Best Knowledge Graph Courses to Enhance Your Skills
Imagine organizing vast amounts of information as naturally as your brain connects memories and ideas. That’s exactly what knowledge graphs do for modern businesses and organizations, creating an intelligent web of data that mirrors human understanding. Whether you’re a data scientist looking to enhance your skills or an enterprise architect seeking to transform your organization’s information systems, mastering knowledge graphs has become increasingly crucial in today’s data-driven world.
According to Enterprise Knowledge’s training programs, professionals who understand knowledge graphs are uniquely positioned to solve complex data challenges, from creating comprehensive customer views to powering advanced search capabilities. The growing demand for these skills has led to an explosion of specialized courses and certifications, each designed to help you harness the power of this transformative technology.
In this comprehensive guide, we’ll explore the best knowledge graph courses and certifications available today. You’ll discover learning paths suited for every skill level – from beginners just starting their journey to experienced professionals looking to deepen their expertise. We’ll examine key features of top programs, practical applications you’ll master, and essential skills you’ll develop along the way.
Whether your goal is to enhance enterprise data management, build more intelligent search systems, or develop cutting-edge AI applications, understanding knowledge graphs is your gateway to unlocking deeper insights from complex data relationships. Let’s explore how you can build this valuable expertise through carefully selected courses and certifications that align with your professional goals.
Choosing the Right Knowledge Graph Course
Knowledge graph courses have become essential learning paths for data professionals, offering options from introductory tutorials to comprehensive specializations. When selecting a course, consider several critical factors to ensure alignment with your learning objectives and career aspirations. Course content is the primary factor in your decision-making process.
Top-rated programs like DeepLearning.AI’s Knowledge Graphs for RAG focus on practical applications, teaching you to manage and retrieve data using Neo4j’s query language Cypher. The curriculum should match your expertise level, whether you’re a beginner learning fundamentals or an advanced practitioner seeking specialized knowledge in areas like semantic web technologies or graph databases.
Duration and time commitment are crucial factors to consider when choosing a course. Courses can vary significantly in length, ranging from short two-hour guided projects to comprehensive specializations lasting three to six months. For working professionals, shorter modules, such as Coursera’s guided projects, provide flexible learning options that can be completed during off-hours. In contrast, intensive programs like Stanford’s specialization in Probabilistic Graphical Models require several months of dedicated study but offer deeper theoretical foundations.
The value of certification is another important aspect. Professional certificates from recognized institutions like DeepLearning.AI or Stanford carry substantial weight in the job market. However, the real value lies in the practical skills and knowledge gained, rather than the credential itself. Consider whether potential employers in your field value specific certifications as you make your choice.
The reputation of the platform and the delivery format are also worth considering. Leading platforms like Coursera partner with prestigious universities and industry leaders to provide high-quality content. The learning environment should include interactive elements, hands-on projects, and comprehensive support resources that cater to your learning style. Some learners excel in self-paced environments, while others thrive in structured, cohort-based programs that include regular interaction with instructors.
Finally, weigh the cost against the value of each course when making your selection. Although some platforms offer free course access, premium features such as graded assignments, certificates, and personalized feedback often require payment. Evaluate your budget alongside the potential career benefits and skill development opportunities each course offers. Many platforms also provide financial aid or subscription models, making premium content more accessible.
Top Online Platforms for Knowledge Graph Courses
The landscape of knowledge graph education has evolved significantly, with several major platforms emerging as leaders in this specialized field. Each platform offers a unique approach to teaching this complex subject, catering to different learning styles and professional goals.
Coursera stands out with its academically rigorous offerings, featuring courses from prestigious institutions like Stanford and Yale. Their knowledge graph courses dive deep into theoretical foundations while maintaining practical relevance. As noted in a recent Class Central analysis, Coursera’s courses combine comprehensive academic content with hands-on projects, making them ideal for those seeking both theoretical understanding and practical application.
LinkedIn Learning focuses on industry-oriented skills and practical implementation. Their knowledge graph courses feature seasoned professionals sharing real-world experiences and practical techniques. The platform excels at teaching hands-on skills that can be immediately applied in professional settings, with courses typically running shorter than their academic counterparts.
Class Central serves as an invaluable aggregator platform, helping learners navigate the vast landscape of knowledge graph courses across different providers. Their curated collections make it easier to compare courses based on factors like duration, difficulty level, and user ratings. This platform shines for those who want to explore multiple learning options before committing to a specific course.
While all three platforms offer high-quality content, their teaching methodologies differ significantly. Coursera’s university-partnered courses often include peer-reviewed assignments and formal assessments, creating a more structured learning environment. LinkedIn Learning’s courses typically feature bite-sized modules and practical exercises, making them more suitable for busy professionals seeking to upskill quickly.
The integration of knowledge graphs into modern data infrastructure has created an urgent need for skilled professionals. These platforms are responding to this demand with increasingly sophisticated and specialized course offerings.
Dr. Anju Kambadur, Bloomberg’s AI Research Lead
Each platform also offers unique benefits when it comes to certification. Coursera’s certificates, especially those from university partners, often carry significant weight in academic circles. LinkedIn Learning’s certificates, while perhaps less academically oriented, integrate seamlessly with LinkedIn profiles, potentially catching the eye of recruiters and hiring managers in professional networks.
Key Topics Covered in Knowledge Graph Courses
Modern knowledge graph courses delve into several fundamental technologies that form the backbone of semantic data representation. At their core, these courses explore RDF (Resource Description Framework) triples, which serve as the basic building blocks for expressing relationships between entities in a standardized format. Think of RDF triples as digital sentences that connect pieces of information – much like how we naturally express ideas through subject-predicate-object relationships.
SPARQL querying is another crucial component in the knowledge graph curriculum. This specialized query language enables developers and data scientists to retrieve and manipulate data stored in knowledge graphs effectively. Leading courses emphasize SPARQL’s role in building powerful search functionality and extracting meaningful insights from complex data relationships.
The study of ontologies with OWL (Web Ontology Language) represents a significant portion of knowledge graph education. These semantic frameworks help define the vocabulary and rules that govern how information can be represented and related within a knowledge graph. Mastering ontologies allows practitioners to create rich, meaningful data models that capture the nuances of real-world relationships and concepts.
Perhaps most exciting is the exploration of knowledge graphs in artificial intelligence and machine learning applications. Students learn how these structured data representations can enhance AI systems by providing context and reasoning capabilities beyond what’s possible with traditional databases. Knowledge graphs have become particularly valuable in improving the output of large language models, offering a way to ground AI responses in verified, structured information.
Knowledge graphs serve as the foundation of today’s cutting-edge information systems, from improving search results to developing explainable AI systems.
Prof. Dr. Harald Sack, FIZ Karlsruhe
The integration of these topics creates a comprehensive understanding of how to design and implement effective knowledge graphs. Modern courses emphasize practical applications, showing how these technologies work together to power everything from intelligent search engines to sophisticated question-answering systems, making complex data relationships both understandable and actionable.
Benefits of Learning Knowledge Graphs
Knowledge graphs represent a powerful approach to organizing and understanding complex information, transforming how we handle data across industries. Mastering knowledge graph technology allows you to structure and connect information in ways that mirror human understanding while enabling sophisticated machine processing.
One of the most significant advantages of knowledge graphs is their exceptional capability for organizing complex data relationships. Unlike traditional databases, knowledge graphs can capture nuanced connections between different pieces of information through a flexible network structure. Research from AIMultiple highlights this, allowing for richer context and a more natural representation of how different concepts relate to one another.
The impact on information retrieval is particularly noteworthy. Knowledge graphs enable more intuitive and accurate search capabilities by understanding the semantic relationships between different pieces of information. Rather than relying on simple keyword matching, knowledge graph-powered systems can understand the context and meaning behind queries, delivering more relevant results. This semantic understanding makes it possible to find connections that might otherwise remain hidden in traditional data structures.
Entity 1 | Relationship | Entity 2 |
---|---|---|
Neurotrophin-3 | biological process | memory |
Professor | teaches | Course |
Student | enrolls in | Program |
Customer | purchases | Product |
Patient | has | Medical Condition |
Product | belongs to | Category |
Employee | works at | Company |
Company | located in | City |
In artificial intelligence and machine learning, knowledge graphs serve as a crucial foundation for more sophisticated applications. They provide AI systems with structured, contextual information that helps improve everything from recommendation systems to natural language processing. For instance, in healthcare settings, knowledge graphs help AI systems understand complex relationships between symptoms, diseases, and treatments, leading to more accurate diagnostic support tools.
Practical applications extend across numerous industries. In financial services, knowledge graphs help detect fraud patterns and assess risk by analyzing complex networks of transactions and relationships. E-commerce platforms leverage them to create more personalized shopping experiences and product recommendations. Healthcare organizations use knowledge graphs to integrate patient data with medical knowledge bases, enabling more comprehensive and accurate patient care.
Leveraging SmythOS for Knowledge Graph Development
SmythOS transforms knowledge graph development through its innovative visual workflow builder. Unlike traditional approaches that require extensive coding expertise, this platform empowers both technical and non-technical teams to create sophisticated knowledge graph applications using an intuitive drag-and-drop interface.
The platform’s standout feature is its comprehensive debugging environment. SmythOS’s built-in visual debugger enables developers to examine knowledge graph workflows in real-time, allowing for quick identification and resolution of issues. This approach significantly reduces development time while ensuring accuracy in data connections and relationships.
Enterprise organizations particularly benefit from SmythOS’s robust security infrastructure. The platform implements stringent security measures to protect sensitive knowledge bases while maintaining seamless integration capabilities with existing enterprise systems. This enterprise-grade security makes SmythOS an ideal choice for organizations handling confidential information within their knowledge graphs.
The platform’s process agents handle much of the heavy lifting in knowledge graph creation and maintenance. These intelligent agents automatically pull data from various sources and organize information into meaningful connections, reducing manual effort while ensuring consistency across the knowledge graph structure. The automation capabilities minimize human error and accelerate development cycles.
SmythOS’s visual builder sets a new standard for knowledge graph development by offering an extensive library of reusable components. These pre-built blocks seamlessly integrate into workflows, allowing developers to focus on customizing and optimizing their knowledge graphs rather than building basic functionality from scratch. This approach dramatically reduces implementation time while ensuring consistent quality across projects.
SmythOS isn’t just another AI tool. It’s transforming how we approach AI debugging. The future of AI development is here, and it’s visual, intuitive, and incredibly powerful.
Enterprise Knowledge
Conclusion and Future Scope
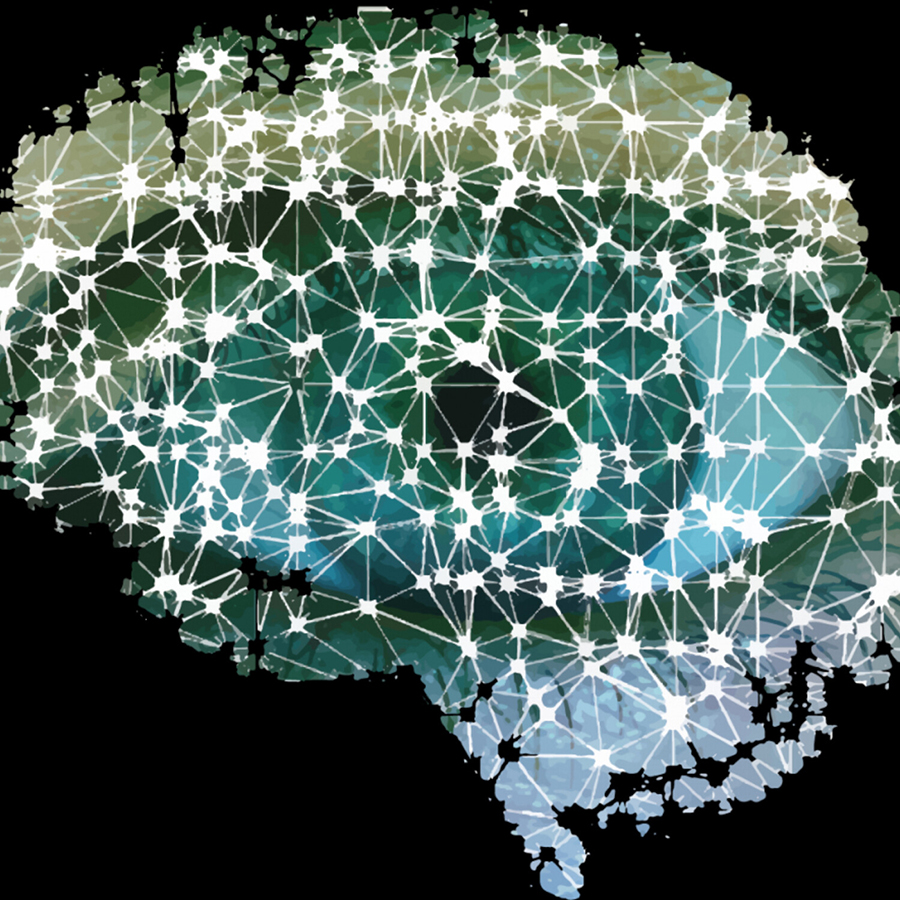
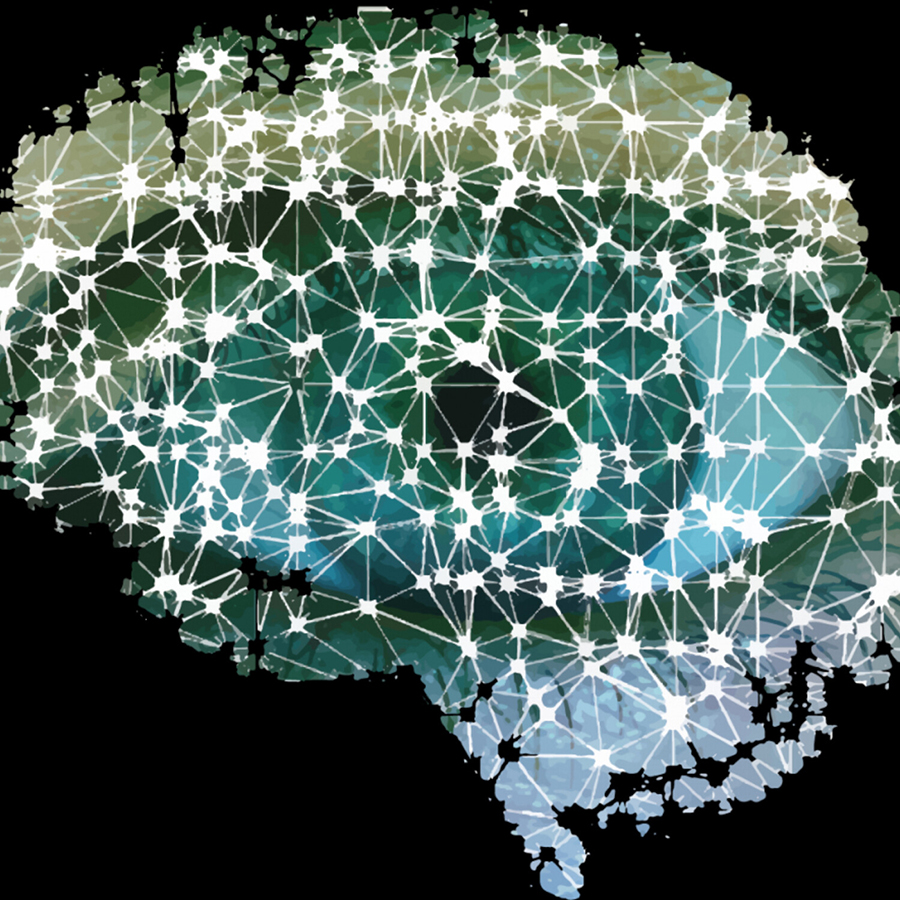
Knowledge graphs represent a transformative force in modern data organization and artificial intelligence. Their ability to structure complex relationships and enhance machine learning capabilities has proven invaluable across numerous domains, from healthcare to finance. The future of knowledge graph technology appears promising, particularly with more sophisticated AI applications emerging.
Recent advancements in Neural Symbolic AI demonstrate how knowledge graphs are evolving to bridge the gap between symbolic and neural approaches, creating AI systems that are both interpretable and versatile. This fusion of deep learning with traditional knowledge representation promises to unlock unprecedented capabilities in data organization and analysis.
The integration of knowledge graphs with large language models represents another frontier in AI development. This combination enhances natural language understanding and enables more sophisticated reasoning capabilities, leading to more accurate and contextually aware AI systems. As these technologies mature, we can expect even more innovative applications across various industries.
Excitingly, knowledge graphs have the potential to democratize AI development. By providing structured representations of complex information, they make advanced AI capabilities more accessible to organizations of all sizes. This democratization will likely accelerate innovation and lead to novel solutions for challenges in data management and artificial intelligence.
The continued evolution of knowledge graph technologies will play a crucial role in shaping the future of AI and data organization. As these systems become more sophisticated and accessible, they will undoubtedly continue to transform how we understand, organize, and utilize information in our connected world.
Last updated:
Disclaimer: The information presented in this article is for general informational purposes only and is provided as is. While we strive to keep the content up-to-date and accurate, we make no representations or warranties of any kind, express or implied, about the completeness, accuracy, reliability, suitability, or availability of the information contained in this article.
Any reliance you place on such information is strictly at your own risk. We reserve the right to make additions, deletions, or modifications to the contents of this article at any time without prior notice.
In no event will we be liable for any loss or damage including without limitation, indirect or consequential loss or damage, or any loss or damage whatsoever arising from loss of data, profits, or any other loss not specified herein arising out of, or in connection with, the use of this article.
Despite our best efforts, this article may contain oversights, errors, or omissions. If you notice any inaccuracies or have concerns about the content, please report them through our content feedback form. Your input helps us maintain the quality and reliability of our information.