Exploring Future Trends in Human-AI Collaboration: What’s Next?
Imagine a workplace where humans and artificial intelligence systems complement each other in powerful ways. This reality is already unfolding across industries, reshaping how we work, create, and solve complex problems.
Recent research from MIT Sloan reveals an interesting insight: while human-AI teams may struggle with certain decision-making tasks, they excel at creative endeavors. This finding challenges our assumptions about artificial intelligence merely replacing human workers and points to a future of true collaboration.
The transformation is particularly evident in fields like healthcare, where AI assists medical professionals in diagnosis while leaving critical thinking and patient care to human expertise. In creative industries, AI handles routine tasks like data analysis and pattern recognition, freeing humans to focus on innovation and artistic expression.
This collaboration leverages the unique strengths of both parties. Humans bring emotional intelligence, contextual understanding, and creative problem-solving abilities that even the most advanced AI systems cannot replicate. Meanwhile, AI contributes unparalleled data processing capabilities, pattern recognition, and consistency in repetitive tasks.
In the coming years, new roles and workflows designed around this human-AI partnership will emerge. Organizations that embrace this collaborative approach, rather than viewing AI as a replacement for human workers, will likely find themselves at the forefront of innovation and productivity.
Enhancing Communication and Knowledge Exchange
Artificial intelligence has fundamentally transformed how humans and machines interact, ushering in unprecedented capabilities for knowledge sharing and communication. Recent research shows that AI systems are redefining traditional communication paradigms, moving beyond simple command-response patterns to more nuanced, context-aware interactions.
Today’s AI technologies demonstrate remarkable prowess in understanding and processing human language. Systems like ChatGPT not only comprehend complex queries but can engage in meaningful dialogue while providing detailed, contextually relevant responses. This advancement represents a significant leap forward in bridging the communication gap between humans and machines.
The evolution of natural language processing has enabled AI to serve as both communicator and mediator, facilitating more efficient knowledge exchange across different domains. Modern AI systems can analyze vast amounts of information, identify patterns, and present insights in accessible formats, making complex data comprehensible to users regardless of their technical expertise.
AI’s role has expanded from being merely a tool for communication to becoming an active participant in knowledge creation and exchange. These systems now engage in sophisticated tasks like automatic summarization, translation between languages, and even generating creative content, fundamentally changing how we access and share information.
Looking ahead, the integration of AI in communication platforms promises even greater possibilities. Multimodal AI systems that combine text, voice, and visual processing capabilities are emerging, offering more natural and intuitive ways for humans to interact with machines. This evolution suggests a future where AI becomes an increasingly seamless part of our daily communications, enhancing both personal and professional knowledge exchange.
Through the rapid growth of AI and AI-powered machines, the role of machines changed from mediators of communication between humans to communicators and partners of humans in human-machine communication.
One particularly promising development is the advancement in AI’s ability to understand context and nuance in human communication. Unlike earlier systems that relied on rigid, predefined responses, modern AI can adapt its communication style based on the user’s needs, preferences, and level of expertise, making knowledge exchange more effective and personalized.
The Role of Metalearning Capacities
Meta-learning represents a fundamental shift in how artificial intelligence systems acquire and apply knowledge. Unlike traditional machine learning approaches that focus on mastering specific tasks, meta-learning empowers AI systems with the ability to learn how to learn – a capability that proves crucial for human-AI collaboration.
Meta-learning enables AI systems to adapt rapidly to new situations by drawing from their previous learning experiences. Just as humans leverage past knowledge to tackle unfamiliar challenges, meta-learning algorithms develop sophisticated learning strategies that improve with each new task they encounter. This adaptive capacity is particularly valuable when AI systems need to work alongside humans in dynamic, ever-changing environments.
The power of meta-learning becomes evident through its practical applications. For instance, in healthcare settings, a meta-learning system can quickly adapt its diagnostic capabilities across different medical imaging technologies or patient demographics without requiring extensive retraining. This flexibility allows healthcare professionals to collaborate more effectively with AI tools across various clinical contexts.
Meta-learning’s primary aim is to provide machines with the skill to learn how to learn.
Meta-learning is particularly powerful for human-AI collaboration due to its ability to handle limited data scenarios. When faced with new tasks, meta-learning systems can achieve high performance using just a few examples, mirroring how humans can quickly grasp new concepts without extensive training. This capability proves invaluable in professional settings where data may be scarce or expensive to obtain.
The adaptability of meta-learning systems also addresses a key challenge in human-AI collaboration: the need for AI to understand and adjust to different human working styles and preferences. Through meta-learning, AI systems can develop more nuanced approaches to interaction, learning from each engagement to improve future collaborations. This continuous adaptation helps create more natural and effective partnerships between human operators and AI systems.
Cognitive and Emotional Intelligence Integration
Throughout the pandemic, many envisioned having an AI-powered virtual therapist that could genuinely empathize and understand moods and behaviors. This vision highlights a crucial development in artificial intelligence: the integration of cognitive and emotional intelligence capabilities. Today, this fusion is essential for creating truly intuitive human-AI collaborations.
AI systems with enhanced emotional intelligence can recognize, interpret, and respond appropriately to human emotions, creating more meaningful interactions. As highlighted in recent research, these systems can potentially transform behavioral health services by providing personalized therapeutic interventions and enhancing patient care through emotionally intelligent responses.
The integration of cognitive capabilities with emotional understanding enables AI systems to process complex information while considering the emotional context. This combination is particularly valuable in healthcare settings, where both clinical accuracy and emotional sensitivity are crucial. For instance, AI systems can analyze patient data while accounting for emotional states, leading to more comprehensive and nuanced care recommendations.
However, this integration faces significant challenges. The subjective nature of emotions makes them difficult to quantify and program into AI systems. Additionally, there are valid concerns about privacy, bias, and the ethical implications of AI systems interpreting human emotions. As one study notes, there’s often a lack of consensus on defining emotions and challenges in generalizing emotional expressions across different cultures and contexts.
Challenges | Benefits |
---|---|
Ethical concerns | Enhanced user engagement |
Bias in data | Improved personalized support |
Lack of standardized metrics | Better therapeutic interventions |
Privacy issues | Increased overall effectiveness of human-AI interactions |
Difficulty in quantifying emotions | More meaningful interactions |
Despite these challenges, the benefits of integrating cognitive and emotional intelligence in AI systems are compelling. These systems can provide more personalized support, enhance user engagement, and improve the overall effectiveness of human-AI interactions. For example, in therapeutic settings, AI systems can adapt their responses based on both the logical content of conversations and the emotional undertones, providing more meaningful support.
The ability to understand and control one’s emotions is crucial for navigating various challenges and complexities in life, and emotional empowerment can be a valuable tool for achieving this.
Nature Mental Health Research, 2024
Looking ahead, the success of cognitive and emotional intelligence integration in AI systems will depend on continued research, ethical guidelines, and technological advancement. Striking the right balance between technological capability and emotional sensitivity remains crucial for creating AI systems that can truly understand and respond to human needs.
Overcoming Cognitive Challenges
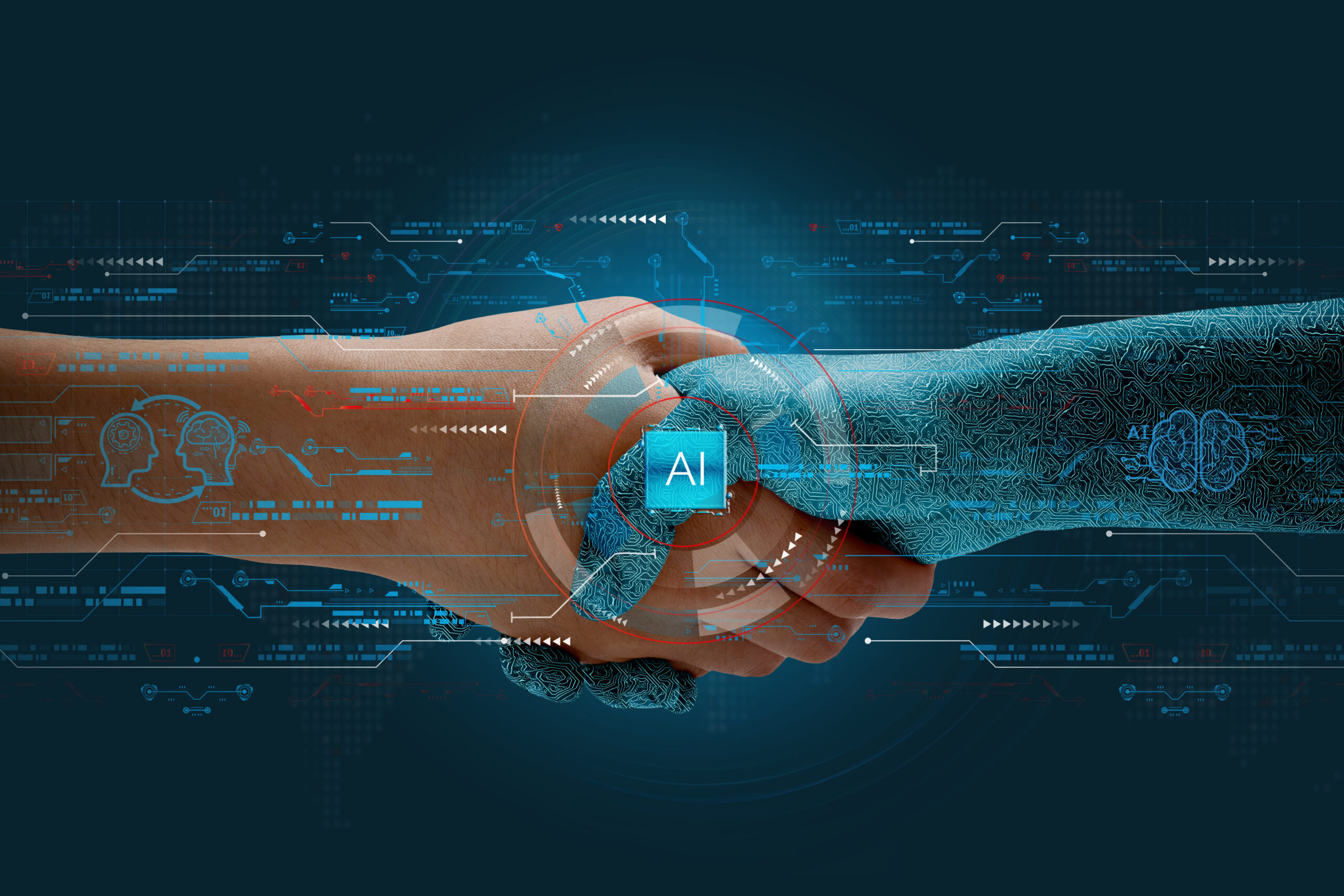
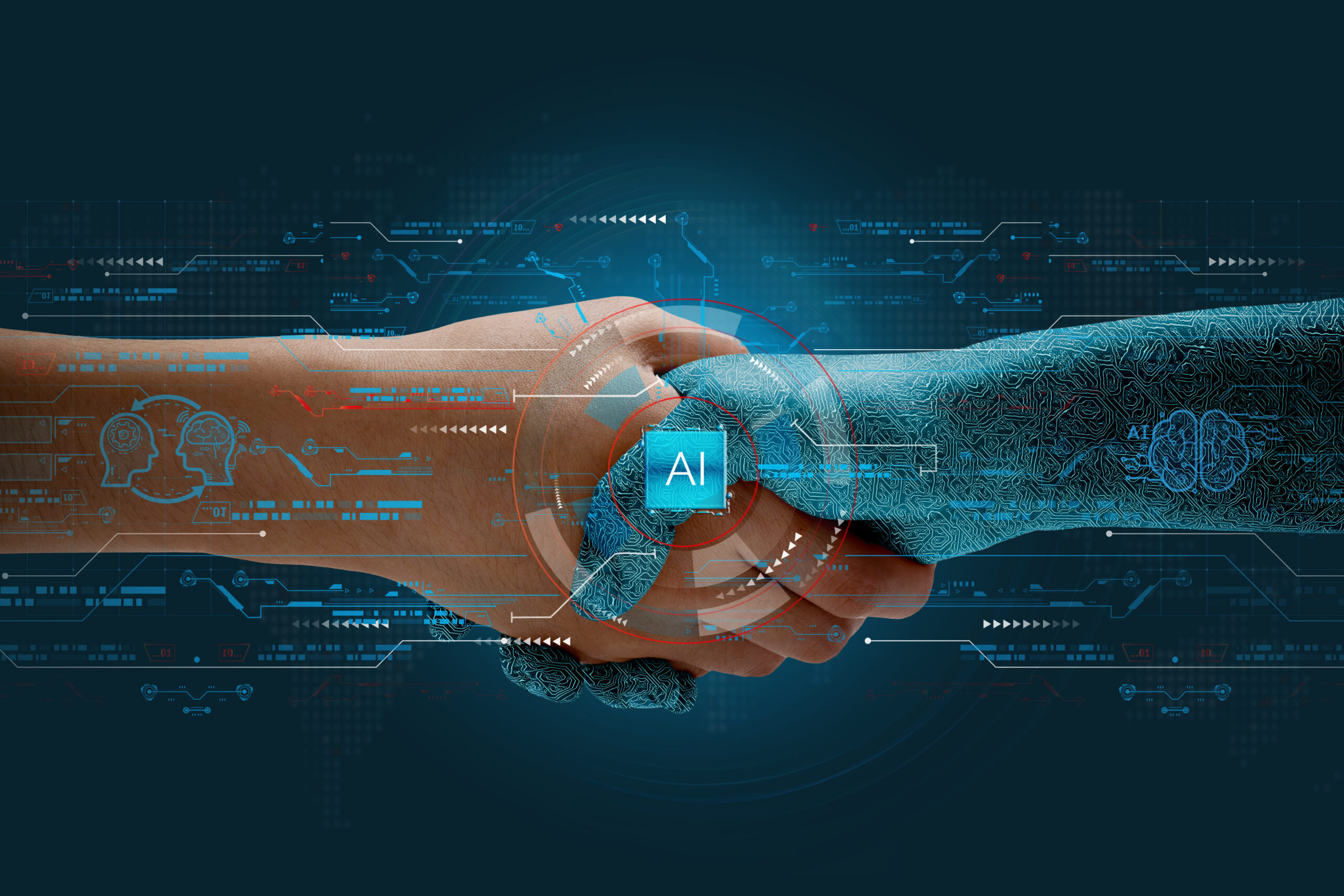
The integration of artificial intelligence into organizational decision-making processes brings unique cognitive hurdles that teams must actively address. Recent research examining human-AI collaboration reveals that while AI systems can enhance decision quality, success depends heavily on our ability to navigate the cognitive complexities of this partnership.
A fundamental challenge lies in the unconscious limitations of human metaknowledge – our understanding of what we know and don’t know. Unlike simple reluctance to use AI tools, these inherent cognitive constraints can severely impact how effectively we leverage AI capabilities. Teams often struggle to recognize their own biases and knowledge gaps when working alongside AI systems.
To overcome these barriers, organizations must first acknowledge that AI excels in situations characterized by information overload and unfamiliarity – precisely where human intuitive thinking tends to falter. By consciously delegating data-intensive tasks to AI while reserving contextual and creative decisions for human judgment, teams can establish more effective collaborative workflows.
One promising strategy involves implementing structured decision-making frameworks that clearly delineate human and AI responsibilities. This approach helps reduce cognitive load by preventing decision paralysis and over-reliance on either human intuition or AI recommendations alone. When team members understand their specific roles in the collaborative process, they can focus their cognitive resources more efficiently.
Another crucial aspect is developing robust feedback mechanisms. Regular evaluation of collaborative outcomes helps teams identify patterns in their decision-making processes and adjust their approach accordingly. This iterative learning process gradually builds the mental models necessary for more effective human-AI collaboration.
Building Cognitive Resilience
Successful human-AI collaboration requires developing what experts call ‘cognitive resilience’ – the ability to maintain clear judgment while working with AI systems. This involves training teams to recognize when they might be falling into common cognitive traps, such as automation bias or overthinking simple decisions.
Organizations can foster this resilience through targeted training programs that focus on metacognitive skills. These programs should emphasize understanding both the capabilities and limitations of AI systems, helping team members develop a realistic appreciation of when to rely on AI insights and when to trust their professional judgment.
Regular exposure to diverse decision-making scenarios helps build this cognitive flexibility. Teams should practice working with AI tools across various contexts, gradually increasing complexity as their collaboration skills improve. This approach helps develop the mental agility needed to navigate unexpected situations effectively.
Creating supportive environments that encourage open discussion about cognitive challenges is equally important. When team members feel comfortable sharing their struggles and insights about working with AI, it becomes easier to identify and address common obstacles collectively.
Establishing clear protocols for questioning AI recommendations also helps maintain cognitive engagement. Rather than accepting AI outputs passively, teams should develop structured approaches for evaluating AI-generated insights against their professional expertise and contextual understanding.
Enhancing Decision Quality
The ultimate goal of addressing cognitive challenges is to improve decision quality. Research shows that when teams effectively manage their cognitive limitations, they can leverage AI capabilities to reduce bias and enhance analytical rigor in their decision-making processes.
Implementing systematic review processes helps teams maintain high standards while working with AI. This includes regular assessments of decision outcomes and careful documentation of the reasoning behind key choices, whether they were driven by human insight, AI recommendations, or a combination of both.
Organizations should also invest in tools and processes that support transparent decision-making. When team members can clearly trace how decisions are made, they’re better equipped to identify potential cognitive biases and correct course as needed.
Encouraging a balance between speed and deliberation is crucial. While AI can accelerate decision-making processes, teams need to maintain enough cognitive engagement to catch potential errors or oversights. Setting appropriate timeframes for different types of decisions helps strike this balance.
Finally, fostering a culture of continuous learning and adaptation is essential. As AI capabilities evolve, teams must regularly update their mental models and collaboration strategies to maintain effective partnerships with these systems.
Impacts on Decision-Making Processes
AI-powered decision-making is fundamentally transforming how organizations operate and strategize. Research demonstrates that AI effectively reduces human cognitive burdens and mitigates decision biases by offering data-driven support and predictive analytics. Companies no longer need to rely solely on human intuition when making critical choices. AI systems can rapidly analyze vast amounts of data, identify complex patterns, and generate actionable insights that would be impossible for humans to process manually. This enhanced analytical capability enables organizations to make more informed decisions in time-sensitive situations.
The integration of AI into decision-making processes brings unprecedented speed and accuracy. Where traditional decision-making might take weeks of data gathering and analysis, AI-powered systems can provide comprehensive insights in minutes or hours. This dramatic reduction in decision cycle time gives organizations a significant competitive advantage in fast-moving markets.
Beyond speed, AI collaboration improves decision quality through its ability to consider multiple variables simultaneously. While human decision-makers might focus on a handful of key factors, AI systems can analyze hundreds or thousands of variables to identify subtle relationships and potential outcomes that humans might overlook. Generative AI systems can help overcome some of the problems affecting human decision making, such as limited working memory, short attention spans, and decision fatigue, especially when making decisions under pressure.
Organizations are seeing tangible benefits from AI-assisted decision-making. Teams can now focus on strategic thinking and creative problem-solving while AI handles the heavy lifting of data analysis and pattern recognition. This shift in responsibilities allows human decision-makers to leverage their unique strengths in judgment and contextual understanding while being supported by AI’s computational power.
However, it’s important to note that AI doesn’t replace human decision-makers – it empowers them. The most effective implementations of AI in decision-making processes create a symbiotic relationship where human insight and machine intelligence complement each other. This partnership leads to more nuanced, comprehensive, and effective decision-making across all levels of the organization.
Enhanced Creativity and Innovation
When human intelligence meets artificial intelligence, remarkable innovations emerge across industries. Rather than replacing human creativity, AI amplifies it by handling repetitive tasks and data analysis, allowing people to focus on higher-level creative thinking and strategic decisions.
In healthcare, the synergy between human expertise and AI has revolutionized diagnostics and treatment planning. Medical professionals now collaborate with AI systems to analyze complex medical images with unprecedented accuracy, enabling earlier detection of diseases like cancer while maintaining the critical human element of patient care and decision-making.
AI will be a disruptive technological force like we’ve never seen before – and we don’t yet know the full spectrum of capabilities it can offer
The financial sector demonstrates another compelling example of human-AI creative collaboration. Financial analysts leverage AI’s ability to process vast amounts of real-time data and identify market trends that would be impossible to detect manually. This partnership enables professionals to craft more nuanced and personalized investment strategies, combining AI-driven insights with human judgment and experience.
In scientific research, the combination of human intuition and AI processing power has accelerated innovation dramatically. Researchers now employ AI to analyze complex datasets and simulate scenarios in fields ranging from climate modeling to drug discovery. This collaboration has led to breakthroughs that would have been unimaginable just a decade ago, while still relying on human expertise to interpret and apply these findings meaningfully.
Manufacturing has seen similar transformative results through human-AI collaboration. Workers and AI systems now work together in predictive maintenance and quality control, with AI handling data-intensive monitoring while humans provide crucial oversight and strategic decision-making. This partnership has not only improved efficiency but has also sparked innovative approaches to long-standing industrial challenges.
The creative industries have also been enriched by human-AI collaboration. Rather than stifling human creativity, AI serves as an enabler, analyzing patterns and suggesting innovative solutions that humans can then refine and perfect. This symbiotic relationship has opened new dimensions of creative expression while maintaining the authenticity and emotional depth that only humans can provide.
Conclusion and Future Directions
The integration of human and artificial intelligence capabilities through collaborative systems represents a transformative frontier in technological advancement. Research shows that these hybrid partnerships unlock remarkable potential by combining human creativity and contextual understanding with AI’s analytical prowess and data processing capabilities.
The evolution of platforms like SmythOS signals a pivotal shift in how we approach human-AI collaboration. By providing a robust foundation for building autonomous agents that can integrate with human workflows, SmythOS enables organizations to develop sophisticated collaborative systems that enhance rather than replace human capabilities. Its visual debugging environment and enterprise-grade security controls create a framework where developers can safely experiment with and deploy collaborative AI solutions.
The field of human-AI collaboration will likely see continued innovation in areas such as adaptive interfaces, contextual awareness, and real-time learning systems. As highlighted in the latest research, successful collaborative systems must balance automation with meaningful human oversight while ensuring transparency in AI decision-making processes.
The future workplace will increasingly rely on these collaborative systems to drive productivity and innovation. SmythOS’s ability to handle complex integrations and manage resource scaling automatically positions it as a valuable platform for organizations looking to harness the transformative potential of human-AI collaboration. As we move forward, the focus must remain on developing systems that augment human capabilities while maintaining the essential elements of human creativity, ethical judgment, and strategic thinking.
By thoughtfully implementing these collaborative systems through platforms like SmythOS, organizations can create more efficient, innovative, and human-centric work environments that leverage the best of both human and artificial intelligence. The journey toward effective human-AI collaboration is just beginning, and the possibilities for transformation across industries are boundless.
Last updated:
Disclaimer: The information presented in this article is for general informational purposes only and is provided as is. While we strive to keep the content up-to-date and accurate, we make no representations or warranties of any kind, express or implied, about the completeness, accuracy, reliability, suitability, or availability of the information contained in this article.
Any reliance you place on such information is strictly at your own risk. We reserve the right to make additions, deletions, or modifications to the contents of this article at any time without prior notice.
In no event will we be liable for any loss or damage including without limitation, indirect or consequential loss or damage, or any loss or damage whatsoever arising from loss of data, profits, or any other loss not specified herein arising out of, or in connection with, the use of this article.
Despite our best efforts, this article may contain oversights, errors, or omissions. If you notice any inaccuracies or have concerns about the content, please report them through our content feedback form. Your input helps us maintain the quality and reliability of our information.