Enhancing Research Outcomes through Human-AI Collaboration: Key Insights and Strategies
Imagine a world where artificial intelligence enhances our capabilities in remarkable ways. This reality is unfolding as research shows the biggest performance improvements happen when humans and smart machines work together.
The synergy between human intelligence and AI systems represents one of the most transformative developments in modern technology. Like a well-choreographed dance, humans and AI each bring unique strengths to the partnership – with AI handling data analysis and routine tasks while humans contribute creativity, judgment, and strategic thinking.
For developers and technical leaders building the next generation of AI systems, understanding this collaborative relationship is crucial. The future isn’t about AI versus humans, but rather how these technologies can augment human capabilities while preserving the irreplaceable human elements of innovation and decision-making.
Throughout this exploration of human-AI collaboration, we’ll uncover how organizations are achieving remarkable results by combining human intuition with AI’s processing power. We’ll examine real-world examples of successful partnerships between humans and AI systems, analyze the challenges teams face in implementation, and look ahead to emerging opportunities in this rapidly evolving field.
Companies see the biggest performance gains when humans and smart machines collaborate. People are needed to train machines, explain their outputs, and ensure their responsible use. AI, in turn, can enhance humans’ cognitive skills and creativity, free workers from low-level tasks, and extend their physical capabilities.
Harvard Business Review
Benefits of Human-AI Collaboration
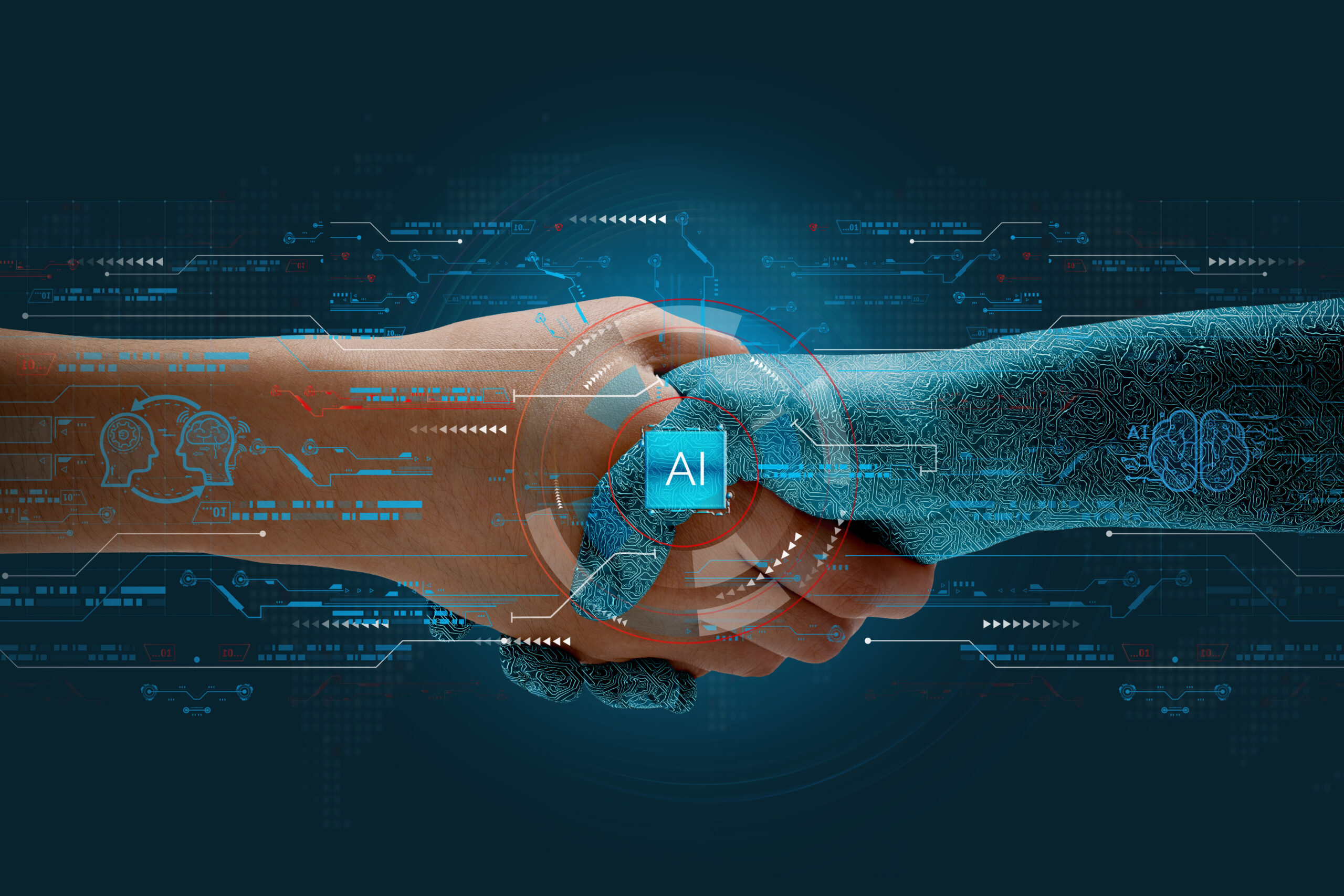
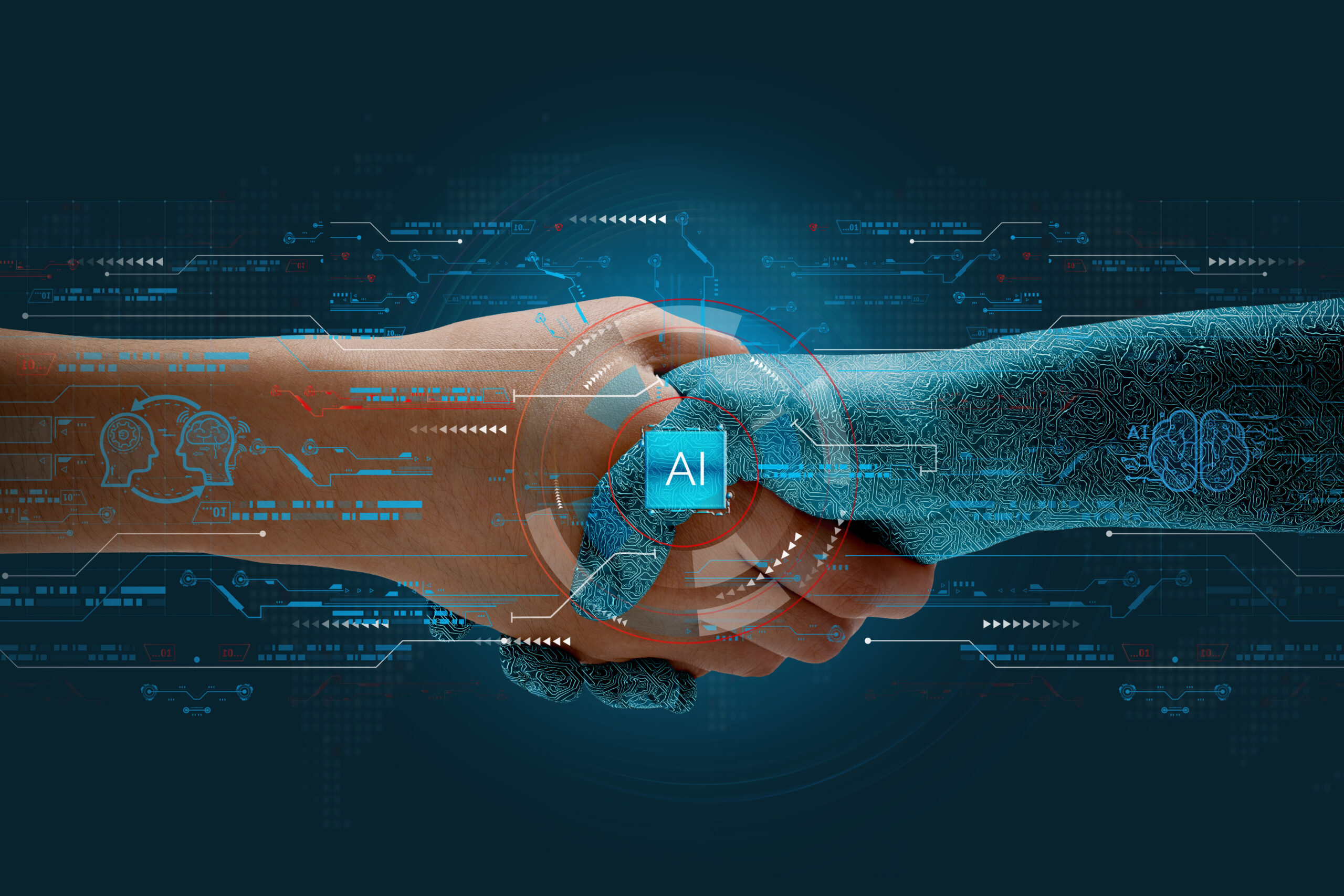
Artificial intelligence isn’t replacing human expertise – it’s dramatically enhancing it. Much like how calculators empowered financial professionals to focus on complex analysis rather than basic computations, AI is amplifying human capabilities across industries in unprecedented ways.
In healthcare, AI serves as an invaluable partner to medical professionals, significantly improving diagnostic accuracy and patient care. For instance, AI systems excel at analyzing medical images with remarkable precision, enabling radiologists to detect diseases earlier and with greater confidence. Rather than replacing doctors, AI augments their decision-making capabilities, allowing them to focus on nuanced patient care and complex treatment planning.
The financial sector demonstrates another powerful example of human-AI synergy. While AI processes vast amounts of real-time market data to identify patterns and trends, human financial analysts apply their judgment, emotional intelligence, and strategic thinking to craft personalized investment strategies. This collaboration enhances both efficiency and decision quality in ways neither humans nor machines could achieve alone.
Education is experiencing a similar transformation through human-AI collaboration. AI-powered tools can analyze student performance patterns and customize learning pathways, but it’s the human teachers who provide the emotional support, contextual understanding, and creative instruction that makes learning meaningful. Together, they create a more effective and personalized educational experience.
The true power of human-AI collaboration lies in its ability to enhance our uniquely human strengths. While AI handles data processing and routine tasks with unprecedented speed and accuracy, humans excel at creative problem-solving, emotional intelligence, and ethical decision-making. This complementary relationship drives innovation by freeing professionals to focus on higher-value activities that require human ingenuity.
Trust is a crucial factor influencing interactions between human beings, including their interactions with AI. Understanding the trust dynamics between AI and humans is crucial, particularly in the field of healthcare, where life is at risk.
Journal of Medical Internet Research
Perhaps most importantly, successful human-AI collaboration requires finding the right balance. Organizations that thoughtfully integrate AI capabilities while preserving human judgment and creativity are seeing remarkable improvements in both efficiency and innovation. The key isn’t to replace human intelligence, but to augment it in ways that enhance our natural capabilities and drive meaningful progress across industries.
Challenges in Human-AI Collaboration
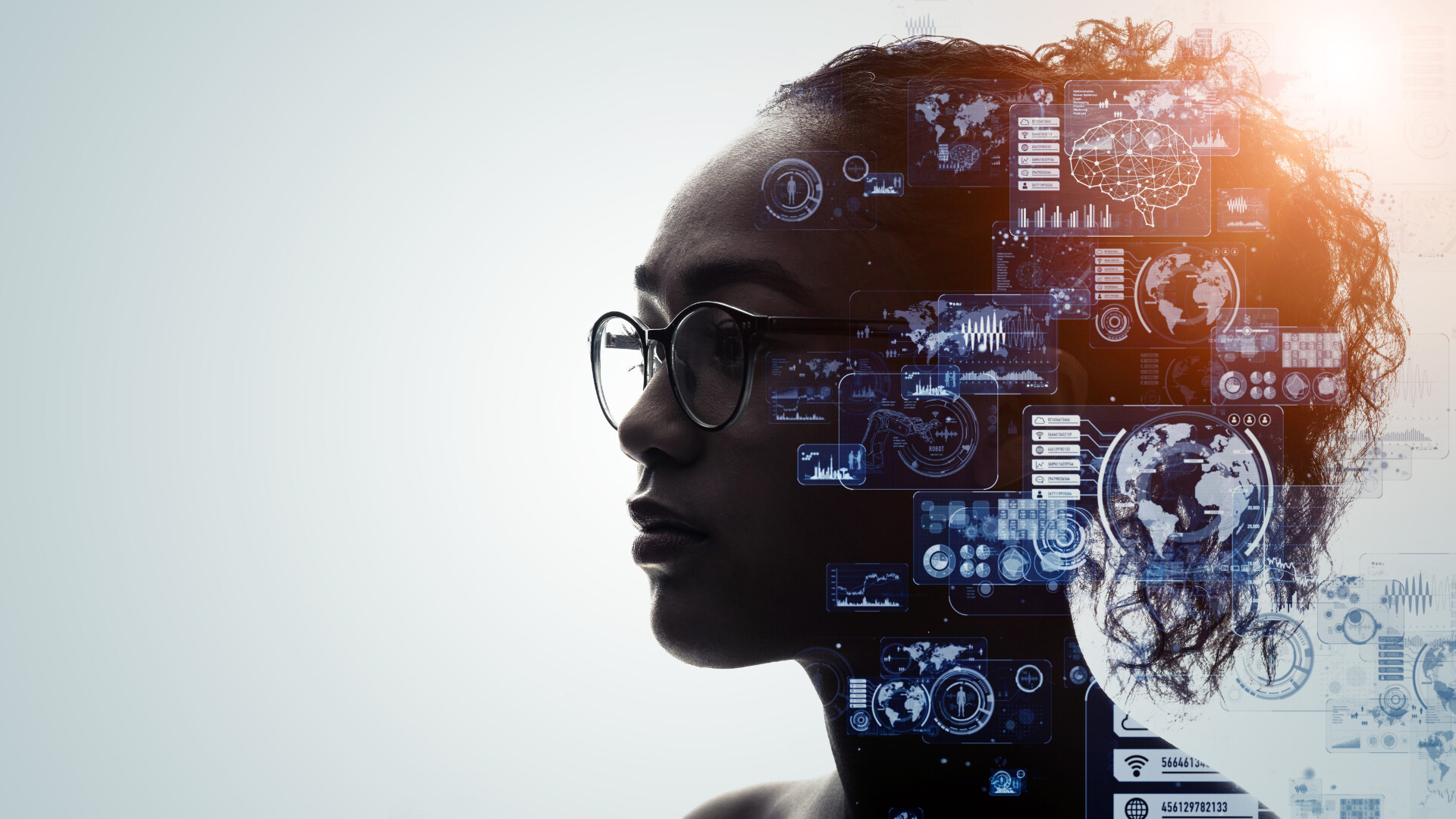
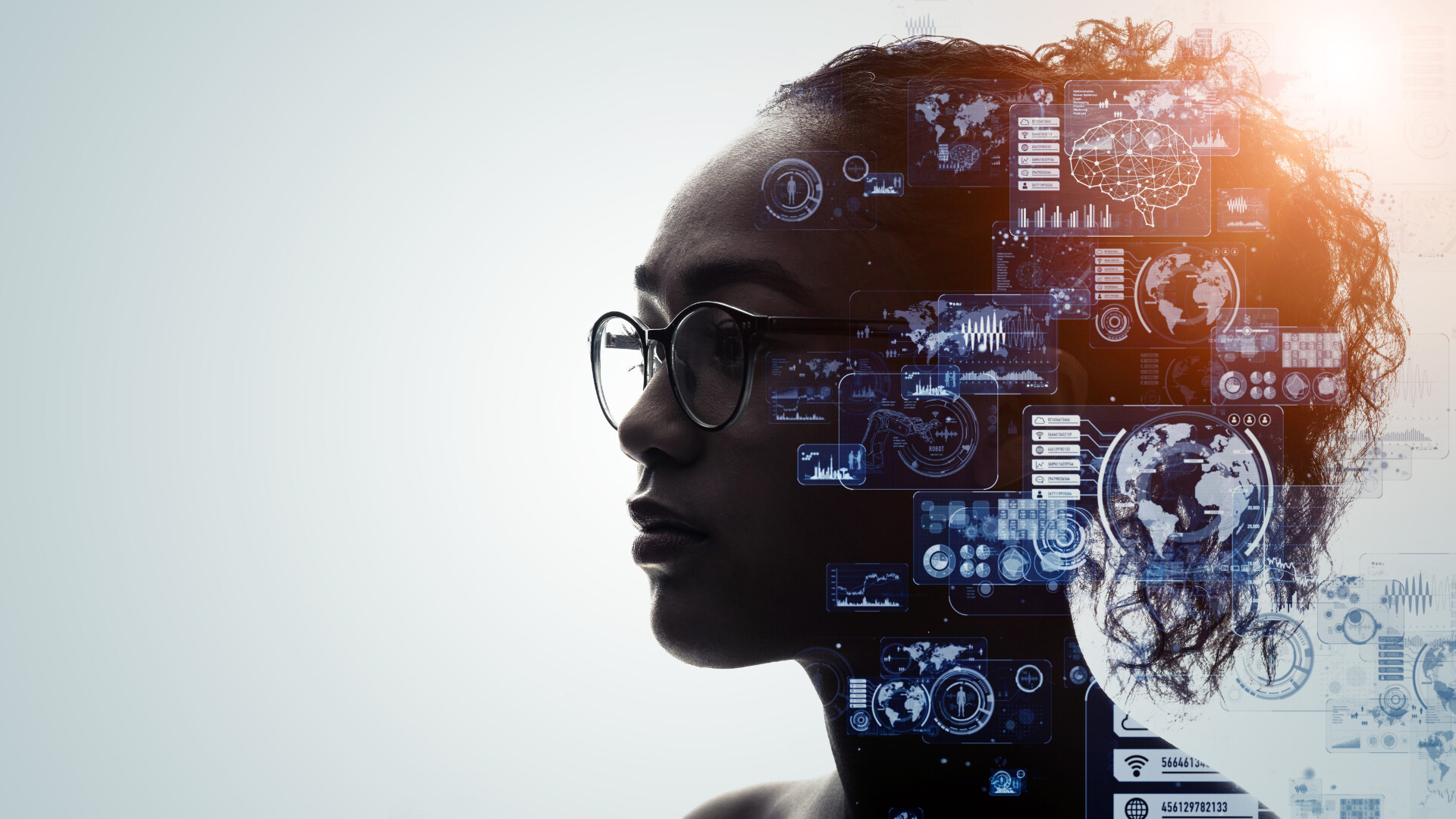
A contemplative person with digital data overlays. – Via denisonconsulting.com
Trust emerges as a critical hurdle in human-AI partnerships. According to the National Institute of Standards and Technology (NIST), the challenge extends beyond technical capabilities to broader societal factors that influence how humans interact with AI systems. When AI makes decisions affecting admissions, loans, or hiring, the stakes for trust become particularly high.
Cognitive barriers pose another significant challenge. Users often struggle to form accurate mental models of AI systems, leading to either over-reliance or unnecessary skepticism. This misalignment between human expectations and AI capabilities can result in costly mistakes or missed opportunities for effective collaboration. For instance, operators may trust AI systems too readily in high-pressure situations, even when showing signs of malfunction.
Interoperability issues create friction in human-AI workflows. While AI systems excel at processing vast amounts of data, they often struggle to seamlessly integrate with human decision-making processes. The challenge lies not just in technical compatibility but in creating interfaces that support natural, intuitive interaction while maintaining operational effectiveness.
Bias represents perhaps the most pervasive challenge in human-AI collaboration. AI systems can inadvertently perpetuate or amplify existing societal biases through their training data and algorithms. Research indicates this isn’t just a data problem – bias manifests in the broader context of how AI systems are developed, deployed, and used in real-world scenarios.
The speed and complexity of AI systems present unique challenges for human oversight. AI can process information and make decisions at rates far exceeding human cognitive capabilities, creating a fundamental tension in collaborative scenarios. This speed differential makes it difficult for humans to maintain meaningful control and understanding of AI-driven processes, potentially leading to errors or unintended consequences.
Key Research Areas in Human-AI Collaboration
The frontier of human-AI collaboration spans several cutting-edge research domains that are reshaping how we think about machine intelligence. At the heart of this evolution lies the critical shift from viewing AI systems as mere tools to understanding them as collaborative partners capable of meaningful interaction with human users.
One of the most significant research trajectories focuses on what researchers call the “transition from interaction to human-AI collaboration.” As highlighted in recent studies, AI systems are increasingly being developed to function not just as assistive tools but as intelligent teammates that can actively participate in collaborative problem-solving scenarios. This represents a fundamental shift in how we approach human-AI partnerships.
The emerging field of hybrid intelligence has become another crucial area of investigation. Rather than pursuing AI development in isolation, researchers are exploring ways to combine human and machine intelligence synergistically. This approach acknowledges that while machines excel at processing vast amounts of data and identifying patterns, humans possess unique cognitive capabilities that remain difficult to replicate artificially.
A particularly fascinating research direction centers on AI explainability and transparency. The infamous “AI black box” problem – where AI systems make decisions that are difficult for humans to understand – has spawned an entire subfield dedicated to making AI systems more interpretable. This work is essential for building trust and enabling meaningful collaboration between humans and AI systems.
Interdisciplinary approaches have emerged as a cornerstone of advancing human-AI collaboration. Modern research teams increasingly combine expertise from computer science, psychology, human factors engineering, and social sciences. This cross-pollination of ideas has proven crucial for developing AI systems that can effectively understand and adapt to human needs while maintaining high technical performance.
We need to enhance current processes for developing AI systems by incorporating HCI processes and methods, such as iterative prototyping and UX testing, as well as enhancing current software verification/validation methodology by effectively managing evolving machine behavior in AI systems.
Wei Xu and Marvin Dainoff, Enabling Human-Centered AI
The ethical dimensions of human-AI collaboration have also become a central research focus. Scientists are actively working to develop frameworks that ensure AI systems not only perform effectively but also operate within established ethical boundaries. This includes studying how to prevent algorithmic bias, maintain human autonomy, and ensure transparency in decision-making processes.
Evaluating Human-AI Collaboration
Human-AI collaboration across industries necessitates measuring these partnerships’ effectiveness. A comprehensive evaluation framework combines quantitative performance metrics and qualitative human factors to paint a complete picture of collaborative success.
At the quantitative level, key metrics focus on measurable outcomes. For example, research has shown that metrics like task completion time, error rates, and prediction accuracy help assess the technical performance of human-AI teams. A medical diagnosis system might track diagnostic accuracy rates and the time saved compared to manual review, providing concrete data on efficiency gains.
However, numbers only tell part of the story. Qualitative metrics examine the human experience of collaboration through factors like trust, communication clarity, and user satisfaction. Consider a financial analyst working with an AI fraud detection system—while accuracy rates matter, the analyst’s confidence in understanding and appropriately questioning the AI’s decisions is equally crucial for effective collaboration.
Adaptability serves as another vital metric, measuring how well both human and AI partners adjust their approach based on feedback and changing conditions. For instance, in manufacturing settings, teams track how effectively AI systems modify their behavior based on worker input while also assessing how smoothly human operators adapt to the AI’s capabilities.
Metric | Description |
---|---|
Task Completion Time | Measures the time taken to complete tasks when humans and AI collaborate compared to when done manually. |
Error Rates | Quantifies the frequency of errors in task execution by human-AI teams versus individual performance. |
Prediction Accuracy | Assesses the correctness of AI’s predictions and decisions in collaborative tasks. |
Trust | Evaluates the level of confidence humans have in AI systems during collaboration. |
Communication Clarity | Measures how effectively the AI system can communicate its processes and decisions to human collaborators. |
User Satisfaction | Gauges the overall satisfaction of human users with the AI collaboration process. |
Adaptability | Evaluates how well both human and AI partners adjust their approaches based on feedback and changing conditions. |
System Reliability | Measures the consistency and dependability of the AI system in collaborative scenarios. |
Regular evaluation should examine both immediate performance indicators and longer-term collaborative health metrics. Short-term measures might include daily productivity gains, while longitudinal assessment tracks evolving trust levels, skill development, and process refinements over time.
The most effective evaluation frameworks take a balanced approach, recognizing that successful human-AI collaboration requires both technical excellence and human-centric design. As research indicates, organizations should measure not just what the partnership achieves, but how smoothly and sustainably it operates.
Beyond individual metrics, evaluators must consider the broader collaborative context—including organizational culture, workflow integration, and evolving capability requirements. This holistic view helps ensure that human-AI teams don’t just perform well in controlled testing, but thrive in real-world applications.
The field continues to refine best practices for evaluation as human-AI collaboration expands into new domains. Regular assessment using a thoughtfully designed combination of quantitative and qualitative metrics remains essential for optimizing these partnerships and realizing their full potential.
Future Directions in Human-AI Collaboration
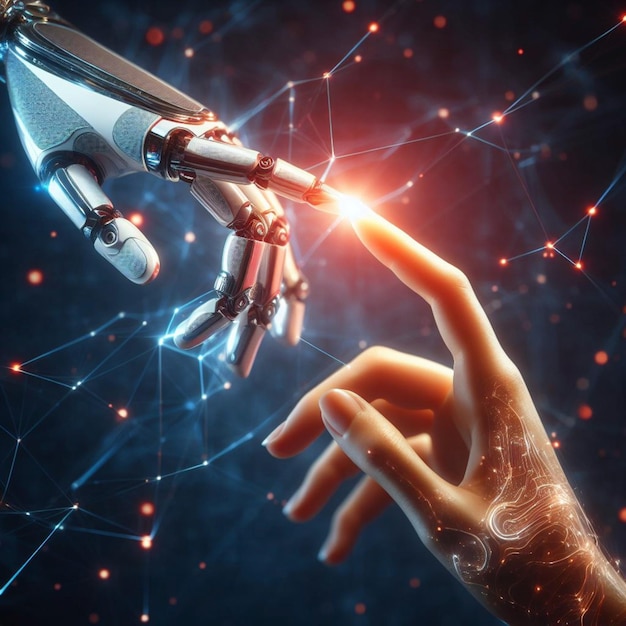
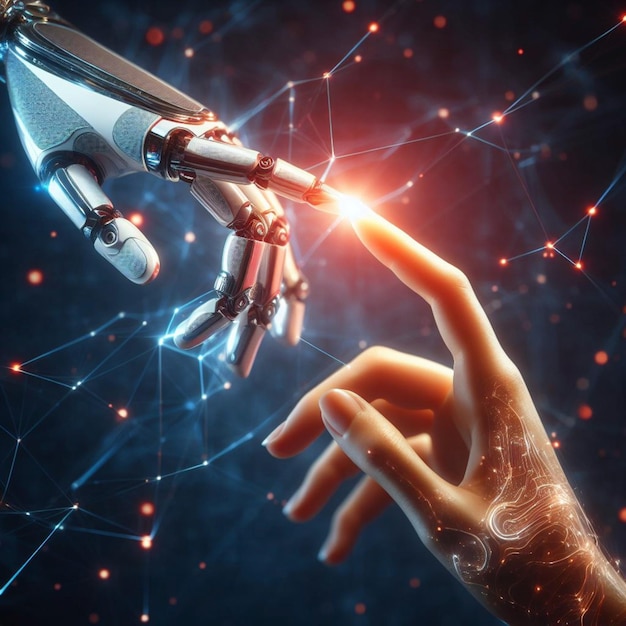
A robotic hand and human hand connect symbolizing collaboration. – Via freepik.com
The landscape of human-AI collaboration is transforming. The focus is on creating sophisticated partnerships between human intelligence and AI systems. The rapid advancement of AI technologies demands thoughtful integration that preserves human agency while maximizing collaborative potential.
Developing AI systems as true collaborative partners requires advances in AI’s ability to understand context, adapt to human needs, and engage in natural, bidirectional communication. Future systems need improved emotional intelligence and situational awareness, enabling them to provide nuanced and contextually appropriate support to human counterparts.
Effective human-AI teams leverage the strengths of both human and artificial intelligence while overcoming individual limitations. This involves developing AI systems that augment human capabilities without undermining autonomy or decision-making authority. Successful implementation requires transparency, trust-building, and clear communication channels between human and AI team members.
Addressing critical challenges includes developing robust frameworks for AI transparency and accountability. Future systems must clearly explain their reasoning processes, allowing human teammates to understand and validate AI-generated insights and recommendations. This transparency is essential for building trust and ensuring effective collaboration in high-stakes environments.
The future of human-AI collaboration requires a shift in system design approach. Rather than focusing solely on technological capabilities, successful implementation demands attention to human factors, ethical considerations, and team interaction dynamics. This holistic approach ensures that advances in AI technology enhance human capabilities rather than diminish or replace them.
Last updated:
Disclaimer: The information presented in this article is for general informational purposes only and is provided as is. While we strive to keep the content up-to-date and accurate, we make no representations or warranties of any kind, express or implied, about the completeness, accuracy, reliability, suitability, or availability of the information contained in this article.
Any reliance you place on such information is strictly at your own risk. We reserve the right to make additions, deletions, or modifications to the contents of this article at any time without prior notice.
In no event will we be liable for any loss or damage including without limitation, indirect or consequential loss or damage, or any loss or damage whatsoever arising from loss of data, profits, or any other loss not specified herein arising out of, or in connection with, the use of this article.
Despite our best efforts, this article may contain oversights, errors, or omissions. If you notice any inaccuracies or have concerns about the content, please report them through our content feedback form. Your input helps us maintain the quality and reliability of our information.