Exploring the Applications of Multi-Agent Systems in Real-World Scenarios
Imagine a world where complex problems are solved by a swarm of interconnected agents working in harmony. Welcome to the fascinating realm of multi-agent systems (MAS), an innovative approach that tackles intricate challenges across diverse fields.
Multi-agent systems are the unsung heroes of our interconnected world. These distributed networks of AI-powered agents collaborate to solve problems too complex for any single entity to handle alone. From the sports arena to the energy grid, and from our roads to disaster response, MAS are reshaping the landscape of problem-solving.
What makes multi-agent systems so powerful is their ability to divide and conquer. By breaking down complex tasks into manageable pieces, MAS can tackle problems with unmatched efficiency and adaptability. These systems don’t just work; they learn, adapt, and evolve to meet the ever-changing demands of our dynamic world.
This article explores the diverse applications of multi-agent systems, examining how they transform industries and open up new possibilities in fields like sports analytics, energy management, and autonomous driving. We’ll uncover the unique capabilities that make MAS so effective and examine the tangible benefits they bring.
Get ready for a journey into the world of multi-agent systems. Whether you’re a tech enthusiast, an industry professional, or simply curious about the future of AI, discover how these collaborative networks of intelligent agents are shaping the world around us, one distributed task at a time.
Enhancing Sports Training and Medicine with Multi-Agent Systems
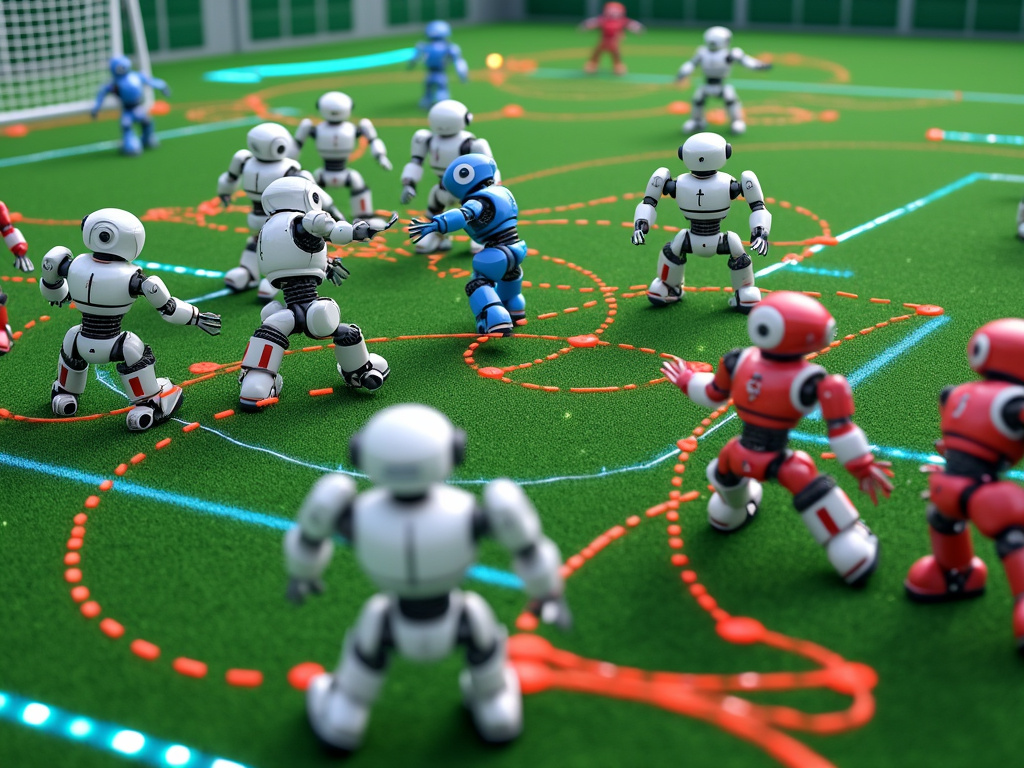
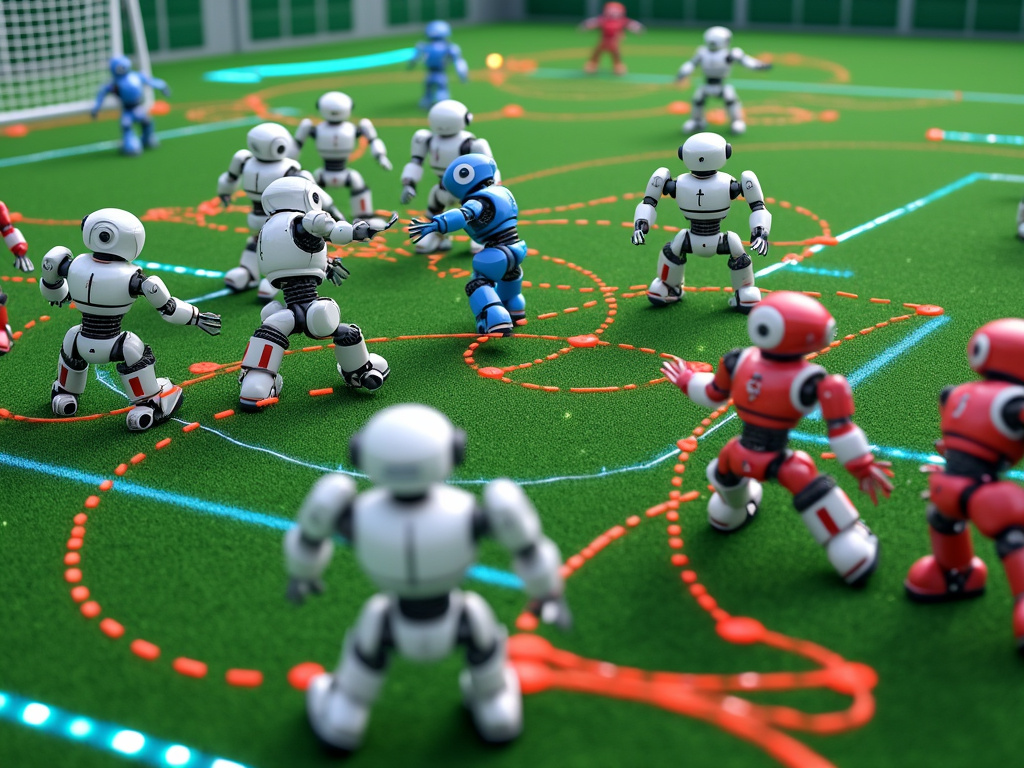
The world of sports training and medicine is undergoing a seismic shift, thanks to the advent of multi-agent systems (MAS).
These sophisticated networks of interacting intelligent agents are revolutionizing how we approach athlete performance, injury prevention, and recovery. By harnessing the power of smart diagnostics and decision support, MAS are providing coaches, trainers, and medical professionals with unprecedented insights and capabilities.
One of the most exciting applications of MAS in sports is in the realm of performance analysis. Imagine a system where multiple AI agents simultaneously track an athlete’s movements, vital signs, and environmental factors during training or competition. This network of observers can detect subtle patterns and anomalies that might escape even the most keen-eyed human coach. For instance, a MAS could identify a slight imbalance in a sprinter’s gait that, if left uncorrected, could lead to injury or suboptimal performance.
But MAS doesn’t stop at just observing; it’s actively shaping training regimens. By analyzing vast amounts of data from various sources, these systems can suggest personalized training routines tailored to each athlete’s unique physiology, strengths, and weaknesses. A gymnast preparing for the Olympics might receive a dynamically adjusted training plan that takes into account factors like muscle fatigue, recovery rates, and even psychological readiness.
The future of sports medicine lies in predictive analytics and personalized interventions. Multi-agent systems are the key to unlocking this potential.
In the field of sports medicine, MAS are proving to be game-changers for injury prevention and recovery. These systems can analyze an athlete’s movement patterns, stress levels, and physiological markers to predict potential injuries before they occur. For a professional basketball player, this might mean receiving an alert to modify their jump shot technique to reduce strain on their knees, potentially extending their career by years.
When injuries do occur, MAS offer invaluable support in the recovery process. By continuously monitoring an athlete’s progress and comparing it to vast databases of similar cases, these systems can provide real-time adjustments to rehabilitation protocols. A recovering soccer player might receive personalized exercises and progress tracking through a smartphone app, with the underlying MAS ensuring their recovery is optimized and safe.
The impact of MAS extends beyond individual athletes to entire teams and sports organizations. These systems can analyze team dynamics, opponent strategies, and even fan engagement to provide holistic insights that can influence everything from game tactics to franchise management decisions.
As we look to the future, the potential of MAS in sports training and medicine seems boundless. From elite athletes pushing the limits of human performance to weekend warriors seeking to stay fit and injury-free, multi-agent systems are set to transform how we approach physical activity and wellness. The integration of MAS with emerging technologies like virtual reality training environments and advanced wearable sensors promises to unlock new frontiers in sports science.
While the technology is impressive, it’s important to remember that MAS are tools to enhance, not replace, human expertise. The most effective implementations of these systems will likely be those that seamlessly blend artificial intelligence with the intuition and experience of skilled coaches and medical professionals.
As multi-agent systems continue to evolve and become more sophisticated, we can expect to see even more remarkable applications in sports training and medicine. The era of truly personalized, data-driven athletic development is here, and it’s changing the game for athletes at all levels.
Smart Energy Management
Multi-agent systems (MAS) are transforming smart grid management in the energy sector. These intelligent networks integrate diverse energy sources, optimizing their usage for maximum efficiency. By coordinating the output from thermal, wind, solar, and hydroelectric power plants, MAS ensure a stable and reliable power supply while minimizing waste.
The benefits of smart energy management systems include:
- Enhanced grid stability
- Improved energy distribution
- Reduced power wastage
- Seamless integration of renewable sources
- Lower operational costs
Smart grids powered by MAS can adapt in real-time to fluctuating energy demands. This dynamic responsiveness allows the system to balance loads effectively, drawing from the most efficient sources as needed. For instance, during peak hours, the system might prioritize solar and wind power, while relying more on hydroelectric or thermal sources during off-peak times.
The coordination capabilities of MAS extend beyond power plants to smart houses and factories. These intelligent agents can manage energy consumption at the consumer level, optimizing usage patterns to reduce overall demand on the grid. This comprehensive approach to energy management results in a more sustainable and cost-effective power infrastructure.
As we move towards a greener future, the role of smart energy management systems becomes increasingly crucial. By harnessing the power of multi-agent systems, we can create a more resilient, efficient, and environmentally friendly energy landscape.
Autonomous Driving and Traffic Management
The future of transportation is being shaped by autonomous driving technologies that leverage multi-agent systems (MAS) for seamless vehicle coordination and traffic management. Self-driving cars are part of an interconnected ecosystem that includes other vehicles, pedestrians, and traffic infrastructure.
Autonomous vehicles can communicate and cooperate using advanced sensors and artificial intelligence. Self-driving cars share real-time data about road conditions, potential hazards, and their intended actions. This constant exchange of information creates a collaborative environment where vehicles work together to navigate complex traffic scenarios.
The benefits of MAS in autonomous driving are profound. By enabling vehicles to coordinate their movements, MAS significantly reduces the risk of collisions and improves overall road safety. Imagine a scenario where a self-driving car detects a pedestrian stepping onto the road – it can instantly relay this information to nearby vehicles, allowing them to slow down or change lanes preemptively.
Beyond safety, MAS also holds the key to unlocking unprecedented levels of traffic efficiency. Through coordinated decision-making, autonomous vehicles can optimize traffic flow, reducing congestion and travel times. For instance, during merging situations on highways, vehicles can seamlessly adjust their speeds and positions to create gaps, eliminating the stop-and-go pattern that often leads to traffic jams.
Multi-agent systems are the unsung heroes of autonomous driving, orchestrating a complex dance of vehicles to create safer and more efficient roads for everyone.
Dr. Amanda Prorok, University of Cambridge
Companies like Waymo are at the forefront of implementing these technologies, using sophisticated traffic simulations to test and refine their autonomous driving systems. These simulations allow engineers to model countless scenarios and edge cases, ensuring that self-driving cars can handle the unpredictability of real-world traffic.
As autonomous vehicles become more prevalent, the role of traffic management systems will evolve. Traditional traffic lights and signs may give way to dynamic, AI-driven systems that adapt in real-time to changing traffic conditions. This synergy between autonomous vehicles and smart infrastructure promises to revolutionize urban mobility, making our cities more livable and sustainable.
The integration of MAS in autonomous driving represents a paradigm shift in how we approach transportation. By fostering cooperation between vehicles, pedestrians, and infrastructure, we’re building an intelligent, responsive transportation network that prioritizes safety, efficiency, and sustainability.
Implementing Multi-Agent Systems in Healthcare
Multi-Agent Systems (MAS) are enhancing diagnostics, patient monitoring, and data analysis in healthcare. These interconnected systems are transforming medical care, leading to more efficient and effective services.
In diagnostics, MAS improve accuracy and speed by leveraging distributed intelligence. AI agents analyze different aspects of a patient’s medical data— from lab results to imaging scans—providing a comprehensive diagnostic picture quicker than traditional methods. This collaborative approach reduces missed diagnoses and allows for earlier detection of health issues.
Patient monitoring benefits significantly from MAS. These systems track vital signs, medication adherence, and lifestyle factors, alerting healthcare providers to concerning changes in real-time. This proactive approach can prevent complications and reduce hospital readmissions. For example, a study of heart failure patients showed that a MAS-based monitoring system could predict and prevent adverse events with remarkable accuracy, potentially saving lives and reducing healthcare costs.
MAS demonstrate transformative potential in data analysis. By processing vast amounts of medical data from diverse sources, these systems identify patterns and insights that might escape human observation. This capability is valuable in epidemiology and public health, where MAS can track disease outbreaks, predict future health trends, and inform policy decisions.
MAS significantly improve the coordination of patient care, a historically challenging aspect of healthcare. These systems facilitate seamless communication between healthcare providers, ensuring access to up-to-date patient information. This coordinated approach reduces errors, eliminates redundant tests, and leads to better patient outcomes.
Case Study: MAS in Action
A recent implementation at a large urban hospital network demonstrates MAS effectiveness. The network used a multi-agent system to manage patient flow, coordinate care teams, and optimize resource allocation. Within six months, the hospital reported a 15% reduction in average length of stay, a 20% improvement in operating room utilization, and a significant increase in patient satisfaction scores.
The system’s success was due to its ability to adjust dynamically to changing conditions, making real-time decisions that human managers might struggle to coordinate. For instance, when a sudden influx of emergency cases threatened to overwhelm the ER, the MAS quickly reallocated resources from less critical areas, ensuring timely care for all patients.
Multi-agent systems in healthcare are not just about efficiency; they create a more responsive, patient-centered care experience. By automating complex decision-making processes, healthcare professionals can focus on the human aspect of care.
Dr. Elena Rodriguez, Chief of Innovation at HealthTech Solutions
While the benefits of MAS in healthcare are clear, challenges remain. Issues of data privacy, system interoperability, and the need for regulatory frameworks are active areas of research and development. As these challenges are addressed, we can expect more widespread adoption of MAS across the healthcare sector, promising a future of personalized, efficient, and effective medical care for all.
MAS in Industrial Automation
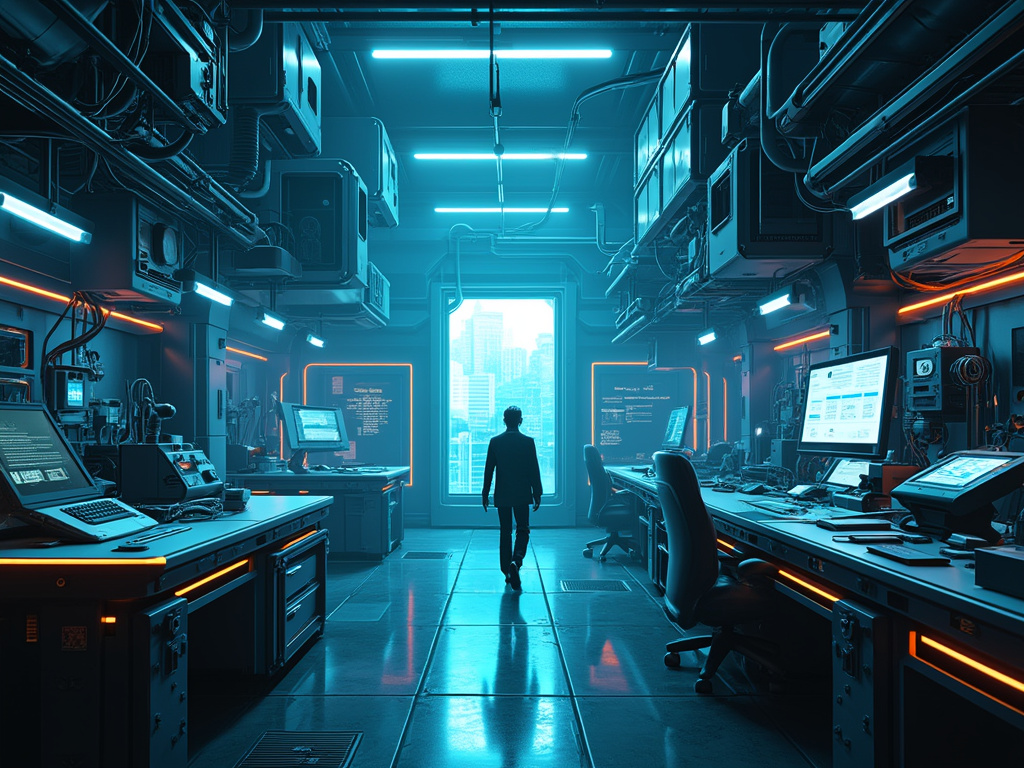
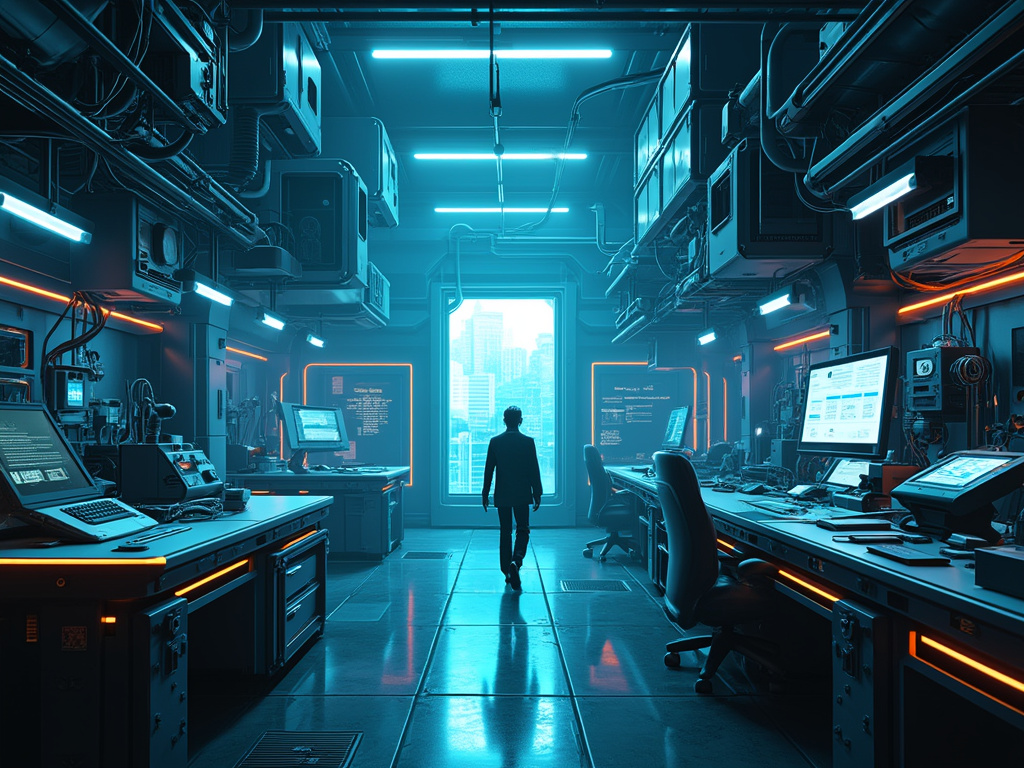
A figure in a high-tech lab with robotic machines.
Multi-agent systems (MAS) are transforming industrial automation by enabling more flexible, adaptive, and efficient manufacturing and logistics processes. These distributed AI systems consist of multiple autonomous agents that can communicate, coordinate, and make decisions to optimize operations in real-time.
In manufacturing, MAS coordinate the activities of robots, machines, and other equipment on the factory floor. For example, Toyota uses a multi-agent system called the Autonomous Negotiating Flexible Manufacturing System to dynamically allocate tasks and resources across its production lines. This allows Toyota to rapidly reconfigure manufacturing processes in response to changes in demand or supply chain disruptions.
Logistics and supply chain management also benefit significantly from MAS implementations. DHL employs multi-agent systems to optimize routing and scheduling of its delivery vehicles. The agents representing individual trucks can communicate and coordinate to dynamically adjust routes based on real-time traffic data, new delivery requests, and other changing conditions. This has enabled DHL to reduce fuel consumption by 15% and increase on-time deliveries.
The most impactful application of MAS in industrial settings is in real-time decision making and optimization. Traditional centralized control systems struggle to handle the complexity of modern manufacturing and logistics operations. In contrast, MAS can process massive amounts of sensor data and make split-second decisions locally. Siemens has reported a 30% increase in overall equipment effectiveness after implementing a multi-agent control system in its electronic component plants.
While MAS offer compelling benefits, implementing these systems does present challenges. Integrating MAS with legacy industrial control systems requires significant engineering effort. There are also concerns around security, reliability, and predictability of emergent agent behaviors. However, as more success stories emerge, the adoption of MAS in industrial automation is poised to accelerate in the coming years.
Multi-agent systems are enabling a new paradigm of flexible, intelligent industrial automation. As MAS technology matures, we will see dramatic improvements in manufacturing agility, supply chain resilience, and operational efficiency across industries.
Dr. Elena Rodriguez, Industrial AI Researcher
Ultimately, multi-agent systems are proving to be a powerful tool for enhancing industrial automation. By facilitating real-time optimization and decision-making, MAS drive measurable improvements in productivity and cost-efficiency. As more manufacturers and logistics providers adopt this technology, we can expect even greater innovation in smart factories and supply chains of the future.
Challenges and Future Directions
Multi-agent systems (MAS) have demonstrated remarkable potential in tackling complex problems across various domains. However, as these systems evolve and expand, they face significant challenges that researchers and developers must address to unlock their full potential.
One of the primary hurdles in MAS is ensuring secure communications between agents. As agents exchange sensitive information and collaborate on critical tasks, protecting their interactions from unauthorized access or malicious interference becomes paramount. Developing robust encryption protocols and authentication mechanisms tailored for the dynamic nature of multi-agent environments is crucial for widespread adoption in high-stakes applications.
Another pressing challenge lies in enabling effective coordination among agents, especially in large-scale systems with heterogeneous agents. Achieving consensus, managing conflicting goals, and optimizing resource allocation in real-time pose significant technical and algorithmic challenges. Researchers are exploring advanced machine learning techniques and decentralized decision-making frameworks to enhance agent coordination and adaptability in complex, unpredictable environments.
The future of multi-agent systems appears promising, with several exciting directions on the horizon. One area of focus is the integration of context awareness into MAS, allowing agents to better understand and respond to their environment and the intentions of other agents. This enhanced situational awareness could dramatically improve the efficiency and effectiveness of agent interactions.
Another frontier is the development of more sophisticated learning mechanisms that enable agents to collectively acquire knowledge and skills over time. Techniques such as federated learning and multi-agent reinforcement learning show promise in creating systems that can continuously adapt and improve their performance through shared experiences.
As these challenges are addressed, MAS applications are expected to expand into new domains. From optimizing smart city infrastructure to coordinating swarms of autonomous vehicles or drones, the potential applications are vast. The key to realizing this potential lies in overcoming the current limitations while maintaining the flexibility and scalability that make multi-agent systems so powerful.
Last updated:
Disclaimer: The information presented in this article is for general informational purposes only and is provided as is. While we strive to keep the content up-to-date and accurate, we make no representations or warranties of any kind, express or implied, about the completeness, accuracy, reliability, suitability, or availability of the information contained in this article.
Any reliance you place on such information is strictly at your own risk. We reserve the right to make additions, deletions, or modifications to the contents of this article at any time without prior notice.
In no event will we be liable for any loss or damage including without limitation, indirect or consequential loss or damage, or any loss or damage whatsoever arising from loss of data, profits, or any other loss not specified herein arising out of, or in connection with, the use of this article.
Despite our best efforts, this article may contain oversights, errors, or omissions. If you notice any inaccuracies or have concerns about the content, please report them through our content feedback form. Your input helps us maintain the quality and reliability of our information.