Knowledge Graph Applications: Transforming Data into Actionable Insights
Have you ever wondered how Netflix knows exactly what show to recommend, or how Google instantly understands whether you’re searching for Apple the company or apple the fruit? These everyday digital experiences are powered by knowledge graphs – one of the most transformative technologies reshaping how we organize, understand, and extract meaning from vast amounts of data.
Data can be overwhelming, but knowledge graphs offer a powerful solution for making sense of complex information networks. By creating structured, interconnected representations of data, these sophisticated systems are revolutionizing everything from healthcare research to financial fraud detection. According to Search Engine Land, knowledge graphs excel at ‘connecting data points and revealing relationships between them,’ fundamentally transforming how organizations derive value from their information assets.
What makes knowledge graphs particularly fascinating is their ability to mirror how the human brain processes information – through connections and relationships rather than isolated facts. Just as we naturally understand that a smartphone is related to apps, features, and manufacturers, knowledge graphs build similar webs of meaning that machines can interpret and act upon.
Whether you’re a data scientist working on advanced AI applications or a business leader seeking to better understand your customer relationships, knowledge graphs offer a compelling framework for transforming raw data into actionable insights. In this article, we’ll explore the diverse applications of knowledge graphs across industries and uncover the best practices that can help you harness their full potential.
Enhancing Search Engines with Knowledge Graphs
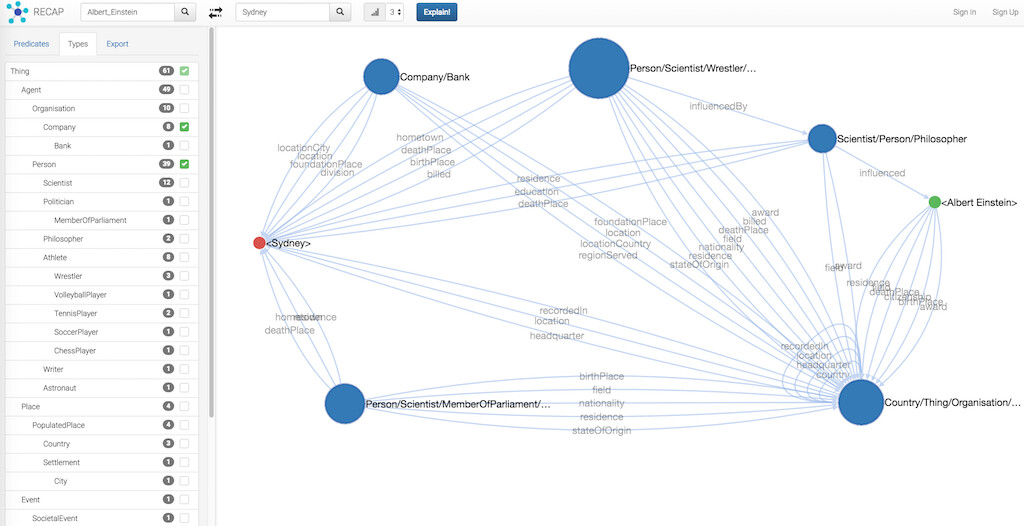
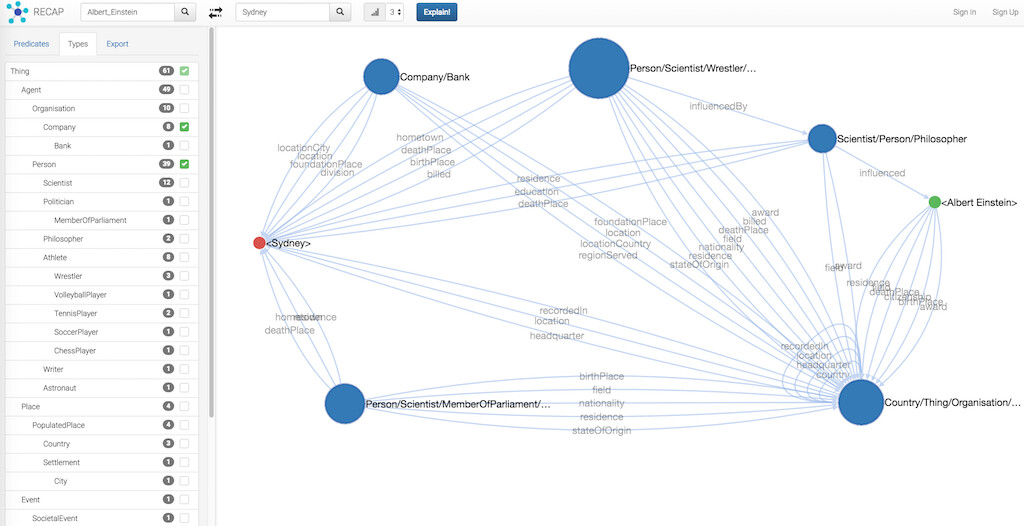
Knowledge graphs have revolutionized how search engines understand and respond to user queries. By creating an interconnected web of information, these sophisticated frameworks enable search engines to grasp the deeper meaning and context behind search terms, moving beyond simple keyword matching to truly comprehend user intent.
At its core, a knowledge graph represents real-world entities and the relationships between them in a structured format. For example, when searching for “Leonardo da Vinci,” the knowledge graph understands not just the name, but recognizes him as an artist, inventor, and historical figure, along with his connections to works like the Mona Lisa and inventions like the aerial screw.
Google’s Knowledge Graph, launched in 2012, exemplifies how this technology transforms search results. Rather than simply matching keywords, it leverages structured data to understand the relationships between people, places, and things. This enables the search engine to provide comprehensive, contextually relevant information directly in search results.
The impact of knowledge graphs extends beyond basic search functionality. They enable search engines to handle complex queries by understanding semantic relationships and context. When a user searches for “famous artist who painted the Mona Lisa,” the knowledge graph can identify Leonardo da Vinci as the correct answer, even though his name wasn’t explicitly mentioned in the query.
Through semantic inference and graph traversal capabilities, knowledge graphs help search engines uncover indirect relationships and patterns that might not be immediately obvious. This allows for more nuanced and accurate search results, ensuring users receive the most relevant information for their queries.
Traditional keyword matching often fails to understand the true intent behind a search query. Knowledge graphs go beyond keywords to grasp the context and relationships between entities.
Search Engine Land
Modern search engines utilize knowledge graphs to deliver dynamic recommendations and contextually aware results. As new information is added to the graph, the system’s understanding evolves, allowing for increasingly sophisticated query processing and more accurate search results.
This continuous enhancement of search capabilities demonstrates why knowledge graphs have become indispensable in modern search technology.
Recommendation Systems Powered by Knowledge Graphs
Knowledge graphs have transformed how recommendation systems deliver personalized content by addressing challenges like data sparsity and cold-start problems. Unlike traditional methods, knowledge graph-based systems use rich semantic relationships between users, items, and their properties to generate more accurate and diverse suggestions.
These advanced systems represent information as interconnected entities and relationships. For instance, when you watch a movie on Netflix, the system understands the movie’s genre, director, actors, and how these elements connect to other content you might enjoy. This web of relationships enables deeper personalization than traditional collaborative filtering.
By construction, knowledge graphs can easily be linked between each other. Beyond the simple lists of properties already managed by previous versions of recommender systems, knowledge graphs represent and leverage semantically rich relations between entities.
Modern recommendation engines powered by knowledge graphs propagate user preferences across this semantic network. When a user interacts with an item, the system can traverse related entities to find similar content through multiple meaningful paths. This leads to more nuanced recommendations that consider context and indirect relationships rather than just surface-level similarities.
The implementation of knowledge graphs in recommendation systems has shown promise in addressing the cold-start problem. Even when a user or item is new, the rich contextual information in the knowledge graph allows for immediate, relevant recommendations based on known properties and relationships. This capability has made knowledge graph-based systems increasingly valuable for platforms needing to quickly engage new users with personalized content.
Enterprise applications of these systems have demonstrated significant improvements in recommendation quality. By incorporating knowledge graphs, businesses can offer more diverse suggestions while maintaining relevance, helping users discover content they might have missed through traditional methods. This balance between exploration and personalization is crucial for modern digital platforms seeking to enhance user engagement and satisfaction.
Fraud Detection and Security Applications
Financial institutions face a growing challenge in detecting increasingly sophisticated fraud schemes. Knowledge graphs provide a powerful solution by connecting seemingly unrelated pieces of information into a comprehensive network of relationships that can reveal suspicious patterns. Picture a spider web of connections linking bank accounts, transactions, and user behaviors. When fraudsters try to hide their activities by using multiple accounts or complex transaction patterns, knowledge graphs can quickly spot these hidden relationships.
For example, one major bank used knowledge graphs to identify repeat offenders bringing in counterfeit goods by connecting information across thousands of documents, addresses, phone numbers, and previous cases. In e-commerce, these systems act like digital detectives, monitoring millions of transactions in real-time. When someone tries to use stolen credit card information to make purchases from different locations or with suspicious patterns, the knowledge graph can instantly flag these activities by recognizing unusual connections between shipping addresses, IP locations, and purchase histories.
The technology is especially powerful because it can adapt and learn from new fraud patterns. Rather than relying on rigid rules that criminals can figure out and bypass, knowledge graphs build an evolving understanding of what normal and suspicious behaviors look like. This makes it much harder for fraudsters to escape detection. Banks and online retailers aren’t the only ones benefiting from this technology. Insurance companies use knowledge graphs to detect fraudulent claims by connecting information about accidents, medical records, and claim histories. When someone tries to file multiple claims for the same incident or works with others to stage accidents, the system can spot these schemes by identifying suspicious patterns in the relationships between people, events, and claims. Graph databases for fraud detection are state-of-the-art because they contain massive amounts of data, and even if one piece of information is incorrect or missing, the system will still be able to identify fraudulent activity effectively.
The impact of this technology extends beyond just catching criminals. By quickly spotting and stopping fraud attempts, knowledge graphs help protect customers’ money and personal information while keeping costs down for businesses. This means safer transactions for everyone and lower fees for honest customers.
Enterprise Knowledge Management
Modern enterprises face a significant challenge: managing vast amounts of institutional knowledge spread across documents, databases, and employees’ minds. Knowledge graphs have emerged as a powerful solution, fundamentally transforming how organizations capture and utilize their collective wisdom.
Enterprise knowledge management through knowledge graphs creates an interconnected web of information that mirrors how humans naturally think and associate ideas. This structured approach allows organizations to map relationships between data points, making previously hidden connections visible and actionable.
Knowledge graphs excel at breaking down traditional information silos that plague large organizations. By creating semantic relationships between different data sources, employees can quickly find relevant information regardless of where it’s stored. This interconnected approach means that searching for a specific product might automatically surface related customer feedback, technical documentation, and expert contacts within the organization.
Enhancing Employee Efficiency
One of the most significant advantages of knowledge graph implementation is its impact on employee productivity. Instead of spending hours searching through disconnected systems, workers can quickly access contextually relevant information through intuitive interfaces.
The system’s ability to understand relationships between different pieces of information means that employees often discover useful connections they wouldn’t have found otherwise. For instance, a sales representative researching a client might automatically receive insights about similar accounts, successful strategies, and potential cross-selling opportunities.
Knowledge graphs also facilitate better decision-making by providing a comprehensive view of available information. When employees can see the full context of a situation, including historical data, related cases, and expert opinions, they’re better equipped to make informed choices.
The technology particularly shines in onboarding new employees. Instead of relying solely on formal training materials, new hires can explore interconnected knowledge bases that provide practical, real-world context for their roles. This accelerates the learning curve and reduces the time needed to achieve full productivity.
Perhaps most importantly, knowledge graphs help preserve institutional knowledge when employees leave or change roles. By capturing not just information but also the relationships between different pieces of knowledge, organizations maintain their intellectual capital even as teams evolve.
Streamlining Information Access
Data accessibility takes on new meaning with knowledge graph implementation. Traditional search systems often struggle with understanding context and intent, but knowledge graphs excel at both. They can interpret queries in a more nuanced way, delivering more relevant results.
The natural language processing capabilities of modern knowledge graph systems mean employees can ask complex questions in plain English and receive meaningful answers. This eliminates the need for specialized query languages or technical expertise to access information.
Security and governance become more manageable with knowledge graphs. Organizations can implement granular access controls while maintaining the interconnected nature of their information. This ensures sensitive data remains protected while still allowing appropriate information sharing.
The system’s ability to maintain data lineage and relationships helps ensure compliance with regulatory requirements. Organizations can track how information flows through their systems and who has accessed it, making audit processes more straightforward.
As data volumes continue to grow, the scalability of knowledge graphs becomes increasingly important. Modern implementations can handle billions of relationships while maintaining performance, making them suitable for even the largest enterprises.
Leveraging SmythOS for Knowledge Graph Development
Knowledge graph development demands sophisticated tools and seamless integration capabilities, which is precisely where SmythOS enters the picture. This enterprise platform streamlines the complex process of building and managing knowledge representations through its comprehensive visual development environment.
At the core of SmythOS’s knowledge graph capabilities lies its visual builder interface, enabling developers and data scientists to construct intricate knowledge networks without diving deep into code. This intuitive approach accelerates development while maintaining the sophisticated functionality required for enterprise-grade knowledge management.
The platform’s built-in monitoring tools provide real-time insights into knowledge graph performance and health. Teams can track query patterns, identify bottlenecks, and optimize their knowledge representations through detailed analytics dashboards. This visibility ensures that knowledge graphs remain efficient and responsive as they scale.
Perhaps most notably, SmythOS breaks down traditional integration barriers through its enterprise-ready architecture. The platform seamlessly connects with existing IT infrastructure, supporting major graph databases and semantic technologies. This interoperability ensures that organizations can leverage their existing data investments while building more sophisticated knowledge representations.
Security remains paramount when handling enterprise knowledge bases, and SmythOS addresses this through robust security controls. The platform implements enterprise-grade protection measures to safeguard sensitive information within knowledge graphs, enabling confident deployment even in regulated environments.
For teams new to knowledge graph development, SmythOS offers a unique advantage through its free runtime environment. This allows organizations to prototype and test knowledge graph integrations without significant upfront investment, reducing the barriers to adoption while maintaining professional-grade capabilities.
The platform’s approach to knowledge graph development reflects a deep understanding of enterprise needs, combining powerful functionality with practical usability. Through visual workflows and intuitive debugging tools, SmythOS makes sophisticated knowledge representation accessible to broader technical teams while maintaining the depth required for complex use cases.
Future Directions in Knowledge Graph Technology
Knowledge graph technology is transforming how we understand and process complex data relationships. The integration of machine learning with semantic technologies offers new opportunities for more intuitive and powerful data systems.
At the enterprise level, organizations are shifting from traditional siloed databases to knowledge graphs, creating dynamic, interconnected information ecosystems. This change enables more nuanced context awareness in AI applications, leading to more accurate and meaningful insights.
The emergence of hybrid systems combining symbolic reasoning with neural networks is particularly exciting. These solutions bridge the gap between human-like reasoning and machine efficiency, potentially resolving challenges in natural language processing and automated decision-making.
Security and scalability remain critical focus areas. As knowledge graphs grow in complexity, ensuring data integrity while maintaining performance is crucial. Industry leaders are exploring innovative approaches to graph partitioning and distributed processing to address these challenges.
The future points toward more accessible implementations of knowledge graph technology. Whether for small businesses or large enterprises, democratizing these tools will likely accelerate innovation across sectors. Staying informed about these advancements is essential for organizations aiming to maintain their competitive edge in a data-driven world.
Last updated:
Disclaimer: The information presented in this article is for general informational purposes only and is provided as is. While we strive to keep the content up-to-date and accurate, we make no representations or warranties of any kind, express or implied, about the completeness, accuracy, reliability, suitability, or availability of the information contained in this article.
Any reliance you place on such information is strictly at your own risk. We reserve the right to make additions, deletions, or modifications to the contents of this article at any time without prior notice.
In no event will we be liable for any loss or damage including without limitation, indirect or consequential loss or damage, or any loss or damage whatsoever arising from loss of data, profits, or any other loss not specified herein arising out of, or in connection with, the use of this article.
Despite our best efforts, this article may contain oversights, errors, or omissions. If you notice any inaccuracies or have concerns about the content, please report them through our content feedback form. Your input helps us maintain the quality and reliability of our information.