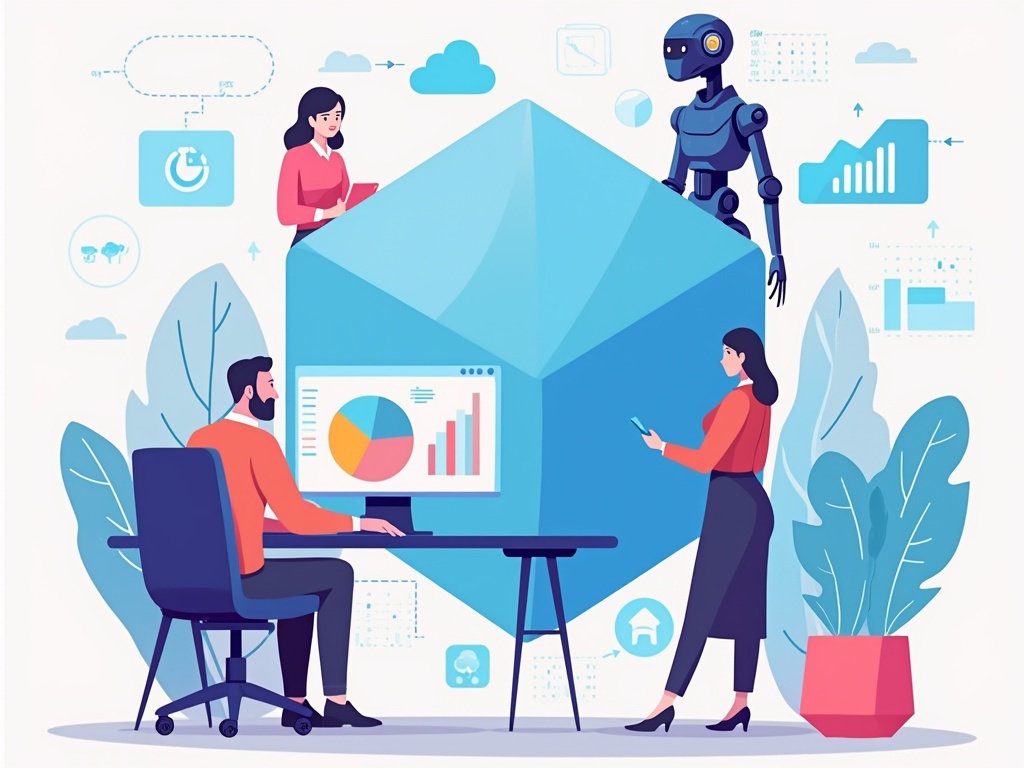
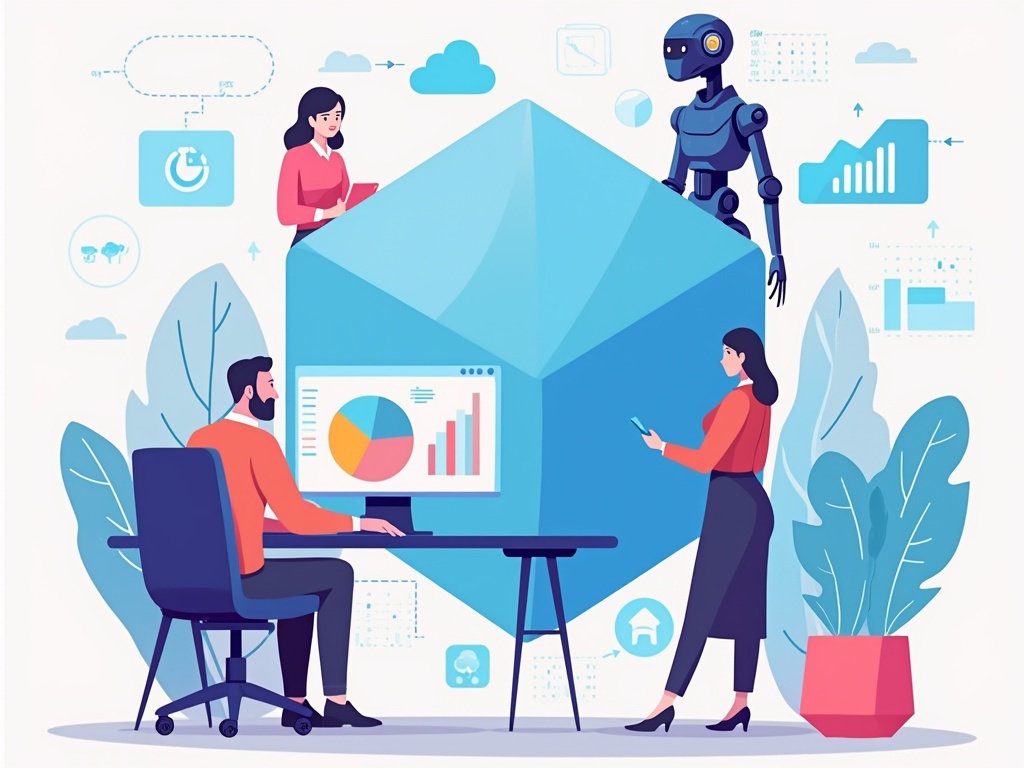
Modern AI assistants need to balance technological capability with human-centric design. Success hinges on creating systems that process information efficiently and connect with users intuitively.
Transparency is a cornerstone principle when developing AI assistants. According to WillowTree’s research, assistants must clearly identify themselves as AI-powered solutions from the start. This upfront honesty helps build trust and prevents confusion about capabilities.
The user interface is the critical bridge between AI capability and user needs. A minimalist, uncluttered design keeps interactions focused and purposeful. Clear call-to-action buttons and intuitive navigation elements guide users naturally through conversations. The interface should adapt seamlessly across devices while maintaining a consistent experience.
Data integration capabilities form the backbone of effective AI assistants. By connecting to relevant knowledge bases and data sources, assistants can provide accurate, contextual responses in real-time. This requires robust error handling and data preprocessing to ensure information quality. Regular updates to the knowledge base help assistants stay current and relevant.
Understanding and Acting on User Intent
At the heart of any successful AI assistant is its ability to accurately grasp user intent. This goes beyond simple keyword matching to understand the context, nuance, and underlying goals in user queries. Natural Language Processing (NLP) tools like spaCy and NLTK enable assistants to parse complex language patterns and respond appropriately.
Effective intent recognition requires training on diverse datasets that represent real-world user interactions. This helps assistants handle variations in language, including colloquialisms and industry-specific terminology. The goal is to make interactions feel natural while maintaining high accuracy in task completion.
Level-setting for a smart assistant experience goes beyond traditional chatbot functionality. Today’s users expect AI to understand context and navigate complex conversations with human-like comprehension.
WillowTree Insights
Response generation must prioritize both speed and accuracy. AI assistants should provide immediate acknowledgment of user inputs while taking the necessary time to process complex requests thoroughly. This balanced approach helps maintain user engagement while ensuring quality responses.
Continuous learning and refinement play crucial roles in maintaining assistant effectiveness. User feedback helps identify areas for improvement, while performance metrics guide optimization efforts. Regular model updates incorporate new learning and adapt to changing user needs.
Handling Biases in Training Data
The quest for fair and unbiased AI systems begins with training data. When AI models learn from biased datasets, they perpetuate and sometimes amplify existing societal prejudices. Consider Amazon’s experimental recruiting tool that showed bias against women—a stark reminder of how training data bias can lead to discriminatory outcomes.
Data bias typically emerges in three key ways: through sampling bias, where certain groups are underrepresented; through measurement bias, where data collection methods favor particular outcomes; and through prejudice bias, where historical discriminations are encoded in the data. For instance, facial recognition systems have repeatedly shown lower accuracy rates for darker-skinned individuals due to training datasets that overwhelmingly featured lighter-skinned faces.
To identify potential biases, organizations must implement rigorous auditing processes. This includes analyzing data distributions across protected attributes like gender, race, and age, as well as examining proxy variables that might indirectly encode these characteristics. Regular data audits should also assess the completeness, accuracy, and representativeness of training datasets.
The first step in addressing bias is acknowledging that no dataset is neutral—every dataset carries the imprints of the social contexts in which it was created.
Dr. Timnit Gebru, AI Ethics Researcher
Mitigating bias requires a multi-faceted approach. Organizations should diversify their data sources to ensure broader representation. This might involve actively collecting data from underrepresented groups or using synthetic data generation techniques to balance datasets. Implementing pre-processing techniques can help identify and remove biased patterns before training begins.
Maintaining data integrity throughout this process is crucial. Organizations must establish clear documentation practices that track data provenance, preprocessing steps, and any modifications made to address bias. This documentation should also include regular assessments of the impact these changes have on model performance and fairness metrics.
The ethical implications of biased training data extend far beyond technical considerations. When AI systems make decisions based on biased data, they can perpetuate historical inequities and create new forms of discrimination. This raises important questions about accountability and the responsibility of organizations to ensure their AI systems promote fairness rather than reinforce existing social biases.
To maintain strong data integrity while addressing bias, organizations should adopt several best practices. These include establishing diverse data collection teams, implementing robust validation procedures, and creating clear guidelines for identifying and addressing potential biases. Regular external audits can provide additional oversight and help ensure accountability in bias mitigation efforts.
Integrating AI Assistants with Existing Systems
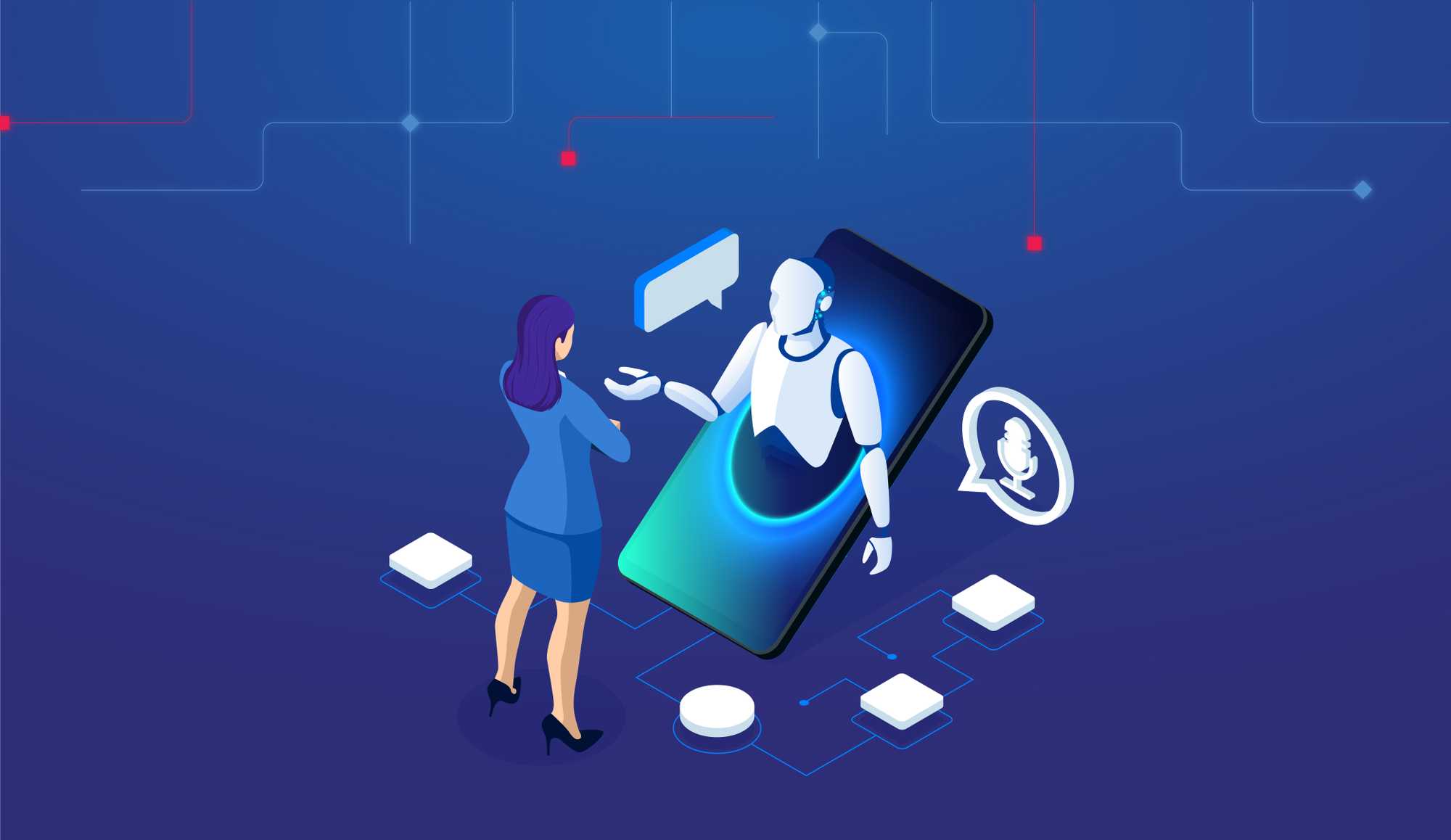
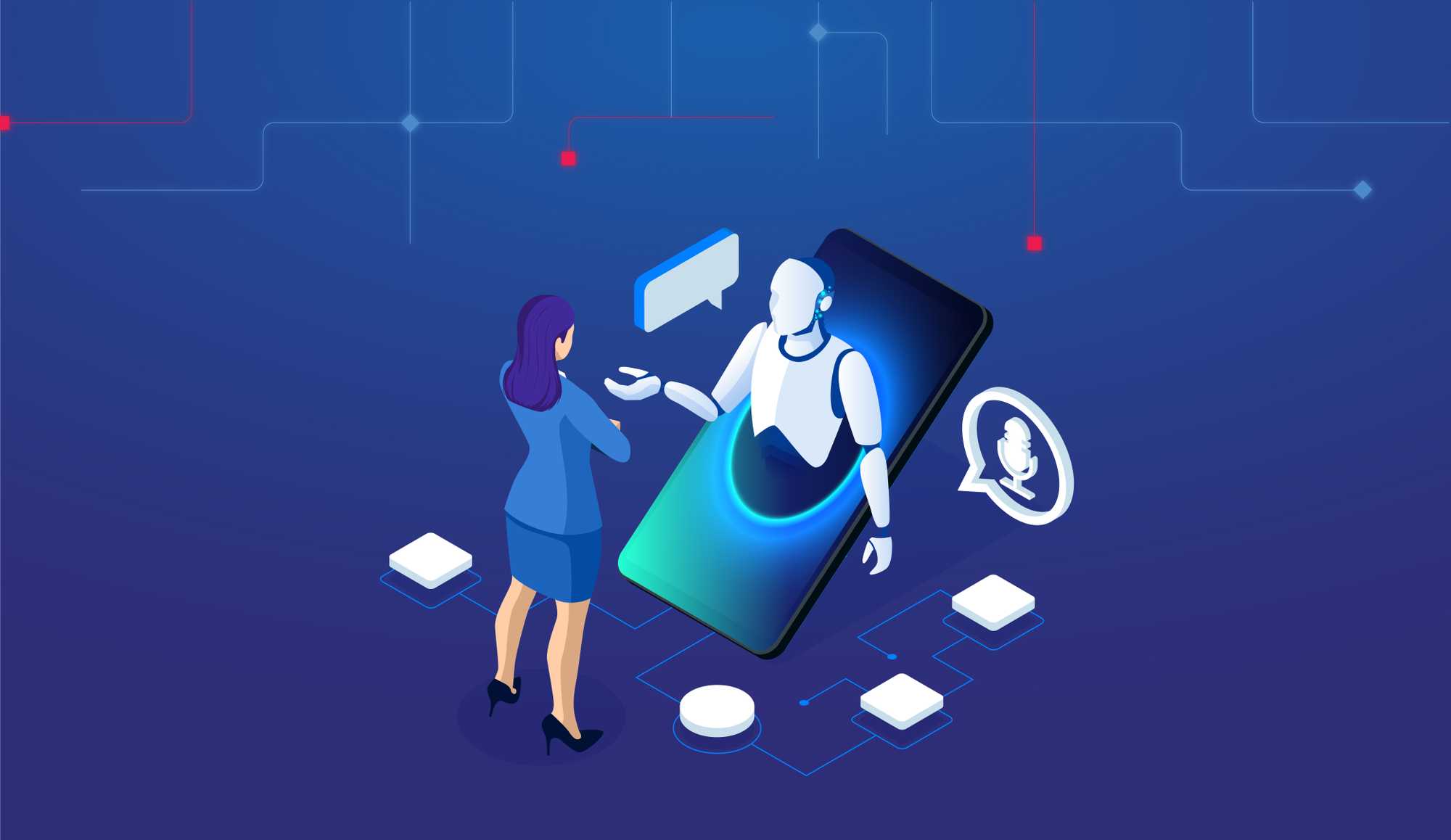
Engaging with a virtual assistant via smartphone. – Via nitcoinc.com
The implementation of AI assistants within established IT infrastructures presents both opportunities and challenges for modern enterprises. While these intelligent systems promise to enhance workplace productivity, their successful integration requires careful consideration of several technical and operational factors.
System compatibility is a primary concern when merging AI assistants with legacy infrastructure. As highlighted by industry experts at Spoke.ai, organizations must conduct thorough compatibility assessments to identify potential technical hurdles, such as data format mismatches and communication protocol differences. This evaluation phase is crucial for preventing integration issues.
Scalability is another critical consideration in AI assistant deployment. Organizations must ensure their infrastructure can handle increasing computational demands as AI usage grows. This includes implementing robust data pipelines capable of processing larger volumes of information and maintaining consistent performance levels even as user demands increase.
Security considerations are paramount when integrating AI assistants, particularly regarding data protection and privacy. Organizations must implement comprehensive security protocols, including encrypted data transmission, secure authentication mechanisms, and regular security audits. These measures help safeguard sensitive information while maintaining compliance with regulatory requirements.
From a practical standpoint, successful integration demands a strategic approach to change management. Companies should consider starting with pilot programs in specific departments before expanding deployment. This measured approach allows organizations to gather valuable feedback, refine processes, and address any operational challenges that emerge during the initial implementation phase.
Real-time monitoring and performance optimization are vital in maintaining system health post-integration. Organizations should establish clear metrics for measuring AI assistant performance and implement continuous monitoring systems to identify and address potential issues proactively. This ongoing oversight ensures the integrated system remains efficient and effective over time.
Data is a valuable and sensitive asset that must be kept secure. Data security helps you to maintain user trust, support your business objectives, and meet your compliance requirements.
Google Cloud Security Framework
Integration success also hinges on proper data management strategies. Organizations must ensure their data infrastructure can support AI assistants’ requirements, including data quality, accessibility, and governance. This involves implementing robust data cleaning procedures, establishing clear data handling protocols, and maintaining proper documentation of all data-related processes.
To maximize integration effectiveness, organizations should develop comprehensive training programs for employees who will interact with AI assistants. This human element often determines the success or failure of AI integration efforts, making it essential to invest in proper training and support resources for end-users.
Leveraging SmythOS for AI Assistant Development
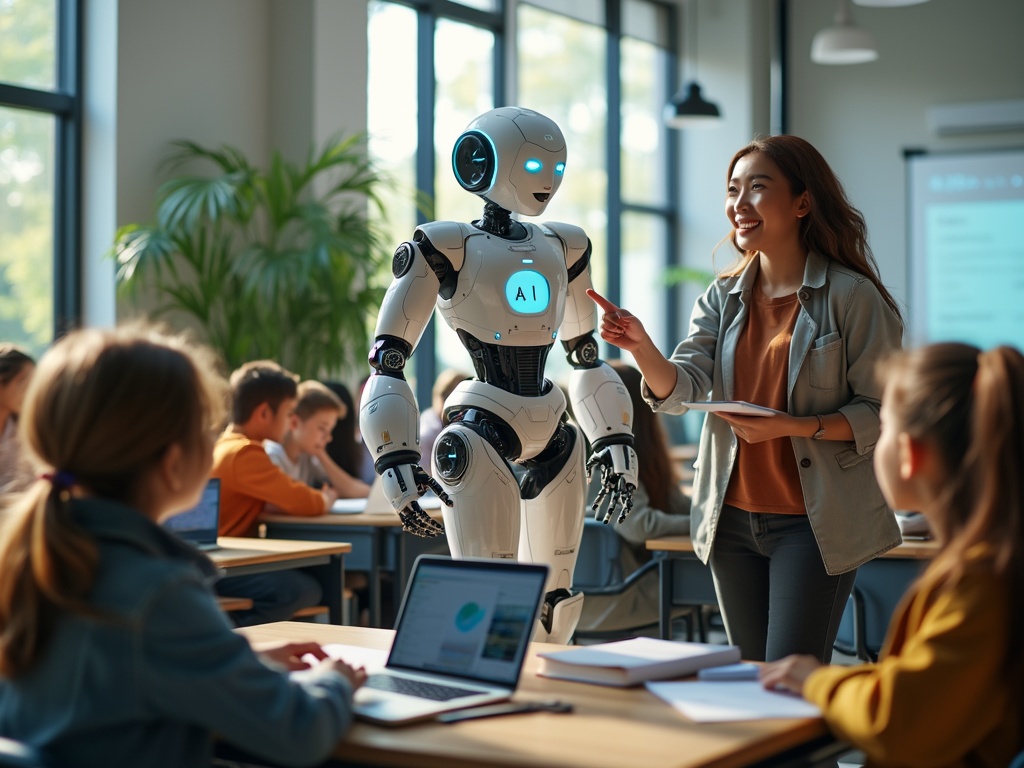
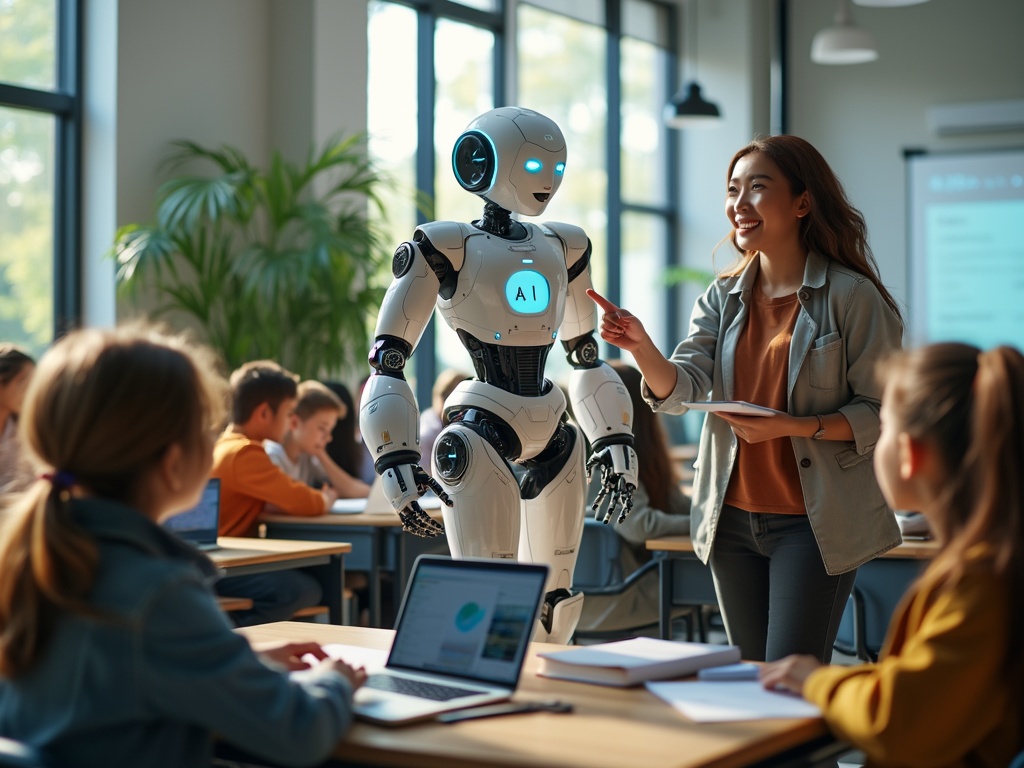
Students engage with AI in a modern classroom.
SmythOS transforms the complex process of building AI assistants into an intuitive visual experience. Through its comprehensive drag-and-drop interface, developers can design sophisticated AI agents without writing extensive code, democratizing access to advanced AI development capabilities.
At the core of SmythOS’s offering is its powerful visual debugging environment, which provides unprecedented visibility into AI decision paths and behavior. This real-time insight enables developers to quickly identify and resolve issues, significantly reducing the development cycle and ensuring more reliable AI assistants. The platform’s built-in monitoring capabilities offer detailed performance metrics, allowing teams to continuously optimize their AI solutions.
One of SmythOS’s standout features is its seamless integration with major graph databases. This capability allows organizations to leverage their existing data infrastructure while expanding its utility through advanced knowledge graph capabilities. The platform’s robust support for graph databases enables AI assistants to process complex relationships and patterns that would be difficult to manage in traditional database systems.
Security remains paramount in the SmythOS platform, particularly crucial for enterprise AI deployments. The system implements comprehensive security measures that protect data integrity while enabling authorized access and collaboration. These enterprise-grade security features ensure that sensitive information remains protected without compromising the platform’s functionality.
The platform’s process agents handle much of the heavy lifting in AI assistant development, automatically managing data flows and organizing information into meaningful connections. This automation significantly reduces the time and effort required to build and maintain complex AI systems, minimizing the potential for human error while maximizing efficiency.
Feature | SmythOS | Crew AI |
---|---|---|
Interface | Drag-and-drop, no-code | Configurable, developer-focused |
Integration Ecosystem | Extensive (APIs, AI models, tools like Slack, Trello, GitHub, Stripe) | Limited |
Multi-Agent Orchestration | Yes | Yes |
Deployment Options | APIs, chatbots, ChatGPT plugins, Alexa skills | Primarily within its own framework |
Memory and Context Management | Yes | Not explicitly mentioned |
Explainability and Transparency | Yes | Not explicitly mentioned |
Debugging Tools | Yes | Not explicitly mentioned |
Multimodal Capabilities | Yes | Not explicitly mentioned |
According to recent user reviews, SmythOS’s visual builder and debugging tools have proven particularly valuable for teams looking to accelerate their AI development process. The platform’s intuitive interface and comprehensive feature set have made it possible for organizations to deploy sophisticated AI assistants more efficiently than ever before.
By combining visual workflows, robust debugging capabilities, and enterprise-level security, SmythOS creates an environment where organizations can harness the full power of AI assistants without getting overwhelmed by technical complexities. This approach makes sophisticated AI technology accessible to teams of all skill levels, democratizing access to this powerful automation solution.
Future Directions in Semantic AI and AI Assistants
Semantic AI technology is at a transformative threshold, with industry analysis projecting contributions between $2.6 trillion and $4.4 trillion annually to the global economy. These systems are evolving beyond simple pattern matching to true contextual comprehension.
A promising development is the emergence of multimodal capabilities in semantic AI systems. These advancements enable the simultaneous processing of text, images, speech, and complex data relationships, opening new frontiers in industries like healthcare and finance where nuanced interpretation is crucial. The integration of knowledge graphs with advanced natural language processing represents another significant leap forward, allowing systems to draw increasingly sophisticated connections between disparate information sources.
Accessibility is becoming a key focus in semantic AI development. The trend toward smaller, more specialized models is democratizing access to these powerful tools, enabling organizations of all sizes to harness semantic AI’s potential without requiring massive computational resources. This optimization trend promises to make the technology more sustainable and practically applicable across diverse business contexts.
However, significant challenges remain. As noted in recent industry research, data privacy and security concerns continue to pose critical challenges, particularly as these systems process increasingly sensitive information. The need for transparent, interpretable AI systems that can explain their decision-making processes grows more pressing as semantic AI applications expand into regulated industries.
SmythOS emerges as a vital enabler in this evolving landscape, offering a comprehensive platform that bridges the gap between cutting-edge semantic AI capabilities and practical business applications. Its visual builder approach and enterprise-grade security features address critical market needs, while support for major graph databases positions it as a versatile solution for implementing advanced AI capabilities.
Looking ahead, the focus will likely shift toward enhanced interpretability, improved accuracy, and seamless integration with existing business processes. The convergence of semantic understanding with increasingly sophisticated knowledge representation systems positions this technology as a cornerstone of next-generation artificial intelligence solutions, promising to transform how we interact with and derive value from AI systems.
Last updated:
Disclaimer: The information presented in this article is for general informational purposes only and is provided as is. While we strive to keep the content up-to-date and accurate, we make no representations or warranties of any kind, express or implied, about the completeness, accuracy, reliability, suitability, or availability of the information contained in this article.
Any reliance you place on such information is strictly at your own risk. We reserve the right to make additions, deletions, or modifications to the contents of this article at any time without prior notice.
In no event will we be liable for any loss or damage including without limitation, indirect or consequential loss or damage, or any loss or damage whatsoever arising from loss of data, profits, or any other loss not specified herein arising out of, or in connection with, the use of this article.
Despite our best efforts, this article may contain oversights, errors, or omissions. If you notice any inaccuracies or have concerns about the content, please report them through our content feedback form. Your input helps us maintain the quality and reliability of our information.