Explainable AI Regulations: Navigating Legal and Ethical Standards for Transparent AI Systems
Artificial intelligence increasingly shapes critical decisions affecting our lives, making regulations around Explainable AI (XAI) a crucial framework for ensuring transparency and accountability in AI systems. Imagine an AI determining your loan application or job candidacy—wouldn’t you want to understand how it reached its decision?
The push for explainable AI isn’t just about satisfying curiosity. As AI systems become more complex and widespread, their ability to communicate their decision-making processes to users has become paramount for building trust and ensuring responsible deployment. Yet, many organizations struggle with balancing the power of sophisticated AI models against the need for transparency and interpretability.
Today’s regulatory landscape around XAI reflects this tension. While the European Union leads with comprehensive frameworks like the AI Act requiring explainability for high-risk AI systems, other regions take varying approaches. Some favor industry self-regulation, while others are just beginning to grapple with these challenges. This fragmented global response creates both opportunities and obstacles for organizations implementing AI systems.
The stakes couldn’t be higher. Without proper explainability requirements, AI systems risk perpetuating biases, making unaccountable decisions, or losing public trust entirely. Yet overly restrictive regulations could stifle innovation and prevent beneficial AI applications from reaching those who need them most.
We’ll explore how different jurisdictions approach XAI regulations, examine the technical challenges of implementing explainability, and investigate the practical implications for organizations deploying AI systems.
The Need for Explainable AI in Regulatory Compliance
The rapid evolution of artificial intelligence systems has prompted regulatory bodies worldwide to establish stringent transparency requirements, with the EU AI Act emerging as a landmark framework for ensuring AI accountability. This legislation mandates that high-risk AI systems must provide clear explanations for their decision-making processes, marking a pivotal shift toward responsible AI development.
Under the EU AI Act’s requirements, organizations deploying AI systems must implement robust transparency mechanisms. These requirements are particularly stringent for high-risk AI applications, which must undergo thorough conformity assessments and provide detailed documentation of their inner workings. Non-compliance can result in substantial penalties – up to €30 million or 6% of global annual revenue – underscoring the critical importance of explainability in AI systems.
Explainable AI (XAI) technologies have become essential tools for meeting these regulatory demands. Rather than operating as black boxes, AI systems must now provide clear rationales for their outputs, enabling stakeholders to understand how decisions are reached. This transparency is crucial not only for regulatory compliance but also for building trust with users who are increasingly concerned about algorithmic bias and fairness.
Real-world implementations demonstrate the practical value of explainable AI in regulatory compliance. Financial institutions, for instance, must now explain how their AI systems make lending decisions to comply with anti-discrimination laws. Healthcare providers using AI for diagnosis must ensure their systems can clearly articulate the reasoning behind medical recommendations, satisfying both regulatory requirements and professional standards.
Beyond mere compliance, explainable AI offers tangible benefits for organizations. By providing insight into decision-making processes, XAI enables better risk management, facilitates audit trails, and helps identify potential biases before they impact operations. This proactive approach not only satisfies regulatory requirements but also enhances the overall quality and reliability of AI systems.
Key Elements of Effective Explainable AI Regulations
Artificial intelligence (AI) systems are increasingly influencing critical decisions in our lives, leading to the development of regulatory frameworks designed to ensure these systems remain transparent, fair, and accountable. Effective AI regulations rely on several key elements that protect individuals while fostering innovation.
One fundamental requirement is model transparency, which mandates that AI systems disclose how they arrive at their decisions. Organizations must provide clear and understandable explanations of their AI models’ inner workings. Rather than functioning as opaque “black boxes,” these systems should operate more like “glass boxes,” allowing regulators and affected individuals to comprehend the decision-making process.
Interpretability is another essential component, focusing on making AI outputs understandable to humans. Legal experts emphasize that organizations must ensure their AI systems offer meaningful information about the logic behind automated decisions, rather than just technical jargon. This practice helps build trust and enables individuals to effectively challenge outcomes that impact them.
Fairness in AI systems requires careful consideration of potential biases and discriminatory effects. Regulatory frameworks increasingly mandate that organizations test their AI models for unfair treatment of various groups and implement safeguards to prevent discrimination. This includes regular audits of outcomes and proactive measures to identify and address emerging fairness issues.
The ability to audit AI decisions serves as a critical oversight mechanism. Organizations must maintain comprehensive records of their AI systems’ development, testing, and deployment. This documentation allows regulators to verify compliance and helps organizations demonstrate their due diligence in addressing potential risks and harms.
Effective AI regulations acknowledge that explainability requires a holistic approach. Glass-box modeling techniques, which prioritize interpretable algorithms over complex black-box models, help organizations meet regulatory requirements while maintaining performance. These methods facilitate a clearer understanding of how inputs relate to outputs, making it easier to identify and rectify issues.
Challenges and Solutions in Implementing Explainable AI
The surge in AI adoption across critical domains has unveiled a pressing challenge: making complex AI systems transparent and interpretable while maintaining their performance. As organizations integrate AI into high-stakes decisions affecting healthcare, finance, and criminal justice, the ability to explain AI’s decision-making process has become paramount.
One of the fundamental challenges in implementing explainable AI lies in the inherent complexity of modern AI systems. Recent research highlights the significant trade-off between model accuracy and interpretability, forcing developers to balance performance against transparency. Neural networks, particularly deep learning models, often operate as ‘black boxes,’ making their decision-making processes opaque to both developers and end-users.
The implementation of explainable AI also faces technical hurdles in standardization. The lack of universal evaluation metrics for interpretability makes it challenging to assess and compare different explainability approaches effectively. This absence of standardized benchmarks has led to fragmented solutions across the industry, complicating the widespread adoption of explainable AI frameworks.
Emerging Solutions and Frameworks
To address these challenges, researchers and practitioners have developed robust explainability frameworks that offer promising solutions. SHAP (SHapley Additive exPlanations) has emerged as a leading technique, providing a unified approach to explaining model outputs based on game theory principles. SHAP values help quantify each feature’s contribution to model predictions, offering both global and local interpretability.
LIME (Local Interpretable Model-agnostic Explanations) offers another powerful solution, particularly valuable for understanding individual predictions. By creating simplified local approximations of complex models, LIME helps stakeholders comprehend specific decisions without sacrificing the underlying model’s sophistication.
Criteria | SHAP | LIME |
---|---|---|
Full Name | SHapley Additive exPlanations | Local Interpretable Model-agnostic Explanations |
Explanation Type | Global and Local | Local |
Model Complexity | Accommodates complex models | More straightforward computationally |
Stability and Consistency | High stability | Can be unstable |
Computational Expense | High, except for tree-based models | Lower |
Output Format | Feature importance values | Interpretable models like decision trees |
Example Use Case | Credit scoring | Fraud detection |
The evolution of explainable AI introduces ethical considerations, particularly surrounding biased models and the ‘right to explanation.’ Establishing robust regulatory frameworks becomes imperative to ensure fairness, mitigate biases, and empower users with the ability to comprehend decisions made by AI systems.
Organizations have also begun implementing hybrid approaches that combine multiple explainability techniques. These integrated solutions allow for more comprehensive model interpretation while maintaining acceptable levels of performance. For instance, some frameworks utilize SHAP for global model understanding while employing LIME for detailed analysis of individual cases.
Success in implementing explainable AI ultimately requires a holistic approach that considers both technical and organizational factors. This includes investing in proper documentation, establishing clear governance frameworks, and ensuring ongoing collaboration between technical teams and domain experts. As the field continues to evolve, these solutions will play an increasingly crucial role in building trustworthy and transparent AI systems.
Global Perspectives on Explainable AI Regulations
The global regulatory landscape for explainable AI reveals distinct approaches across major jurisdictions, with the European Union taking the most comprehensive stance through its AI Act. The EU has established a risk-based framework that mandates transparency and explainability requirements, particularly for high-risk AI systems. Under Article 13 of the EU AI Act, providers must ensure systems are sufficiently transparent and accompanied by clear documentation explaining their capabilities and limitations.
In contrast, the United States has adopted a more flexible, principles-based approach that emphasizes industry self-regulation and voluntary guidelines. While the US pioneered explainable AI research through DARPA’s XAI program in 2016, its regulatory framework remains relatively loose. The National Institute of Standards and Technology (NIST) has developed guidance on AI explainability principles, but these are not legally binding requirements like their EU counterparts.
The United Kingdom charts a middle path, focusing on practical industry guidance while building institutional capacity for AI oversight. Through collaboration between the Information Commissioner’s Office and the Alan Turing Institute, the UK has produced comprehensive technical guidelines on explaining AI decisions. This guidance provides detailed frameworks for different stakeholder groups, from technical teams to compliance officers.
A notable difference lies in enforcement mechanisms. The EU AI Act includes specific penalties for non-compliance, with fines up to 7% of global annual turnover. The US relies more on existing regulatory frameworks and market forces, while the UK emphasizes building collaborative relationships between regulators and industry to promote best practices.
Region | Regulatory Approach | Enforcement Mechanisms | Penalties |
---|---|---|---|
EU | Comprehensive, risk-based framework | Conformity assessments, transparency requirements, oversight by national competent authorities | Up to 6% of global annual revenue or €30 million, whichever is greater |
US | Principles-based, flexible, industry self-regulation | Guidelines and voluntary standards by agencies like NIST, sector-specific regulations | Varies by sector, generally relies on existing regulatory frameworks |
UK | Practical guidance, industry collaboration | High-level principles implemented by existing regulators, sector-specific guidelines | Sector-specific penalties, collaborative enforcement approach |
Implementation timelines also vary significantly. The EU has established clear deadlines for compliance, with requirements phasing in over 6-36 months after adoption. The US approach allows for more gradual adoption of explainability practices, while the UK’s framework enables continuous evolution of standards through regular stakeholder engagement.
Cross-border considerations are increasingly important as AI systems operate globally. The EU’s regulations have extraterritorial reach, affecting any provider serving EU users, while US and UK approaches focus primarily on domestic markets. This creates complexity for multinational organizations that must navigate multiple regulatory regimes simultaneously.
Best practices emerging from these different approaches suggest that successful AI explainability frameworks should balance prescriptive requirements with flexibility for innovation. This includes establishing clear documentation standards, providing context-specific explanations tailored to different stakeholders, and maintaining robust audit trails of AI decision-making processes.
Looking ahead, international standardization efforts through organizations like ISO and IEEE are working to harmonize explainability requirements across jurisdictions. However, significant differences in regulatory philosophy and implementation approaches are likely to persist, requiring organizations to maintain adaptable compliance strategies.
Leveraging SmythOS for Compliant Explainable AI Development
Building AI systems that can explain their decisions is essential in today’s regulatory landscape. SmythOS addresses this challenge with its platform designed for developing transparent, explainable AI solutions that meet compliance requirements.
At the core of SmythOS’s explainability features is its visual workflow builder. Unlike traditional ‘black box’ AI systems, SmythOS enables developers to create AI agents with clear decision paths that can be easily audited and understood. The drag-and-drop interface allows teams to map out automation logic visually, making the decision-making process transparent from the start.
The platform’s visual debugging capabilities set a new standard for AI transparency. Developers can trace how their AI agents process information and arrive at conclusions in real-time. This insight is crucial for identifying potential biases, ensuring fairness, and maintaining compliance with regulations like GDPR that mandate explainable automated decision-making.
SmythOS’s real-time monitoring system provides continuous visibility into AI operations, allowing teams to track performance metrics and decision outputs as they occur. This feedback loop is essential for maintaining oversight and quickly addressing any concerns about AI behavior or decision-making patterns.
The platform goes beyond technical transparency by incorporating natural language explanations into its AI agents. This means complex AI decisions can be communicated in clear, human-readable terms that stakeholders at all levels can understand—from developers and compliance officers to end-users affected by AI decisions.
As AI becomes more prevalent in our daily lives, the importance of XAI will only grow. It’s about creating AI systems that we can truly trust and rely on.
Through its combination of visual tools, real-time monitoring, and natural language capabilities, SmythOS makes it possible to develop AI systems that are not just powerful, but also trustworthy and compliant with current and emerging regulations. This approach to explainable AI development helps organizations build confidence in their AI solutions while meeting their regulatory obligations.
Future Directions for Explainable AI Regulations
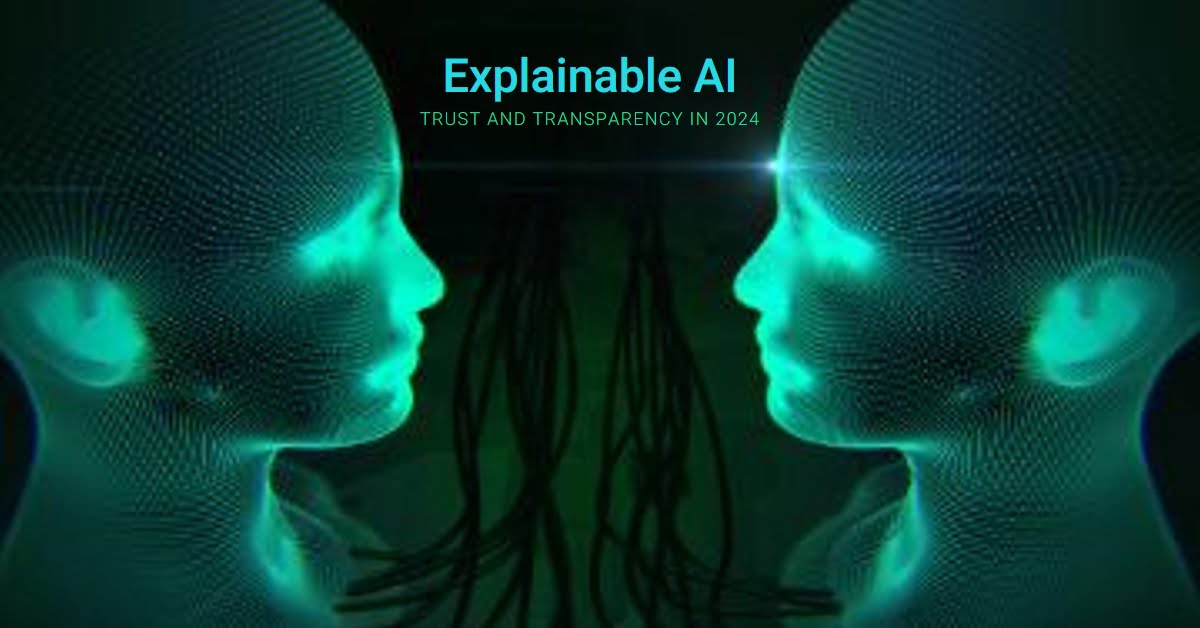
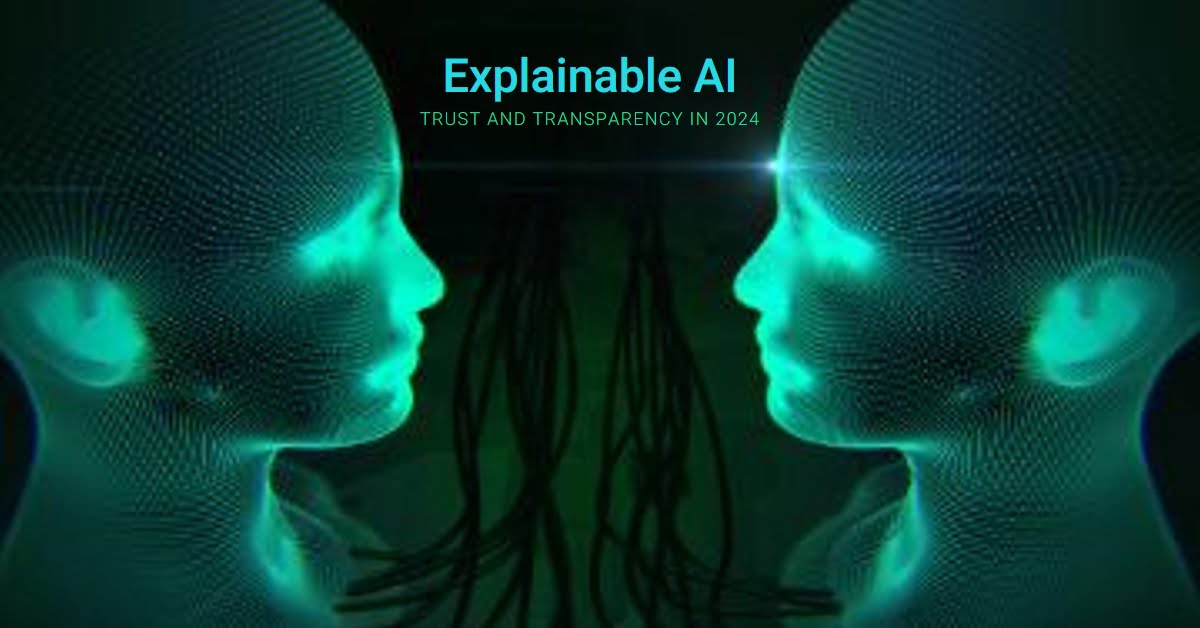
The regulatory landscape for explainable AI stands at a critical inflection point. Recent developments like the EU’s AI Act and the G7’s Hiroshima Process on Generative AI show that global policymakers are moving toward more comprehensive regulatory frameworks. The Biden Administration’s Executive Order on Safe, Secure, and Trustworthy AI signals a shift toward standardized requirements for AI transparency and accountability.
Risk-based approaches are becoming the cornerstone of future AI regulations. Instead of applying blanket rules, regulators are developing tiered frameworks matching oversight to the risks posed by different AI applications. This suggests future guidelines will be increasingly precise, with specific requirements tailored to various AI use cases and their associated risks.
Integration with existing regulatory systems represents another crucial frontier. Jurisdictions like the EU demonstrate through initiatives like the Digital Services Act and Cyber Resilience Act that future frameworks will likely weave AI oversight into broader digital governance structures. This will help create more cohesive and effective regulatory environments.
International collaboration is gaining momentum in shaping future AI regulations. The Bletchley Declaration and G7’s International Guiding Principles illustrate a growing recognition that effective AI governance requires coordinated global action. This trend points toward more harmonized international standards for AI transparency and accountability.
Regulatory sandboxes are emerging as vital tools for developing practical, evidence-based regulations. These controlled testing environments allow regulators and companies to collaborate in real time, suggesting that future guidelines will be increasingly grounded in practical experience rather than theoretical frameworks.
Looking ahead, AI regulations are expected to become more sophisticated and nuanced, shaped by ongoing technological advances and lessons from early implementation efforts. The future of AI governance will likely emphasize adaptability, international cooperation, and a balance between innovation and accountability – ensuring that as AI systems grow more powerful, our ability to understand and oversee them grows in parallel.
Last updated:
Disclaimer: The information presented in this article is for general informational purposes only and is provided as is. While we strive to keep the content up-to-date and accurate, we make no representations or warranties of any kind, express or implied, about the completeness, accuracy, reliability, suitability, or availability of the information contained in this article.
Any reliance you place on such information is strictly at your own risk. We reserve the right to make additions, deletions, or modifications to the contents of this article at any time without prior notice.
In no event will we be liable for any loss or damage including without limitation, indirect or consequential loss or damage, or any loss or damage whatsoever arising from loss of data, profits, or any other loss not specified herein arising out of, or in connection with, the use of this article.
Despite our best efforts, this article may contain oversights, errors, or omissions. If you notice any inaccuracies or have concerns about the content, please report them through our content feedback form. Your input helps us maintain the quality and reliability of our information.