Explainable AI in Healthcare: Improving Transparency and Trust in Medical Decision-Making
Picture a doctor confidently explaining to a patient exactly how an AI system arrived at their diagnosis. This isn’t science fiction—it’s the transformative reality of Explainable AI in healthcare. As artificial intelligence increasingly shapes medical decisions, understanding how these systems think has become crucial for both healthcare providers and patients.
Traditional AI models have often operated as “black boxes”—complex systems making decisions without showing their reasoning. However, recent advances in Explainable AI are revolutionizing healthcare diagnostics, offering unprecedented transparency in how artificial intelligence reaches its conclusions about patient care.
The stakes couldn’t be higher in healthcare, where decisions directly impact lives. When an AI system recommends a treatment plan or flags potential health risks, doctors need to understand the reasoning behind these recommendations. Explainable AI makes this possible by providing clear insights into the decision-making process, ensuring that healthcare professionals can verify the logic and accuracy of AI-generated insights.
What makes Explainable AI so vital in healthcare? Consider a scenario where an AI system detects early signs of a serious condition. Rather than simply flagging the concern, Explainable AI can highlight specific patterns in patient data that led to this conclusion, empowering doctors to make more informed decisions and explain their reasoning to patients.
This transparency isn’t just about building trust—it’s about saving lives. By making AI systems more interpretable, healthcare providers can catch potential errors, identify biases, and ensure that artificial intelligence truly serves its purpose: improving patient outcomes through more accurate, accountable, and transparent medical decisions.
Enhancing Diagnoses with Explainable AI
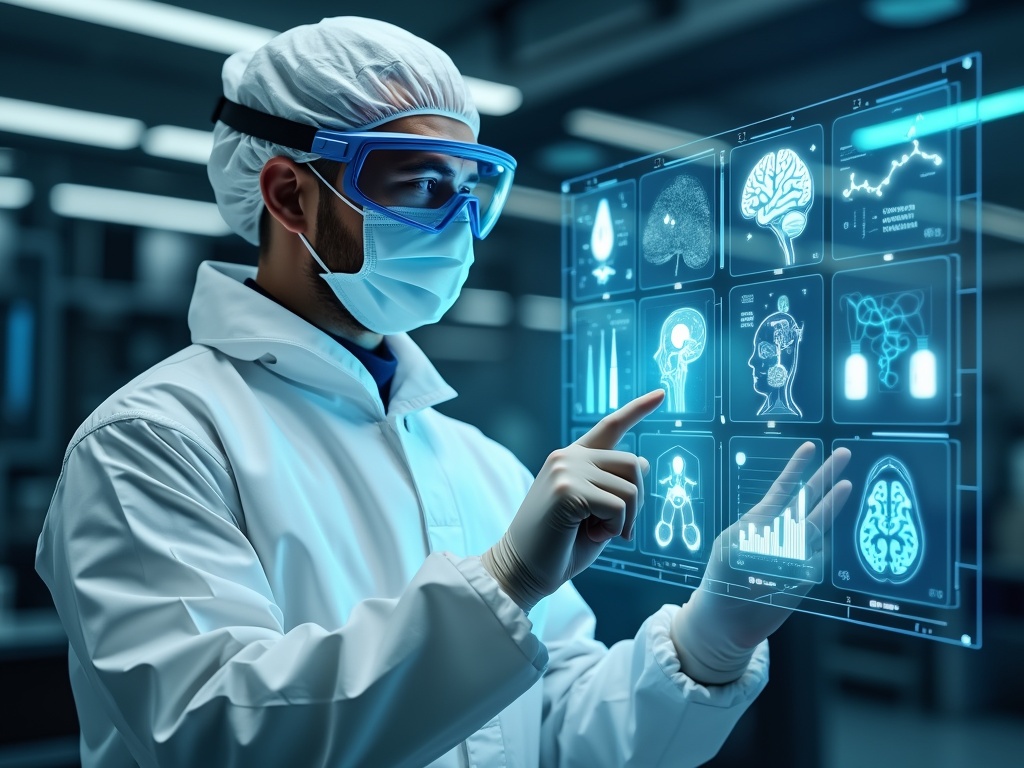
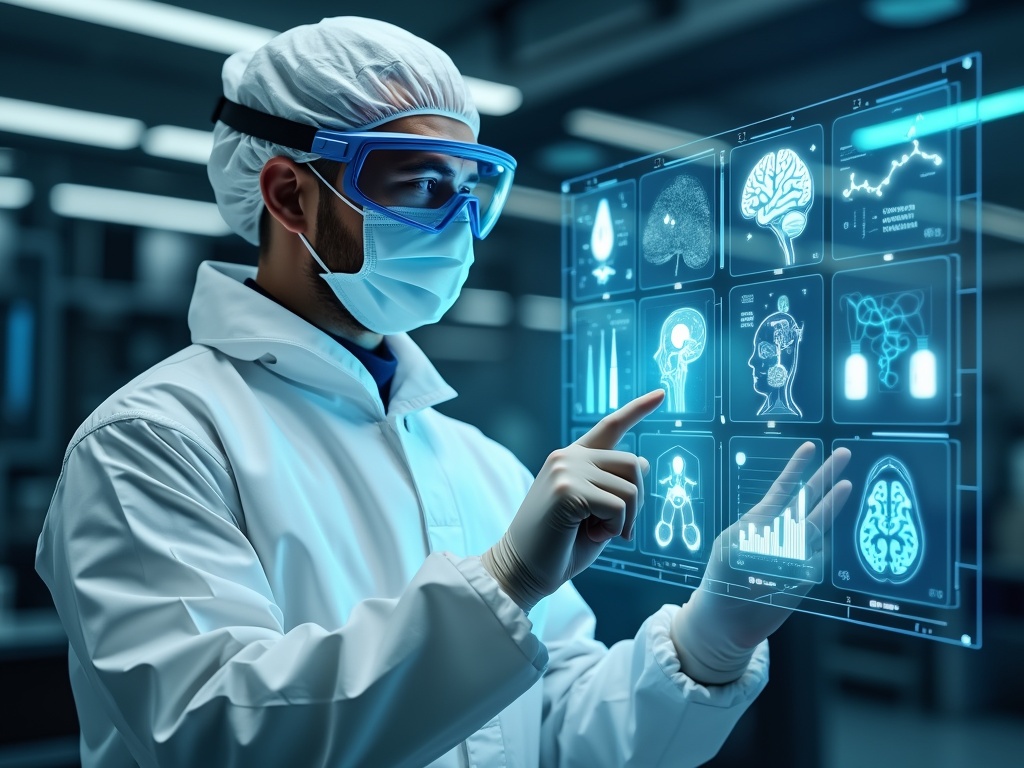
Explainable artificial intelligence (XAI) is transforming medical diagnostics by providing clear insights into how AI-driven recommendations are made. Unlike traditional ‘black box’ AI systems, XAI enables healthcare professionals to understand and validate diagnostic decisions.
A significant example of XAI’s impact is in retinopathy diagnosis, where AI systems not only detect signs of disease but also highlight specific biomarkers that inform their decisions. This transparency allows clinicians to verify AI findings against their judgment, leading to more accurate and trustworthy diagnoses.
XAI also enhances skin cancer detection by pinpointing suspicious areas within images and explaining their significance. This visual feedback helps dermatologists understand why the AI flags certain lesions as potentially cancerous, improving their decision-making.
XAI serves as an educational tool by identifying diagnostic markers and teaching healthcare providers about subtle patterns they might have overlooked. This collaborative learning process strengthens the partnership between human expertise and AI, ultimately improving diagnostic accuracy.
XAI builds trust between healthcare providers and AI technologies by providing transparent insights into decision-making processes. Clinicians can confidently integrate AI recommendations into their workflow while maintaining their professional judgment and accountability.
The integration of explainable AI in healthcare ensures that artificial intelligence serves as a powerful tool for enhancing human expertise rather than replacing it. This collaborative approach represents the future of medical diagnostics.
From International Journal of Data Analytics and Strategy, 2022
Streamlining Resource Optimization
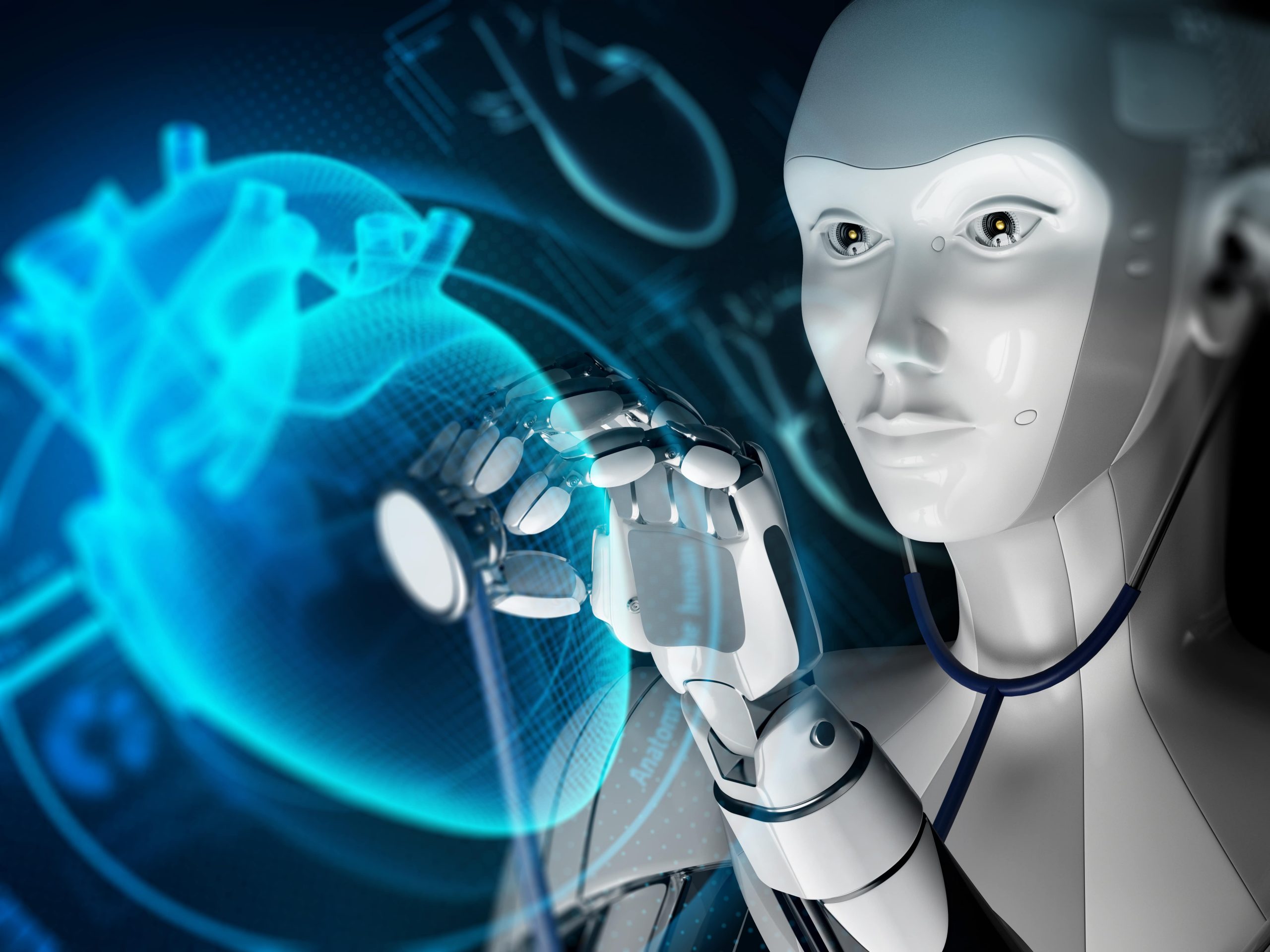
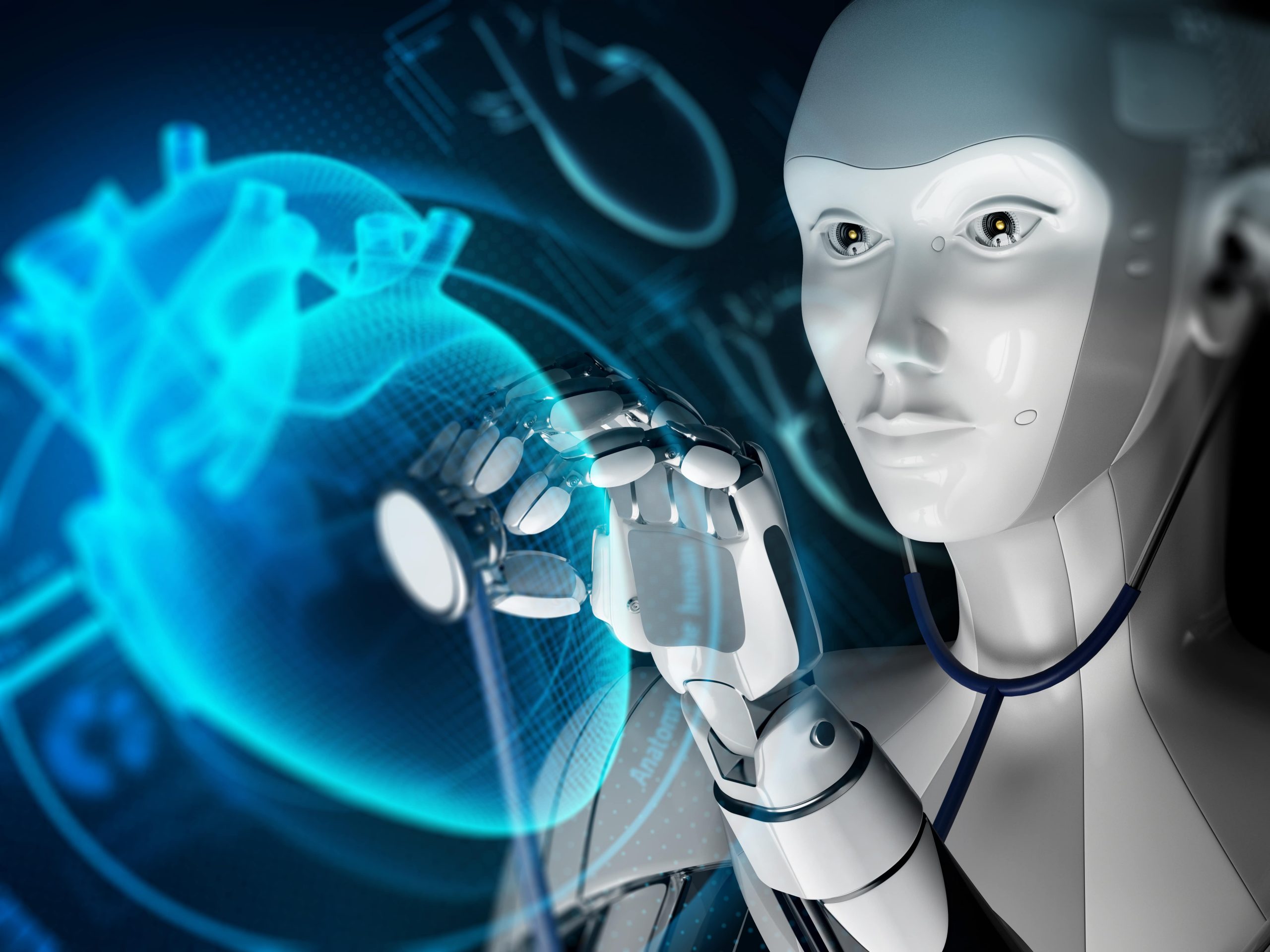
AI in healthcare: beyond diagnosis and treatment. – Via capestart.com
Explainable AI revolutionizes resource optimization by illuminating the intricate decision-making processes that often remain hidden in traditional AI systems. Through detailed analysis of data flow patterns, organizations can now understand exactly how and why AI makes specific resource allocation recommendations, leading to more informed operational decisions.
Healthcare systems particularly benefit from this transparency in resource management. As noted in a recent study, explainable AI directly addresses computation and communication challenges in resource-limited environments, enabling more efficient distribution of medical resources and staff.
Aspect | Traditional AI | Explainable AI |
---|---|---|
Transparency | Low | High |
Interpretability | Poor | Good |
Trust | Limited | Enhanced |
Bias Detection | Difficult | Feasible |
Debugging | Complex | Simplified |
Resource Allocation | Opaque | Clear |
Operational Costs | Higher due to inefficiencies | Lower with optimized deployment |
Long-term Planning | Based on historical data | Predictive with clear insights |
The impact on operational costs proves significant when organizations implement explainable AI solutions. Rather than relying on opaque algorithms, managers can visualize and verify resource allocation decisions, catching potential inefficiencies before they impact the bottom line. This visibility helps identify underutilized resources and optimize their deployment across different departments or functions.
System efficiency gains emerge through AI’s ability to process vast amounts of operational data while providing clear justifications for its recommendations. This combination of processing power and transparency enables organizations to fine-tune their resource allocation strategies based on concrete evidence rather than assumptions or historical precedents.
The practical applications extend beyond immediate resource allocation. Organizations can use these insights to develop more effective long-term planning strategies, predict future resource needs with greater accuracy, and adapt quickly to changing operational demands. This forward-looking capability helps prevent resource bottlenecks while maintaining optimal service levels across the organization.
Improving Patient Care Decision-Making
Healthcare professionals are witnessing a remarkable transformation in patient care as Explainable AI (XAI) enhances clinical decision-making.
Unlike traditional ‘black box’ AI systems, XAI provides transparent insights into how and why specific medical recommendations are made, fundamentally changing how doctors and patients collaborate on treatment decisions. In intensive care settings, where split-second decisions can mean the difference between life and death, XAI has demonstrated particular value. For example, in a groundbreaking study by Saqib et al., AI systems were able to predict patient deterioration up to 4 hours in advance while providing clear explanations for their alerts, enabling medical teams to take preventive action with confidence.
The transparency offered by XAI addresses a critical challenge in healthcare – building trust between care providers and patients. When doctors can clearly explain the reasoning behind AI-suggested treatments, patients feel more empowered to participate in their care decisions. This collaborative approach leads to better treatment adherence and ultimately improved outcomes. In the ICU specifically, XAI helps clinicians interpret complex patient data by highlighting key factors influencing a recommendation. Rather than simply suggesting a course of action, these systems explain which vital signs, lab results, or other clinical indicators shaped their analysis. This depth of insight allows medical teams to validate AI recommendations against their clinical expertise.
Perhaps most importantly, XAI transforms the doctor-patient dynamic by facilitating more meaningful conversations about care options. Instead of presenting AI recommendations as mysterious directives, physicians can walk patients through the logical reasoning process, making complex medical decisions more accessible and understandable. This enhanced communication builds the foundation for truly collaborative decision-making centered on patient needs and preferences.
Aid in the Pharmaceutical Approval Process
Artificial intelligence plays a vital role in drug development and approval. Explainable AI (XAI) has emerged as a critical technology that addresses one of the industry’s biggest challenges: bringing transparency to AI-driven decision-making in drug development.
Regulatory agencies like the FDA and EMA require clear explanations for how AI systems make recommendations about drug safety and efficacy. XAI meets this need by providing detailed insights into the reasoning behind each prediction and decision. When an AI system flags potential safety concerns or recommends specific dosing parameters, XAI tools can break down exactly which factors led to those conclusions.
The technology employs several key approaches to ensure accountability. Advanced techniques like SHAP (SHapley Additive exPlanations) and LIME (Local Interpretable Model-agnostic Explanations) allow regulators to peek inside the “black box” of AI decision-making. These tools provide visual representations and clear explanations of how different variables influence the AI’s recommendations, making complex statistical models accessible to regulatory reviewers.
XAI Approach | Description | Benefits |
---|---|---|
SHAP (SHapley Additive exPlanations) | Provides explanations by assigning importance values to each feature based on game theory. | Helps regulators understand the contribution of each factor in AI models, ensuring transparency. |
LIME (Local Interpretable Model-agnostic Explanations) | Generates local explanations by approximating the model locally around the prediction. | Allows reviewers to see how AI models make predictions for specific instances, enhancing interpretability. |
Feature Importance Analysis | Ranks features based on their impact on the model’s predictions. | Enables identification of key variables influencing AI decisions, aiding in regulatory scrutiny. |
Model Visualization Techniques | Uses visual tools to illustrate how AI models process data and make decisions. | Facilitates understanding of complex models through visual representations, improving trust. |
Beyond meeting compliance requirements, XAI accelerates the approval process by enabling faster, more informed regulatory decisions. Rather than spending months analyzing raw data, reviewers can quickly understand the key factors driving AI predictions about drug safety and effectiveness. This streamlined review process helps promising treatments reach patients sooner while maintaining rigorous safety standards.
Patient safety remains paramount throughout this technologically-enhanced approval process. XAI ensures that every AI-driven decision about drug development, from initial screening to final approval recommendations, can be thoroughly validated. When concerns arise, regulators can trace exactly how the AI reached its conclusions and verify that all safety protocols were properly followed.
Leveraging SmythOS for Explainable AI
Healthcare decisions increasingly rely on artificial intelligence, making transparency and trust paramount. SmythOS addresses these needs by providing a comprehensive platform that makes AI systems more explainable and accountable. Through its intuitive visual workflow builder, healthcare professionals can construct sophisticated AI agents while maintaining complete visibility into their decision-making processes.
At the core of SmythOS’s approach is its built-in monitoring capabilities. These tools provide unprecedented insight into how AI agents process information and arrive at conclusions. Healthcare providers can track their AI systems in real-time, ensuring decisions align with established medical protocols and ethical guidelines. This continuous oversight helps build trust among medical professionals and patients alike.
The platform’s visual tools transform complex AI operations into clear, understandable workflows. Healthcare teams can see exactly how their AI agents analyze data and make recommendations. Recent research emphasizes that such transparency is essential for identifying potential biases and communicating risks effectively in medical AI applications.
SmythOS’s integration capabilities further enhance its value in healthcare settings. The platform seamlessly connects with existing medical systems and databases while maintaining strict security protocols. This interoperability ensures that AI agents can access comprehensive patient data while adhering to privacy regulations and institutional policies.
The reliability of SmythOS stems from its robust debugging and quality assurance features. Healthcare organizations can thoroughly test their AI agents before deployment, verify their accuracy across diverse patient populations, and quickly identify any potential issues. This comprehensive approach to quality control helps ensure that AI-driven healthcare decisions remain both accurate and trustworthy.
Conclusion and Future Directions
The integration of explainable AI in healthcare represents a transformative shift in medical technology, offering transparency in clinical decision-making processes. Through advanced interpretation techniques and robust validation frameworks, these systems are gradually earning the trust of healthcare professionals while maintaining high performance standards. Understanding and validating AI-driven decisions has become instrumental in bridging the gap between technological capabilities and clinical requirements.
The evolution of explainable AI in healthcare will likely focus on several critical areas. First, developing more sophisticated explanation methods that can effectively communicate complex medical decisions to both clinicians and patients. Second, establishing standardized evaluation frameworks to assess the quality and reliability of AI explanations. Third, creating more intuitive interfaces that seamlessly integrate these explanations into clinical workflows.
Most importantly, the future success of explainable AI in healthcare hinges on continued collaboration between AI developers, healthcare providers, and regulatory bodies. This partnership will be crucial in developing systems that not only meet technical requirements but also address the practical needs of clinical environments. As healthcare systems worldwide grapple with increasing demands and complexities, explainable AI stands ready to enhance decision-making transparency while improving patient outcomes.
The journey toward fully integrated explainable AI in healthcare is ongoing, but the foundation has been laid. With continued refinement and dedication to transparency, these technologies will play an increasingly vital role in shaping the future of medical practice, ultimately leading to more informed, efficient, and patient-centered care delivery.
Last updated:
Disclaimer: The information presented in this article is for general informational purposes only and is provided as is. While we strive to keep the content up-to-date and accurate, we make no representations or warranties of any kind, express or implied, about the completeness, accuracy, reliability, suitability, or availability of the information contained in this article.
Any reliance you place on such information is strictly at your own risk. We reserve the right to make additions, deletions, or modifications to the contents of this article at any time without prior notice.
In no event will we be liable for any loss or damage including without limitation, indirect or consequential loss or damage, or any loss or damage whatsoever arising from loss of data, profits, or any other loss not specified herein arising out of, or in connection with, the use of this article.
Despite our best efforts, this article may contain oversights, errors, or omissions. If you notice any inaccuracies or have concerns about the content, please report them through our content feedback form. Your input helps us maintain the quality and reliability of our information.