AI Chips: Understanding the Backbone of Modern AI
Imagine a world where machines can think, learn, and process information at lightning speed. This isn’t science fiction—it’s the reality of AI chips, the unsung heroes powering the artificial intelligence revolution. But what exactly are these technological marvels, and why do they matter?
AI chips are the specialized brains behind today’s most advanced AI systems. Unlike traditional processors, these powerhouses are custom-built to handle the complex computations that make artificial intelligence possible. They’re the reason your smartphone can recognize your face, and why autonomous vehicles can navigate city streets.
At their core, AI chips are all about speed and efficiency. They crunch numbers faster than you can blink, enabling AI models to process vast amounts of data in real-time. This breakneck pace isn’t just impressive—it’s essential for applications like natural language processing, image recognition, and predictive analytics.
But speed isn’t the only trick up their sleeve. AI chips are masters of multitasking, thanks to their parallel processing capabilities. Imagine a chef with a hundred hands, each preparing a different part of a meal simultaneously. That’s how AI chips tackle complex problems, breaking them down into smaller tasks and solving them all at once.
Energy efficiency is another feather in their cap. As HONOR UK points out, AI chips can perform more calculations per watt than their general-purpose counterparts. This means more processing power without draining your device’s battery or racking up massive energy bills in data centers.
This article will explore the fascinating world of AI chips. We’ll dive into the different types of AI chips, from GPUs to ASICs, and see how they’re reshaping industries from healthcare to finance. We’ll also look at the challenges facing AI chip development and peek into the crystal ball to see what the future might hold.
Get ready to discover how these tiny marvels are changing the world as we know it.
Different Types of AI Chips
As artificial intelligence (AI) reshapes industries, the hardware powering these innovations has become increasingly specialized. Here are the main types of AI chips and how they transform machine learning and data processing.
Graphics Processing Units (GPUs)
Originally designed for rendering video games, GPUs excel at parallel processing, making them ideal for training complex AI models.
Imagine painting a thousand canvases simultaneously—that’s what GPUs do for AI. They process massive amounts of data in parallel, speeding up the learning process for neural networks.
Companies like NVIDIA lead with their A100 GPUs, capable of training language models and computer vision systems at unprecedented speeds.
Field-Programmable Gate Arrays (FPGAs)
FPGAs are the chameleons of the AI chip world. These versatile chips can be reprogrammed on the fly, adapting to different AI tasks as needed.
Think of FPGAs as building blocks that can be rearranged to create different structures. This flexibility is valuable for companies experimenting with various AI applications or needing frequent system updates.
Microsoft has leveraged FPGAs in their Azure cloud services to accelerate AI workloads while maintaining adaptability for evolving algorithms.
Application-Specific Integrated Circuits (ASICs)
ASICs are the specialists of AI chips. Designed for one task, they offer unparalleled efficiency for specific AI applications.
Imagine a chef’s knife crafted solely for slicing sushi—that’s an ASIC. It may not be versatile, but it excels at its intended purpose.
Google’s Tensor Processing Units (TPUs) are a prime example of ASICs, optimized for the TensorFlow deep learning framework and delivering exceptional performance for certain AI workloads.
The right chip can make all the difference in AI performance. GPUs for training, FPGAs for flexibility, ASICs for specialization—each has its role in powering the AI revolution.
As AI evolves, so will the chips that power it. The race to develop faster, more efficient AI hardware is shaping the future of technology, from smartphones to supercomputers. By understanding these different types of AI chips, we gain insight into the incredible computational power driving today’s AI breakthroughs.
Applications and Benefits of AI Chips
AI chips are transforming artificial intelligence, powering applications that were once science fiction. From large language models to autonomous vehicles, these specialized processors are at the heart of significant technological advancements. Here are some key applications where AI chips are making an impact:
Accelerating Large Language Models
AI chips play a crucial role in accelerating large language models (LLMs) like GPT-3 and its successors. These models require immense computational power to process and generate human-like text. According to Jamie Shotton, chief scientist at Wayve, “The use of large language models will be revolutionary for autonomous driving technology.” By leveraging AI chips, researchers and developers can train and run these models more efficiently, enabling breakthroughs in natural language understanding, translation, and generation. This has implications for industries ranging from customer service to content creation.
Edge AI
Edge AI is another frontier where AI chips are making waves. By processing data locally on devices rather than in the cloud, edge AI enables faster response times and enhanced privacy. AI chips for edge computing allow smartphones, IoT devices, and industrial equipment to run complex AI algorithms without constant connectivity to centralized servers. Imagine a smart home device that can understand and respond to voice commands instantly, or a manufacturing robot that can make split-second decisions based on real-time sensor data. These are the kinds of applications that edge AI chips are making possible.
Autonomous Vehicles
The dream of self-driving cars is becoming a reality, thanks in large part to AI chips. These processors enable autonomous vehicles to interpret vast amounts of sensor data in real-time, make complex decisions, and navigate safely through unpredictable environments. AI chips in autonomous vehicles are not just about processing power; they are about doing so with incredible energy efficiency. This is crucial for electric vehicles, where every watt of power needs to be optimized for range and performance.
Robotics
In robotics, AI chips enable machines to perceive their environment, make decisions, and interact with humans in increasingly sophisticated ways. From warehouse automation to humanoid robots, these processors are the brains behind the next generation of intelligent machines.
Benefits of AI Chips
The benefits of AI chips extend far beyond their specific applications. Here are some key advantages they offer:
AI chips are designed to handle the unique computational demands of artificial intelligence algorithms. Their architecture allows for massive parallelism, enabling them to perform complex calculations much faster than traditional CPUs. This enhanced processing power doesn’t just mean faster results; it opens up new possibilities. Tasks that were once computationally infeasible can now be tackled with AI chips, pushing the boundaries of what’s possible in AI research and applications.
One of the most significant benefits of AI chips is their energy efficiency. As AI workloads grow, the energy consumption of data centers and devices has become a pressing concern. AI chips address this by delivering more performance per watt than general-purpose processors. According to NVIDIA, “By transitioning from CPU-only operations to GPU-accelerated systems, HPC and AI workloads can save over 40 terawatt-hours of energy annually, equivalent to the electricity needs of nearly 5 million U.S. homes.” This efficiency gain reduces operational costs and contributes to sustainability efforts in the tech industry.
In many AI applications, milliseconds matter. Whether it’s an autonomous vehicle making a split-second decision to avoid a collision or a financial algorithm executing trades, the ability to perform complex AI tasks in real-time can be a matter of life and death—or millions of dollars. AI chips excel at delivering this real-time performance, processing vast amounts of data and making decisions faster than the blink of an eye. This capability is pushing the boundaries of what’s possible in fields like augmented reality, robotics, and smart city infrastructure.
As we look to the future, the importance of AI chips in driving technological progress cannot be overstated. They are the unsung heroes powering the AI revolution, enabling innovations that are reshaping our world in profound ways. From the smartphones in our pockets to the cars we drive (or that drive us), AI chips are quietly transforming our daily lives, one computation at a time.
Challenges in AI Chip Development
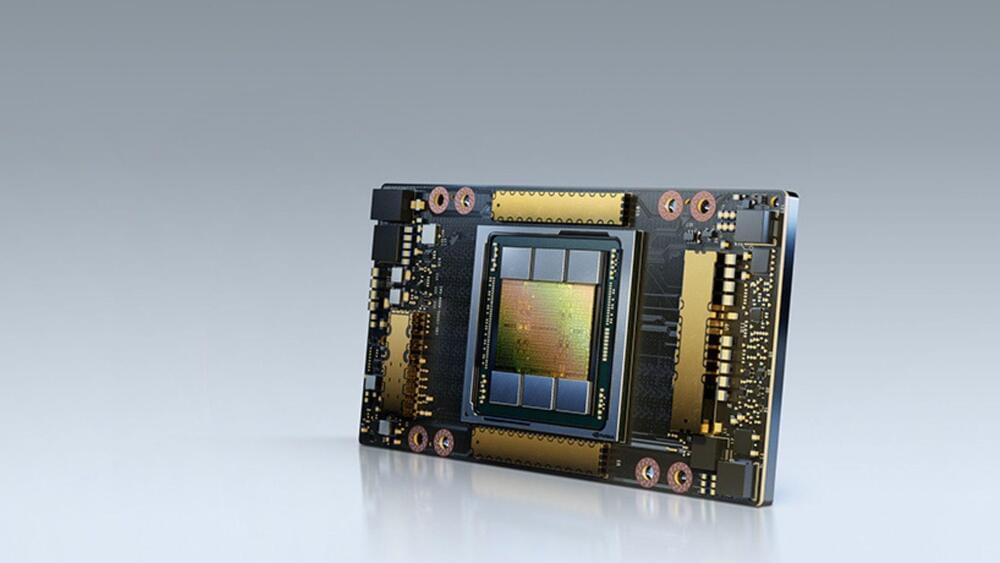
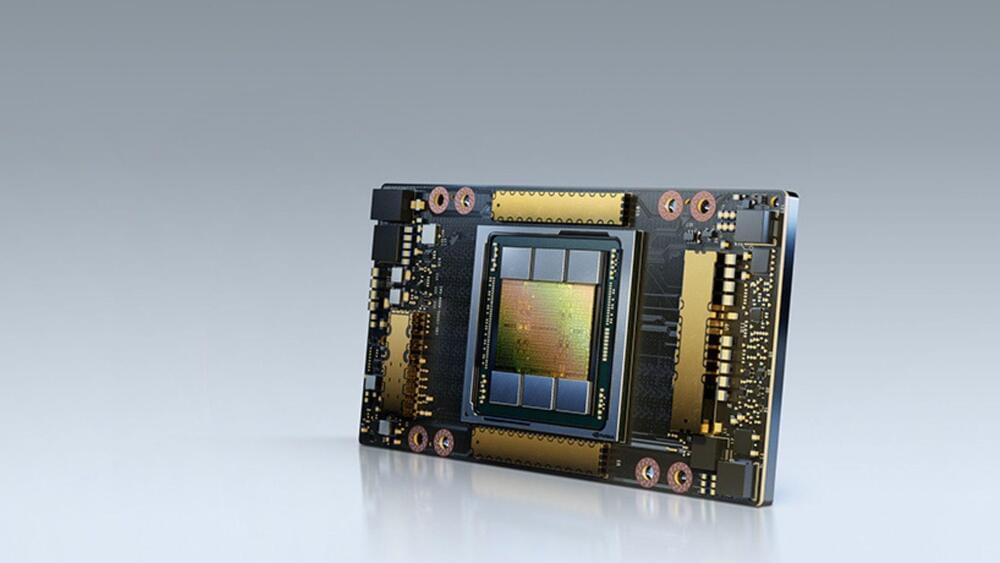
The rapid advancement of artificial intelligence (AI) technologies has placed unprecedented demands on the semiconductor industry, particularly in the development of specialized AI chips. However, this progress faces significant hurdles that threaten to slow the pace of innovation and deployment of AI systems worldwide.
Supply chain bottlenecks stand out as a critical challenge in AI chip development. The crushing demand for AI has revealed the limits of the global supply chain for powerful chips used to develop and field AI models. This surge in demand has overwhelmed the few sources of supply, creating a scarcity of high-end GPUs essential for advanced AI work.
Compounding the supply issue, GPU manufacturers themselves face shortages of crucial components. Sid Sheth, founder and CEO of AI startup d-Matrix, points out that silicon interposers – a key technology for marrying computing chips with high-bandwidth memory – are in short supply, further constraining production capacity.
Computational Constraints
Beyond supply chain issues, AI chip development grapples with significant computational constraints. The insatiable appetite for computing power in AI applications pushes current chip designs to their limits, necessitating continuous innovation in chip architecture and manufacturing processes.
These constraints are particularly evident in the development of large language models (LLMs) and other advanced AI systems. As models grow in complexity, they demand ever-increasing computational resources, challenging chip designers to create more efficient and powerful processors.
Year | Manufacturer | Market Share (%) |
---|---|---|
2023 | Nvidia | 84% |
2023 | AMD | 16% |
2023 | Intel | ~0% |
The race to overcome these constraints has led to a concentration of power in the hands of a few leading companies. Nvidia, for instance, controls an estimated 84% of the market for discrete GPUs, highlighting both the intensity of competition and the technological barriers to entry in this field.
Geopolitical Issues
Perhaps the most complex challenge facing AI chip development is the growing impact of geopolitical tensions. As countries compete to secure the economic, political, and military advantages of AI, businesses seeking to develop or deploy AI chips must navigate increasing regulatory complexity and possible geopolitical bottlenecks in the AI value chain.
The United States has implemented sweeping measures targeting China’s semiconductor sector, restricting the export of chipmaking equipment and high-bandwidth memory. These actions have sparked concerns over potential supply chain disruptions and intensified the global scramble for AI supremacy.
Such geopolitical maneuvering has far-reaching implications. It forces companies to reconsider their global supply chains, potentially leading to the regionalization of chip production and a fragmentation of the global AI ecosystem. This could result in increased costs, reduced efficiency, and slower innovation in AI chip development.
The Path Forward
Addressing these challenges requires a multifaceted approach. Investments in domestic chip manufacturing capacity, such as those encouraged by the CHIPS Act in the United States, aim to alleviate supply chain vulnerabilities. However, these solutions take time to implement and may not fully address the global nature of the semiconductor industry.
In the meantime, companies are exploring creative solutions to mitigate the impact of chip shortages. This includes developing smaller AI models that are less computationally intensive and exploring new computational methods that reduce reliance on traditional CPUs and GPUs.
As the AI chip landscape continues to evolve, collaboration between industry, government, and academia will be crucial in overcoming these challenges. Only through concerted efforts can we ensure the continued advancement of AI technologies while navigating the complex web of supply chain, computational, and geopolitical issues that define the current state of AI chip development.
Future Directions of AI Chips
Artificial intelligence is on the brink of a transformation, driven by advancements in AI chip technology. The future of AI chips gleams with promise, offering glimpses of unprecedented power and efficiency.
At the forefront of this evolution stands in-memory computing, a paradigm shift redefining the architecture of AI chips. This approach integrates computation directly within memory units, dramatically reducing the energy-intensive task of shuttling data between separate processing and storage components.
Imagine AI systems that operate at lightning speeds while consuming minimal energy. Researchers at institutions like Princeton University are working to realize this vision, developing chips that promise to slash power consumption by up to 25 times compared to current models.
The Rise of Specialized Architectures
As AI applications diversify, so do the chips that power them. We are witnessing the emergence of highly specialized chip architectures, each tailored to excel at specific AI tasks. These designs represent a fundamental rethinking of how we approach computation for AI.
Neuromorphic chips mimic the structure and function of the human brain, promising to bring us closer to AI systems that can learn and adapt with the fluidity and efficiency of biological neural networks.
AI Chip Type | Key Features | Applications |
---|---|---|
GPUs | High parallel processing capabilities | Training complex AI models, language models, computer vision |
FPGAs | Reprogrammable, adaptable to different tasks | Experimentation with AI applications, cloud services |
ASICs | Highly efficient for specific tasks | Deep learning frameworks, specialized AI workloads |
TPUs | Optimized for TensorFlow | Deep learning, high-performance AI tasks |
NPUs | Neural network acceleration, inference optimization | Facial recognition, real-time AI processing in mobile and edge devices |
Quantum computing looms on the horizon, offering the potential to solve complex AI problems that would be intractable for classical computers. While still in its infancy, quantum AI chips could one day revolutionize fields like drug discovery and climate modeling.
Overcoming Current Limitations
The road to the future is not without its challenges. Current AI chips face limitations in terms of power consumption, heat generation, and scalability. However, researchers are tackling these hurdles head-on with innovative solutions that push the boundaries of materials science and chip design.
Exploration of new materials beyond traditional silicon, such as graphene and other two-dimensional materials, offers the possibility of creating chips that are more powerful, energy-efficient, and heat-resistant.
Advancements in 3D chip stacking and packaging are allowing for denser, more capable AI processors. This approach not only increases computational power but also improves energy efficiency by reducing the distance data needs to travel.
The Impact on AI Applications
The ripple effects of these advancements in AI chip technology will be felt across a wide spectrum of applications. From enhancing the capabilities of edge devices to powering massive language models, the next generation of AI chips will enable more sophisticated and responsive AI systems.
Imagine autonomous vehicles with onboard AI that can process sensor data in real-time with unparalleled accuracy. Or consider AI-powered medical devices that can analyze complex biological data instantaneously, potentially revolutionizing diagnostics and treatment planning.
The future of AI chips is not just about raw computational power; it is about creating smarter, more efficient systems that can bring the benefits of AI to every corner of our lives. As these technologies mature, we can expect to see AI capabilities that were once confined to massive data centers making their way into our smartphones, wearables, and even everyday appliances.
A Collaborative Future
The journey towards the next generation of AI chips is a collaborative effort, bringing together experts from diverse fields such as computer science, materials engineering, and neuroscience. This interdisciplinary approach is crucial for overcoming the complex challenges that lie ahead.
The future is bright, and the possibilities are boundless. The innovations we are seeing today are laying the foundation for AI systems that are not just more powerful, but also more accessible, efficient, and integrated into our daily lives than ever before.
The race to develop the AI chips of tomorrow is more than just a technological competition; it is a quest to unlock new realms of human knowledge and capability. As these chips evolve, they will enable AI to tackle some of the most pressing challenges facing humanity, from climate change to healthcare. The future of AI chips is, in many ways, the future of human progress itself.
Leveraging SmythOS for AI Chip Integration
Staying productive can be challenging. However, with the right strategies, you can significantly improve your efficiency. Organize your workspace, eliminate distractions, and prioritize tasks. Additionally, taking regular breaks and staying hydrated can enhance your focus. Remember, productivity isn’t about working harder, but working smarter.
SmythOS stands at the forefront of AI chip integration, offering a comprehensive platform that simplifies the complex process of incorporating artificial intelligence into various applications. This innovative system provides developers and enterprises with powerful tools to harness the full potential of AI chips, streamlining development and deployment.
At the heart of SmythOS’s offering is its intuitive visual builder. This feature empowers developers to create sophisticated AI workflows without diving deep into code. By providing a drag-and-drop interface, SmythOS democratizes AI development, allowing even those without extensive programming backgrounds to design and implement AI solutions that leverage specialized chips.
Debugging AI applications can be a daunting task, but SmythOS rises to the challenge with its built-in debugging tools. These instruments offer real-time insights into AI processes, enabling developers to identify and resolve issues swiftly. The ability to troubleshoot efficiently not only accelerates development timelines but also ensures the reliability of AI-driven applications in production environments.
In an era where data security is paramount, SmythOS doesn’t cut corners. The platform boasts enterprise-grade security measures, safeguarding sensitive information and intellectual property. This robust security framework instills confidence in organizations looking to integrate AI chips into their critical applications without compromising on data protection.
SmythOS isn’t just a tool; it’s a catalyst for innovation. It transforms the daunting task of AI agent development into an intuitive, visual experience that anyone can master.
Alexander De Ridder, Co-Founder and CTO of SmythOS
By leveraging SmythOS, organizations can significantly reduce the time and resources typically required for AI chip integration. The platform’s comprehensive approach addresses key challenges in AI development, from conceptualization to deployment, making it an invaluable asset for businesses looking to stay competitive in the AI-driven marketplace.
SmythOS’s support for AI chip integration extends beyond mere compatibility. The platform is designed to optimize performance, ensuring that AI applications can fully capitalize on the processing power of specialized hardware. This optimization translates to faster execution times and more efficient resource utilization, critical factors in scaling AI solutions.
For enterprises venturing into AI chip integration, SmythOS offers a clear path forward. Its user-friendly interface, coupled with powerful backend capabilities, strikes a balance between accessibility and sophistication. This approach not only accelerates the adoption of AI technologies but also fosters innovation by lowering the barriers to entry for AI development.
As the AI landscape continues to evolve, SmythOS remains committed to staying ahead of the curve. The platform’s adaptability ensures that it can incorporate new advancements in AI chip technology, providing users with a future-proof solution for their AI integration needs. This forward-thinking approach positions SmythOS as a long-term partner in an organization’s AI journey, rather than just a temporary solution.
Conclusion and Future of AI Chips
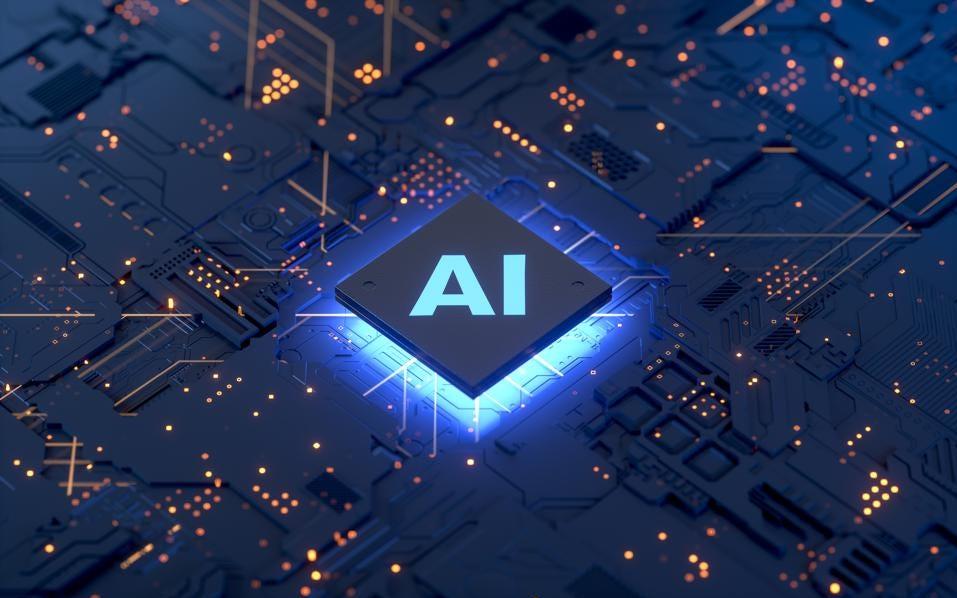
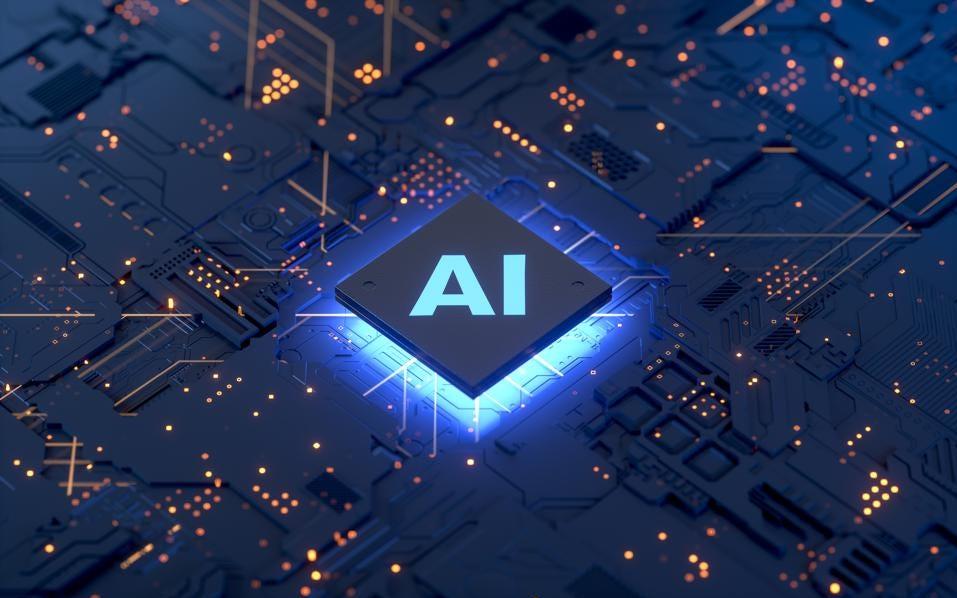
A glowing AI chip on an intricate circuit board. – Via icdrex.com
AI chips are the unsung heroes propelling us forward in this new technological era. These tiny engineering marvels are the heart of artificial intelligence, driving innovations that once existed only in science fiction.
The journey of AI chip development has been remarkable. From the early days of CPUs to the parallel processing prowess of GPUs, and now to specialized AI accelerators, we have seen a relentless pursuit of computational power and efficiency. This evolution has enabled breakthroughs in machine learning, natural language processing, and computer vision that are reshaping industries and our daily lives.
However, the path ahead is challenging. As AI models grow in complexity and size, chip designers must balance performance with energy efficiency. Recent advancements, such as Nvidia’s Blackwell GPU, showcase the industry’s commitment to overcoming these hurdles. The quest for more powerful and efficient AI chips continues, driving researchers to explore novel architectures and materials.
Looking to the future, the potential of AI chips seems boundless. We’re on the brink of AI-driven scientific discoveries, autonomous systems navigating complex scenarios, and personalized AI assistants understanding context and nuance. Realizing these possibilities hinges on pushing the boundaries of chip design and manufacturing.
Platforms like SmythOS play a crucial role in this landscape. By providing tools that simplify AI development and deployment, SmythOS empowers organizations to harness the full potential of AI chips. Its intuitive interface and robust capabilities bridge the gap between cutting-edge hardware and practical applications, accelerating innovation across industries.
In conclusion, AI chips are not just facilitating technological progress; they are reshaping our technological future. The challenges are formidable, but so is the collective ingenuity of researchers, engineers, and innovators worldwide. With each breakthrough in AI chip technology, we move closer to a world where artificial intelligence enhances human capability in ways we are only beginning to imagine.
Last updated:
Disclaimer: The information presented in this article is for general informational purposes only and is provided as is. While we strive to keep the content up-to-date and accurate, we make no representations or warranties of any kind, express or implied, about the completeness, accuracy, reliability, suitability, or availability of the information contained in this article.
Any reliance you place on such information is strictly at your own risk. We reserve the right to make additions, deletions, or modifications to the contents of this article at any time without prior notice.
In no event will we be liable for any loss or damage including without limitation, indirect or consequential loss or damage, or any loss or damage whatsoever arising from loss of data, profits, or any other loss not specified herein arising out of, or in connection with, the use of this article.
Despite our best efforts, this article may contain oversights, errors, or omissions. If you notice any inaccuracies or have concerns about the content, please report them through our content feedback form. Your input helps us maintain the quality and reliability of our information.