Advancing Agriculture with Human-AI Collaboration: A Path to Sustainable Farming
Picture a farm where artificial intelligence and human expertise work in perfect harmony—drones scanning vast fields while farmers interpret the data to make crucial decisions. This powerful partnership between human intelligence and AI technology is transforming agriculture in ways previously confined to science fiction.
As recent research highlights, the integration of AI with traditional farming knowledge is transforming everything from crop management to resource optimization. By combining AI’s analytical capabilities with farmers’ generations of expertise, modern agriculture is achieving unprecedented levels of precision and productivity.
The synergy between human insight and machine intelligence is particularly evident in crop management, where AI assists rather than replaces human decision-making. While AI algorithms process vast amounts of data from soil sensors and satellite imagery, it’s the farmer’s intimate understanding of their land that turns this information into actionable strategies.
This technological revolution isn’t just about boosting yields—it’s about creating sustainable farming practices that can feed a growing world population while preserving our planet’s resources. Through this collaboration, farmers are discovering smarter ways to reduce water usage, minimize chemical inputs, and protect soil health.
We will explore how the partnership between humans and AI is transforming modern farming practices. We will examine real-world applications that showcase this transformative potential, address the current challenges that must be overcome, and look ahead to future developments that promise even greater advancements in agricultural productivity and sustainability.
Current Applications of AI in Agriculture
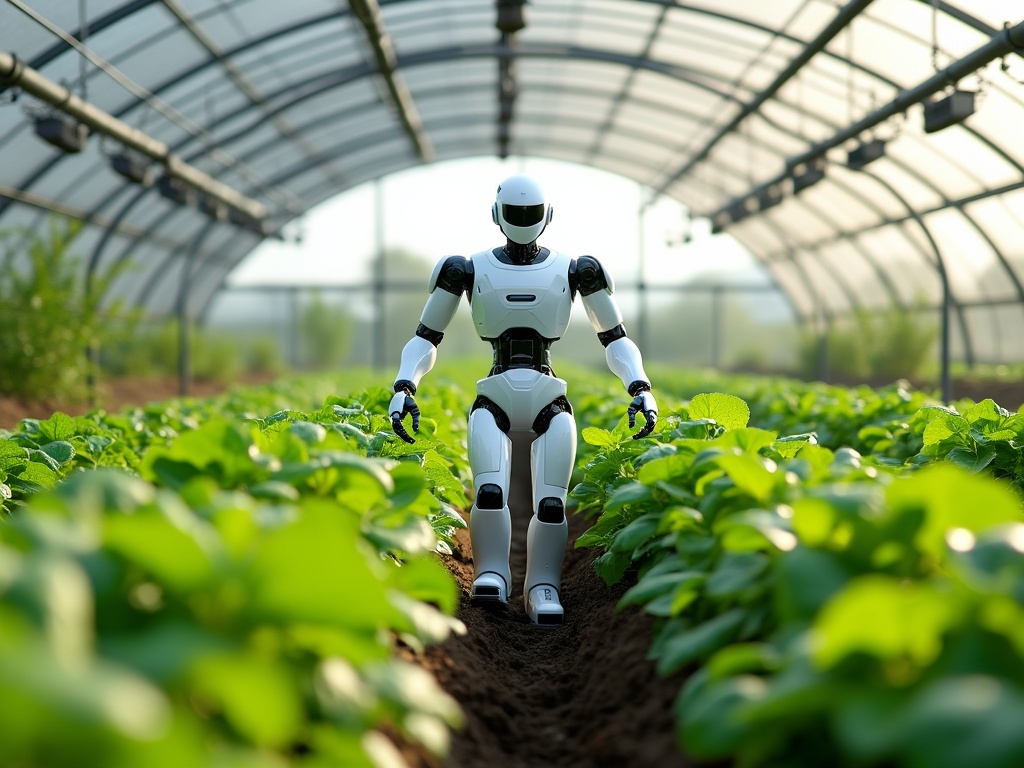
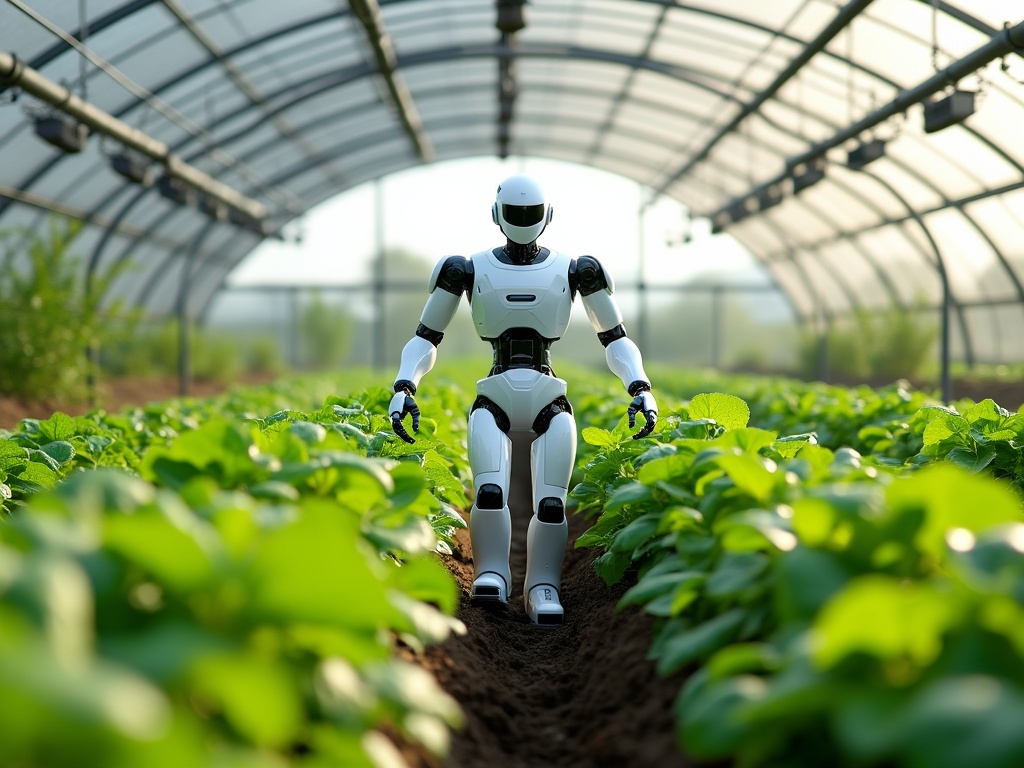
Artificial intelligence is transforming traditional farming into a high-precision, data-driven industry. Modern farmers are increasingly relying on AI-powered systems to make smarter decisions about every aspect of their operations, from planting to harvest.
Precision farming, one of AI’s most impactful agricultural applications, uses sophisticated algorithms to analyze data from multiple sources like soil sensors, satellite imagery, and weather stations. These AI systems enable farmers to make data-driven decisions that optimize resource allocation while minimizing environmental impact. Rather than applying uniform amounts of water and fertilizer across entire fields, farmers can precisely target specific areas based on their unique needs.
Benefit | Description |
---|---|
Increased Crop Yields | Optimized farming practices tailored to specific field conditions lead to faster plant growth and higher yields. |
Resource Efficiency | Precise management of water, fertilizers, and pesticides reduces waste and lowers input costs. |
Environmental Sustainability | Minimized use of chemicals and optimized inputs promote long-term environmental stewardship. |
Labor Cost Savings | Automation and reduced need for manual labor result in significant labor cost savings. |
Improved Decision-Making | Data-driven insights from IoT devices and sensors enable informed decisions regarding crop rotation, pest management, and resource allocation. |
In crop monitoring, AI and machine learning algorithms process vast amounts of data collected through sensors and drones. These systems can detect early signs of crop stress, disease, or pest infestation before they become visible to the human eye. This early warning capability allows farmers to take preventive action quickly, potentially saving entire harvests that might otherwise be lost to disease or pests.
Automated harvesting represents another frontier where AI is making significant strides. Computer vision systems, powered by deep learning algorithms, can now identify ripe produce and guide robotic harvesters with remarkable precision. These AI-driven harvesting systems work tirelessly, reducing labor costs while ensuring crops are picked at optimal ripeness to maximize quality and yield.
The integration of AI technologies with Internet of Things (IoT) devices has created powerful synergies in agricultural management. Real-time data analysis allows farmers to monitor crop health, soil conditions, and weather patterns continuously. This constant stream of information, processed through AI algorithms, helps farmers optimize irrigation schedules, predict maintenance needs for equipment, and forecast potential yield with unprecedented accuracy.
The integration of artificial intelligence and machine learning into crop management is transforming modern agriculture by enhancing efficiency, sustainability, and resilience
Danish Gul and Rizwan Ul Zama Banday, IntechOpen
These AI applications are not just improving efficiency; they’re fundamentally changing how we approach agriculture. By enabling more precise resource management and better decision-making, AI helps farmers produce more food while using fewer resources—a crucial advancement as we face the challenges of feeding a growing global population while preserving our environment.
Challenges in Human-AI Collaboration
The agricultural sector’s shift toward AI integration brings unprecedented opportunities, but significant hurdles remain in creating effective human-AI partnerships. Technical limitations pose immediate challenges, from unreliable internet connectivity in rural areas to hardware constraints that affect real-time data processing. These infrastructure gaps can lead to delayed responses and impaired decision-making when farmers need immediate insights.
Data bias emerges as another critical concern, particularly in agricultural applications. When AI systems train primarily on data from large-scale industrial farms, they often misrepresent the needs of smaller, diverse farming operations. A recent study highlighted how unclear accountability in AI decisions can erode user trust, especially when dealing with autonomous farm equipment and decision support systems.
Perhaps the most formidable barrier is the deep-rooted resistance to change within farming communities. Generations of agricultural knowledge and tried-and-true methods make many farmers skeptical of AI-driven approaches. This resistance isn’t merely about technology adoption; it reflects legitimate concerns about maintaining control over crucial farming decisions and protecting traditional agricultural wisdom.
Skill gaps further complicate the integration process. Many agricultural workers lack the technical expertise to effectively operate and maintain AI systems, while AI specialists often don’t fully grasp the nuances of agricultural practices. This knowledge divide can lead to implementation failures and missed opportunities for optimization.
Addressing these challenges requires a multi-faceted approach. Investment in rural digital infrastructure must accompany focused training programs that bridge the technical divide. AI systems need to be designed with input from diverse farming operations, ensuring their recommendations account for varying scales and methods of agriculture. Most importantly, developers must work to create transparent, explainable AI solutions that complement rather than replace human agricultural expertise.
Enhancing Crop Management with AI
Modern agriculture faces unprecedented challenges, from unpredictable weather patterns to increasing resource constraints. Artificial intelligence has emerged as a solution, transforming how farmers monitor and manage their crops. Through advanced predictive analytics and real-time monitoring systems, AI provides farmers with insights that were previously impossible to obtain.
AI-powered solutions have dramatically improved pest control efficiency through early detection and targeted interventions. Computer vision and deep learning algorithms can identify pest infestations before they become visible to the human eye, allowing farmers to take preventive measures precisely where needed. This targeted approach not only protects crops more effectively but also reduces the overall use of pesticides by 30-50 percent compared to traditional blanket spraying methods.
Precision irrigation represents another breakthrough in AI-driven crop management. Smart irrigation systems analyze real-time soil moisture data, weather forecasts, and crop water requirements to deliver exactly the right amount of water to each section of a field. This level of precision has led to water savings of up to 30% while improving crop yields through optimal moisture management.
AI has transformed fertilizer application from a rough estimate into a precise science. Through continuous soil monitoring and crop health analysis, AI systems can determine exactly when and where nutrients are needed. This optimization has resulted in both cost savings for farmers and reduced environmental impact from excess fertilizer runoff.
The integration of AI into agriculture has ushered in a new era of precision farming, offering significant potential to enhance productivity, sustainability, and resilience in the face of growing challenges such as climate change and food security.
While implementing AI systems requires initial investment, the returns in terms of improved productivity and resource efficiency make it an increasingly attractive option for farms of all sizes. From small family farms to large agricultural operations, AI tools are helping farmers make more informed decisions, reduce waste, and ultimately produce more food with fewer resources.
Future Directions in Human-AI Agricultural Collaboration
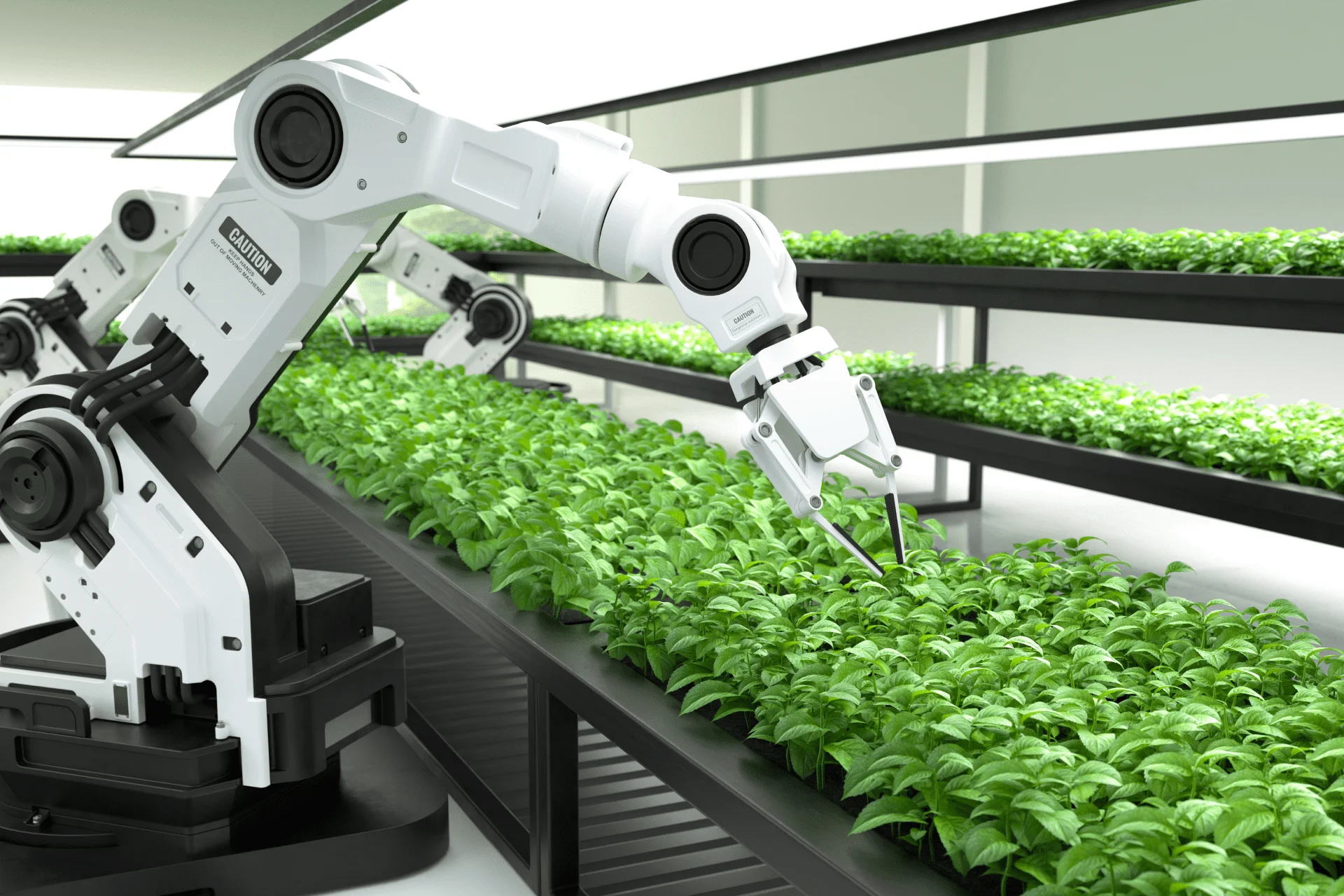
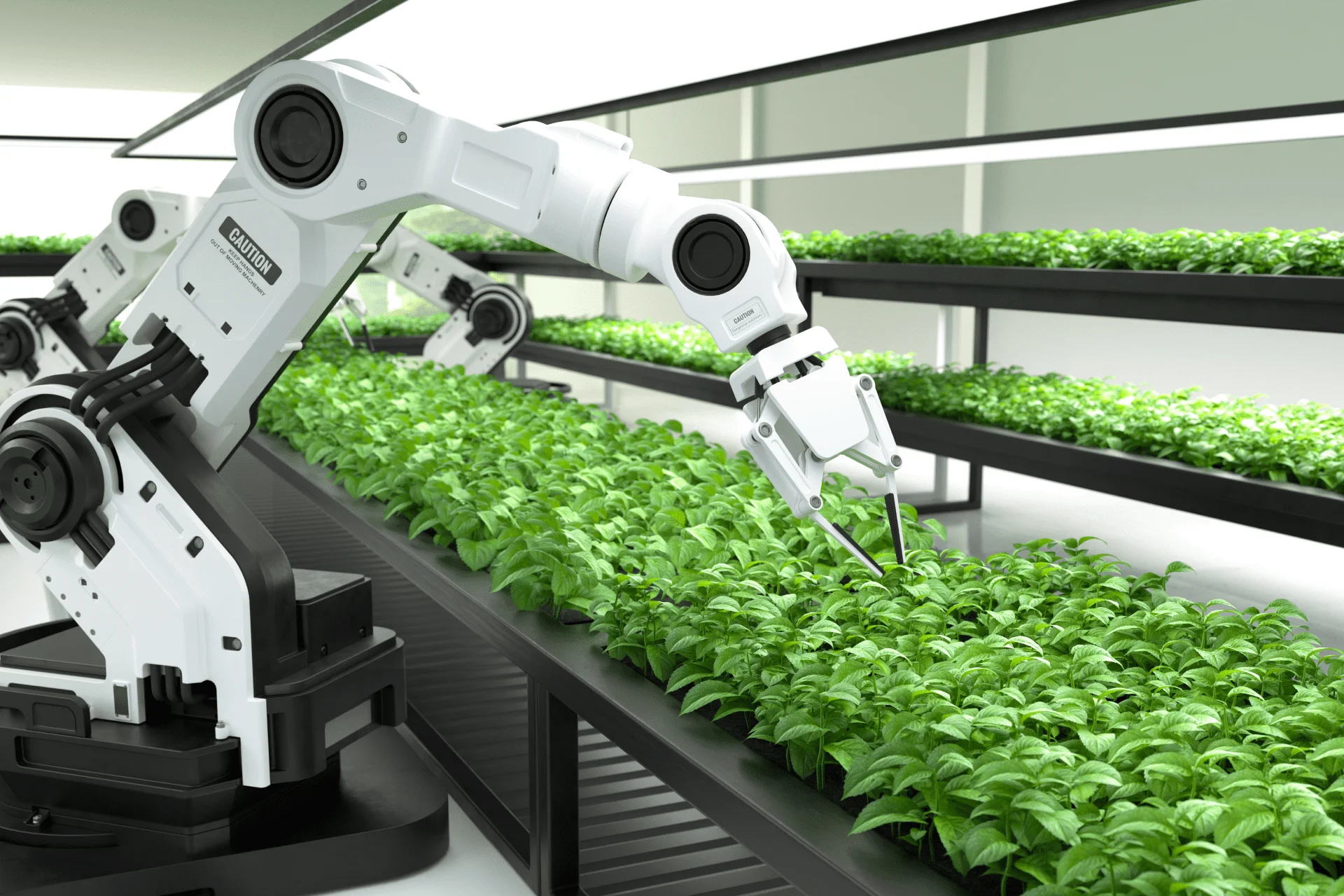
Robotic arms in agriculture enhancing plant care. – Via mindy-support.com
AI-powered systems are helping farmers achieve remarkable results, including a 21% increase in yields and a 9% reduction in pesticide use. These early successes suggest significant transformative potential in the future. Edge computing and sophisticated AI models are reshaping traditional farming practices. Modern agricultural AI systems can analyze vast amounts of field data in real time, encompassing factors like soil conditions and crop health indicators. This enables farmers to make precise, data-driven decisions, marking a shift from reactive to predictive farming approaches.
The integration of Internet of Things (IoT) devices with AI is creating an extensive network of agricultural intelligence. Smart sensors deployed across fields continuously monitor everything from moisture levels to pest presence, while AI algorithms process this data to generate actionable insights. Farmers now have access to detailed, real-time analytics that guide their decision-making.
These technologies are becoming increasingly accessible to farmers of all sizes. User-friendly interfaces and more affordable IoT solutions mean that advanced farming technology is no longer just for large industrial operations. Small-scale farmers can also leverage these tools to optimize their operations and compete more effectively in the market.
The increasing use of AI in agriculture has amplified these benefits. By leveraging AI and digital technology, farmers have achieved significant increases in yields and substantial reductions in pesticide use, while also boosting income per acre. Looking ahead, we can anticipate even deeper integration between human expertise and AI capabilities. Machine learning models will likely become more sophisticated at understanding local farming conditions, while improved IoT devices will offer increasingly detailed insights into crop and soil health.
This synergy between human knowledge and artificial intelligence promises to make farming more sustainable, productive, and resilient in the face of climate challenges. These technological advancements are also addressing critical sustainability issues. AI-driven precision farming techniques are reducing water waste, minimizing chemical usage, and optimizing resource allocation. This not only benefits the environment but also ensures the long-term viability of agricultural operations.
Leveraging SmythOS for Seamless Integration
Agricultural innovation demands powerful, reliable technology that can seamlessly unite various data streams and automated processes. SmythOS steps up to this challenge with a comprehensive platform specifically designed to transform complex agricultural operations into streamlined, intelligent workflows.
The platform’s intuitive visual workflow builder eliminates traditional coding barriers, enabling farmers and agricultural technologists to rapidly develop and deploy AI-driven solutions. Rather than wrestling with complicated code, users can drag and drop components to create sophisticated automation sequences that monitor crops, analyze soil conditions, and optimize resource usage.
SmythOS’s built-in monitoring capabilities provide unprecedented visibility into agricultural operations. From tracking irrigation systems to monitoring autonomous equipment, the platform offers real-time insights that help farmers make data-driven decisions. This constant oversight ensures that AI applications perform optimally while identifying potential issues before they impact crop yields.
Feature | Benefit |
---|---|
Universal Integration | Unifies disparate tools, data, and processes into a single digital ecosystem |
AI Collaboration | Enables employees to work alongside AI agents naturally |
Predictive Intelligence | Predicts factors such as market trends and internal changes |
Adaptive Learning | Adapts and evolves with the business |
Democratized Innovation | Empowers every employee to become an AI-supported problem solver |
What truly sets SmythOS apart is its extensive integration framework. The platform connects seamlessly with over 300,000 data sources and APIs, allowing agricultural operations to incorporate information from weather stations, soil sensors, satellite imagery, and other vital sources. This robust connectivity ensures that AI agents can access and analyze all relevant data to make informed decisions about crop management.
The platform’s enterprise-grade security controls provide peace of mind for agricultural operations handling sensitive data. Whether managing proprietary crop data or coordinating autonomous equipment, SmythOS maintains rigorous security standards while offering the flexibility needed for rapid development and deployment of agricultural solutions.
The blending of AI’s speed and scalability with human wisdom and oversight is how we manifest the best possible futures. Neither is sufficient alone.
For agricultural enterprises looking to embrace AI innovation, SmythOS offers a powerful yet accessible platform that simplifies the development and deployment of intelligent farming solutions. Its combination of visual development tools, comprehensive monitoring, and seamless integration capabilities positions it as a cornerstone technology for the future of smart agriculture.
Conclusion on Human-AI Collaboration in Agriculture
The integration of artificial intelligence in agriculture marks a pivotal transformation in food production and sustainability. Through sophisticated data analysis and automation, AI technologies optimize resource allocation, improve crop yields, and enable precise farming practices. As highlighted by the World Economic Forum’s AI for Agriculture Innovation initiative, farmers implementing AI solutions have achieved significant improvements, including 21% increases in yields and substantial reductions in pesticide use.
However, the success of agricultural AI systems depends on effective human-AI collaboration. While AI excels at processing vast amounts of data and identifying patterns, human expertise remains irreplaceable in interpreting results, making nuanced decisions, and understanding the complex interplay of local farming conditions. This partnership creates a synergy that amplifies the strengths of both.
Maximizing these collaborative benefits requires thoughtful integration that prioritizes both technological advancement and human empowerment. As farming operations become increasingly digitized, balancing automation with human oversight ensures AI serves as a tool for enhancement rather than replacement. This approach preserves the invaluable knowledge of experienced farmers and creates new opportunities for agricultural innovation.
Critical challenges remain in achieving seamless human-AI collaboration, including the need for comprehensive training, addressing technological literacy gaps, and ensuring equitable access to AI tools across different scales of farming operations. Yet, these challenges present opportunities for developing more inclusive and adaptable agricultural technologies that can benefit farmers worldwide.
The future of agriculture will likely be shaped by how effectively we foster collaboration between human expertise and artificial intelligence. By focusing on sustainable practices, ethical implementation, and continuous learning, the agricultural sector can harness the full potential of AI while preserving the essential human elements that have always been at the heart of farming.
Last updated:
Disclaimer: The information presented in this article is for general informational purposes only and is provided as is. While we strive to keep the content up-to-date and accurate, we make no representations or warranties of any kind, express or implied, about the completeness, accuracy, reliability, suitability, or availability of the information contained in this article.
Any reliance you place on such information is strictly at your own risk. We reserve the right to make additions, deletions, or modifications to the contents of this article at any time without prior notice.
In no event will we be liable for any loss or damage including without limitation, indirect or consequential loss or damage, or any loss or damage whatsoever arising from loss of data, profits, or any other loss not specified herein arising out of, or in connection with, the use of this article.
Despite our best efforts, this article may contain oversights, errors, or omissions. If you notice any inaccuracies or have concerns about the content, please report them through our content feedback form. Your input helps us maintain the quality and reliability of our information.