Enhancing Decision-Making with Human-AI Collaboration: A Smarter Approach
Imagine a world where human intuition and artificial intelligence work in perfect harmony. Critical decisions are made through a partnership between human expertise and AI’s analytical capabilities. This isn’t science fiction—it’s the emerging reality of human-AI collaboration in decision-making.
According to groundbreaking research published in Nature Human Behaviour, the relationship between humans and AI is more nuanced than previously thought. While the combination doesn’t always outperform either humans or AI working independently, there are specific domains where this collaboration truly shines, particularly in creative and complex problem-solving tasks.
Organizations today face significant challenges in integrating AI into their decision-making processes. From healthcare providers using AI for diagnoses to financial institutions leveraging algorithms for risk assessment, the stakes are high. Success isn’t just about implementing the latest technology—it’s about understanding how humans and machines can complement each other’s strengths while mitigating their weaknesses.
This article will explore three critical aspects of human-AI collaboration: the integration challenges organizations must overcome, effective strategies for mitigating cognitive and algorithmic biases, and the vital role of interdisciplinary teamwork in building successful AI systems. We’ll dive into real-world examples, examine current research findings, and provide practical insights for organizations looking to harness this powerful partnership.
As Microsoft’s corporate vice president Gurdeep Pall notes, It’s essential that employees believe in the capabilities of their AI counterparts
. This trust, combined with proper implementation and understanding, forms the foundation of effective human-AI collaboration in decision-making.
Integration Challenges in Human-AI Collaboration
Organizations face complex hurdles when incorporating AI systems into their decision-making frameworks. While AI promises enhanced efficiency and innovation, the integration process requires navigating both technical and human factors to ensure success.
Legacy system compatibility presents one of the most significant technical obstacles. According to recent research, organizations frequently encounter interoperability issues when connecting AI solutions with outdated technology, resulting in data silos and reduced efficiency.
The challenge of technical detachment emerges when AI systems operate in isolation from existing workflows. This disconnect can lead to fragmented processes where AI insights fail to integrate seamlessly with human decision-making channels. Organizations must bridge this gap by developing standardized protocols that enable smooth information flow between AI systems and human teams.
Human oversight and control mechanisms represent another critical integration challenge. As Heather Dawe, Head of Data at UST, points out:
The bias in data is trained into the models. And in some ways, the model can enhance this bias, which causes major challenges in the model appearing to be racist or sexist. It’s not as simple as removing sensitive features such as gender and race; even without them, models will internalize stereotypes.
Heather Dawe, Head of Data at UST
Scaling AI solutions presents another hurdle. As organizations grow, their AI systems must evolve to handle increasing data volumes and user demands. Without proper scalability planning, companies risk facing performance bottlenecks and decreased system responsiveness that can cripple decision-making processes.
Security concerns add another layer of complexity. Organizations must implement robust protection mechanisms for AI systems while ensuring they remain accessible to authorized decision-makers. This balance between security and accessibility often requires sophisticated access control systems and continuous monitoring protocols.
The skills gap poses a significant obstacle in achieving smooth integration. Many organizations lack personnel with the expertise to manage AI systems and interpret their outputs effectively. This shortage of qualified talent can lead to suboptimal use of AI capabilities and missed opportunities for improving decision-making processes.
Perhaps most crucially, organizations must maintain the delicate balance between automation and human judgment. While AI excels at processing vast amounts of data and identifying patterns, human intuition and experience remain essential for contextual understanding and ethical considerations in decision-making.
Mitigating Biases from AI Systems
AI systems are only as fair as the data they learn from. When training data lacks diversity or contains hidden prejudices, AI models can perpetuate and even amplify societal biases, leading to discriminatory outcomes that affect real people’s lives. Understanding how to identify and address these biases is crucial for developing ethical AI systems.
A real-world example illustrates the impact of biased training data. In one notable case, an AI-powered facial recognition system showed significantly lower accuracy rates for certain demographic groups because those groups were underrepresented in the training dataset. This type of bias can have serious consequences when these systems are used in critical applications like security or healthcare.
Diversifying Training Data Sources
Creating representative training datasets requires intentional effort to include diverse perspectives and experiences. Organizations should actively seek out data from varied sources that reflect different demographics, cultures, and contexts. This means going beyond convenient or readily available data sources to ensure comprehensive representation.
Data augmentation techniques can help expand the diversity of existing datasets. This might involve applying transformations to create additional examples while preserving important characteristics. For instance, in image recognition tasks, variations in lighting, angle, and scale can help the model learn more robust features.
Cross-validation across different demographic groups is essential to verify that the model performs consistently well for all populations. If performance metrics show significant disparities between groups, this signals a need to rebalance the training data or adjust the model architecture.
Evaluating Datasets for Bias
Statistical analysis tools can help identify potential biases in training data before they affect model behavior. Key metrics include demographic parity (checking if outcomes are independent of protected attributes) and equalized odds (ensuring similar error rates across groups).
Regular audits of both training data and model predictions help catch bias issues early. This involves examining not just overall accuracy, but also analyzing performance across different subgroups and edge cases. Documentation of data sources, collection methods, and potential limitations provides transparency and aids in identifying possible sources of bias.
The goal is not just to avoid bias, but to actively promote fairness and inclusion in AI systems. This requires ongoing vigilance and a commitment to improvement.
Dr. Bob McGrew, AI Research Officer
Engaging domain experts and diverse stakeholders in the data evaluation process brings valuable perspectives that technical metrics alone might miss. Their insights can help identify subtle forms of bias and suggest ways to make datasets more representative.
Success in mitigating AI bias requires a holistic approach that combines technical solutions with ethical considerations. By making conscious choices about training data and implementing robust evaluation procedures, we can work toward AI systems that serve all users fairly and effectively.
Interdisciplinary Collaboration for Effective Decision-Making
The fusion of diverse expertise and perspectives is essential for successfully integrating artificial intelligence into real-world applications. As organizations increasingly adopt AI technologies, bringing together professionals from computer science, domain experts, social scientists, and ethicists creates a more comprehensive approach to development and implementation.
Recent research from the AI & Society Journal demonstrates that cross-disciplinary teams are better equipped to address the complex challenges of AI integration. When data scientists collaborate with industry specialists and ethicists, they can better identify potential biases, understand practical limitations, and ensure AI systems align with human values and organizational goals.
The effectiveness of interdisciplinary collaboration stems from each field’s unique contribution. Computer scientists bring technical expertise in algorithm development, while social scientists provide insights into human behavior and societal impact. Domain experts contribute practical knowledge of implementation challenges, and ethicists ensure responsible development aligned with moral principles.
Discipline | Role | Contribution |
---|---|---|
Computer Science | Technical Expertise | Algorithm development, system architecture, data processing |
Social Science | Human Behavior Insight | Understanding societal impacts, human-AI interaction dynamics |
Domain Experts | Practical Knowledge | Implementation challenges, real-world applicability, domain-specific requirements |
Ethicists | Ethical Oversight | Ensuring responsible AI development, aligning with moral principles |
Communication plays a pivotal role in bridging different disciplinary perspectives. Successful teams establish common vocabulary and frameworks that enable meaningful dialogue across specialties. This shared understanding helps unify diverse goals into cohesive project objectives while maintaining respect for each discipline’s distinct value.
Cross-disciplinary collaboration in AI development isn’t just beneficial – it’s absolutely essential for creating systems that are both technically sound and socially responsible.
International Conference on Human-AI Collaboration, 2023
Organizations that embrace interdisciplinary approaches often see improved outcomes in their AI initiatives. These collaborative efforts lead to more robust solutions that consider technical feasibility, practical implementation, societal impact, and ethical implications from the outset rather than as afterthoughts.
Continuous Monitoring and Feedback Loops
Continuous monitoring and user feedback are crucial in refining AI decision-making systems. Research shows that comprehensive monitoring during training, testing, and inference stages provides deep insights into AI system behavior and performance.
Real-world deployment of AI systems requires vigilant tracking of multiple performance indicators. By analyzing model outputs, feature behaviors, and contextual metadata, organizations can detect potential issues before they impact critical operations. This proactive approach helps maintain system reliability while identifying opportunities for enhancement.
User feedback is invaluable for improving AI systems. When users interact with AI decision-making tools, their experiences highlight gaps between intended and actual system performance. This feedback enables developers to fine-tune models, adjust parameters, and enhance the overall user experience.
Feedback loops create an iterative improvement cycle. As users provide input on system decisions and recommendations, development teams can address shortcomings, validate changes, and deploy refined versions. This continuous refinement process helps AI systems evolve alongside user needs and expectations.
KPI Category | Key Performance Indicator | Description |
---|---|---|
Operational Efficiency | Task Completion Time | Measures the speed at which AI completes tasks. |
Operational Efficiency | Resource Utilization | Tracks the amount of computational resources used by AI systems. |
Customer Satisfaction | Customer Satisfaction Score | Assesses the level of satisfaction among customers interacting with AI systems. |
Customer Satisfaction | Engagement Rate | Measures how often customers engage with AI-driven services. |
Revenue Growth | Revenue Increase | Tracks the increase in revenue attributed to AI initiatives. |
Revenue Growth | Conversion Rate | Measures the rate at which AI-driven interactions convert into sales. |
Performance Metrics | Accuracy | Quantifies how accurately the AI system performs its tasks. |
Performance Metrics | Precision | Measures the proportion of true positive results among all positive results. |
Performance Metrics | Recall | Measures the proportion of true positive results among all actual positives. |
Performance Metrics | F1 Score | Harmonic mean of precision and recall. |
Data Integrity | Data Quality | Ensures the input data is accurate and consistent. |
Data Integrity | Data Consistency | Tracks the uniformity of data used by AI systems. |
Model Drift | Model Performance Over Time | Monitors changes in model performance to detect degradation. |
Model Drift | Retraining Frequency | Tracks how often the AI model is retrained to maintain performance. |
Explainability and Transparency | Model Explainability | Measures how well the AI model’s decisions can be understood by humans. |
Explainability and Transparency | Decision Transparency | Tracks the clarity of the AI system’s decision-making process. |
Security and Privacy | Security Incidents | Monitors the number of security breaches related to AI systems. |
Security and Privacy | Compliance Rate | Measures adherence to data privacy regulations. |
Regular assessment of both quantitative metrics and qualitative feedback ensures AI systems remain aligned with their intended purpose. Organizations must establish clear monitoring frameworks that track technical performance while incorporating user perspectives. This balanced approach leads to more effective and trustworthy AI decision-making tools.
Success in AI implementation requires moving beyond simple deployment to embrace ongoing optimization. By combining rigorous monitoring practices with active user feedback channels, organizations can build more robust and reliable AI systems that truly serve their users’ needs while maintaining high standards of performance and accuracy.
Leveraging SmythOS for Enhanced Collaboration
SmythOS transforms human-AI collaboration through its comprehensive suite of built-in monitoring capabilities, providing unprecedented visibility into agent behaviors and interaction patterns. The platform’s sophisticated monitoring system tracks real-time performance metrics, message exchange rates, and resource utilization—critical data points that ensure optimal collaboration between human operators and AI agents.
At the heart of SmythOS lies its innovative visual debugging environment, which revolutionizes how teams interact with autonomous agents. Unlike traditional platforms that require diving into complex logs, SmythOS presents agent interactions in an intuitive, visual format that both technical and non-technical team members can easily understand. This democratization of AI development enables broader participation in building and refining collaborative AI solutions.
The platform’s extensive API integration capabilities represent another cornerstone of enhanced collaboration. SmythOS seamlessly connects with over 300,000 apps, APIs, and data sources, enabling AI agents to access and share information across different systems without complicated setup procedures. This robust interoperability ensures effective collaboration regardless of the agents’ roles or the systems they interact with.
Security remains paramount in SmythOS’s collaborative framework, with enterprise-grade controls protecting all agent interactions. As noted by Alexander De Ridder, Co-Founder and CTO of SmythOS, the platform excels at creating AI agents that integrate seamlessly with existing enterprise systems while maintaining robust security protocols. This comprehensive protection addresses a critical concern in modern AI systems, particularly for organizations handling sensitive data.
Beyond basic functionality, SmythOS employs advanced event-triggered actions that allow agents to respond dynamically to changes in their environment. This sophisticated orchestration ensures that agent-human collaboration remains contextual and purposeful, leading to more efficient outcomes. The platform’s ability to combine any AI model, tool, workflow, and data source into a cohesive system creates a powerful foundation for building complex, interoperable agent networks that enhance human capabilities rather than replace them.
SmythOS is not just a tool; it’s a paradigm shift in AI development. It empowers a new generation of developers to create AI solutions that were once the domain of tech giants.
The impact of SmythOS on human-AI collaboration extends beyond traditional automation. By providing sophisticated monitoring tools, seamless integration capabilities, and robust security features, the platform creates an environment where humans and AI agents can work together effectively, each leveraging their unique strengths to achieve superior results. This synergy represents the future of work—where human creativity and AI capabilities combine to drive innovation and productivity.
Future Directions in Human-AI Collaboration
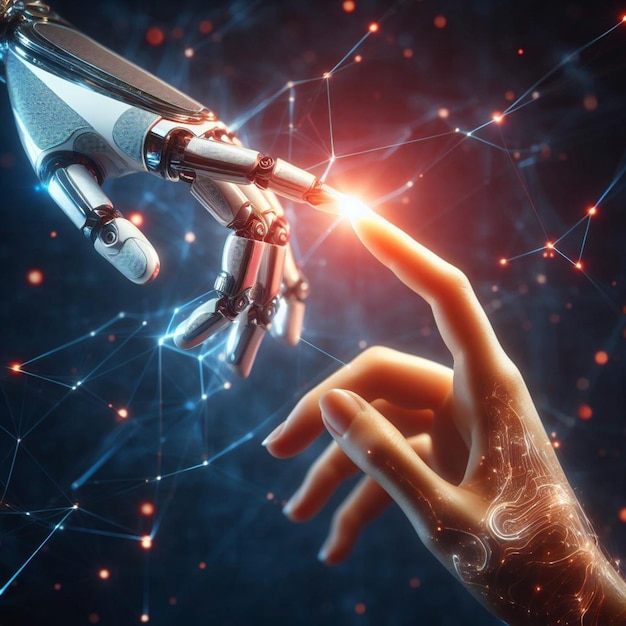
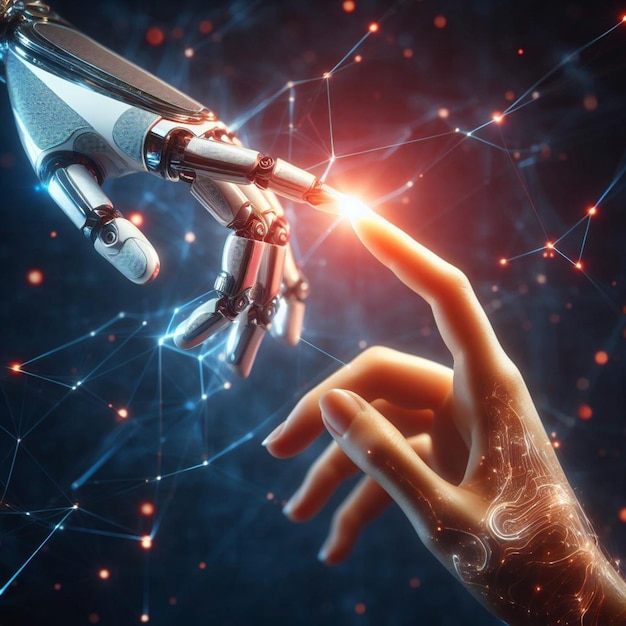
The trajectory of human-AI collaboration stands at a pivotal crossroads. As seen through initiatives like IBM’s AI advancement programs, the next decade promises significant changes in how humans and machines work together to solve complex challenges.
Quantum computing is a game-changing force in this evolution. By drastically reducing the computational resources needed for AI training and operation, quantum technologies will enable more sophisticated and responsive AI systems that can process information across multiple states simultaneously, moving beyond traditional binary limitations.
The integration of multimodal AI represents another transformative shift. Future systems will seamlessly blend text, voice, visual, and sensory inputs to create more natural and intuitive human-AI interactions. This advancement will particularly benefit fields like healthcare, where comprehensive data analysis and real-time decision support can significantly improve patient outcomes.
Ethics and governance frameworks must evolve in parallel with these technological developments. Establishing robust regulatory standards, transparent AI decision-making processes, and mechanisms for accountability will be crucial in building trust and ensuring fair collaboration between humans and AI systems.
Energy sustainability presents both a challenge and opportunity. While current AI systems consume substantial power, innovations in quantum computing and efficient chip designs promise to dramatically reduce energy requirements. This evolution is essential for scaling AI applications across industries while maintaining environmental responsibility.
The future of AI lies not in replacing human intelligence, but in creating symbiotic relationships where machines enhance human capabilities while preserving human agency and creativity.
Bernard Marr, AI and Future Technologies Expert
As we move forward, the focus must shift from mere automation to genuine collaboration. This means developing AI systems that can adapt to human needs, learn from feedback, and maintain appropriate levels of autonomy while respecting human oversight. Success in this endeavor will require continued investment in research, cross-disciplinary collaboration, and a commitment to ethical innovation.
Last updated:
Disclaimer: The information presented in this article is for general informational purposes only and is provided as is. While we strive to keep the content up-to-date and accurate, we make no representations or warranties of any kind, express or implied, about the completeness, accuracy, reliability, suitability, or availability of the information contained in this article.
Any reliance you place on such information is strictly at your own risk. We reserve the right to make additions, deletions, or modifications to the contents of this article at any time without prior notice.
In no event will we be liable for any loss or damage including without limitation, indirect or consequential loss or damage, or any loss or damage whatsoever arising from loss of data, profits, or any other loss not specified herein arising out of, or in connection with, the use of this article.
Despite our best efforts, this article may contain oversights, errors, or omissions. If you notice any inaccuracies or have concerns about the content, please report them through our content feedback form. Your input helps us maintain the quality and reliability of our information.