Explainable AI in Healthcare: Promoting Transparency and Trust in Patient Care
Imagine a doctor making a critical medical decision based on an AI system’s recommendation, but having no insight into how that recommendation was made. This scenario highlights one of healthcare’s most pressing challenges: the need for transparent and explainable artificial intelligence.
Healthcare professionals increasingly rely on AI systems to analyze complex medical data, assist with diagnoses, and recommend treatments. Yet, without understanding how these systems reach their conclusions, clinicians may hesitate to trust and implement AI-driven recommendations. This is where Explainable AI (XAI) emerges as a crucial solution, offering clarity and transparency in medical decision-making.
Think of XAI as a translator between complex AI algorithms and healthcare professionals – it reveals the reasoning behind AI-generated insights in ways that doctors and patients can understand. Rather than receiving unexplained outputs from a black box system, clinicians can see which factors influenced the AI’s analysis, from specific biomarkers to patterns in medical imaging.
In an era where regulatory compliance and patient trust are paramount, XAI addresses both the technical and human aspects of healthcare innovation. It helps ensure that AI systems meet stringent healthcare regulations while providing medical professionals with the confidence to incorporate AI insights into their clinical practice.
Throughout this article, we’ll explore how XAI is transforming healthcare delivery across multiple fronts – from enhancing diagnostic accuracy to improving treatment planning.
The Role of Explainable AI in Diagnostics
The landscape of medical diagnostics is undergoing a profound transformation through Explainable AI (XAI), which illuminates the traditionally opaque decision-making processes of AI systems. Rather than simply providing predictions, XAI offers clinicians detailed insights into how and why specific diagnostic conclusions are reached, addressing a critical need for transparency in healthcare.
In radiology, for instance, XAI employs visualization techniques like Gradient-weighted Class Activation Mapping (Grad-CAM) to highlight precisely which areas of a medical image influenced the AI’s diagnostic assessment. As noted in a recent study, this approach achieved 90% accuracy in pneumonia detection while providing clear visual explanations that resonated with healthcare professionals.
Beyond image analysis, XAI systems break down complex patient data into interpretable components, allowing clinicians to understand the relative importance of different health indicators in reaching a diagnosis. For example, when evaluating a patient’s risk of cardiovascular disease, XAI can quantify how factors like blood pressure, cholesterol levels, and family history contribute to the final assessment, enabling doctors to make more informed treatment decisions.
The impact of XAI extends to clinical decision support, where it helps validate AI predictions by exposing potential biases or limitations in the underlying models. This transparency is crucial for building trust – clinicians can confidently incorporate AI insights into their diagnostic process when they understand the reasoning behind each recommendation.
Perhaps most significantly, XAI serves as a bridge between cutting-edge technology and clinical expertise. Rather than replacing human judgment, it augments medical professionals’ decision-making capabilities by providing explainable, evidence-based insights that can be meaningfully integrated into patient care. This collaborative approach ensures that advanced AI capabilities enhance rather than obscure the diagnostic process, ultimately leading to better patient outcomes.
Challenges in Implementing Explainable AI in Healthcare
Healthcare organizations eager to harness the power of explainable AI (XAI) face significant hurdles that extend beyond technical implementation. From safeguarding sensitive patient data to ensuring seamless integration with existing medical systems, the path to successful XAI deployment demands careful navigation and strategic planning.
Data privacy emerges as a paramount concern when implementing XAI solutions in healthcare settings. As highlighted by recent research, healthcare institutions must carefully balance the need for algorithmic transparency with strict patient confidentiality requirements. Protected health information requires robust security measures while still allowing AI systems to provide meaningful, explainable insights to healthcare providers.
Integration with existing healthcare systems presents another formidable challenge. Legacy medical infrastructure, from electronic health records to diagnostic imaging systems, often operates on proprietary platforms that weren’t designed with AI integration in mind. Healthcare providers must carefully orchestrate the implementation of XAI solutions to ensure they complement rather than disrupt existing workflows and clinical processes.
Challenge | Solution |
---|---|
Data Privacy | Implement robust security measures to protect patient confidentiality while ensuring transparency in AI insights. |
Integration with Existing Systems | Carefully orchestrate the implementation of XAI solutions to complement existing workflows and clinical processes. |
Interdisciplinary Collaboration | Facilitate ongoing dialogue between medical professionals, data scientists, ethicists, and regulatory experts to refine and validate AI explanations. |
Regulatory Compliance | Develop robust documentation processes and establish clear audit trails for AI-assisted medical decisions to meet standards like HIPAA and GDPR. |
Building Trust | Start with less critical applications to build confidence and expertise, provide regular training for medical staff, and maintain clear communication channels between technical and clinical teams. |
The complexity of healthcare decisions demands unprecedented levels of interdisciplinary collaboration. Medical professionals, data scientists, ethicists, and regulatory experts must work in concert to develop XAI systems that are both technically sound and clinically relevant. This collaboration extends beyond initial development, requiring ongoing dialogue to refine and validate AI explanations in real-world medical scenarios.
Regulatory compliance adds another layer of complexity to XAI implementation. Healthcare organizations must navigate evolving frameworks like HIPAA and GDPR while ensuring their AI systems maintain explainability standards. This requires developing robust documentation processes and establishing clear audit trails for AI-assisted medical decisions.
The success of XAI in healthcare ultimately depends on our ability to bridge the gap between clinicians’ needs and developers’ goals. We must create systems that not only make accurate predictions but also provide explanations that align with clinical workflows and decision-making processes.
To overcome these challenges, healthcare organizations should consider implementing staged rollouts of XAI systems, starting with less critical applications to build confidence and expertise. Regular training programs for medical staff, clear communication channels between technical and clinical teams, and continuous monitoring of system performance can help ensure successful integration while maintaining high standards of patient care.
Benefits of Explainable AI for Clinicians and Patients
Explainable artificial intelligence (XAI) offers powerful advantages that enhance healthcare delivery for both medical professionals and patients. For clinicians, XAI transforms the traditionally opaque ‘black box’ of AI systems into transparent, understandable decision-making tools that they can confidently integrate into their practice.
From the clinical perspective, XAI significantly improves diagnostic accuracy by allowing doctors to understand exactly how the AI arrives at its conclusions. When an AI system flags a potential diagnosis, it can highlight the specific features it analyzed and explain the reasoning behind its assessment. As demonstrated in recent studies, this level of transparency enables clinicians to validate the AI’s findings against their medical expertise and catch potential errors or biases.
For patients, XAI ensures they can fully understand and participate in decisions about their care. Rather than being presented with unexplained AI recommendations, patients receive clear explanations about how the technology analyzed their case and arrived at specific treatment suggestions. This transparency builds trust and empowers patients to engage more actively in their treatment journey.
Explainable AI allows doctors and patients to move forward with greater confidence, knowing they understand not just what the AI recommends, but why it makes those recommendations.
Beyond individual benefits, XAI also improves the overall efficacy of treatment by fostering better collaboration between clinicians and patients. When both parties clearly understand the AI’s analysis and recommendations, they can have more productive discussions about treatment options and make more informed decisions together.
The trust-building aspect of XAI cannot be overstated. Healthcare professionals are more likely to adopt and rely on AI systems they can understand and verify. Similarly, patients show greater confidence in AI-supported diagnoses when they can see the logical reasoning behind them. This increased trust leads to better adherence to treatment plans and ultimately improved patient outcomes.
Looking ahead, as healthcare increasingly integrates AI technologies, the role of XAI in ensuring transparency and maintaining human oversight becomes even more critical. It serves as the bridge between cutting-edge AI capabilities and the human-centered practice of medicine, ensuring that technological advancement enhances rather than diminishes the doctor-patient relationship.
Case Studies of Explainable AI in Action
The healthcare sector has witnessed remarkable transformations through the implementation of Explainable AI (XAI), particularly in improving diagnostic accuracy and patient care. By examining real-world applications, we can better understand how XAI enhances medical decision-making while maintaining transparency and trust.
In one notable implementation, researchers developed a sophisticated XAI system for detecting pneumonia from chest X-rays. As reported by Ihongbe et al., the system achieved 90% accuracy while providing clear visual explanations through gradient-weighted class activation mapping (Grad-CAM). This approach enabled radiologists to understand exactly which areas of the X-ray influenced the AI’s diagnostic decisions, leading to more confident clinical assessments.
Study | Application | Performance Metric | Explanation Method |
---|---|---|---|
Ihongbe et al. | Pneumonia detection from chest X-rays | 90% accuracy | Grad-CAM |
Oncological Ultrasound Review | Oncological ultrasound image analysis | 14 XAI methods evaluated | Visualization, semantics, example-based, hybrid |
XAI-EWS | Predicting critical conditions from healthcare records | Preventive actions taken based on predictions | Visual explanations |
Colorectal Cancer Study | Analyzing pathological signatures | Actionable insights for treatment planning | Highlighting genetic and transcriptomic patterns |
Another compelling case study involves the early warning system XAI-EWS, which analyzes electronic healthcare records to predict critical conditions. The system not only forecasts potential complications but also provides straightforward visual explanations for its predictions, allowing healthcare providers to take preventive actions with greater confidence in the AI’s reasoning.
The transparency provided by XAI has proven particularly valuable in oncology. Researchers successfully implemented an XAI model for analyzing pathological signatures in colorectal cancer cases. The system could explain its predictions by highlighting specific genetic and transcriptomic patterns related to DNA repair and immune activation, providing oncologists with actionable insights for treatment planning.
Perhaps most significantly, a comprehensive study demonstrated how XAI integration improved diagnostic confidence among clinicians. Healthcare professionals reported increased trust in AI-assisted decisions when provided with clear explanations of the underlying logic. This transparency not only enhanced the adoption of AI tools but also led to better collaboration between human expertise and machine intelligence.
These implementations showcase XAI’s potential to bridge the gap between advanced AI capabilities and practical clinical applications. By making AI decisions interpretable and transparent, healthcare providers can leverage these powerful tools while maintaining their critical role in patient care decisions. The success of these case studies suggests that XAI will continue to play an increasingly important role in shaping the future of healthcare delivery.
Future Directions for Explainable AI in Healthcare
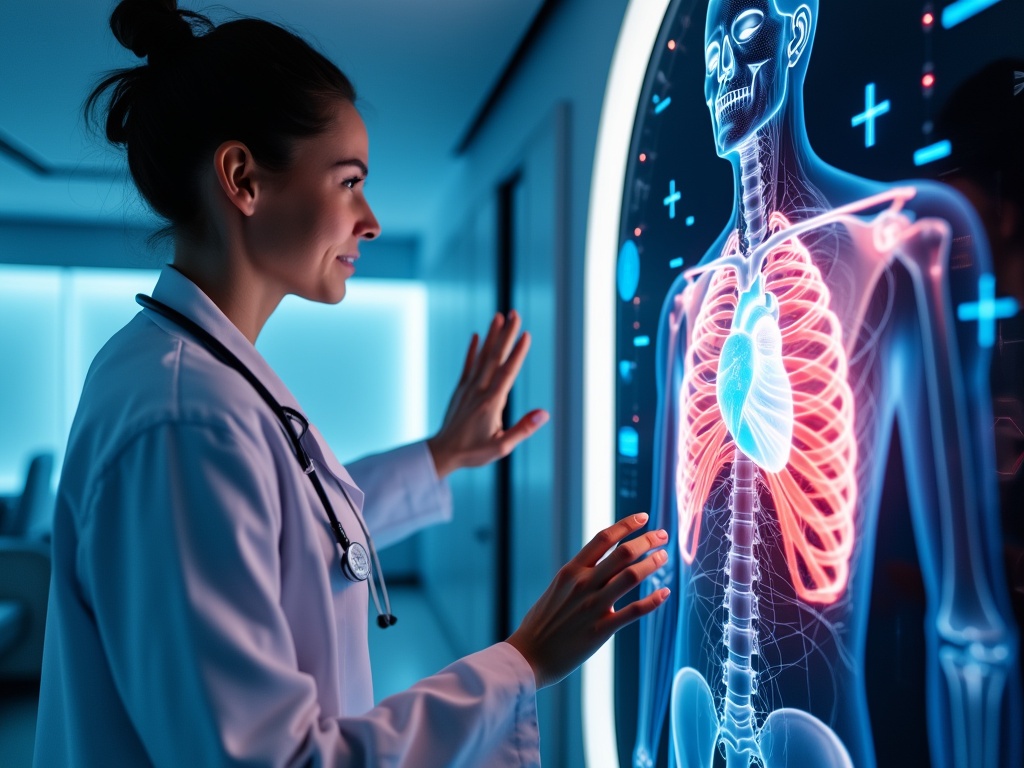
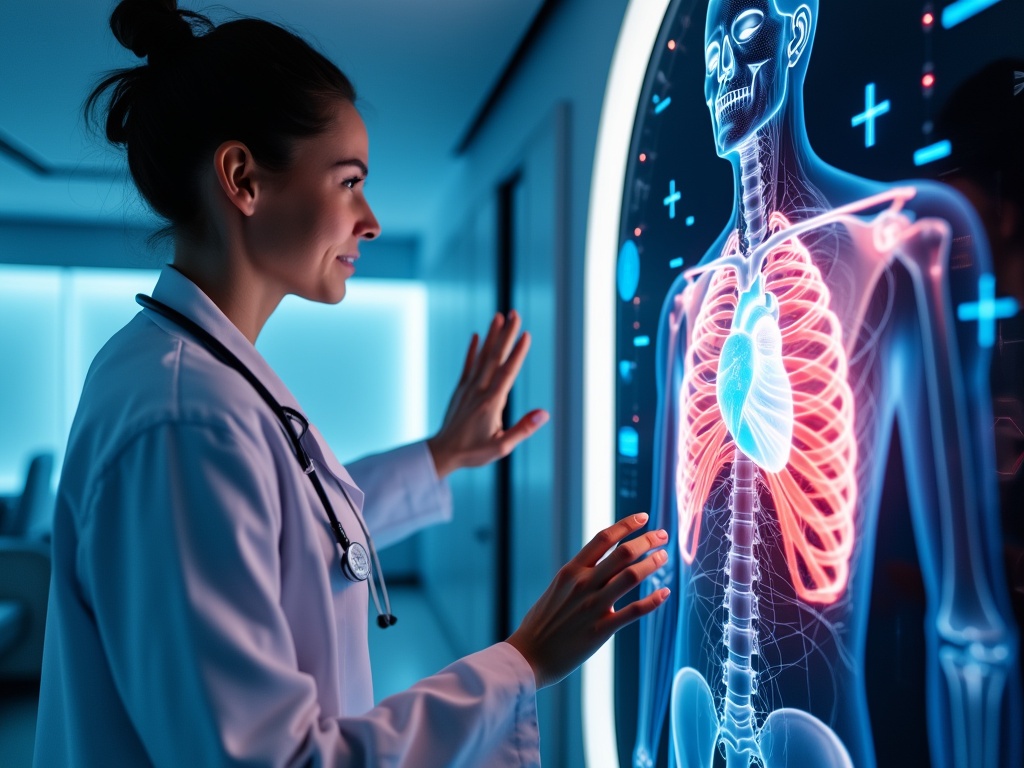
The evolving landscape of healthcare AI stands at a pivotal moment, with explainable AI (XAI) emerging as a cornerstone for building trust and transparency in medical decision-making. Significant developments in recent years have demonstrated the transformative potential of XAI systems that can not only provide accurate predictions but also explain their reasoning in ways meaningful to healthcare professionals.
One of the most promising trends is the advancement of multimodal XAI solutions that can integrate diverse types of medical data. A recent study published in Nature Digital Medicine highlights that next-generation XAI systems will need to handle both longitudinal patient data and various data types, from medical imaging to electronic health records. This integration promises more comprehensive and contextually aware diagnostic support while maintaining transparency in decision-making processes.
The emergence of platforms like SmythOS represents a significant step forward in making XAI more accessible and practical for healthcare settings. These platforms provide complete visibility into agent decision-making processes through visual workflows and built-in debugging capabilities. By offering enterprise-grade audit logging and support for multiple explanation methods, they address the critical need for accountability in healthcare AI applications.
Looking ahead, we can expect to see increased focus on developing XAI systems that balance sophisticated analysis with intuitive explanations. The integration of natural language explanation capabilities will make AI insights more accessible to healthcare professionals while maintaining the technical rigor necessary for medical applications. Visual representation of decision paths and real-time monitoring of agent decisions will become standard features, enabling healthcare providers to understand and validate AI recommendations quickly.
Perhaps most significantly, the future of XAI in healthcare will be shaped by its ability to integrate seamlessly with existing medical workflows and monitoring systems. As these systems evolve, they will need to provide explanations that are not only technically accurate but also clinically relevant and actionable. The emphasis will be on developing solutions that enhance rather than complicate the decision-making process for healthcare professionals.
Last updated:
Disclaimer: The information presented in this article is for general informational purposes only and is provided as is. While we strive to keep the content up-to-date and accurate, we make no representations or warranties of any kind, express or implied, about the completeness, accuracy, reliability, suitability, or availability of the information contained in this article.
Any reliance you place on such information is strictly at your own risk. We reserve the right to make additions, deletions, or modifications to the contents of this article at any time without prior notice.
In no event will we be liable for any loss or damage including without limitation, indirect or consequential loss or damage, or any loss or damage whatsoever arising from loss of data, profits, or any other loss not specified herein arising out of, or in connection with, the use of this article.
Despite our best efforts, this article may contain oversights, errors, or omissions. If you notice any inaccuracies or have concerns about the content, please report them through our content feedback form. Your input helps us maintain the quality and reliability of our information.