Top Frameworks for Effective Human-AI Collaboration: Building Smarter Systems Together
Imagine walking into your workplace and finding an AI partner ready to collaborate with you—not as a replacement, but as an intelligent teammate designed to enhance your capabilities. This future is rapidly becoming reality through human-AI collaboration frameworks, which are transforming how people and artificial intelligence systems work together.
At their core, these frameworks represent structured approaches that enable productive partnerships between human expertise and AI capabilities. They are practical blueprints addressing critical questions like: How should tasks be divided between humans and AI? When should humans take the lead, and when should AI step in? How can we ensure this collaboration remains ethical and beneficial?
What makes these frameworks fascinating is their potential to create what researchers call “hybrid intelligence”—a powerful fusion of human intuition and creativity with AI’s computational prowess and pattern recognition abilities. Through carefully designed interaction protocols and feedback mechanisms, these frameworks help establish trust, maintain transparency, and optimize how humans and AI complement each other.
The stakes are incredibly high. Recent research has shown that effective human-AI collaboration can dramatically improve decision-making accuracy, boost productivity, and unlock innovative solutions that neither humans nor AI could achieve alone. Yet creating these frameworks isn’t without its challenges—from ensuring ethical AI behavior to maintaining meaningful human control.
This article explores the key components that make these frameworks work, examines the hurdles organizations face in implementing them, and uncovers practical strategies for building successful human-AI partnerships. Whether you’re a business leader, developer, or simply curious about the future of work, understanding these frameworks is crucial for navigating the evolving landscape of human-AI collaboration.
Core Elements of Human-AI Collaboration
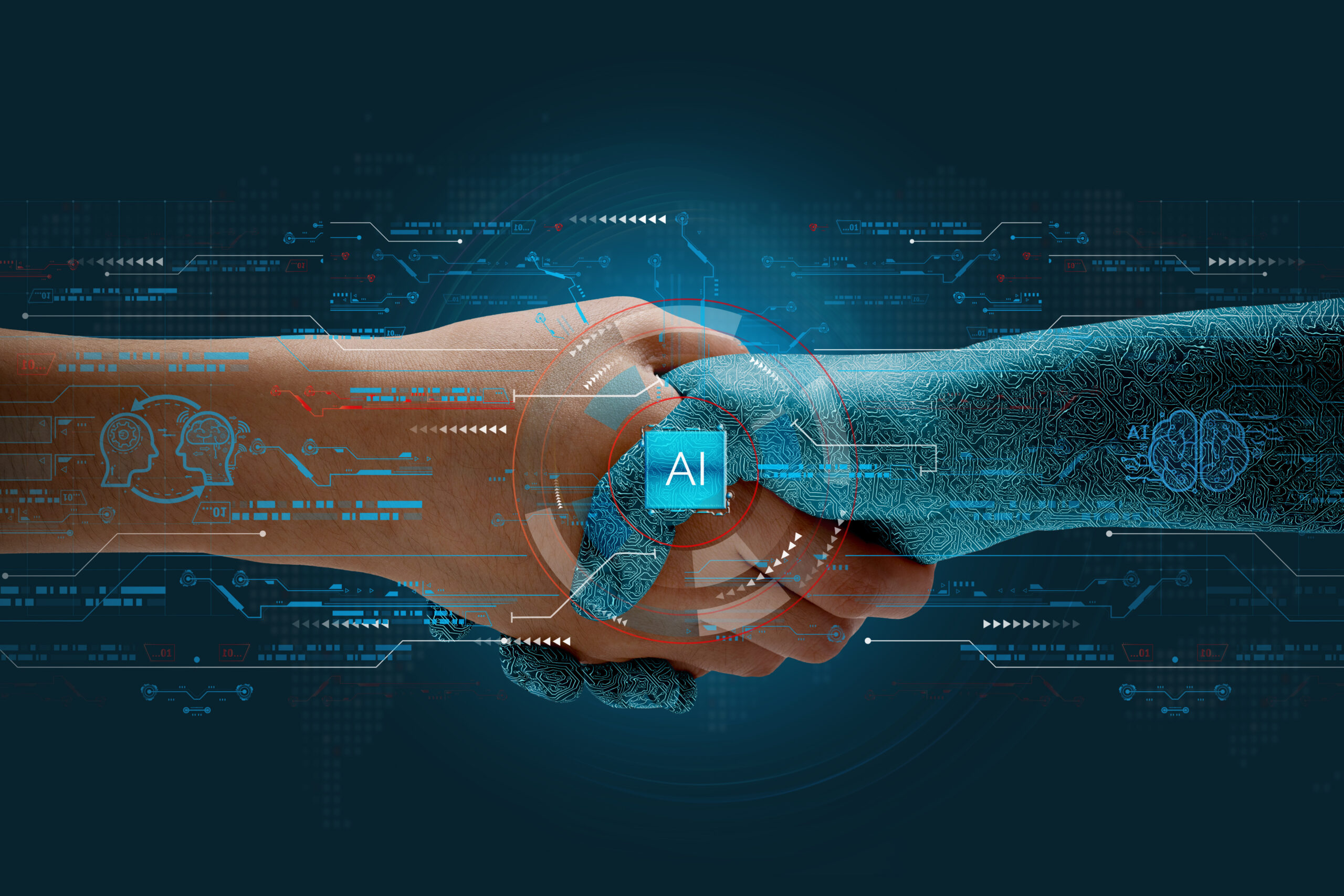
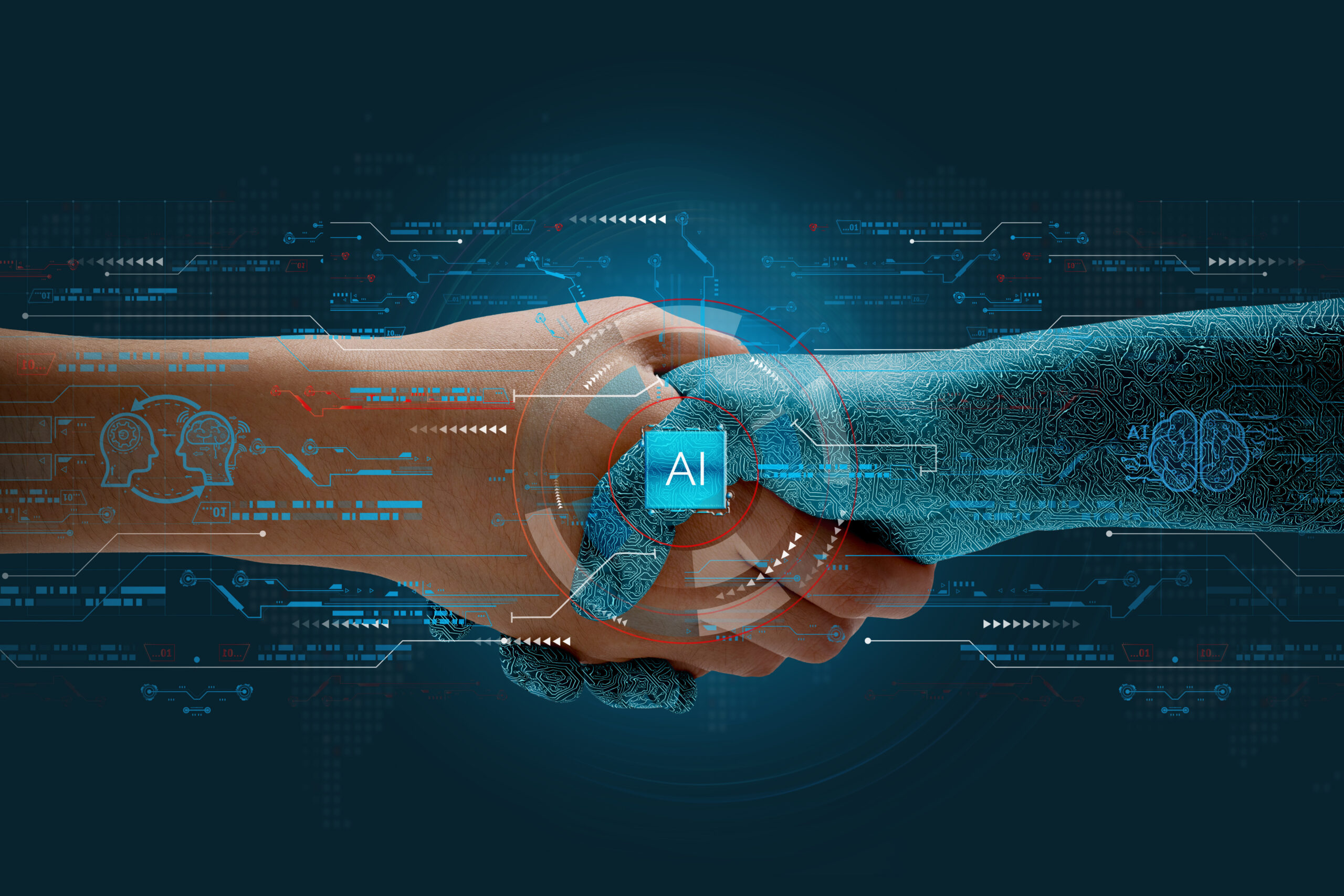
Building effective partnerships between humans and artificial intelligence requires understanding several fundamental components that shape how we work together. Tasks, goals, interaction patterns, and thoughtful allocation of responsibilities form the foundation of successful human-AI teamwork.
At the heart of human-AI collaboration lies the careful consideration of tasks. Not all activities are equally suited for AI assistance. Research shows that AI excels at processing vast amounts of data and handling repetitive operations, while humans bring critical thinking and nuanced judgment to complex scenarios. Understanding these complementary strengths allows organizations to assign tasks appropriately.
Clear shared goals provide essential direction and purpose to human-AI partnerships. When both human and AI team members understand the desired outcomes, they can align their efforts more effectively. For example, in customer service, the shared goal might be resolving customer inquiries quickly while maintaining high satisfaction levels – with AI handling routine questions and humans managing more complex issues.
The interaction between humans and AI represents another crucial element. Effective collaboration requires seamless communication channels and intuitive interfaces that allow humans to understand AI actions and provide necessary input. Like any successful partnership, the interaction should feel natural and supportive rather than forced or confusing.
Task allocation – deciding who does what and when – demands careful consideration of both human and AI capabilities. This isn’t simply about dividing work, but rather creating synergistic workflows where each party’s strengths complement the other. For instance, in healthcare diagnostics, AI might analyze medical images to flag potential concerns, while human doctors make final diagnoses using their broader medical knowledge and patient context.
A particularly important aspect of human-AI collaboration is adaptability. As circumstances change and new challenges arise, the division of tasks and interaction patterns must evolve. This dynamic nature of collaboration ensures that both human and AI capabilities are utilized optimally as technology advances and human skills develop.
The diverse but complementary capabilities of human-AI teams foster effective collaboration and enable the achievement of complex goals while ensuring human wellbeing, motivation, and productivity.
Kluge et al., 2021
Understanding and implementing these core elements effectively can transform how organizations approach human-AI collaboration. When properly balanced, these elements create a collaborative environment where both humans and AI can thrive, leading to improved outcomes and more satisfying work experiences for human team members.
Challenges in Implementing Human-AI Collaboration
The evolving landscape of artificial intelligence presents opportunities for human-AI collaboration, yet significant hurdles remain before we can fully realize its potential. Technical teams and organizations face complex challenges that extend beyond software integration issues.
Integration challenges arise when organizations blend AI capabilities with human workflows. Research shows that human-AI collaboration often performs less effectively than AI systems working independently, highlighting the need for thoughtful implementation strategies that optimize these partnerships.
Trust is a critical barrier in human-AI collaboration. When AI systems operate as black boxes, making decisions without clear explanations, human team members hesitate to rely on their outputs. This trust deficit can lead to resistance and underutilization of AI capabilities, even when the technology could enhance productivity and decision-making.
Managing inherent biases presents another significant challenge. AI systems can inadvertently perpetuate or amplify existing biases present in their training data. Development teams must implement robust bias detection and mitigation strategies while ensuring transparency about potential limitations.
Such tensions may occur during human-AI collaboration or competition. Task conflict and relationship conflict are the two major types of conflict.
Journal of Human-AI Interaction
Ethical considerations add another layer of complexity. Organizations must establish clear guidelines for AI usage that respect privacy, ensure fairness, and maintain human agency in decision-making processes. This includes developing frameworks for accountability and oversight that protect both users and stakeholders while fostering productive collaboration between human and artificial intelligence systems.
Ethical Considerations in Human-AI Collaboration
Developing ethical frameworks for human-AI collaboration represents one of the most critical challenges in modern technology development. As highlighted by the World Economic Forum, ensuring AI systems align with human values isn’t just a technical challenge; it’s a societal imperative that requires careful consideration of fairness, transparency, and accountability.
Fairness in AI systems goes beyond simple equal treatment. When AI makes decisions that affect human lives, whether in healthcare, hiring, or criminal justice, these systems must actively work to prevent discriminatory outcomes. This means carefully examining training data for historical biases and implementing rigorous testing protocols to ensure equitable treatment across different demographic groups.
Transparency emerges as another crucial pillar in ethical AI development. AI systems making high-stakes decisions must provide clear explanations for their actions; no more inscrutable ‘black box’ algorithms. This transparency allows humans to understand, validate, and when necessary, challenge AI-generated recommendations. Organizations must prioritize explainable AI techniques that make decision-making processes accessible to both technical and non-technical stakeholders.
The question of accountability presents unique challenges in human-AI collaboration. When AI systems make mistakes or cause harm, who bears responsibility? The developers? The organizations deploying them? The AI itself? Creating clear accountability frameworks requires establishing explicit chains of responsibility and implementing robust oversight mechanisms. Regular audits, impact assessments, and governance structures must be put in place to ensure AI systems remain accountable to human values and ethical principles.
Addressing bias in AI systems demands particular attention. Historical biases in training data can lead to AI systems perpetuating or even amplifying existing societal inequities. Teams developing AI must proactively identify potential sources of bias, implement rigorous testing protocols, and continuously monitor system outputs for signs of discriminatory patterns.
The path forward requires a collaborative approach involving diverse stakeholders—from technologists and ethicists to policymakers and affected communities. Only through this inclusive dialogue can we develop AI systems that truly serve humanity’s best interests while respecting fundamental ethical principles.
Applications of Human-AI Collaboration Frameworks
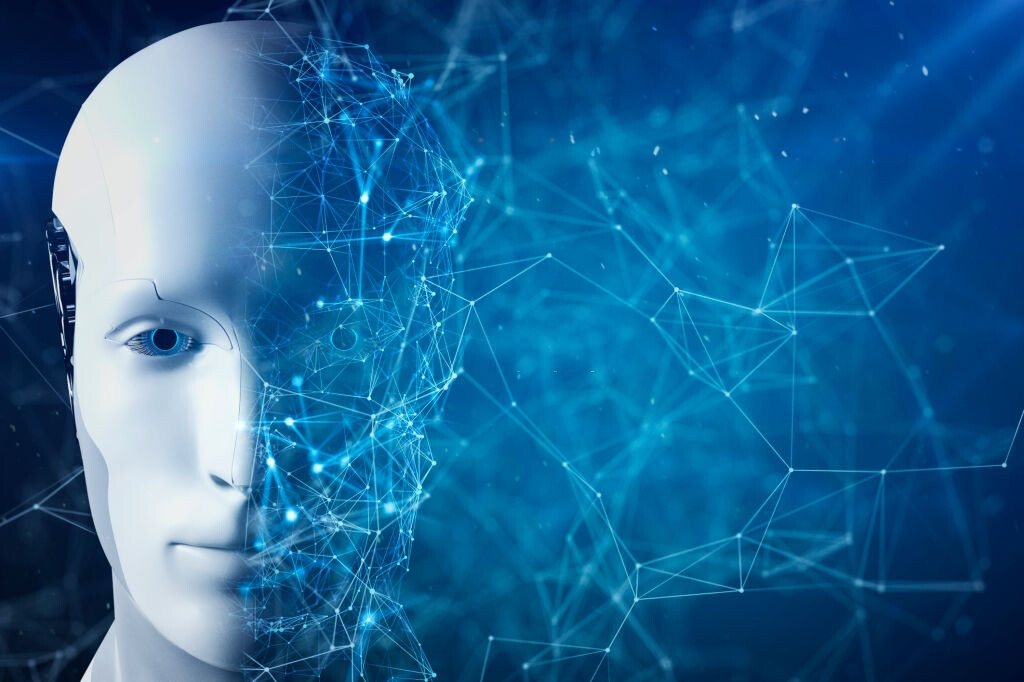
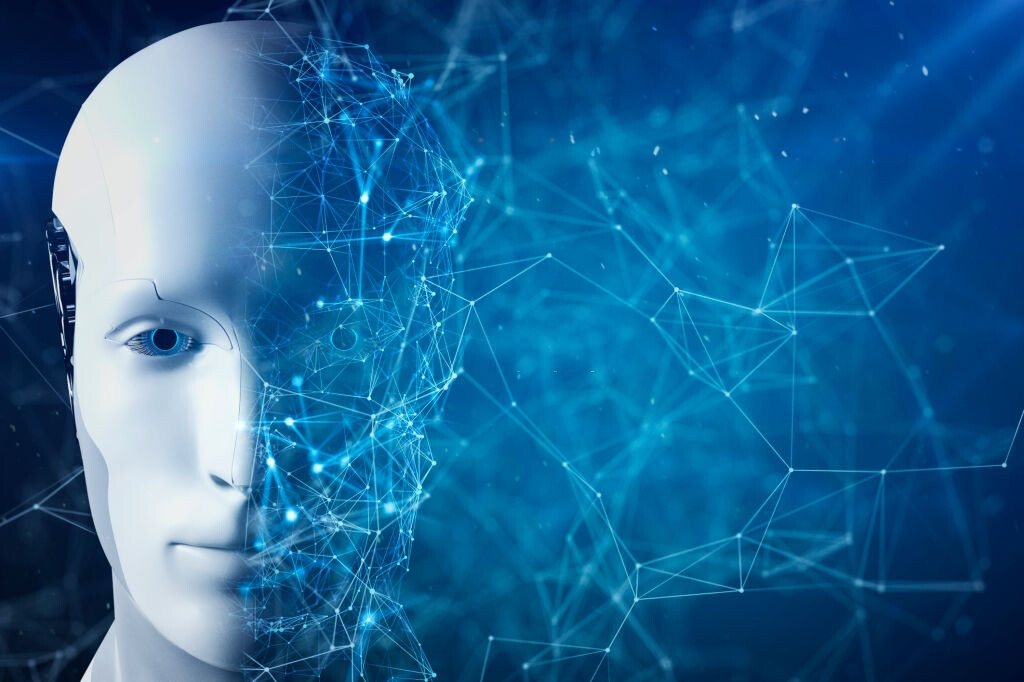
Exploring human-AI collaboration in a digital world – Via innomaint.com
Human-AI collaboration has evolved into sophisticated frameworks delivering tangible value across multiple sectors. Healthcare stands at the forefront, where AI-powered diagnostic tools are transforming patient care and clinical decision-making.
In healthcare, AI enhances diagnostic accuracy and enables earlier disease detection. Radiologists now work alongside AI systems that serve as a second set of eyes, improving the detection of subtle abnormalities in medical imaging. The combined expertise of human physicians and AI leads to more accurate diagnoses and better patient outcomes.
Manufacturing has witnessed remarkable efficiency gains through human-AI collaboration. Smart factories employ AI systems that work with human operators to optimize production lines, predict equipment maintenance needs, and ensure quality control. This partnership has led to reduced downtime, improved product quality, and enhanced workplace safety.
In the financial sector, AI frameworks augment human decision-making in complex scenarios. Investment analysts collaborate with AI systems to process vast amounts of market data, identify patterns, and make more informed trading decisions. The human element remains crucial in interpreting AI-generated insights and considering broader market contexts that machines might miss.
Educational institutions are revolutionizing learning through AI-enabled personalized instruction. Teachers work with AI platforms to track student progress, identify learning gaps, and customize curriculum delivery. This collaboration ensures students receive targeted support while preserving the irreplaceable human elements of mentorship and emotional guidance.
Every minute that a patient doesn’t receive care or intervention, a little bit more of their brain becomes irreversibly damaged.
Dr. Po-Hao Chen, Diagnostic Radiologist
Real-world implementations demonstrate how these frameworks enhance organizational efficiency while maintaining human oversight. For instance, medical facilities using AI-powered diagnostic tools have shown significant improvements in early disease detection rates, while manufacturing plants report substantial reductions in production errors through human-AI collaborative quality control systems.
Sector | Application | Details |
---|---|---|
Healthcare | AI-Assisted Diagnostics | AI systems like SepsisLab predict and visualize the likelihood of sepsis, aiding clinicians in decision-making. |
Search and Rescue | Smart Drones | AI-enabled drones autonomously detect and follow forest paths to find missing persons, reducing response times. |
Creative Arts | Music Composition | Generative AI creates new melodies, rhythms, and harmonies, offering fresh creative insights to composers. |
Customer Service | AI-Powered Chatbots | Chatbots handle routine queries, freeing human agents to address more complex issues, improving response times. |
Finance | Predictive Analytics | AI helps investment professionals make data-driven decisions by analyzing vast datasets and identifying trends. |
Manufacturing | Smart Factories | AI systems optimize production lines, predict equipment maintenance needs, and ensure quality control. |
Best Practices for Building Effective Human-AI Teams
The landscape of human-AI collaboration has evolved dramatically, moving beyond simple automation to create genuine partnerships between human operators and AI systems. Drawing from extensive research and real-world implementations, several key practices have emerged as crucial for building successful human-AI teams.
Clear communication stands as the cornerstone of effective human-AI collaboration. Research has shown that coordination between humans and AI teammates heavily relies on well-structured communication protocols. This means establishing precise channels for information exchange and ensuring AI systems can effectively convey their decision-making processes and limitations to their human counterparts.
Continuous feedback mechanisms serve as the neural network of human-AI teams, enabling constant refinement and improvement. Like a basketball team practicing their plays, human-AI teams need regular opportunities to assess their performance and adjust their strategies. This involves implementing systematic ways to track successes and failures, allowing both human team members and AI systems to learn from their interactions and optimize their collaborative approach.
Adaptive learning capabilities represent another critical element for success. AI systems must demonstrate flexibility in adjusting to different human working styles and preferences. Similarly, human team members need to develop an understanding of AI capabilities and limitations. This mutual adaptation process creates a more cohesive and effective team dynamic.
The establishment of clear roles and responsibilities helps prevent confusion and overlap in team functions. Rather than viewing AI as a replacement for human capabilities, successful teams position AI as a complement to human strengths. For instance, while AI might excel at rapid data analysis, humans typically better handle complex decision-making that requires emotional intelligence or ethical considerations.
Creating Trust and Understanding
Building trust between human and AI team members requires transparency in AI operations and decision-making processes. When team members understand how their AI counterparts arrive at conclusions, they are more likely to develop appropriate levels of trust and reliance on the system.
Regular training and skill development opportunities help both human and AI team members stay current with evolving capabilities and requirements. This might include simulations of various scenarios, hands-on practice with new features, and structured learning sessions about system updates or improvements.
Performance monitoring and evaluation should be ongoing, with metrics that reflect both individual and team success. These assessments should consider not just task completion rates but also the quality of collaboration and the team’s ability to handle unexpected situations effectively.
Through collaborative intelligence, AI can complement humans, not replace them. Collaborative intelligence empowers teams to achieve goals that might have been out of reach without the help of AI.
Organizations must also establish clear protocols for handling disagreements or conflicts between human and AI team members. This includes creating procedures for human override when necessary and ensuring that final decision-making authority rests with appropriate human team members in critical situations.
Success in human-AI collaboration ultimately depends on maintaining a balanced approach that leverages the unique strengths of both human and artificial intelligence while acknowledging and compensating for their respective limitations. By following these best practices, organizations can create more resilient and effective teams that maximize the potential of both human and AI capabilities.
Leveraging SmythOS for Enhanced Collaboration
SmythOS enhances human-AI collaboration through its sophisticated platform that seamlessly integrates human capabilities with artificial intelligence. The platform enables natural collaboration between team members and AI agents, similar to how human colleagues work together.
At the core of SmythOS’s collaborative framework is its comprehensive monitoring system, providing unprecedented visibility into the AI ecosystem. Through a centralized dashboard, teams can track agent performance, resource utilization, and system health in real-time, enabling quick identification and resolution of any potential issues impacting collaboration.
The platform’s extensive integration capabilities set it apart in AI collaboration. With connections to over 300,000 apps, APIs, and data sources, SmythOS ensures AI agents can access vast ecosystems of information while maintaining robust security protocols. This connectivity empowers teams to create sophisticated collaborative workflows leveraging diverse data sources and services.
Enterprise-grade security controls form another crucial pillar of SmythOS’s collaborative framework. These measures ensure that autonomous agents operate within secure parameters while protecting sensitive data and maintaining compliance with industry standards. This is particularly valuable for organizations handling confidential information or operating in regulated industries where security is paramount.
By ensuring students truly understand the future of AI Orchestration and are equipped to walk into companies across the globe with a fundamental understanding of how to build multi-agent systems, we believe we can empower future generations to harness the power of artificial intelligence rather than fear it.
The platform’s intuitive visual builder democratizes AI development, making complex agent creation accessible to both technical experts and domain specialists. This inclusive approach ensures that teams can focus on innovation and problem-solving rather than getting bogged down by technical complexities, fostering a more collaborative and productive environment.
Conclusion and Future Directions
The landscape of human-AI collaboration continues to evolve, presenting both exciting opportunities and significant challenges. Successful integration of autonomous agents into human workflows requires careful consideration of trust, privacy, and ethical frameworks. The path forward demands a delicate balance between technological advancement and human values. Recent research from the Massachusetts Institute of Technology highlights a crucial insight: effective human-AI collaboration frameworks must prioritize transparency and accountability. This approach builds trust and ensures that autonomous systems remain aligned with human objectives while maintaining the flexibility to adapt to changing needs.
The development of more sophisticated emotional intelligence capabilities in AI systems represents a promising frontier. Recent studies indicate that human-AI collaboration achieves optimal results when artificial intelligence can effectively interpret and respond to human emotional and cognitive states, creating truly synergistic relationships across various domains.
SmythOS emerges as a notable solution in this evolving landscape, offering robust tools for building and deploying autonomous agents that can seamlessly integrate with human workflows. Its visual debugging environment and enterprise-grade security controls address many of the current challenges in human-AI collaboration, while its ability to handle complex integrations positions it well for future advancements in the field.
Looking ahead, we can expect continued refinement of collaborative frameworks as technology advances. The future of human-AI collaboration lies not in replacement but in enhancement—creating systems that amplify human capabilities while preserving the irreplaceable aspects of human creativity, empathy, and strategic thinking. As these technologies mature, their applications will likely expand into new domains, creating opportunities we have yet to imagine.
Last updated:
Disclaimer: The information presented in this article is for general informational purposes only and is provided as is. While we strive to keep the content up-to-date and accurate, we make no representations or warranties of any kind, express or implied, about the completeness, accuracy, reliability, suitability, or availability of the information contained in this article.
Any reliance you place on such information is strictly at your own risk. We reserve the right to make additions, deletions, or modifications to the contents of this article at any time without prior notice.
In no event will we be liable for any loss or damage including without limitation, indirect or consequential loss or damage, or any loss or damage whatsoever arising from loss of data, profits, or any other loss not specified herein arising out of, or in connection with, the use of this article.
Despite our best efforts, this article may contain oversights, errors, or omissions. If you notice any inaccuracies or have concerns about the content, please report them through our content feedback form. Your input helps us maintain the quality and reliability of our information.