Enhancing Human-AI Collaboration: The Role of Transparency in Building Trust
Picture a world where human intuition meets machine precision – not in some distant future, but right now. Harvard Business Review research involving 1,500 companies reveals a striking insight: the most remarkable performance gains emerge when humans and artificial intelligence work as true partners rather than when AI simply replaces human workers.
Trust lies at the heart of this partnership. While AI excels at processing vast datasets and identifying subtle patterns in milliseconds, humans bring irreplaceable creativity, emotional intelligence, and nuanced judgment to the table. But how do we build genuine trust between human operators and AI systems?
The answer lies in transparency. A recent study in Information Systems Frontiers demonstrates that when AI systems clearly communicate their reasoning and capabilities, both trust and task outcomes improve significantly. This transparency isn’t just about showing how AI works – it’s about creating a genuine dialogue between human and machine intelligence.
Consider a surgeon working alongside an AI diagnostic system. When the AI explains its analysis process and clearly communicates levels of certainty, the surgeon can better integrate this information with their years of clinical experience. This collaborative approach leads to better decisions than either human or AI could achieve alone.
Achieving meaningful transparency in AI systems requires more than just raw data disclosure. It demands thoughtful design that bridges the gap between complex algorithms and human understanding. Organizations succeeding in this space recognize that effective human-AI collaboration isn’t about replacing human judgment – it’s about augmenting it with AI’s unique capabilities while maintaining clear lines of communication and understanding.
Challenges in Human-AI Collaboration
Healthcare professionals face mounting pressure to integrate artificial intelligence into their clinical workflows while maintaining quality patient care. As recent studies highlight, the successful deployment of AI technologies demands careful consideration of human factors, transparency, and trust-building mechanisms.
One primary obstacle is seamlessly incorporating AI systems into existing clinical workflows without disrupting established practices. Healthcare providers must adapt their routines while ensuring AI tools genuinely enhance rather than hinder their ability to deliver care. This integration challenge extends beyond technical implementation to include training requirements, changes in documentation processes, and modifications to team communication patterns.
The presence of algorithmic bias poses another significant concern in human-AI collaboration. AI systems trained on historical medical data may perpetuate existing healthcare disparities or demonstrate reduced accuracy for certain demographic groups. Medical professionals must remain vigilant in identifying potential biases and work closely with technical teams to validate AI recommendations against diverse patient populations.
Establishing and maintaining user trust represents an ongoing challenge in human-AI collaboration. Healthcare workers need to feel confident in AI systems’ reliability while understanding their limitations. This requires transparent explanations of AI decision-making processes and clear protocols for when human judgment should override algorithmic recommendations.
Addressing these challenges requires a methodical approach focused on healthcare workers’ needs and experiences. Regular feedback loops between clinical staff and AI development teams help refine systems to better serve real-world healthcare environments. Additionally, implementing robust governance frameworks ensures appropriate oversight while fostering an environment where humans and AI can effectively collaborate to improve patient outcomes.
Role of Transparency in Building Trust
AI transparency represents a crucial bridge between artificial intelligence systems and human trust. When organizations provide clear visibility into how their AI makes decisions, users gain confidence in these systems for important tasks. Transparency opens a window into the AI’s thinking process, allowing users to understand not just what decisions are made, but how and why they’re made.
According to IBM research, AI transparency helps users access vital information about how an artificial intelligence system was created, what data trained it, and the logic behind its decision-making processes. This level of openness is especially critical in high-stakes industries like healthcare, finance, and human resources, where AI decisions can significantly impact people’s lives.
The impact of transparency on trust becomes clear when we examine real-world implementations. For instance, when financial institutions clearly explain how their AI evaluates loan applications, including the specific factors considered and how they’re weighted, customers are more likely to accept the outcomes, even if unfavorable. This transparency helps dispel the black box perception that often surrounds AI systems.
There are three key elements that make transparency effective in building trust. First, organizations must provide clear documentation of the AI system’s purpose and capabilities. Second, they need to explain the decision-making process in understandable terms. Finally, they should offer insights into how the system is monitored and updated to maintain accuracy and fairness.
Transparency also plays a vital role in identifying and addressing potential biases in AI systems. When organizations are open about their AI’s limitations and actively work to address them, it demonstrates accountability and strengthens user confidence. This approach aligns with emerging regulations and best practices that emphasize the importance of explainable AI in building trustworthy systems.
Transparency is about clearly explaining the reasoning behind the output, making the decision-making process accessible and comprehensible. At the end of the day, it’s about eliminating the black box mystery of AI and providing insight into the how and why of AI decision-making.
Adnan Masood, Chief AI Architect at UST
The practice of transparency extends beyond technical explanations. It includes communicating how AI systems are governed, tested for fairness, and integrated with human oversight. This comprehensive approach to transparency helps create a foundation of trust that supports wider AI adoption and more effective human-AI collaboration.
Implementing Transparency in AI Systems
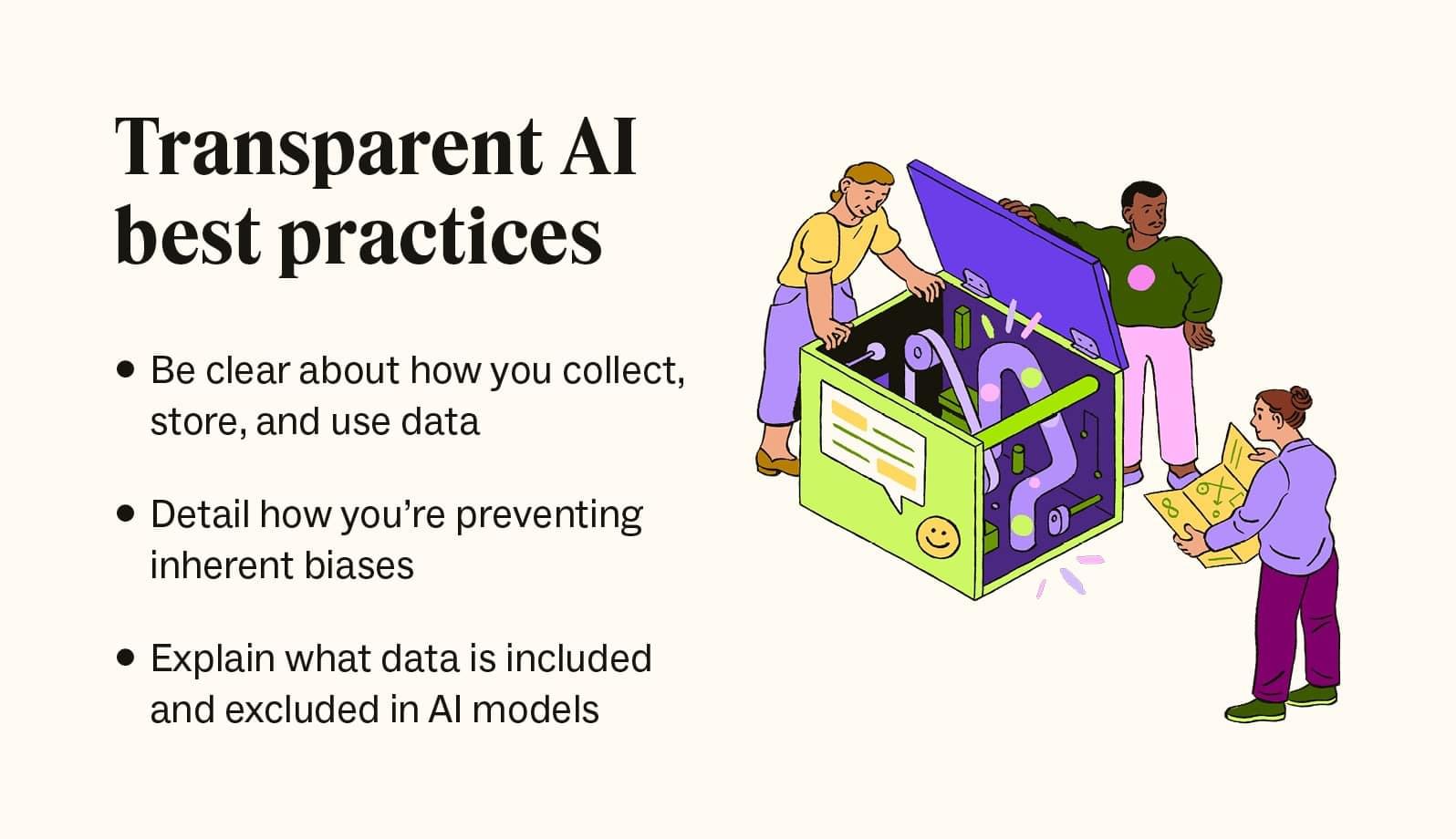
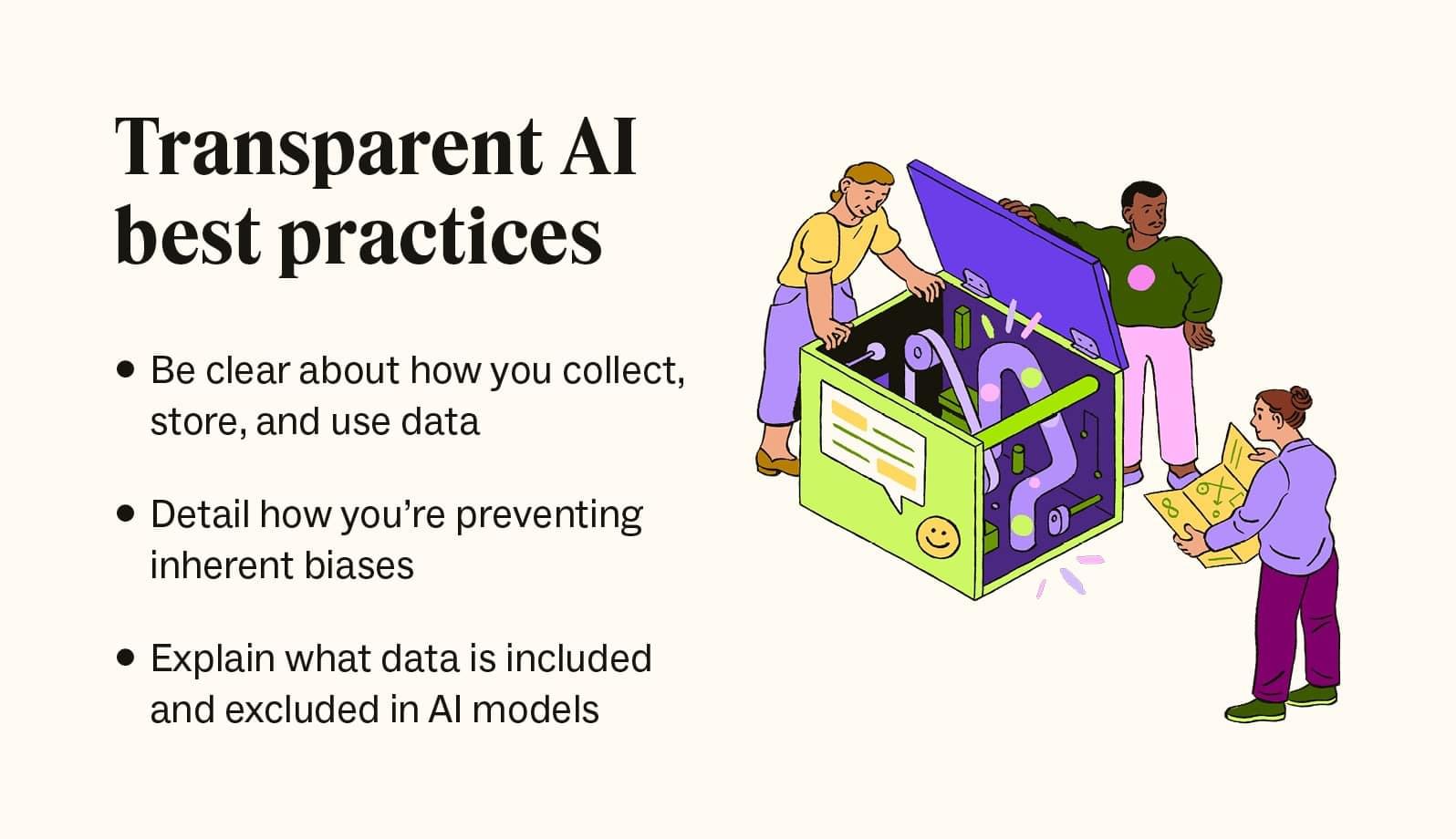
Building transparent AI systems requires methodical implementation of explainability mechanisms from the ground up. Modern AI deployments must balance sophisticated capabilities with clear communication about how decisions are made, creating trust through visibility into the AI’s processes and limitations.
Organizations implementing AI transparency should start by establishing clear documentation practices. This includes detailed records of data sources, model architectures, and training procedures. As noted by industry experts, comprehensive documentation helps stakeholders understand not just what the AI does, but how and why it makes specific decisions.
Model explanations serve as a cornerstone of transparent AI implementation. These tools break down complex algorithmic decisions into understandable components, allowing users to trace the logic behind AI outputs. For instance, techniques like SHAP (SHapley Additive exPlanations) help quantify how each input feature influences the model’s predictions, making the decision process more accessible to non-technical stakeholders.
Establishing Feedback Mechanisms
Effective transparency requires robust feedback loops between AI systems and their users. Regular monitoring and user feedback help identify potential biases, errors, or unexpected behaviors early in the deployment process. This two-way communication ensures the AI system remains aligned with user needs and expectations.
Organizations should implement multiple channels for gathering feedback, from automated performance metrics to direct user input. This comprehensive approach helps capture both quantitative and qualitative insights about the AI’s operation. Studies show that organizations prioritizing user feedback in their AI implementations achieve higher levels of trust and adoption.
Continuous monitoring of these feedback mechanisms allows organizations to track the AI system’s effectiveness over time. Regular analysis of user interactions and system responses helps identify areas where transparency can be improved or where additional explanations may be needed.
Communication Strategies
Clear communication about AI capabilities and limitations forms another crucial aspect of transparency implementation. Organizations must be upfront about what their AI systems can and cannot do, avoiding overpromising or understating potential risks.
Effective communication strategies include:
- Regular updates about system changes and improvements
- Clear documentation of known limitations
- Proactive disclosure of potential biases or uncertainties
- Accessible explanations of complex technical concepts
Organizations should tailor their communication approach to different stakeholder groups, ensuring that technical and non-technical audiences alike can understand how the AI system operates and affects their work.
Validation and Verification
Implementing transparency requires rigorous validation processes to ensure that explanations accurately reflect the AI’s decision-making. Regular audits and assessments help verify that transparency mechanisms are functioning as intended and meeting stakeholder needs.
AI transparency goes beyond compliance and risk mitigation. It fosters a culture of trust and accountability. By embracing transparency in the entire AI lifecycle, you can leverage its massive potential while ensuring your use aligns with the highest ethical principles.
Organizations should establish clear metrics for measuring transparency effectiveness, regularly assessing whether their implementation meets established goals and standards. This ongoing evaluation helps identify areas for improvement and ensures the AI system maintains its commitment to openness and accountability.
Transparency Mechanism | Benefit |
---|---|
SHAP | Quantifies feature influence on predictions |
Feedback Loops | Identifies biases and errors early |
Clear Communication | Builds trust with stakeholders |
Regular Audits | Ensures ongoing transparency |
Case Study: SmythOS in Human-AI Collaboration
SmythOS represents a significant advancement in human-AI collaboration, transforming how organizations orchestrate AI systems alongside human teams. The platform provides comprehensive monitoring and logging capabilities that ensure transparency and control in AI operations, critical for building trust between human operators and AI systems.
What sets SmythOS apart is its visual workflow builder, which democratizes AI development by allowing both technical and non-technical team members to design sophisticated AI workflows without coding expertise. As Alexander De Ridder, Co-Founder and CTO of SmythOS, explains, ‘This isn’t just about AI automating repetitive work but also about creating intelligent systems that learn, grow, and collaborate with humans to achieve far more than either could alone.’
The platform’s success in fostering effective human-AI collaboration is evident in its implementation across various organizations. For instance, digital agencies have leveraged SmythOS to create AI agents that handle content optimization and analysis while human creatives focus on strategy and emotional resonance. This partnership has led to reported efficiency gains of up to 10x in content creation workflows while maintaining high-quality output.
Industry | Efficiency Gain | Specific Outcome |
---|---|---|
Retail (Walmart) | 10% increase in sales | 20% reduction in unnecessary inventory |
Healthcare (Aidoc) | 30% reduction in scan review time | Faster diagnosis and treatment |
Beverage (Coca-Cola) | 10% reduction in transportation costs | Optimized logistics |
Logistics (UPS) | Millions saved annually | Optimized delivery routes and reduced fuel consumption |
Finance (JP Morgan Chase) | 5% reduction in fraudulent transactions | Improved operational efficiency |
A particularly compelling aspect of SmythOS’s approach is its emphasis on ‘constrained alignment’—ensuring AI systems operate within clearly defined parameters while maintaining human oversight. This framework allows organizations to automate routine tasks confidently while preserving human judgment for critical decisions requiring emotional intelligence and ethical consideration.
The platform’s built-in monitoring capabilities provide unprecedented visibility into AI operations, enabling teams to track agent behavior, identify potential issues, and optimize performance in real-time. This transparency has proven crucial for building trust and ensuring accountability in human-AI collaborations, particularly in enterprise environments where reliability and consistency are paramount.
By thinking through this list, I will have a better sense of where I am responsible to make the tool more useful, safe, and beneficial for the people using it. The public can also be better assured that I took these parameters into consideration when working on the design of a system that they may trust and then embed in their everyday life.
Future of Human-AI Collaboration
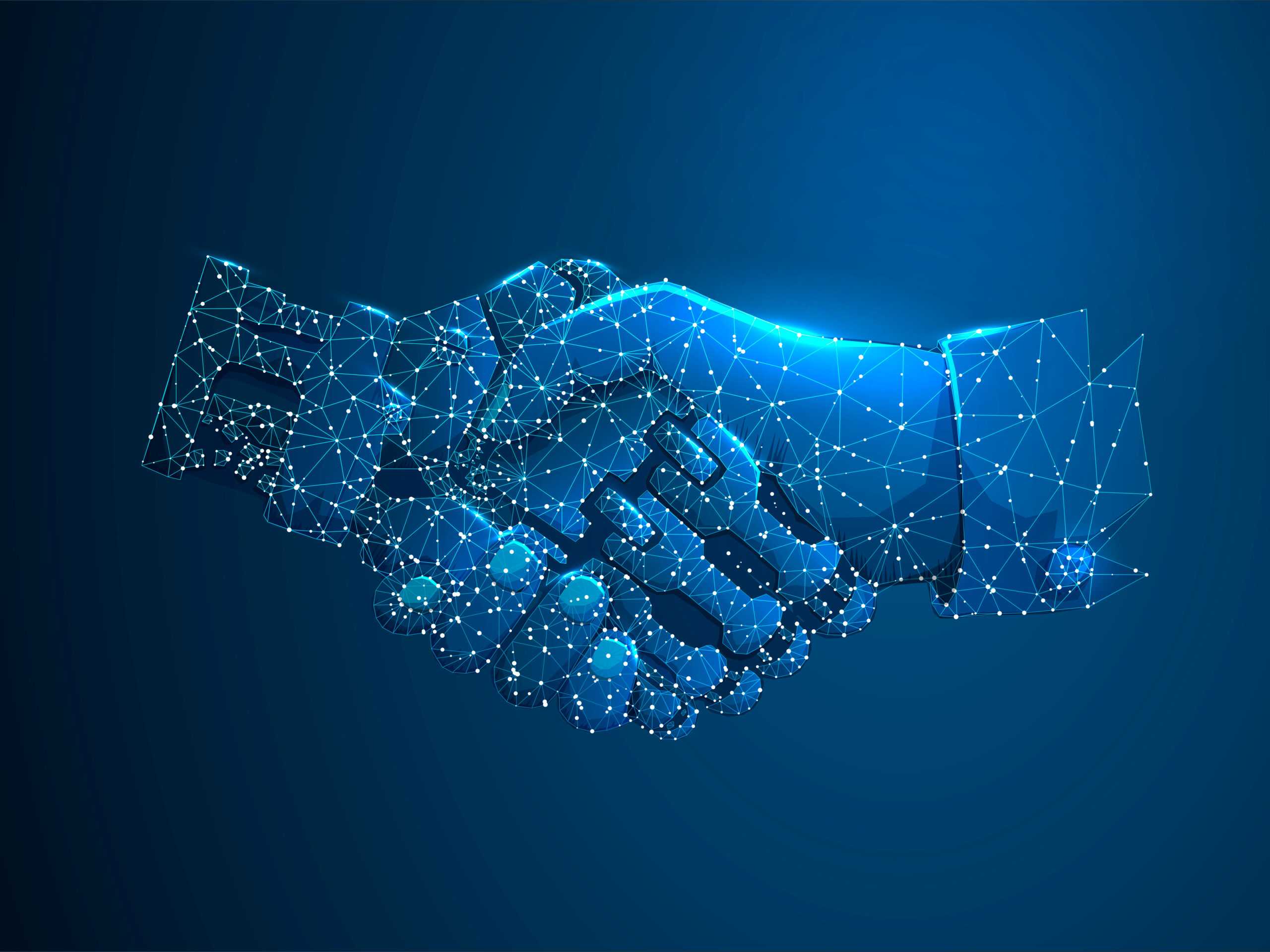
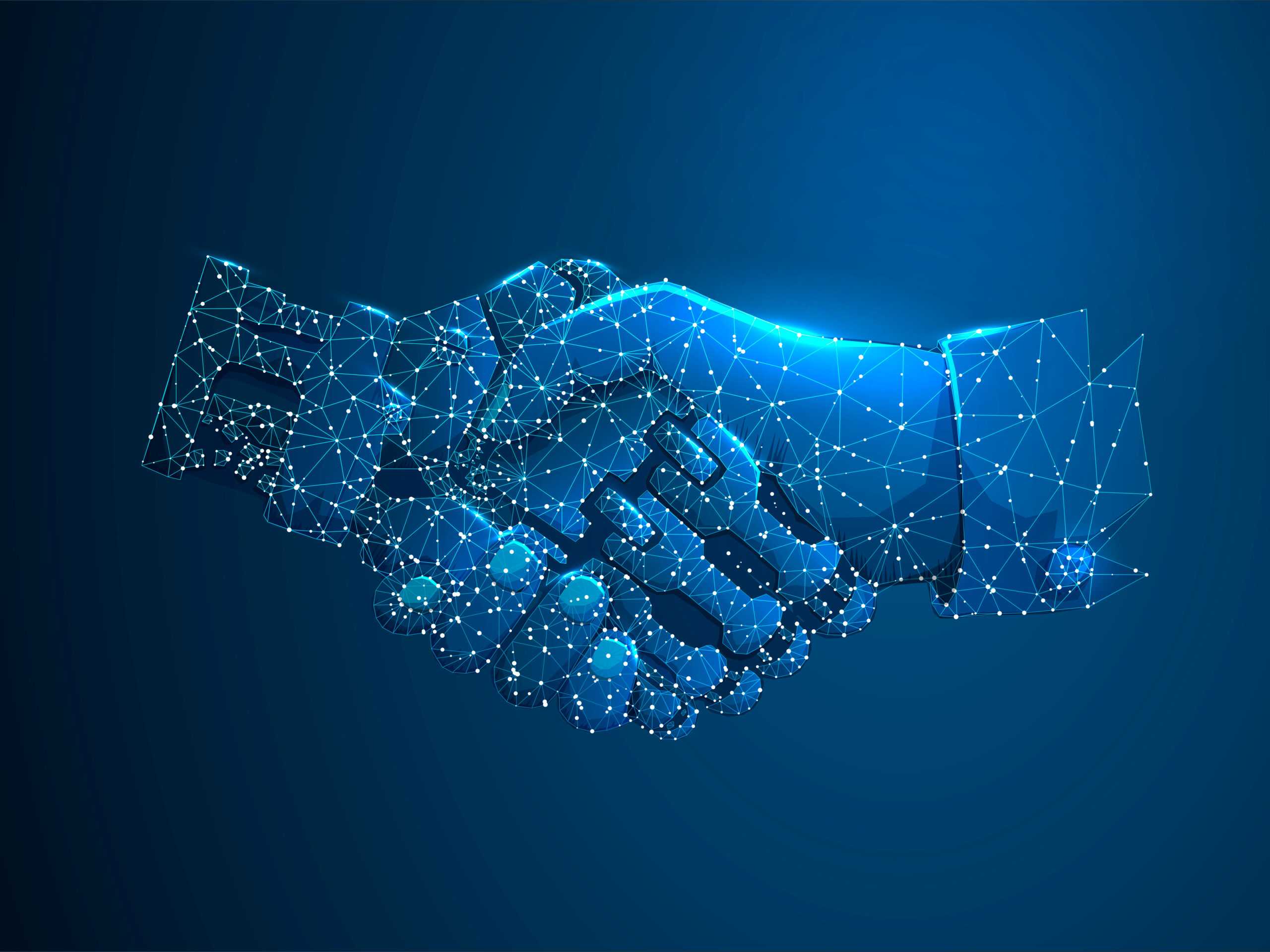
Collaboration and trust in a digital world. – Via csiro.au
The landscape of human-AI collaboration is evolving rapidly, with emerging frameworks focused on increased transparency and improved trust between human users and AI systems. As AI capabilities expand, the emphasis is shifting toward creating more intuitive and reliable partnerships between humans and machines.
Transparency is proving to be a critical foundation for effective collaboration. When AI systems can clearly communicate their reasoning process and decision-making framework, users develop a deeper understanding and confidence in working alongside them. This enhanced visibility into AI operations allows humans to make more informed decisions about when to rely on AI recommendations and when to apply their own expertise.
The future promises more sophisticated frameworks that will bridge the current gaps between human intuition and machine intelligence. These emerging technologies are being designed with user experience at the forefront, moving away from black-box solutions toward systems that provide clear explanations of their processes and limitations. This evolution in AI design reflects a growing recognition that successful human-AI collaboration requires both technical capability and human-centered interaction.
Trust-building mechanisms are becoming increasingly sophisticated, with new frameworks incorporating features like real-time feedback loops and adaptive learning systems that respond to user preferences and behaviors. These advancements are making AI systems more reliable partners in complex decision-making processes, while maintaining the crucial element of human oversight and judgment.
Looking ahead, we can expect to see AI frameworks that seamlessly integrate with human workflows, adapting to different expertise levels and working styles. The focus will be on creating collaborative environments where AI augments human capabilities rather than replacing them, leading to more productive and innovative outcomes across various industries and applications.
Conclusion and Benefits of Using SmythOS
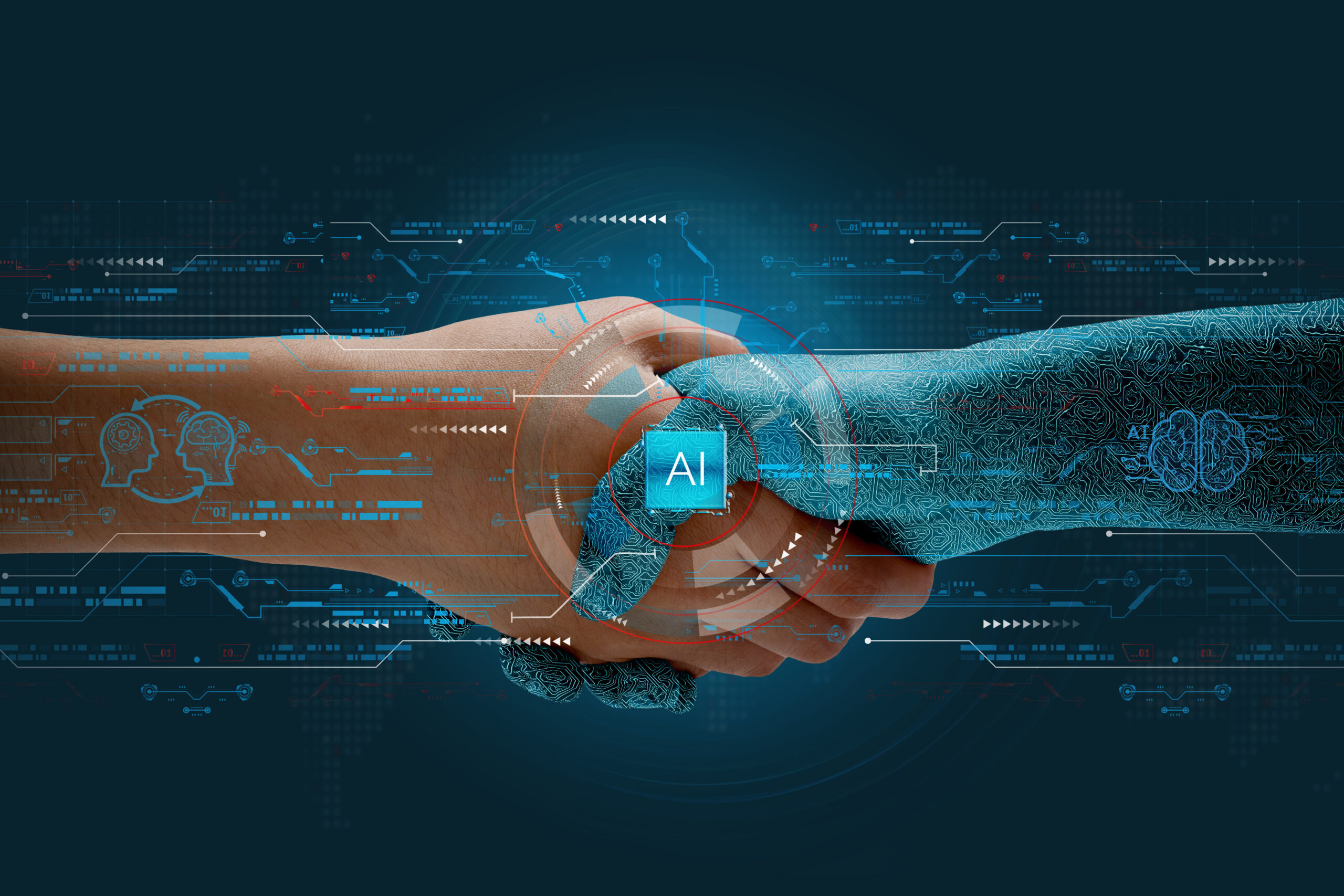
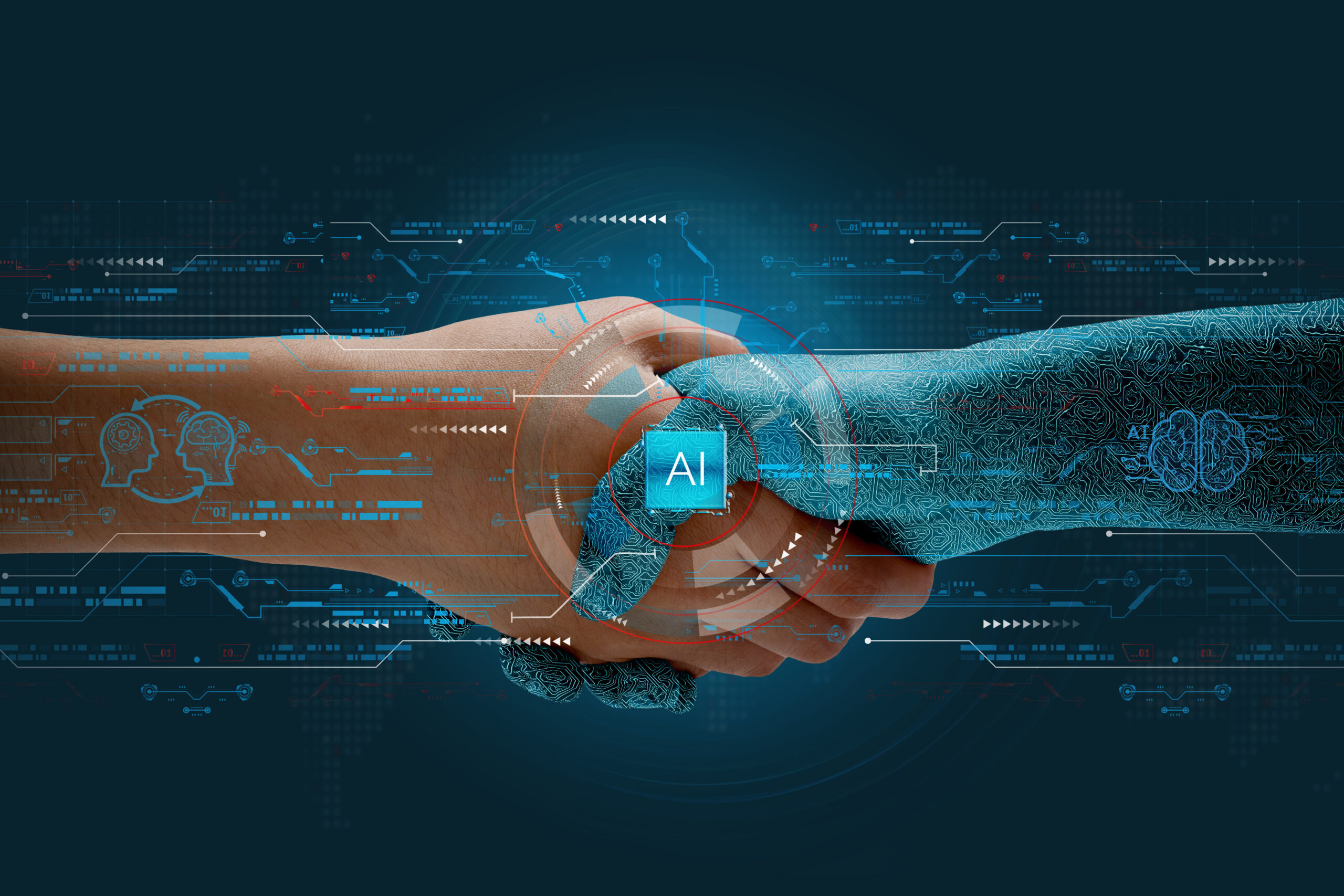
A human and robotic hand symbolizing collaboration with AI. – Via trueanthem.com
The evolution of human-AI collaboration relies heavily on transparency. Research from Microsoft has shown that prioritizing transparency in AI system design recognizes the importance of the people the technology is intended to serve. This transparency is crucial for respecting human agency and expertise while establishing the accountability necessary for effective collaboration.
Achieving meaningful AI transparency presents significant challenges. When AI systems make high-stakes decisions that impact human lives, understanding their reasoning becomes essential. Without clear explanations for AI decisions, human operators cannot accurately validate results or identify potential issues, creating a critical gap that undermines confidence and limits the potential for collaboration.
SmythOS addresses these fundamental challenges through its innovative approach to AI transparency. The platform’s visual builder democratizes AI development, enabling teams across various divisions to understand and modify AI workflows through an intuitive interface. This accessibility transforms AI from an opaque black box into a transparent, controllable tool that enhances human decision-making.
Most importantly, SmythOS’s built-in monitoring features provide unprecedented visibility into AI operations. They enable teams to track performance, identify potential issues, and optimize workflows in real-time. This level of transparency fosters the trust necessary for meaningful human-AI collaboration, all while maintaining enterprise-grade security controls that protect sensitive operations.
As we look toward the future of AI development, platforms like SmythOS that prioritize transparency while ensuring security and performance will be essential. By enabling humans to truly understand and effectively collaborate with AI systems, we move closer to a future where artificial and human intelligence work in genuine partnership, enhancing each other’s capabilities rather than replacing them.
Last updated:
Disclaimer: The information presented in this article is for general informational purposes only and is provided as is. While we strive to keep the content up-to-date and accurate, we make no representations or warranties of any kind, express or implied, about the completeness, accuracy, reliability, suitability, or availability of the information contained in this article.
Any reliance you place on such information is strictly at your own risk. We reserve the right to make additions, deletions, or modifications to the contents of this article at any time without prior notice.
In no event will we be liable for any loss or damage including without limitation, indirect or consequential loss or damage, or any loss or damage whatsoever arising from loss of data, profits, or any other loss not specified herein arising out of, or in connection with, the use of this article.
Despite our best efforts, this article may contain oversights, errors, or omissions. If you notice any inaccuracies or have concerns about the content, please report them through our content feedback form. Your input helps us maintain the quality and reliability of our information.