Transforming Manufacturing with Human-AI Collaboration: Boosting Efficiency and Innovation
Picture a manufacturing floor where robots and humans work in perfect harmony—not as competitors, but as collaborative partners, each bringing their unique strengths to the production line. This is the reality of modern smart manufacturing, where artificial intelligence and human ingenuity combine to create unprecedented levels of efficiency and innovation.
The synergy between human operators and AI systems is transforming how complex manufacturing tasks are handled. While AI-powered systems excel at processing vast amounts of data, maintaining consistency, and performing repetitive tasks with precision, human workers contribute critical thinking, adaptability, and creative problem-solving abilities that machines simply cannot replicate.
The impact of this collaboration is remarkable. Production lines that integrate human-AI partnerships are seeing dramatic improvements in quality control, with AI systems detecting microscopic defects while human operators make nuanced judgments about complex issues. Cycle times are being slashed as smart systems optimize workflows while human workers focus on high-value decision-making and oversight.
What makes this partnership particularly powerful is its ability to evolve and adapt. Unlike traditional automation, modern human-AI collaboration creates a learning loop where both parties continuously enhance each other’s capabilities. Human insights train AI systems to be more intelligent, while AI analytics help workers make better-informed decisions on the factory floor.
Perhaps the most exciting aspect of this technological revolution isn’t about replacing human workers—it’s about augmenting their capabilities and creating more engaging, safer work environments. By handling hazardous or physically demanding tasks, AI systems allow human workers to focus on more rewarding aspects of production, fundamentally transforming manufacturing from a purely mechanical process into an intelligent, collaborative endeavor.
Key Benefits of Human-AI Collaboration
The fusion of human intelligence with artificial intelligence creates a powerful synergy in modern manufacturing environments. When humans and AI systems work together, production efficiency soars through the combination of human judgment and AI’s tireless analytical capabilities. The numbers speak for themselves—manufacturers implementing collaborative AI solutions have seen up to 30-45% productivity gains in their operations.
Enhanced product quality emerges as another crucial advantage of human-AI partnerships. While AI systems excel at detecting microscopic defects and deviations through computer vision and sensor analysis, human workers provide the contextual understanding and creative problem-solving needed to address complex quality issues. This complementary approach leads to remarkably lower defect rates and consistent quality standards across production runs.
Manufacturing flexibility receives a major boost when humans and AI collaborate effectively. AI’s ability to rapidly analyze changing conditions and suggest process adjustments, combined with human operators’ expertise in implementing these changes, allows manufacturing facilities to swiftly adapt to new product requirements or market demands. This agility in production helps companies stay competitive in an increasingly dynamic marketplace.
The synergistic relationship between human workers and AI systems also drives innovation across the manufacturing sector. AI can process vast amounts of production data to identify optimization opportunities, while human insight helps translate these findings into practical improvements. This continuous cycle of analysis and refinement pushes the boundaries of what’s possible in modern manufacturing.
Worker safety and job satisfaction see notable improvements through human-AI collaboration as well. AI systems can handle hazardous or repetitive tasks, freeing human workers to focus on more engaging, strategic activities that require creativity and complex decision-making. This redistribution of work creates a more sustainable and fulfilling manufacturing environment while maintaining high productivity standards.
By combining human expertise with AI capabilities, manufacturers can achieve unprecedented levels of efficiency, quality, and flexibility in their operations, ultimately driving competitive advantage in the industry.
Quality Control Specialist at Siemens
Challenges in Human-AI Collaborative Systems
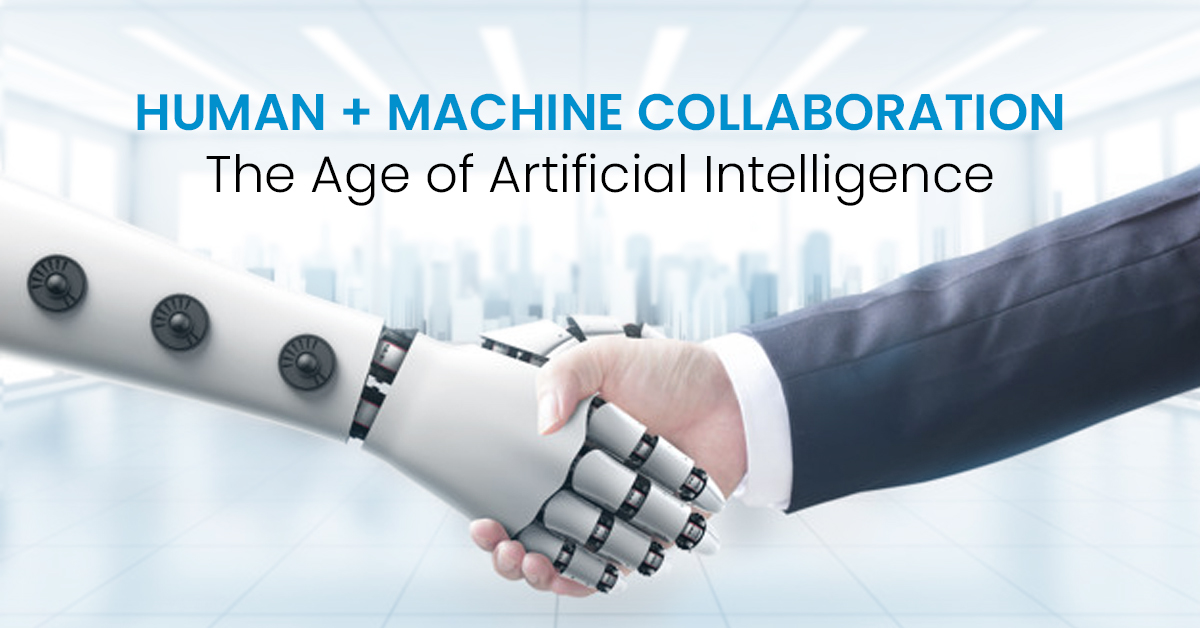
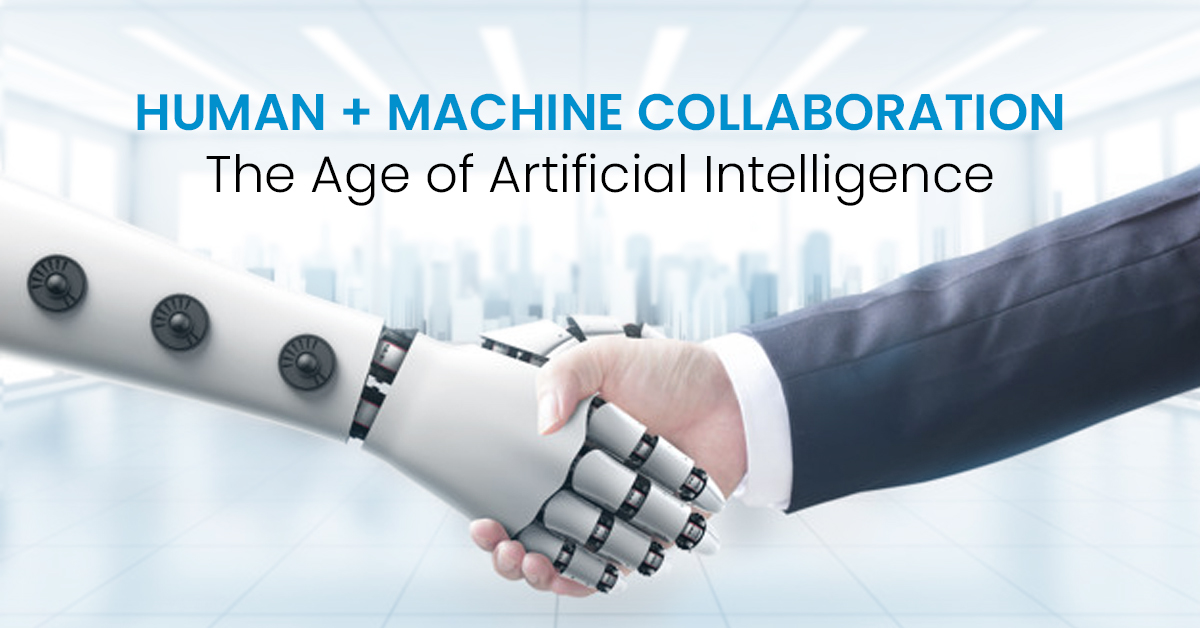
Integrating artificial intelligence with human workflows in manufacturing environments presents complex technical hurdles. Legacy systems and modern AI solutions often speak different languages, creating compatibility issues that can disrupt production processes and impact operational efficiency.
Data bias is a particularly thorny challenge when implementing AI systems. According to research from the National Institute of Standards and Technology (NIST), bias manifests not only in algorithms and training data but also in broader societal contexts that influence technology development. Manufacturing AI systems may inadvertently perpetuate historical biases present in their training data, potentially leading to skewed decision-making in quality control and resource allocation.
Technical integration challenges extend beyond software compatibility. Manufacturing facilities must connect sensors, coordinate robotic systems, and ensure real-time data flow between human operators and AI systems. When these systems fail to communicate effectively, it can lead to production delays and safety concerns.
The human element adds another layer of complexity. Workers must adapt to new interfaces and workflows while maintaining productivity. This adaptation period often reveals gaps in training programs and highlights the need for more intuitive human-machine interfaces that can bridge the technical knowledge gap.
Successful implementation requires unprecedented levels of interdisciplinary collaboration. Engineers, data scientists, human factors experts, and factory floor workers must work together to create seamless operations. This collaboration itself presents challenges in communication and coordination across different domains of expertise.
Context is everything. AI systems do not operate in isolation. They help people make decisions that directly affect other people’s lives.
Reva Schwartz, NIST Principal Investigator for AI bias
Organizations must also contend with maintaining transparency in AI decision-making processes. When AI systems make recommendations or automated decisions, operators and managers need to understand the reasoning behind these choices to maintain trust and ensure accountability in the manufacturing process.
Case Study: Human-AI Collaboration in Quality Control
Manufacturing quality control has entered a new era of precision and efficiency through the powerful combination of human expertise and artificial intelligence. By integrating AI-powered predictive analytics with human oversight, manufacturers are achieving unprecedented levels of accuracy in detecting and preventing defects before they impact production.
A prime example comes from high-precision manufacturing industries like aerospace and medical devices, where even microscopic defects can have serious consequences. AI systems analyze vast amounts of sensor data in real-time to identify subtle patterns and anomalies that might escape human detection, while skilled quality control specialists provide crucial context and decision-making oversight.
The results of this human-AI partnership have been remarkable. According to industry research, manufacturers implementing AI-augmented quality control have seen defect rates drop by up to 50% while significantly reducing the time required for inspections. The AI handles the rapid processing of complex data, enabling human inspectors to focus their expertise on investigating root causes and implementing process improvements.
Manufacturer | Reduction in Defect Rates | Reduction in Inspection Time |
---|---|---|
Car Seat Manufacturer | 30% | From 1 minute to 2.2 seconds per seat |
Automotive Manufacturer | 25% | 40% |
Intel | 30% | 25% improvement in yield rates |
Textile Manufacturer | 37% increase in defect detection rate | 45% reduction in manual inspection time |
Consider a leading automotive manufacturer that deployed an AI quality control system to work alongside their inspection team. The AI monitors thousands of data points per second from sensors throughout the production line, instantly flagging potential issues for human review. This proactive approach allows the quality team to address problems before they result in defective products, maintaining consistently high standards while reducing waste and rework costs.
Predictive analytics has transformed our quality control process from reactive to proactive. Our AI system catches subtle variations that could indicate future defects, while our experienced team members provide the judgment and insight needed to implement effective solutions.
Beyond just identifying defects, this collaborative approach enables continuous improvement of manufacturing processes. The AI system learns from each inspection, becoming increasingly accurate at predicting potential quality issues. Meanwhile, human operators provide invaluable feedback that helps refine the AI’s algorithms and ensure they remain aligned with real-world manufacturing conditions and quality standards.
Future Directions in Human-AI Manufacturing
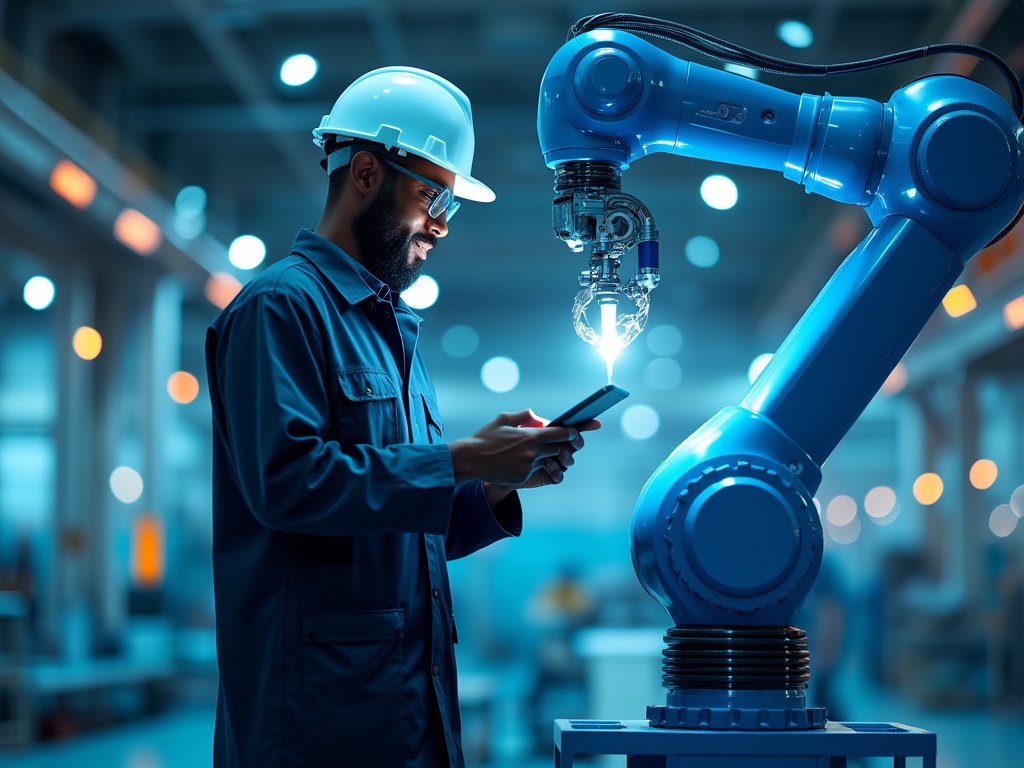
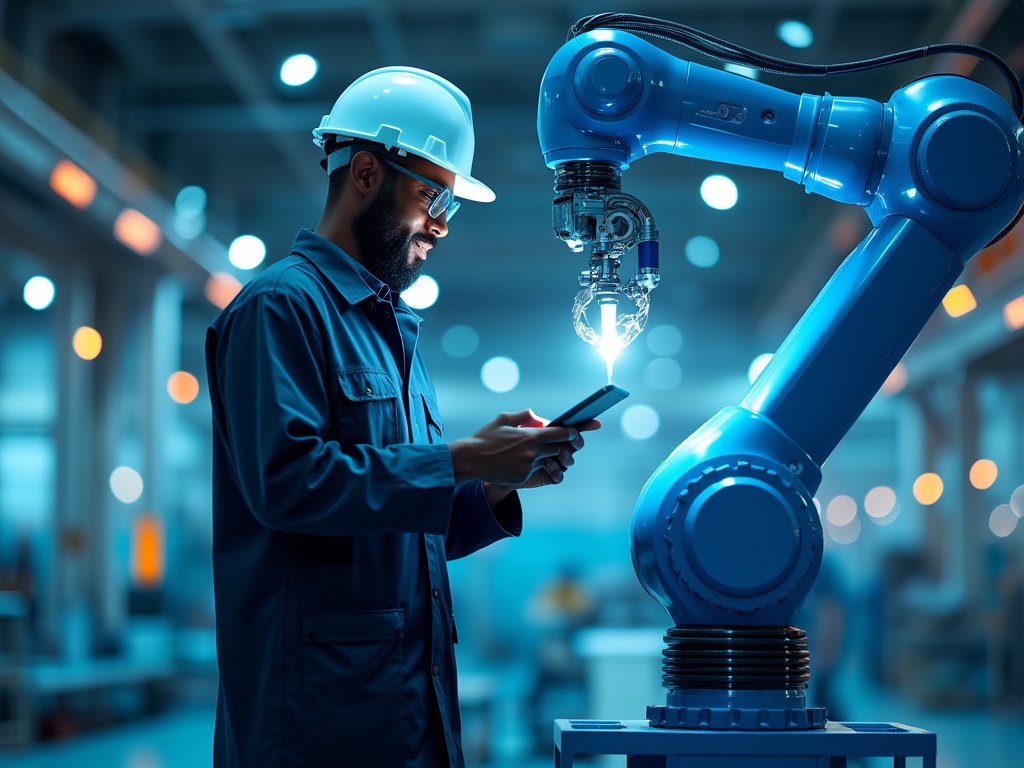
Engineer and robotic arm in a high-tech setting.
The manufacturing landscape is undergoing a significant transformation, where the convergence of human expertise and artificial intelligence promises unprecedented efficiency and innovation. Recent developments in collaborative robotics and AI integration are reshaping traditional manufacturing paradigms, creating smarter, more responsive production environments.
At the forefront of this evolution is the advancement in predictive maintenance capabilities. Modern AI systems can now analyze vast amounts of sensor data to forecast equipment failures weeks or even months in advance, dramatically reducing costly downtime and optimizing maintenance schedules. This proactive approach enables manufacturers to maintain peak operational efficiency while minimizing disruptions.
The integration of edge computing with AI systems is emerging as a game-changing development in manufacturing operations. By processing data closer to its source, manufacturers can achieve near-instantaneous analysis and decision-making capabilities, essential for real-time production adjustments and quality control. This technological synergy is particularly crucial in high-precision manufacturing environments where split-second decisions can impact product quality and operational efficiency.
Enhanced collaboration techniques between humans and AI systems are also taking center stage. Modern manufacturing facilities are implementing sophisticated interfaces that enable seamless interaction between workers and AI-powered systems. These interfaces leverage advanced visualization tools and intuitive controls, allowing operators to monitor, adjust, and optimize production processes with unprecedented precision.
The role of AI in decision-making processes is expanding beyond basic automation. Machine learning algorithms are now capable of analyzing complex production scenarios, suggesting optimizations, and even adapting to changing conditions in real-time. This cognitive flexibility offers manufacturers the agility needed to respond to market demands and production challenges quickly.
AI-driven predictive maintenance represents a significant leap forward in industrial efficiency. By harnessing the power of AI, companies can not only prevent costly equipment failures but also optimize their entire maintenance strategy.
Blog.siemens.com, 2024
Strategy | Key Features | Benefits | Challenges |
---|---|---|---|
Traditional Maintenance | Reactive, fixes issues after failure | Simplicity, lower initial costs | Unpredictable downtime, higher long-term costs |
AI-driven Predictive Maintenance | Proactive, uses AI to forecast failures | Reduced downtime, cost savings, extended asset lifespan | High initial setup cost, data integration complexity |
AI-driven Prescriptive Maintenance | Recommends actions to prevent failures | Optimized interventions, maximized machine uptime | Technical challenges, need for accurate model training |
Conclusion and Future Insights
The manufacturing landscape is evolving as human expertise and artificial intelligence converge, creating new possibilities. McKinsey & Company reports that AI-driven predictive maintenance can boost equipment availability by 20% and reduce inspection costs by 25%. These statistics highlight the transformative potential of human-AI collaboration in manufacturing.
Forward-thinking manufacturers are finding success by creating synergistic partnerships rather than replacing human workers. Integrating AI with human capabilities has shown remarkable results, particularly in quality control and process optimization. AI handles repetitive tasks, allowing humans to focus on strategic decision-making and innovation.
SmythOS plays a crucial role in this transformation, offering a platform that bridges the gap between human expertise and AI capabilities. Its visual builder and intuitive workflow design tools empower manufacturers to implement sophisticated AI solutions without sacrificing human-driven innovation and problem-solving.
Looking to the future, manufacturing excellence will increasingly depend on blending human insight with AI’s analytical prowess. The continuous advancement of AI technologies, coupled with platforms like SmythOS that facilitate seamless integration, points to a future where productivity and innovation are the norm.
The manufacturing sector is ready to embrace this new era of human-machine collaboration. Those who adapt to this paradigm shift, leveraging platforms for smooth integration and efficient workflows, will lead industrial innovation and drive productivity improvements that reshape the manufacturing landscape for years to come.
Last updated:
Disclaimer: The information presented in this article is for general informational purposes only and is provided as is. While we strive to keep the content up-to-date and accurate, we make no representations or warranties of any kind, express or implied, about the completeness, accuracy, reliability, suitability, or availability of the information contained in this article.
Any reliance you place on such information is strictly at your own risk. We reserve the right to make additions, deletions, or modifications to the contents of this article at any time without prior notice.
In no event will we be liable for any loss or damage including without limitation, indirect or consequential loss or damage, or any loss or damage whatsoever arising from loss of data, profits, or any other loss not specified herein arising out of, or in connection with, the use of this article.
Despite our best efforts, this article may contain oversights, errors, or omissions. If you notice any inaccuracies or have concerns about the content, please report them through our content feedback form. Your input helps us maintain the quality and reliability of our information.