Knowledge Graph Examples
Making sense of vast amounts of interconnected information can feel like trying to complete a complex puzzle. This is where knowledge graphs come in—powerful tools that help us see the bigger picture by connecting all the pieces meaningfully.
Think of a knowledge graph as a digital map that shows how different pieces of information relate to each other. Just as a road map connects cities with highways, a knowledge graph links data points with meaningful relationships. For example, knowledge graphs enable search engines to understand that when you search for ‘famous actors’, you’re looking for people who perform in movies—not just random words on a page.
The healthcare industry has embraced knowledge graphs to improve patient care. Doctors can now see connections between symptoms, diseases, treatments, and patient histories in ways that weren’t possible before. This helps them make faster, more informed decisions about patient care while reducing the risk of overlooking important medical relationships.
But the applications don’t stop there. From helping companies manage complex product catalogs to enabling scientists to discover new drug interactions, knowledge graphs are transforming how we handle and learn from data across countless industries. Their ability to represent and process information in ways that mirror human understanding makes them an invaluable tool in our increasingly complex digital world.
As we explore various real-world examples throughout this article, you’ll discover how these versatile tools are helping organizations make better decisions, uncover hidden insights, and tackle some of today’s most challenging data management problems.
Improving Search Engine Performance
Knowledge graphs have fundamentally transformed how search engines deliver results, moving beyond simple keyword matching to truly understanding user intent. Searching for “apple” today allows search engines to distinguish whether you’re interested in the tech company, the fruit, or Apple Records, showcasing a level of contextual awareness that was impossible with traditional search methods.
Unlike conventional search engines that relied heavily on exact keyword matches, modern systems powered by knowledge graphs map intricate relationships between different entities. For example, a search for “who played James Bond” doesn’t just return pages containing those keywords — it understands you’re asking about actors and their relationship to the James Bond franchise, delivering a comprehensive overview of all Bond actors through the years.
Google’s Knowledge Graph exemplifies this evolution in search technology. When you search for a famous musician, it doesn’t just find pages mentioning their name — it understands relationships between the artist, their albums, band members, and musical influences, providing rich, contextual information instantly in the search results.
These context-aware capabilities dramatically improve the search experience. Rather than forcing users to click through multiple pages to piece together information, knowledge graphs enable search engines to present comprehensive, interconnected data directly in the results. A search for “Barack Obama family” immediately shows family relationships, complete with photos and key information about each family member.
The true power of knowledge graphs lies in their ability to understand semantic relationships. When searching for “tallest buildings”, the search engine doesn’t just look for those exact words — it understands the concept of height, architectural structures, and can even make comparisons between different buildings. This semantic understanding enables more natural, conversational searches while delivering more accurate and relevant results.
Applications in Healthcare
Knowledge graphs are transforming how medical professionals make critical decisions in healthcare. By connecting patients’ symptoms, diagnosed conditions, prescribed treatments, and medical histories, these systems enable a nuanced approach to patient care.
One impactful application is in clinical decision support, where knowledge graphs help healthcare professionals understand the rationale behind predictions. When a patient presents with multiple symptoms, the system analyzes similar historical cases, helping doctors identify potential diagnoses. This capability is valuable in complex cases where symptoms may point to several conditions.
Knowledge graphs personalize treatment recommendations by considering each patient’s unique medical profile. When prescribing medications, these systems can flag potential drug interactions based on a patient’s current prescriptions, allergies, and health conditions, preventing adverse reactions and improving patient safety.
The impact of these systems extends to everyday clinical scenarios. For example, in treating a patient with diabetes, a knowledge graph can analyze blood sugar patterns, medication history, lifestyle factors, and potential complications. This comprehensive view allows healthcare providers to make informed decisions about treatment plans and preventive measures.
Beyond individual care, knowledge graphs help identify broader patterns in healthcare delivery. By analyzing relationships across large patient populations, these systems can reveal connections between treatments and outcomes, leading to more effective clinical protocols. This capability transforms medical data into actionable insights that improve patient care quality.
The benefits of knowledge graphs in healthcare are evident in emergency settings, where quick, accurate decisions are crucial. These systems can rapidly process a patient’s complete medical history, current symptoms, and potential drug interactions, providing emergency room doctors with vital information when minutes matter. This advancement represents a significant step forward in delivering safer, more effective healthcare.
Enhancing Recommendation Systems
Modern recommendation systems have evolved beyond simple product suggestions, thanks to the powerful capabilities of knowledge graphs. These intelligent systems now map complex relationships between users, products, and behaviors to deliver highly personalized recommendations that feel surprisingly human. E-commerce giants leverage knowledge graphs to understand not just what customers buy, but why they make those purchases.
For instance, when a customer buys a laptop, the system recognizes patterns showing that buyers often need related items like wireless mice or laptop bags. Research shows that this deeper understanding of product relationships significantly improves recommendation accuracy. S
Streaming services like Netflix and Spotify utilize knowledge graphs to understand the complex web of content preferences. Instead of simply noting that you watched an action movie, these systems delve deeper to identify the specific elements you enjoy—such as intricate plot twists or particular actors. They use these insights to recommend similar content that aligns with your unique preferences.
Social media platforms have particularly excelled in creating personalized recommendations. By analyzing the relationships between users, posts, and engagement patterns, they develop comprehensive maps of user interests. For instance, when you interact with a post about hiking, the platform doesn’t just show you more hiking content; it understands the wider context of outdoor activities and lifestyle choices.
The true strength of knowledge graph-enhanced recommendations is their ability to address the ‘cold start’ problem—helping new users with limited history find items they might enjoy. By recognizing the broader context and relationships between items, these systems can make educated guesses about new users’ preferences based on minimal information.
Knowledge graphs elevate recommendation systems from basic suggestion engines to sophisticated understanding tools that can anticipate and meet user needs, often before they are even articulated. Most impressively, these systems learn and adapt in real time.
Every interaction, purchase, and piece of feedback is incorporated into the knowledge graph, enhancing the accuracy of recommendations over time. This ongoing learning process ensures that users receive increasingly relevant suggestions that evolve with their changing preferences.
Fraud Detection and Security
Knowledge graphs have emerged as a powerful tool in the fight against financial fraud and security threats. These sophisticated systems excel at spotting patterns that humans might miss in vast amounts of data. By connecting millions of discrete data points into meaningful relationships, knowledge graphs can quickly flag suspicious activities that traditional systems often overlook.
Financial institutions are leading the deployment of these intelligent systems. According to recent research, banks and credit card companies use knowledge graphs to analyze complex transaction patterns, transforming their approach to fraud detection. Instead of examining individual transactions in isolation, these systems map out entire networks of financial activity, making it easier to spot coordinated fraud attempts.
The real power of knowledge graphs lies in uncovering hidden relationships. For instance, when a seemingly legitimate transaction occurs, the system can instantly map it against historical patterns, connected accounts, and known fraud indicators. This contextual awareness helps identify sophisticated fraud schemes that might appear normal when viewed in isolation.
Pattern recognition capabilities go beyond simple rule-based detection. Knowledge graphs can adapt and learn from new fraud patterns as they emerge. When fraudsters change their tactics, these systems can quickly identify new suspicious patterns by analyzing deviations from established legitimate behavior. This dynamic approach keeps financial institutions ahead of increasingly sophisticated criminal schemes.
The impact on security extends beyond financial transactions. Knowledge graphs excel at detecting unusual behavioral patterns across any complex system. Whether identifying potential security breaches in computer networks or flagging suspicious patterns in insurance claims, these systems provide a comprehensive view of potential threats by connecting seemingly unrelated data points into meaningful patterns.
Traditional rules-based fraud detection typically identifies less than 50% of fraudulent transactions. Knowledge graph-based systems can push detection rates above 90% while dramatically reducing false positives.
Deloitte Cybersecurity Report
Leveraging SmythOS for Knowledge Graph Integration
Modern enterprises dealing with complex data relationships need robust, scalable solutions for knowledge graph development. SmythOS emerges as a groundbreaking platform that streamlines this process through its innovative visual builder interface. Unlike traditional approaches requiring extensive coding, SmythOS enables teams to construct and integrate knowledge graphs through an intuitive drag-and-drop environment.
The platform’s visual debugging environment sets it apart in knowledge graph development. Instead of wrestling with cryptic error messages, developers can track decision paths in real-time and examine each step of their knowledge graph’s reasoning process. This unprecedented visibility ensures optimal performance and reliability while significantly reducing the time typically spent on troubleshooting integration issues.
SmythOS’s enterprise-grade security framework provides essential protection for sensitive knowledge bases, making it suitable for organizations processing millions of knowledge-based queries. The platform implements robust authentication protocols and access controls, ensuring that valuable graph data remains protected while still enabling seamless integration with existing systems.
What truly distinguishes SmythOS is its comprehensive support for major graph databases and semantic technologies. The platform’s architecture accommodates various graph database formats and semantic standards, allowing organizations to leverage their existing knowledge infrastructure while expanding capabilities. This flexibility enables teams to build upon their current investments while exploring new possibilities in knowledge representation.
For teams looking to test and validate their knowledge graph implementations, SmythOS offers a free runtime environment. This testing capability allows organizations to thoroughly evaluate integrations before committing to full deployment, significantly reducing the risks and costs associated with knowledge graph development. Teams can experiment with different configurations and optimize their graph structures without incurring additional expenses during the crucial development phase.
Conclusion and Future Directions in Knowledge Graphs
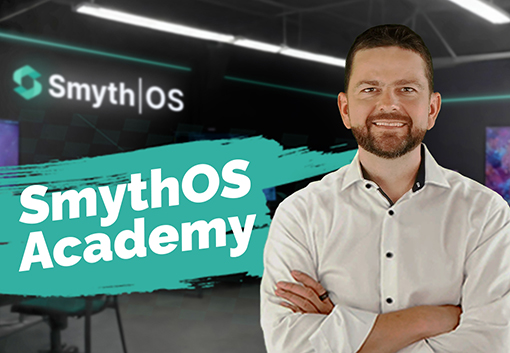
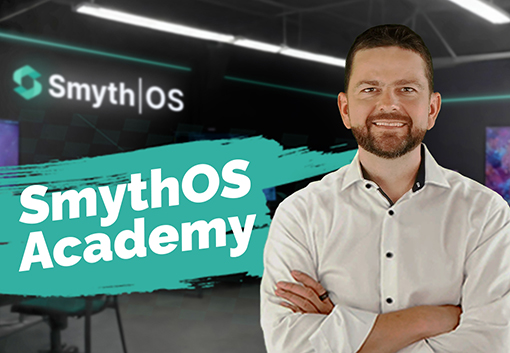
As we stand at the frontier of knowledge graph technology, the possibilities for innovation seem boundless. The evolution of these sophisticated data structures continues to reshape how organizations manage, analyze, and derive value from their complex information networks. Through advancements in automation and machine learning, knowledge graphs are becoming increasingly adept at handling diverse data types and relationships with unprecedented precision.
The integration of knowledge graphs with emerging technologies presents particularly exciting opportunities. Recent research highlights how these systems are becoming more sophisticated in their ability to reason over data, enabling more nuanced insights and decision-making capabilities. This progression toward more intelligent data management promises to transform industries from healthcare to finance, manufacturing to retail.
Automation stands as a key driver in this evolution, with platforms like SmythOS leading the charge in simplifying knowledge graph implementation. By reducing the technical barriers to entry, these tools are democratizing access to advanced data management capabilities, allowing organizations of all sizes to harness the power of connected data. The future points toward even more seamless integration techniques, where knowledge graphs become an integral part of enterprise architecture rather than standalone solutions.
The path forward will likely see knowledge graphs becoming increasingly accessible through no-code and low-code platforms, enabling broader adoption across industries. This democratization of technology will accelerate innovation, as more organizations can experiment with and implement knowledge graph solutions without requiring extensive technical expertise. The focus will shift from basic implementation to sophisticated applications that drive real business value.
As we look ahead, the importance of knowledge graphs in shaping our digital future cannot be overstated. Their ability to connect, contextualize, and derive meaning from vast amounts of data will become increasingly crucial in our data-driven world. The next frontier lies in developing more intuitive interfaces, enhanced automation capabilities, and deeper integration with existing business systems, ultimately leading to more intelligent and responsive organizations.
Last updated:
Disclaimer: The information presented in this article is for general informational purposes only and is provided as is. While we strive to keep the content up-to-date and accurate, we make no representations or warranties of any kind, express or implied, about the completeness, accuracy, reliability, suitability, or availability of the information contained in this article.
Any reliance you place on such information is strictly at your own risk. We reserve the right to make additions, deletions, or modifications to the contents of this article at any time without prior notice.
In no event will we be liable for any loss or damage including without limitation, indirect or consequential loss or damage, or any loss or damage whatsoever arising from loss of data, profits, or any other loss not specified herein arising out of, or in connection with, the use of this article.
Despite our best efforts, this article may contain oversights, errors, or omissions. If you notice any inaccuracies or have concerns about the content, please report them through our content feedback form. Your input helps us maintain the quality and reliability of our information.