Explainable AI in Autonomous Vehicles: Building Transparency and Trust on the Road
Imagine putting your life in the hands of a car that makes thousands of split-second decisions. Wouldn’t you want to understand how and why it makes those choices? This question lies at the heart of explainable AI (XAI) in autonomous vehicles, a groundbreaking technology transforming how self-driving cars communicate their decision-making process to humans.
Today’s autonomous vehicles are sophisticated machines equipped with complex AI systems that analyze vast amounts of sensor data to navigate roads safely. However, the ‘black box’ nature of traditional AI has created a barrier of trust between these vehicles and their potential users. This is where explainable AI steps in, transforming opaque decision-making processes into transparent, understandable explanations.
The stakes couldn’t be higher. When an autonomous vehicle decides to change lanes, brake suddenly, or navigate through a busy intersection, both passengers and pedestrians need to trust these decisions are made with their safety in mind. XAI provides this crucial bridge of understanding, offering clear, real-time insights into the vehicle’s ‘thinking process’ through visual cues, natural language explanations, and detailed analysis of each critical decision.
Recent research shows that XAI systems can significantly enhance the transparency and interpretability of autonomous vehicles, ensuring users and stakeholders understand exactly how decisions are made. This transparency isn’t just about comfort; it’s about building the foundation of trust necessary for widespread adoption of self-driving technology.
As regulations worldwide begin to demand greater accountability from AI systems, XAI has become not just a nice-to-have feature but a critical component of autonomous vehicle development. From explaining emergency maneuvers to providing insights into routine driving decisions, XAI is transforming autonomous vehicles from mysterious black boxes into transparent, trustworthy transportation partners.
Importance of Explainability in Autonomous Vehicles
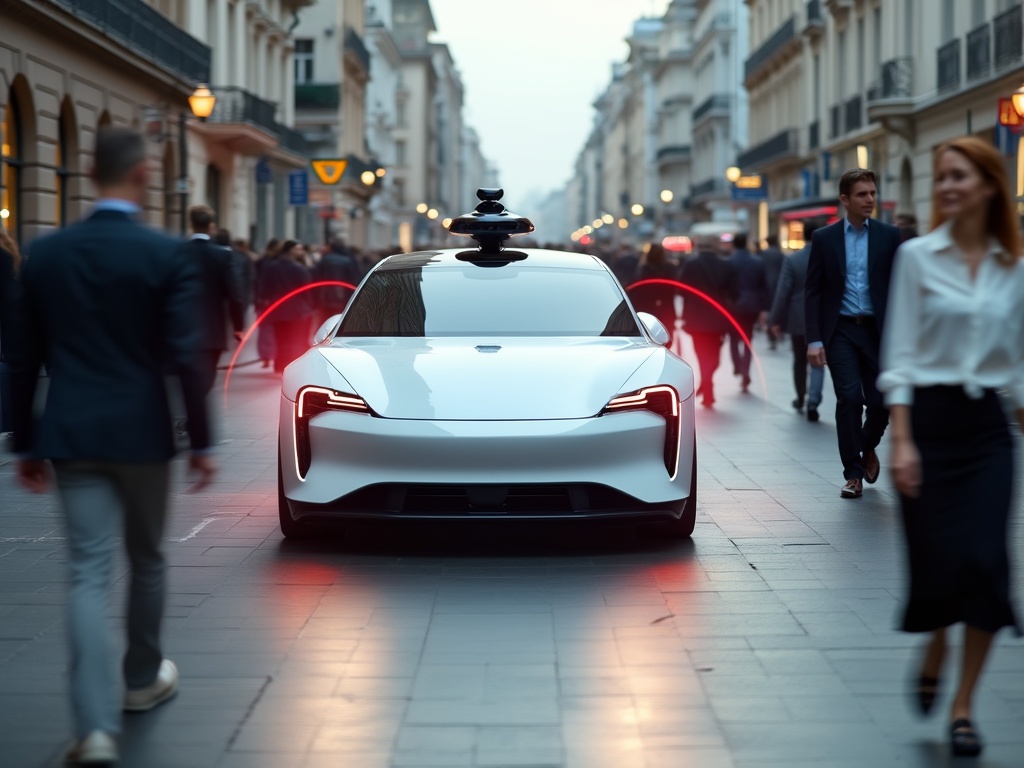
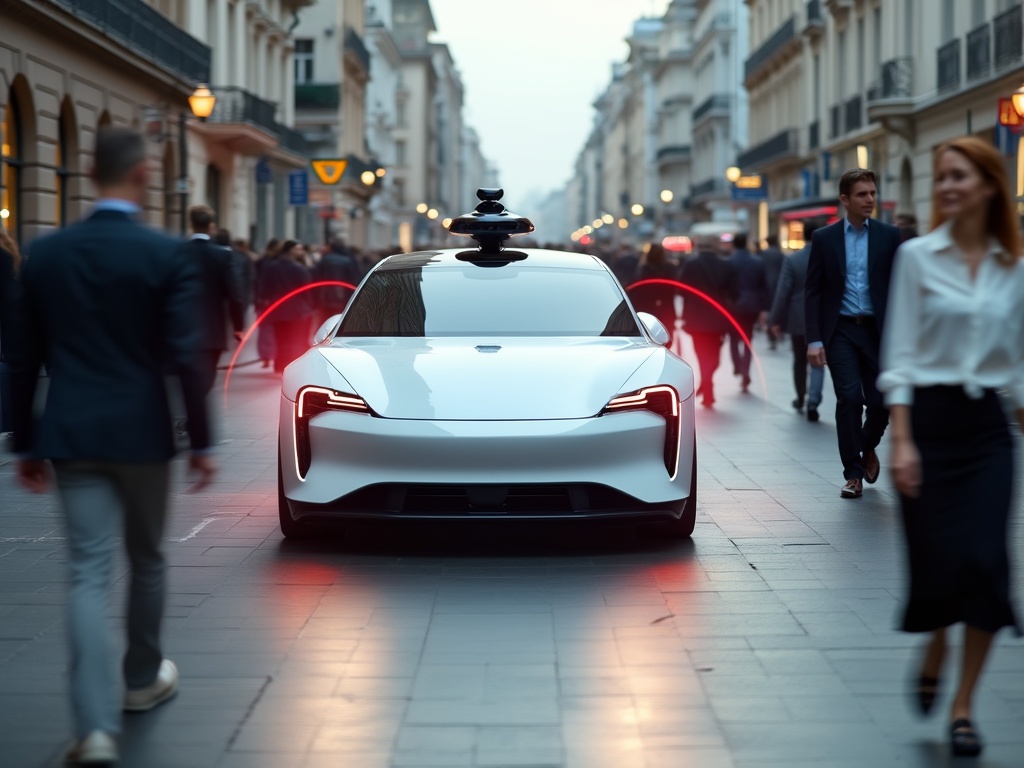
As self-driving vehicles become more prevalent on our roads, the need for transparency in their decision-making processes has never been more critical. Explainable AI (XAI) in autonomous vehicles serves as a bridge between complex technological capabilities and human understanding, addressing three fundamental concerns: safety assurance, regulatory compliance, and public trust.
Safety stands as the paramount concern in autonomous driving. Through XAI, vehicles can provide clear justifications for their actions in real-time – whether it’s explaining why they chose to brake suddenly or change lanes. This transparency allows both passengers and manufacturers to verify that the vehicle is making safe and appropriate decisions. For instance, when an autonomous vehicle encounters a complex traffic scenario, XAI can demonstrate how the system identified potential hazards and selected the safest course of action.
From a regulatory standpoint, explainability has become a crucial requirement across many jurisdictions. The European Union’s guidelines on trustworthy AI explicitly mandate transparency and accountability in AI systems, including autonomous vehicles. This regulatory framework ensures that manufacturers can demonstrate their vehicles’ compliance with safety standards and operational protocols, particularly during accident investigations or safety audits.
Building public trust represents another critical dimension of XAI in autonomous vehicles. When people understand why and how these vehicles make decisions, they’re more likely to feel confident about adopting this technology. This understanding is particularly important for potential users who may be hesitant about surrendering control to an AI system. Clear explanations of vehicle behavior help establish a foundation of trust between human users and autonomous systems.
The implementation of XAI also provides practical benefits for vehicle development and improvement. When engineers and developers can understand the reasoning behind a vehicle’s decisions, they can more effectively identify and correct potential issues, optimize performance, and enhance safety features. This continuous improvement cycle, facilitated by explainable AI, helps ensure that autonomous vehicles become increasingly reliable and trustworthy over time.
State-of-the-Art Techniques for Explainable AI
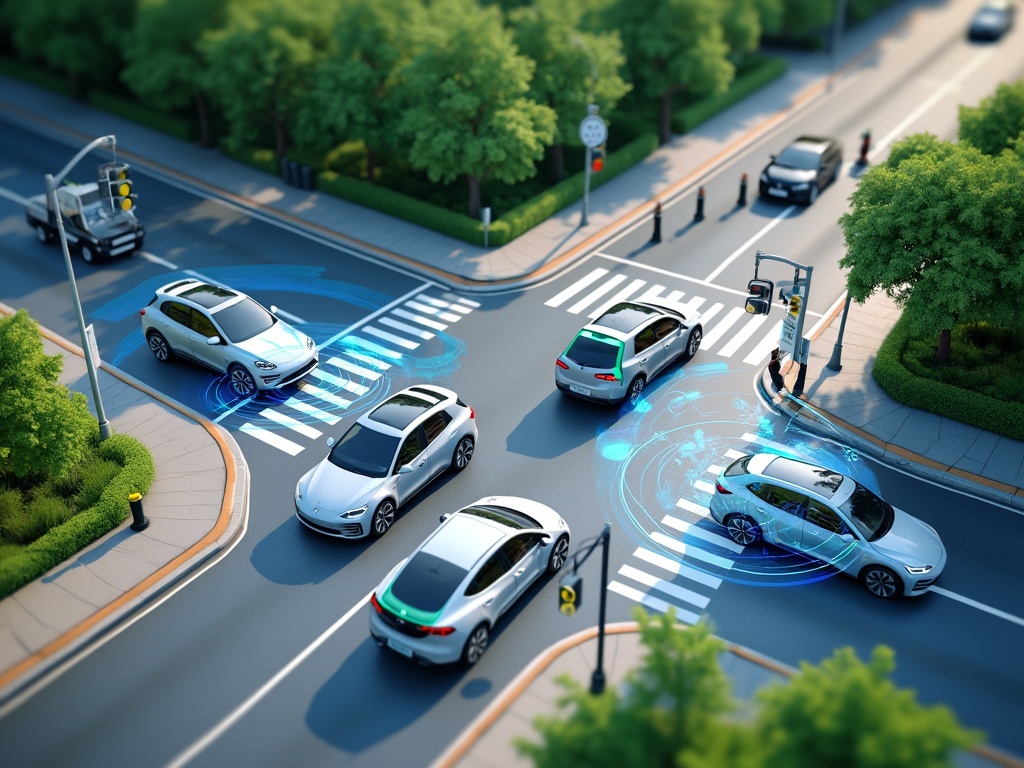
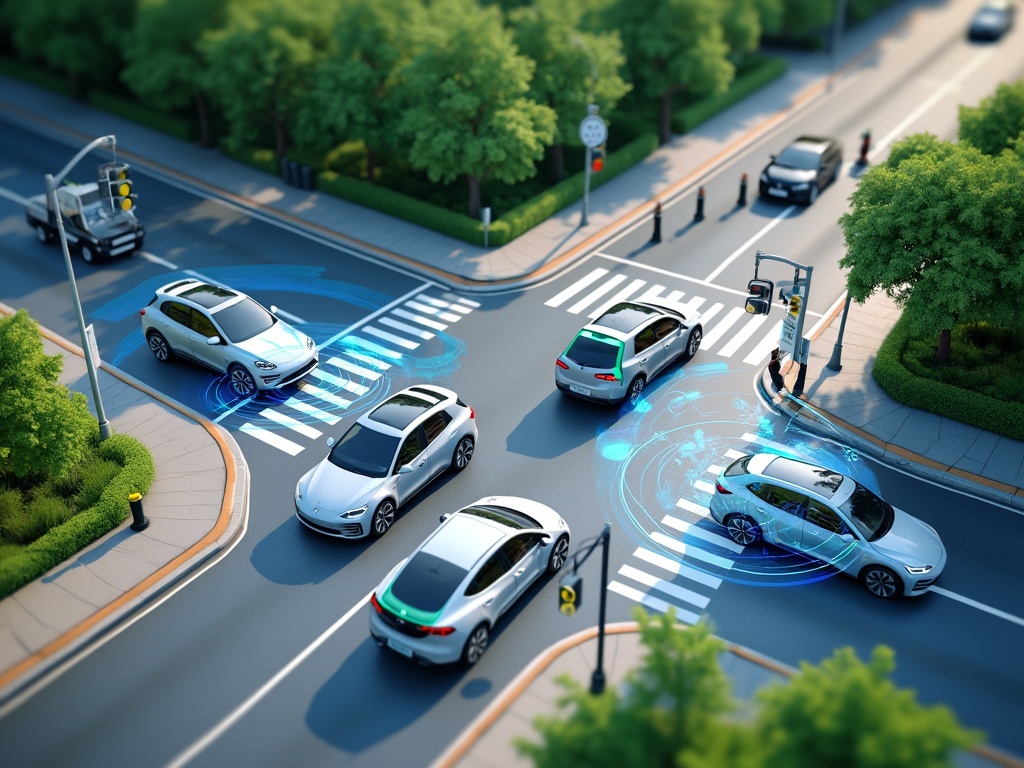
An advanced intersection with self-driving cars and cyclists.
Modern autonomous vehicles employ sophisticated artificial intelligence that can often seem like a ‘black box’ in terms of decision-making. However, recent advances in explainable AI (XAI) techniques have made it possible to peek inside these complex systems and understand their choices on the road.
Model-agnostic approaches represent one of the most versatile XAI techniques. These methods can analyze any AI model’s decisions without needing to understand its internal architecture. For example, researchers have demonstrated how model-agnostic techniques can explain why an autonomous vehicle decides to change lanes or adjust its speed, regardless of the underlying AI implementation.
Post-hoc explanations provide insights after the fact, helping decode why an AI made specific decisions during a drive. These explanations can take various forms, from visual heat maps highlighting important objects the AI detected to natural language descriptions of the reasoning process. When an autonomous vehicle brakes suddenly, post-hoc analysis can reveal whether it responded to a pedestrian, another vehicle, or a traffic signal.
Local interpretability focuses on explaining individual decisions in specific situations. For instance, when an autonomous vehicle approaches an intersection, local interpretability methods can break down exactly which factors – such as traffic light status, presence of pedestrians, or approaching vehicles – influenced its decision to stop or proceed.
Explainable AI is not just about transparency – it’s about building trust between humans and autonomous systems through understanding.
Shahin Atakishiyev, XAI Researcher
Global interpretability, on the other hand, helps us understand the AI’s overall decision-making patterns across many situations. This broader view reveals general driving behaviors and safety priorities programmed into the system, such as maintaining safe following distances or yielding to emergency vehicles.
These various XAI techniques work together to create a comprehensive understanding of autonomous vehicle behavior, making these complex systems more transparent and trustworthy for both passengers and regulators. As self-driving technology continues to evolve, the ability to explain AI decisions clearly and reliably remains crucial for public acceptance and safety assurance.
Applications of XAI in Autonomous Vehicles
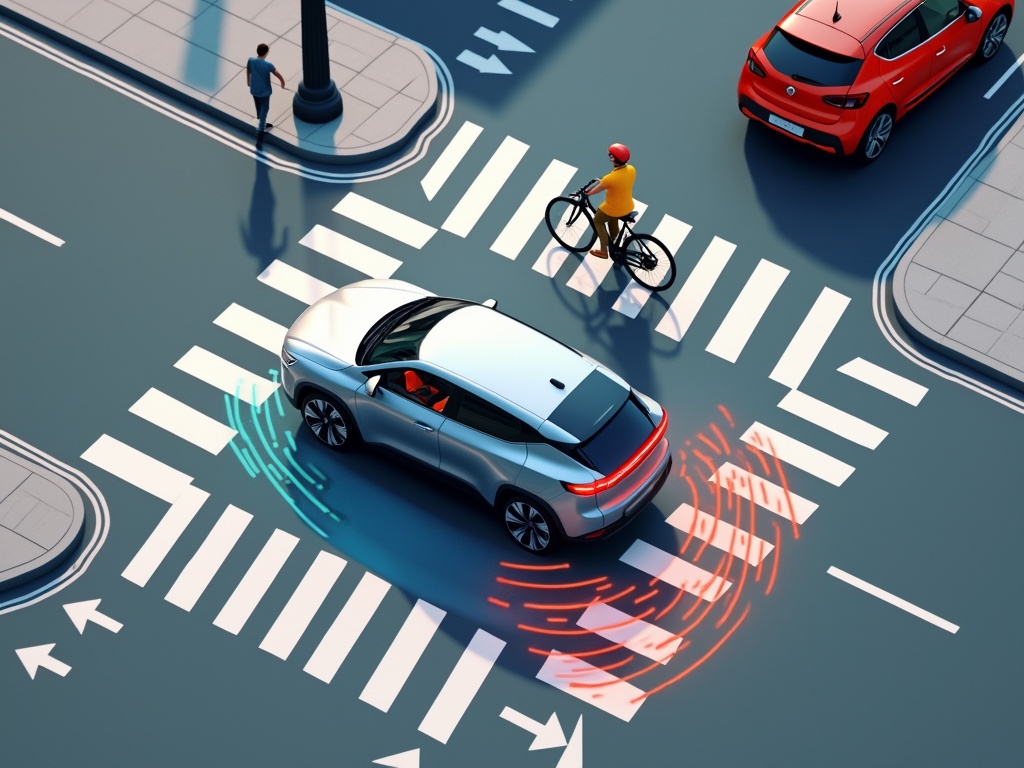
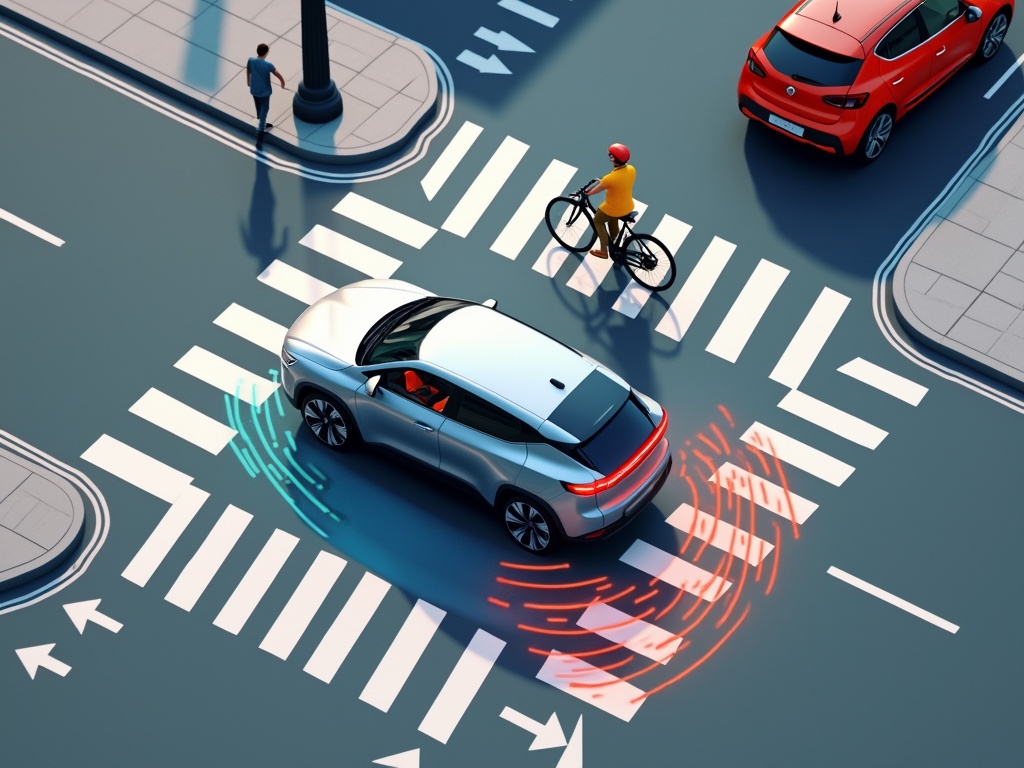
A cyclist and cars at a smart city intersection.
As autonomous vehicles become more prevalent on our roads, explainable artificial intelligence (XAI) plays an increasingly vital role in ensuring their safe and reliable operation. The integration of XAI into self-driving systems addresses one of the most significant challenges in autonomous vehicle adoption: making complex AI decisions transparent and understandable to humans.
Safety enhancement represents one of the most crucial applications of XAI in autonomous vehicles. When a self-driving car makes a sudden decision, such as an emergency brake or lane change, XAI systems provide clear explanations for these actions. For instance, recent research indicates that XAI-enabled vehicles can articulate their decision-making process in real-time, helping passengers understand why the vehicle chose a particular action.
Real-time decision monitoring represents another critical application of XAI in autonomous vehicles. These systems continuously track and explain the vehicle’s behavior, providing instant feedback about environmental perception, risk assessment, and action choices. For example, when approaching an intersection, the system can explain how it interprets traffic signals, pedestrian movements, and other vehicles’ behaviors, making its decisions more transparent and predictable.
Application | Description | Techniques Used | Benefits |
---|---|---|---|
Safety Enhancement | Provides clear explanations for sudden decisions like emergency braking. | Real-time monitoring, XAI layers | Helps passengers understand vehicle actions, improves trust. |
Real-time Decision Monitoring | Tracks and explains vehicle behavior continuously. | Model-agnostic, post-hoc explanations | Makes decisions transparent and predictable. |
Navigation Systems | Explains route choices based on various factors. | Local and global interpretability | Builds trust by clarifying route preferences. |
Compliance Logging | Generates detailed records of vehicle decisions and actions. | Logging and monitoring | Useful for accident investigations, insurance, and regulatory compliance. |
User Acceptance | Provides understandable explanations for vehicle behavior. | Visual and natural language explanations | Increases comfort and confidence in self-driving technology. |
Navigation systems have been dramatically improved through XAI implementation. Rather than simply directing the vehicle, XAI-enhanced navigation can explain route choices based on multiple factors including traffic conditions, weather, road quality, and historical accident data. This transparency helps build trust by helping passengers understand why certain routes are preferred over others.
Compliance logging represents a crucial application that bridges the gap between autonomous operation and regulatory requirements. XAI systems generate detailed, intelligible records of vehicle decisions and actions, which proves invaluable for accident investigations, insurance purposes, and regulatory compliance. These logs provide clear explanations of vehicle behavior that can be understood by investigators, insurance adjusters, and legal professionals.
User acceptance has significantly improved through XAI applications in autonomous vehicles. By providing clear, understandable explanations for vehicle behavior, XAI helps passengers feel more comfortable and confident in self-driving technology. This increased transparency has proven particularly effective in helping users transition from traditional to autonomous vehicles, addressing common concerns about safety and reliability.
Challenges in Implementing XAI for Autonomous Vehicles
As self-driving vehicles become increasingly sophisticated, implementing explainable AI (XAI) systems presents several critical challenges that must be carefully addressed. These challenges go beyond technical hurdles to encompass broader concerns about privacy, integration, and ethical implications.
Data privacy and protection are among the most pressing challenges. According to the EU’s Guidelines for Trustworthy AI, autonomous vehicles collect vast amounts of personal data, including location information, driving patterns, and even biometric data of passengers. Protecting this sensitive information while maintaining the transparency needed for XAI creates a complex balancing act. Manufacturers must implement robust encryption and anonymization techniques while still allowing their AI systems to provide meaningful explanations of decisions.
System integration poses another significant hurdle. Autonomous vehicles rely on multiple interconnected systems—from sensors and cameras to navigation and control units. Implementing XAI requires seamlessly integrating explanation capabilities across these various components without compromising the vehicle’s core functionality. The challenge lies in developing standardized interfaces and protocols that enable different systems to work together while maintaining explainability.
Performance optimization remains a critical concern when implementing XAI. Traditional black-box AI models often prioritize speed and efficiency, but adding explainability layers can introduce computational overhead. Engineers must find innovative ways to generate real-time explanations without significantly impacting the vehicle’s response time or overall performance. This becomes especially crucial in emergency situations where split-second decisions are necessary.
Safety and performance cannot be compromised in the pursuit of explainability—the challenge lies in achieving both without sacrifice.
David J. Hess, Vanderbilt University
Ethical considerations add another layer of complexity to XAI implementation. Autonomous vehicles must make moral decisions in potentially dangerous situations, and these decisions need to be explainable to users, manufacturers, and regulators alike. The challenge extends to addressing potential biases in the AI systems, ensuring fair treatment across different demographic groups, and maintaining transparency in the decision-making process.
Technical scalability presents yet another hurdle. As autonomous vehicle technology evolves, XAI systems must be adaptable enough to accommodate new features and capabilities. This requires developing flexible architectures that can grow and evolve while maintaining consistent explainability across all functions. The challenge lies in creating scalable solutions that can handle increasing complexity without becoming unwieldy or difficult to maintain.
To address these challenges effectively, a multi-faceted approach combining technical innovation with strong regulatory frameworks is essential. This includes developing privacy-preserving XAI techniques, establishing industry-wide standards for system integration, and creating robust testing methodologies to ensure both performance and explainability meet the required standards for safe autonomous vehicle operation.
How SmythOS Enhances Explainable AI
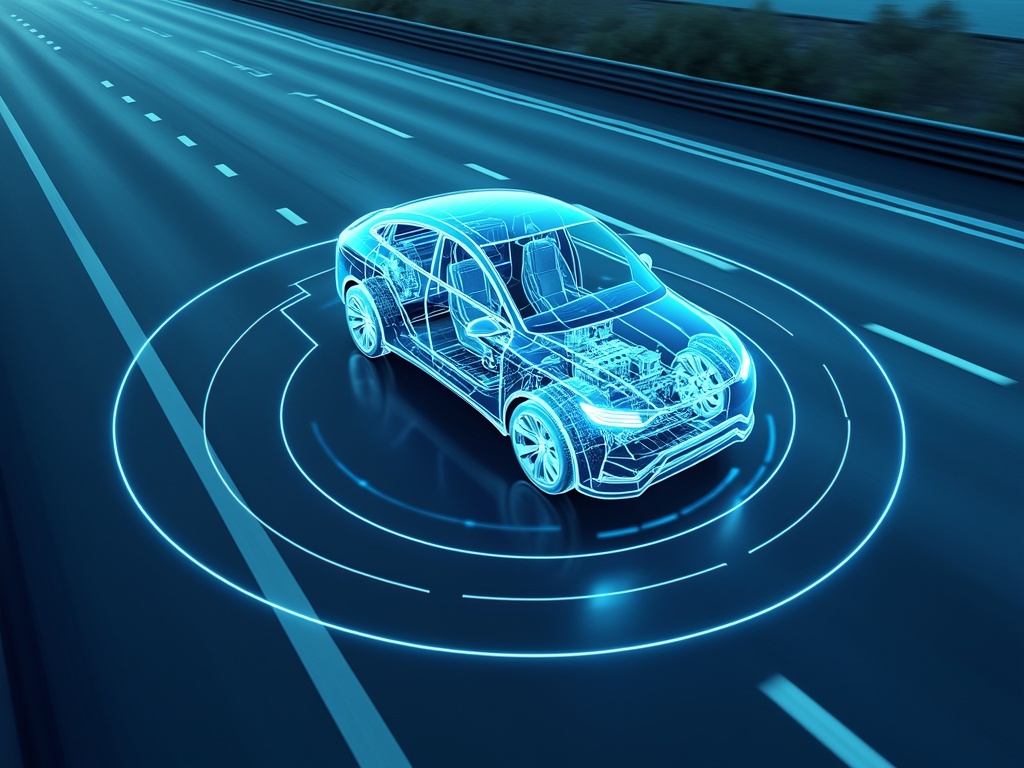
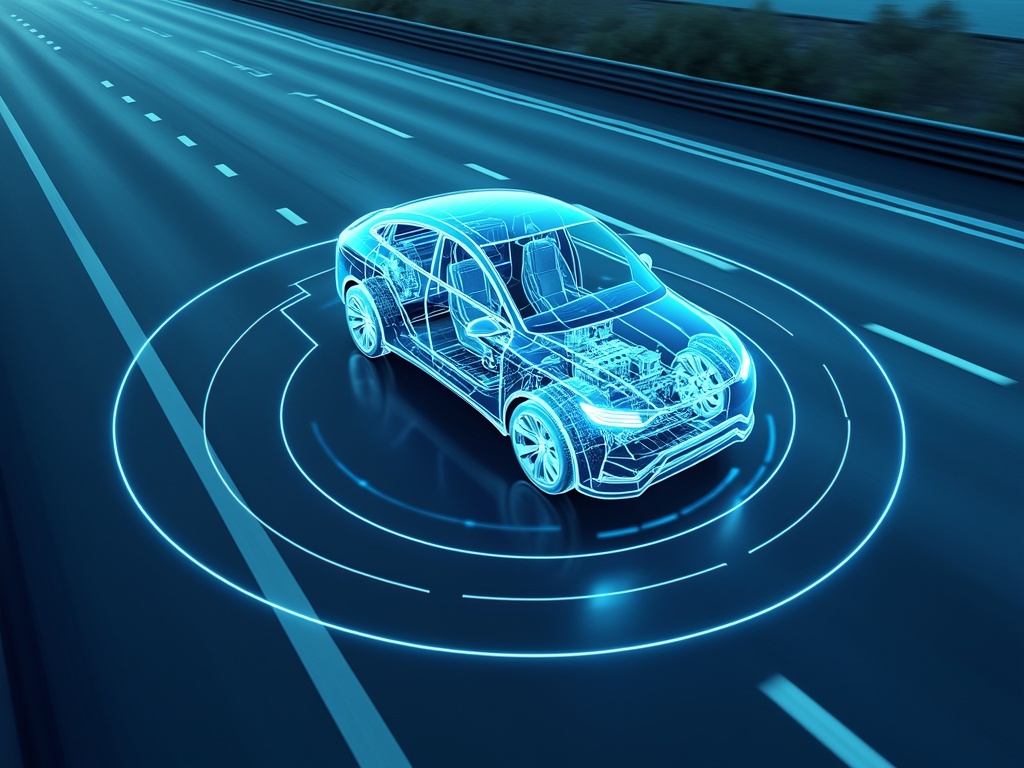
A blue-tinted autonomous vehicle with advanced sensors.
The future of autonomous vehicles relies on one essential factor: trust. SmythOS directly addresses this challenge with its comprehensive platform for explainable AI (XAI), which makes the decision-making processes of self-driving cars transparent and understandable for both developers and users.
At its core, SmythOS provides real-time monitoring capabilities that offer unprecedented visibility into how AI makes decisions. Similar to a flight recorder in an aircraft, the platform tracks and logs every choice an autonomous vehicle makes, allowing developers to see exactly why and how the AI reached specific conclusions. This level of transparency is vital for building trust in autonomous systems that must make split-second decisions on the road.
The platform’s visual debugging environment sets a new standard for XAI development. Instead of struggling with complicated code and unclear error messages, developers can visualize decision pathways in real-time. This intuitive approach significantly eases the process of identifying potential issues, validating decision logic, and ensuring the AI system operates as intended. Recent industry studies indicate that such visual tools can reduce debugging time by up to 60% while enhancing the understanding of AI behaviors.
SmythOS supports various explanation methods, acknowledging that different stakeholders require different levels of insight. For technical teams, the platform provides detailed algorithmic breakdowns and performance metrics. For safety regulators, it offers comprehensive audit trails. And for passengers, it translates complex decisions into simple, understandable explanations — for instance, clarifying why the car decided to change lanes or adjust its speed.
The platform includes enterprise-grade security controls to ensure that, while AI systems remain transparent, sensitive data is protected. This balanced approach allows organizations to uphold accountability without compromising intellectual property or personal information. With its built-in monitoring and logging capabilities, SmythOS enables companies to demonstrate their commitment to safety and transparency, thereby building public confidence in autonomous vehicle technology.
Future Directions for Explainable AI in Autonomous Vehicles
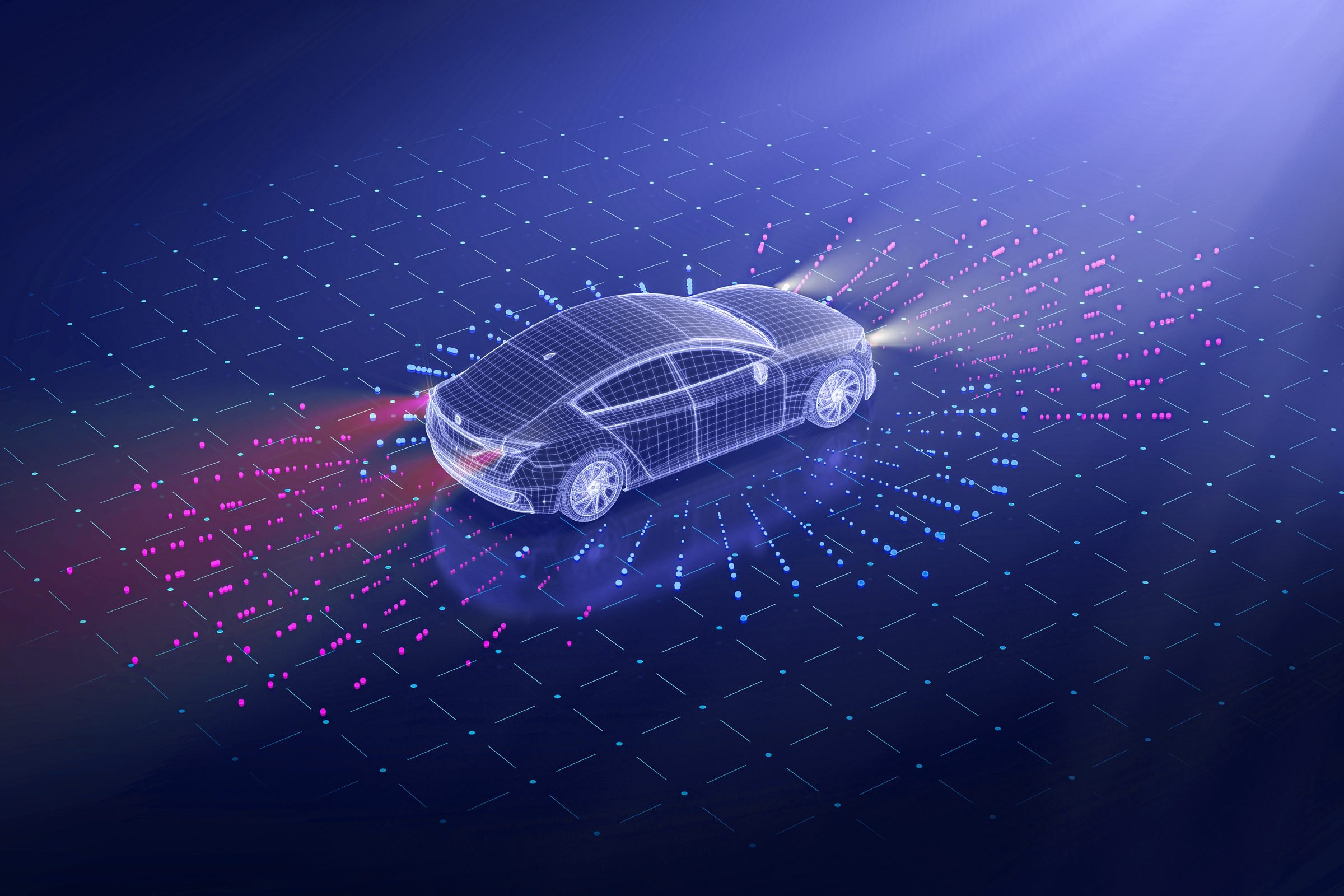
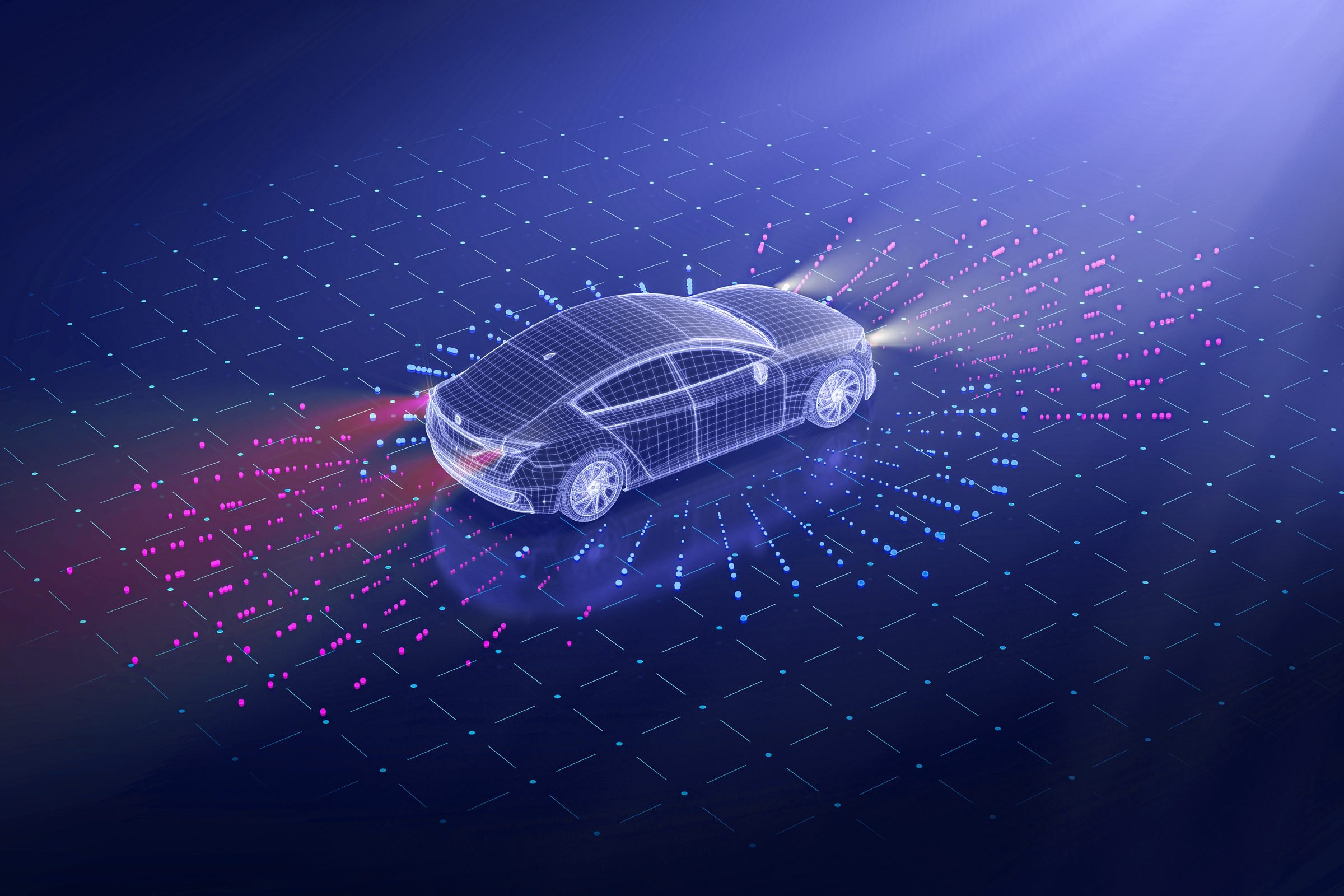
Wireframe electric car with glowing data points – Via rd.com
As autonomous vehicles evolve, integrating explainable AI with blockchain technology presents a transformative path forward. Machine learning advancements, particularly in deep vision and attention-based models, are revolutionizing how self-driving cars interpret and communicate decisions to users.
Blockchain’s immutable record-keeping capabilities offer a promising foundation for enhancing transparency in autonomous driving decisions. By creating an unalterable audit trail of AI decision-making processes, blockchain technology enables stakeholders to verify the safety and reliability of autonomous vehicles with unprecedented confidence. This integration addresses a crucial challenge in autonomous driving – establishing trust between humans and AI systems.
The emergence of more sophisticated machine learning architectures, such as model-based reinforcement learning, is paving the way for more interpretable autonomous systems. These advanced models can not only make decisions but also provide clear explanations for their actions, making autonomous vehicles more accountable and trustworthy.
Looking ahead, we can expect more robust implementations of explainable AI that combine real-time decision-making with transparent documentation of driving behaviors. This evolution will likely include advanced user interfaces that provide intuitive explanations of vehicle decisions, making autonomous driving more accessible for everyday users.
The future success of autonomous vehicles hinges on achieving both technical excellence and social acceptance. As these technologies mature, the focus will increasingly shift toward creating systems that can seamlessly explain their actions while maintaining the highest standards of safety and efficiency. This balanced approach will be crucial in building public trust and accelerating the widespread adoption of autonomous vehicles.
Last updated:
Disclaimer: The information presented in this article is for general informational purposes only and is provided as is. While we strive to keep the content up-to-date and accurate, we make no representations or warranties of any kind, express or implied, about the completeness, accuracy, reliability, suitability, or availability of the information contained in this article.
Any reliance you place on such information is strictly at your own risk. We reserve the right to make additions, deletions, or modifications to the contents of this article at any time without prior notice.
In no event will we be liable for any loss or damage including without limitation, indirect or consequential loss or damage, or any loss or damage whatsoever arising from loss of data, profits, or any other loss not specified herein arising out of, or in connection with, the use of this article.
Despite our best efforts, this article may contain oversights, errors, or omissions. If you notice any inaccuracies or have concerns about the content, please report them through our content feedback form. Your input helps us maintain the quality and reliability of our information.