Enhancing Personalization Through Human-AI Collaboration
Software engineers working shoulder-to-shoulder with AI systems is rapidly transforming how we build technology today. A recent workshop with 22 professional developers revealed fascinating insights into how humans and AI can function as true collaborative partners rather than just user and tool.
As research from leading computer scientists demonstrates, the key to successful human-AI collaboration lies not just in the technology itself, but in how we structure these partnerships. When developers clearly define roles, maintain effective communication channels, and leverage each party’s unique strengths, the results can far exceed what either humans or AI could achieve alone.
This isn’t just about raw productivity gains. The most compelling aspect of human-AI collaboration is how it’s becoming increasingly personalized, with AI systems adapting to individual working styles and preferences. Imagine having an AI teammate that learns your coding patterns, anticipates your needs, and provides suggestions perfectly calibrated to your skill level and project requirements.
This transformative approach brings its own set of challenges. Questions around trust, oversight, and the balance of human judgment versus AI capabilities remain at the forefront of these partnerships. Innovative solutions are emerging to address these concerns while maximizing the potential of human-AI collaboration.
Through this article, we’ll examine the core components that make these partnerships work, dive into real-world applications reshaping software development, and look ahead to future developments that promise to make human-AI collaboration even more seamless and effective. Whether you’re a seasoned developer or just beginning to explore AI integration, understanding these dynamics is crucial for staying ahead in our rapidly evolving technical landscape.
Current Trends in Human-AI Collaboration
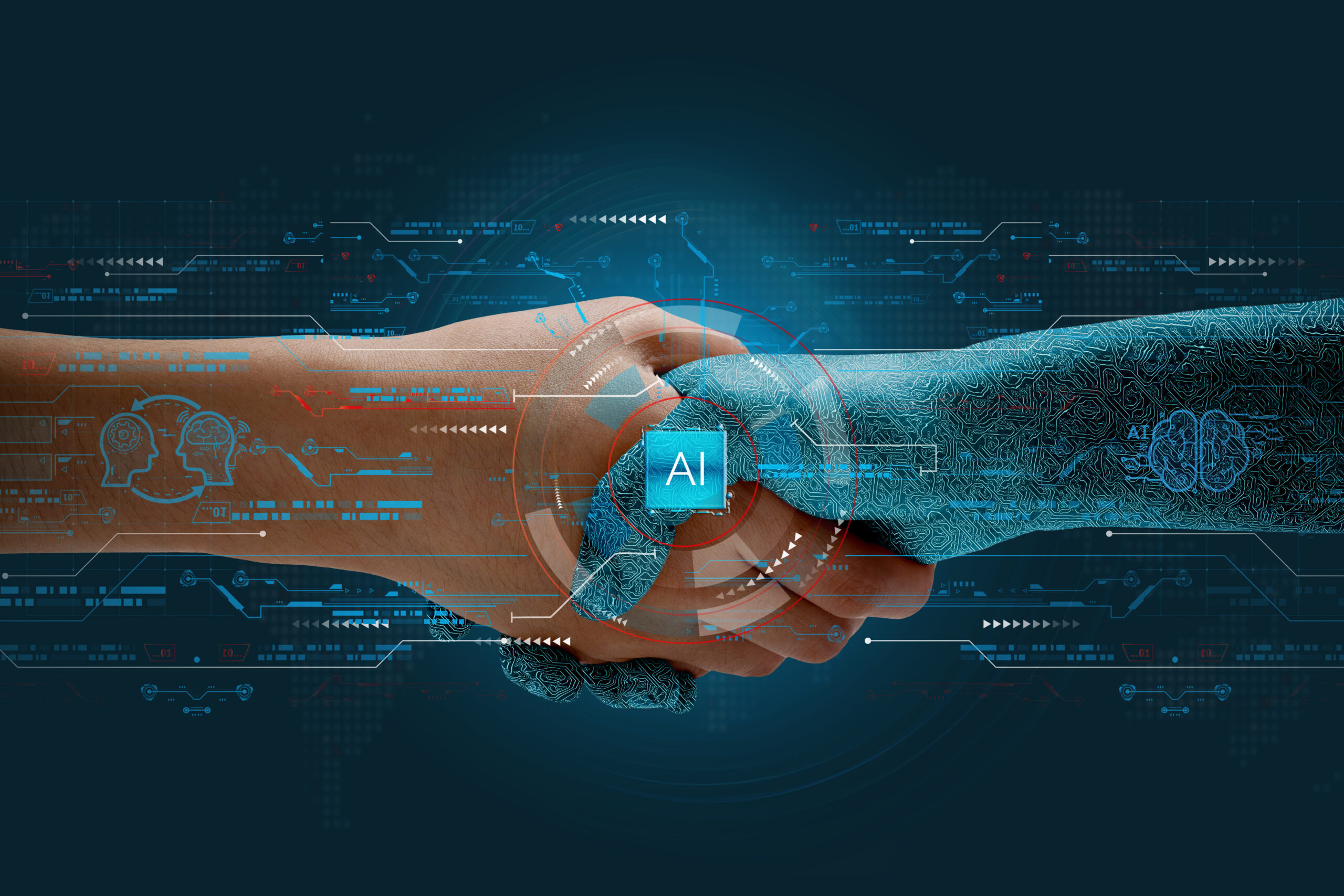
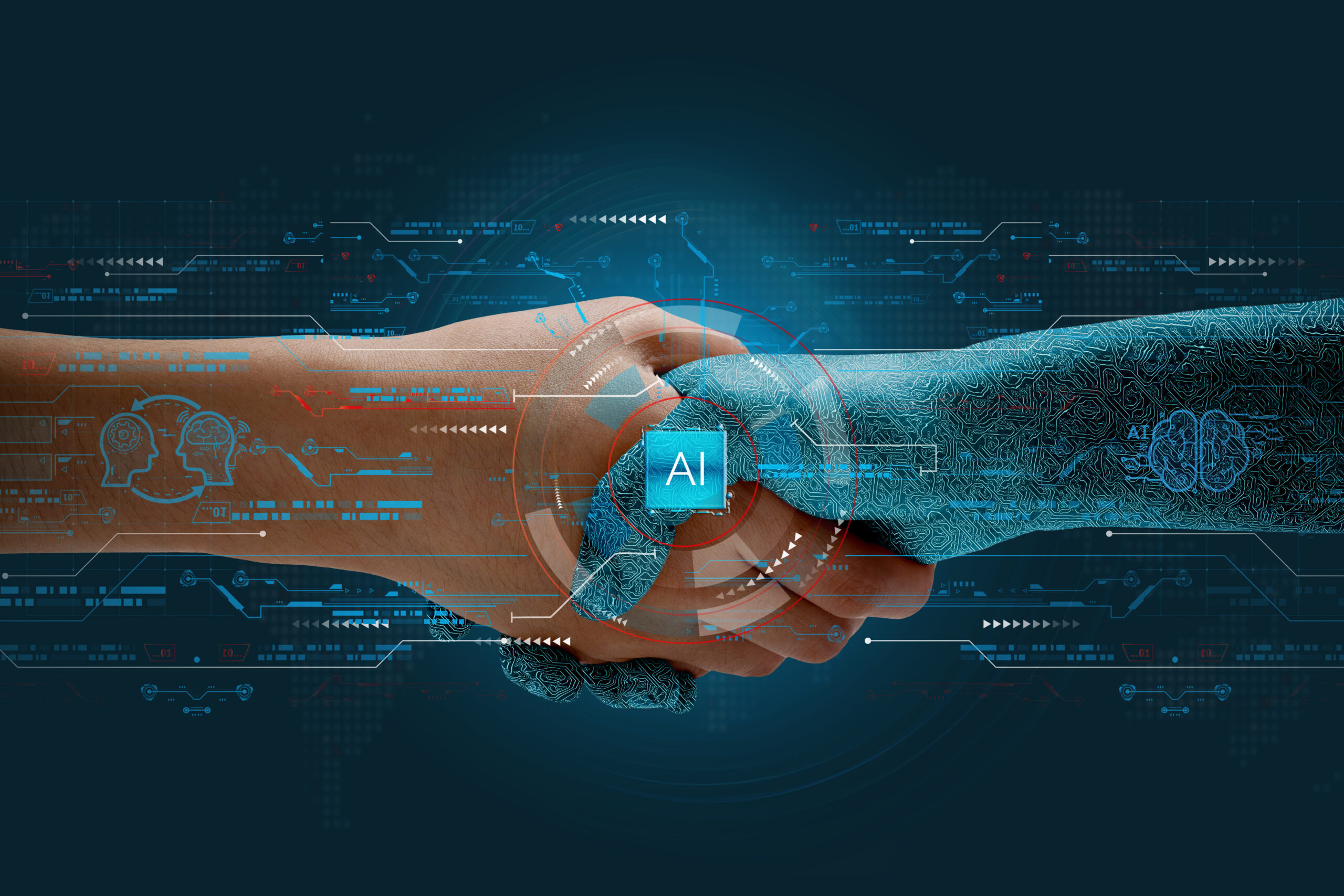
The evolution of human-AI collaboration has progressed beyond simple automation to create powerful partnerships between human expertise and artificial intelligence. Today’s AI platforms focus on augmenting human capabilities, leading to more efficient and effective workplace operations.
In financial services, AI systems like JPMorgan’s COiN program analyze complex financial documents and identify risk patterns, while human analysts concentrate on strategic decision-making and relationship management. This synergy has enhanced efficiency while preserving the human element in financial operations.
Healthcare is another area where human-AI collaboration is advancing. Medical professionals use AI to enhance diagnostic capabilities without replacing their judgment. For example, Johns Hopkins’ TREWS system helps doctors detect sepsis with 82% accuracy, supporting and amplifying human expertise in critical care.
In operational processes, AI transforms how teams manage routine tasks. Modern platforms handle data processing, analysis, and basic decision-making, allowing human workers to focus on strategic planning and creative problem-solving. This shift creates a dynamic workplace where AI manages repetitive tasks, and humans contribute unique insights and emotional intelligence.
Business leaders increasingly value ‘collaborative intelligence,’ where human creativity and AI capabilities combine to achieve outcomes neither could reach alone. Customer service operations now blend AI-powered chatbots for routine inquiries with human agents for complex problem-solving, enhancing efficiency and customer satisfaction.
However, the success of human-AI collaboration depends on thoughtful implementation. Organizations must integrate AI tools while maintaining human oversight and decision-making in critical areas. This balance ensures AI enhances rather than diminishes the human element in workplace operations.
Challenges in Human-AI Collaboration
The integration of AI systems into human workflows presents complex challenges that organizations must thoughtfully address. While AI offers tremendous potential for enhancing productivity and decision-making capabilities, several critical obstacles need careful consideration to ensure successful collaboration between humans and artificial intelligence.
Bias in AI training data emerges as one of the most pressing concerns. As highlighted in recent research by EY, AI systems can perpetuate and amplify existing societal biases. For instance, image generation systems have shown concerning patterns – depicting certain professions predominantly with specific gender or racial characteristics. This bias doesn’t just affect image generation; it extends to critical decisions in hiring, lending, and other crucial business processes.
The challenge of system integration poses another significant hurdle. Many organizations struggle to seamlessly incorporate AI tools into their existing technological infrastructure and workflows. This isn’t simply a technical challenge – it’s about ensuring that AI systems can effectively communicate with legacy systems while maintaining data integrity and security. The complexity increases when considering the need to preserve institutional knowledge and processes while adopting new AI capabilities.
AI hallucinations – instances where AI systems generate inaccurate or fabricated information – represent another significant challenge in human-AI collaboration. These inaccuracies can undermine trust and potentially lead to costly mistakes in business operations. Organizations must implement robust verification processes and maintain human oversight to catch and correct these errors before they impact critical decisions.
The “black box” nature of many AI systems creates additional complications. When AI makes decisions or recommendations, the reasoning behind these choices isn’t always clear or explainable to human users. This lack of transparency can create resistance among workers who need to understand and trust the systems they’re working with. Organizations must balance the power of sophisticated AI algorithms with the need for interpretability and accountability.
Addressing these challenges requires a multifaceted approach that combines technical solutions with thoughtful organizational policies. Companies must invest in diverse training data, implement robust testing frameworks, and establish clear governance structures. Most importantly, organizations need to recognize that effective human-AI collaboration isn’t about replacing human judgment – it’s about augmenting it with AI capabilities while maintaining human oversight and ethical considerations at the forefront.
The Role of Personalization in AI
AI systems are transforming how we interact with technology by delivering increasingly personalized experiences that adapt to our individual needs and preferences. Through sophisticated algorithms and machine learning capabilities, AI platforms can now understand and respond to users in ways that feel remarkably human-like and contextually relevant.
AI personalization works by analyzing vast amounts of user data in real-time, including demographics, behavior patterns, and interaction history. As IBM researchers note, this enables AI systems to gain valuable insights into individual preferences and deliver tailored experiences across various touchpoints throughout the customer journey.
One of the key aspects of AI personalization is natural language processing (NLP), which allows systems to understand the nuances and context of human communication. AI-powered chatbots and virtual assistants leverage NLP to provide personalized responses that go beyond simple pre-programmed answers, creating more meaningful and engaging interactions with users.
The technology also excels at predictive personalization, anticipating user needs before they’re explicitly expressed. For instance, AI systems can analyze past behaviors and preferences to proactively suggest relevant content, products, or solutions. This predictive capability helps streamline user experiences while increasing engagement and satisfaction.
Machine learning algorithms continuously refine these personalization efforts by learning from each interaction. The more a user engages with an AI system, the better it becomes at understanding their unique preferences and adapting its responses accordingly. This creates a reinforcing cycle of increasingly personalized experiences.
Benefit | Description |
---|---|
Enhanced Customer Engagement | Delivering personalized content, product recommendations, and offers captures customers’ attention and keeps them engaged. |
Increased Conversion Rates | AI personalization presents the right products or services to the right customers at the right time, increasing the likelihood of conversion and driving revenue growth. |
Improved Customer Retention | Personalized experiences create a strong emotional connection between customers and brands, fostering loyalty and repeat purchases. |
Optimized Marketing Efforts | AI enables businesses to target marketing campaigns more effectively, yielding better results and higher ROI. |
Reduction in Customer Acquisition Costs | Personalization programs can reduce customer acquisition costs by as much as 50%. |
Increased Revenue | Fast-growing organizations drive 40% more revenue from personalization than their more slowly moving counterparts. |
Competitive Advantage | Providing personalized experiences based on customer preferences offers a significant competitive advantage. |
Insights into Customer Behavior | AI personalization surfaces granular data about users, allowing businesses to gain insights into current and future customer behaviors. |
We’ve entered an era where AI personalization can reduce customer acquisition costs by 50% while increasing revenue by 5-15% and making marketing spending 10-30% more efficient.
McKinsey Study
The impact of AI personalization extends beyond just improving user experience. Organizations implementing these technologies are seeing significant business benefits, including higher engagement rates, improved customer retention, and more efficient resource allocation. By understanding and catering to individual user states, skills, and preferences, AI systems are creating more meaningful and productive human-machine interactions.
Successfully Implementing Collaborative Intelligence
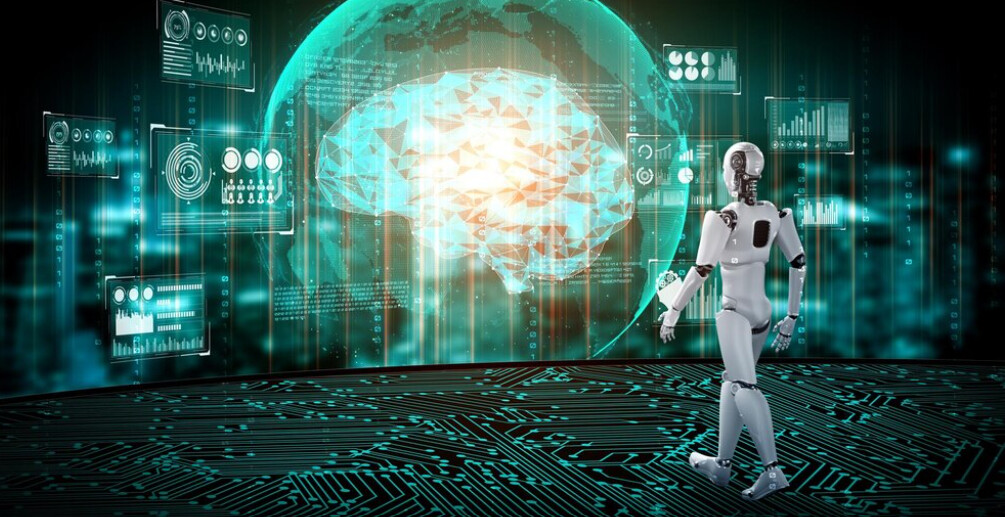
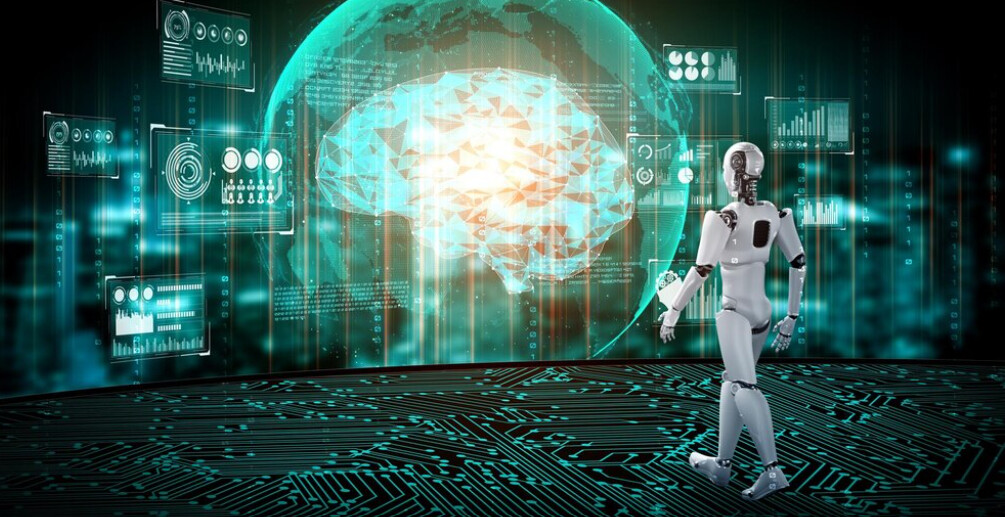
AI collaboration with a humanoid robot and holographic brain. – Via livesarkariyojana.com
The future of work isn’t about humans versus AI—it’s about humans and AI working together as collaborative partners. Organizations that effectively implement collaborative intelligence consistently outperform those focused solely on automation, according to research by Harvard Business Review examining 1,500 companies.
Successfully deploying collaborative intelligence requires a thoughtful, systematic approach focused on augmenting human capabilities rather than replacing them. The key is redesigning business processes to leverage the complementary strengths of both human and AI agents.
Reimagining Business Processes
The first step is conducting a thorough assessment of existing workflows and processes. Leaders need to identify specific areas where AI can enhance human work—whether that’s increasing operational flexibility, improving decision-making capabilities, or enabling greater personalization of products and services.
Rather than simply automating isolated tasks, organizations should take a holistic view. This means examining end-to-end processes and understanding how humans and AI can collaborate throughout the entire workflow. The goal is creating adaptive processes that evolve as AI capabilities advance.
For example, in customer service, AI can handle routine inquiries and data gathering, while human agents focus on complex problem-solving and emotional support. This division of labor plays to the strengths of both human and machine intelligence.
Successful reimagining requires transparent communication about how AI will be integrated. Teams need clarity on task allocation, processes, and expected outcomes involving AI collaboration.
Leaders should start small, testing collaborative approaches on specific projects before scaling more broadly. This allows organizations to refine their approach based on real-world feedback.
Developing Human-AI Interface Skills
For collaborative intelligence to succeed, organizations must invest in developing their workforce’s ability to work effectively with AI systems. This goes beyond basic technical training.
People need to understand how to train AI agents, interpret their outputs, and ensure responsible use. According to research, ten core competencies underpin successful human-AI collaboration, including active listening, clear communication, and the ability to guide productive conversations.
Critical skills include knowing when to rely on AI insights versus human judgment, how to effectively delegate tasks between human and machine agents, and maintaining awareness of AI systems’ limitations.
Organizations should create opportunities for hands-on practice working alongside AI tools in low-stakes environments. This builds comfort and competence with human-machine collaboration.
Measuring and Refining Collaborative Success
Implementing collaborative intelligence should be viewed as an iterative process of continuous improvement. Organizations need clear metrics to evaluate the effectiveness of human-AI partnerships.
Focus on measuring both quantitative outcomes (productivity gains, error reduction) and qualitative factors (employee engagement, job satisfaction). Regular feedback from human team members is essential for identifying friction points and opportunities for optimization.
Leaders should recognize and reward successful examples of human-AI collaboration. This helps create a culture where employees feel empowered to experiment with new collaborative approaches rather than viewing AI as a threat.
The most successful organizations maintain flexibility in their implementation approach, regularly reassessing and adjusting how humans and AI work together based on evolving capabilities and needs.
Future Directions of Human-AI Collaboration
The relationship between humans and machines is set for unprecedented transformation with the evolution of AI. Recent developments in multimodal AI systems, capable of processing text, images, and audio simultaneously, are reshaping our interactions with artificial intelligence. These advances promise more intuitive and meaningful exchanges between humans and AI systems.
One of the most significant shifts on the horizon is the emergence of AI agents that can communicate not just with humans, but with other AI agents. As reported by industry experts, these specialized agents will collaborate to handle complex tasks, from customer support to manufacturing operations, creating an interconnected ecosystem of AI assistants working in harmony.
Personalization stands at the forefront of future AI developments. Rather than one-size-fits-all solutions, AI systems are evolving to adapt to individual user preferences, learning styles, and specific needs. This shift towards personalized AI interactions will fundamentally change how we work, learn, and solve problems, making technology more accessible and effective for everyone.
The biggest impact will come from material sciences and genomics. AI models have already been used to make breakthroughs in material sciences, discovering new crystal structures driving better batteries to more efficient computers.
AI is expected to become more deeply integrated into our daily lives through wearable technology and extended reality (XR) devices. These innovations will create seamless bridges between digital and physical worlds, offering real-time AI assistance and augmented experiences that enhance human capabilities rather than replace them.
The future of human-AI collaboration also brings important considerations about digital identity and privacy. As our interactions with AI systems become more personalized and frequent, the need for secure, portable digital identities becomes paramount. This evolution will require careful balancing of convenience with robust security measures to protect user data and maintain trust in AI systems.
Conclusion on Enhancing Human-AI Collaboration
Effective human-AI collaboration requires thoughtful solutions to current challenges and embracing personalization as a core principle. As organizations implement increasingly complex AI systems, robust tools and frameworks become critical.
Personalization techniques are essential in bridging the gap between human capabilities and AI systems. Adaptive response systems and sophisticated monitoring tools help create intuitive, context-aware collaborative environments that enhance both human and machine performance.
Data privacy and system integration challenges necessitate careful consideration and strategic planning. Research shows that human-AI collaboration is often less effective than AI systems alone, highlighting the importance of selecting the right tools and frameworks for these partnerships.
SmythOS offers a powerful solution, providing developers with comprehensive tools for building and deploying autonomous AI systems. Its visual debugging environment and built-in monitoring capabilities enable teams to create sophisticated collaborative frameworks while maintaining transparency and control over AI operations.
Future success in human-AI collaboration depends on addressing current limitations and embracing tools that facilitate natural interaction between humans and machines. The focus should be on creating systems that augment human capabilities rather than replace them, ensuring collaboration remains effective and ethically sound.
Last updated:
Disclaimer: The information presented in this article is for general informational purposes only and is provided as is. While we strive to keep the content up-to-date and accurate, we make no representations or warranties of any kind, express or implied, about the completeness, accuracy, reliability, suitability, or availability of the information contained in this article.
Any reliance you place on such information is strictly at your own risk. We reserve the right to make additions, deletions, or modifications to the contents of this article at any time without prior notice.
In no event will we be liable for any loss or damage including without limitation, indirect or consequential loss or damage, or any loss or damage whatsoever arising from loss of data, profits, or any other loss not specified herein arising out of, or in connection with, the use of this article.
Despite our best efforts, this article may contain oversights, errors, or omissions. If you notice any inaccuracies or have concerns about the content, please report them through our content feedback form. Your input helps us maintain the quality and reliability of our information.