Chatbots and Analytics
Ever wondered how those friendly chatbots you interact with online are constantly improving? The secret lies in the fascinating world where chatbots and analytics intersect. This article reveals how developers harness data to create smarter, more engaging digital assistants.
Chatbots have evolved from simple rule-based programs to sophisticated AI-powered tools that learn and adapt with each interaction. But how do we measure their effectiveness? Analytics plays a crucial role. By tracking key performance metrics, developers can fine-tune these digital conversationalists for better usability and engagement.
Imagine a chatbot that not only answers your questions but anticipates your needs before you even ask. This isn’t science fiction—it’s the reality that data analysis is making possible. From response times to sentiment analysis, we’ll explore the crucial metrics that shape the chatbots of tomorrow.
Whether you’re a curious user or a developer looking to improve, get ready to dive into the data-driven landscape of modern chatbot development. Discover how numbers and algorithms are creating more human-like digital interactions every day.
Main Takeaways:
- Learn about the key metrics used to evaluate chatbot performance
- Understand how analytics drive improvements in usability and engagement
- Discover the latest trends in data-driven chatbot development
- Gain insights into creating more effective and user-friendly chatbot experiences
Understanding Chatbot Analytics
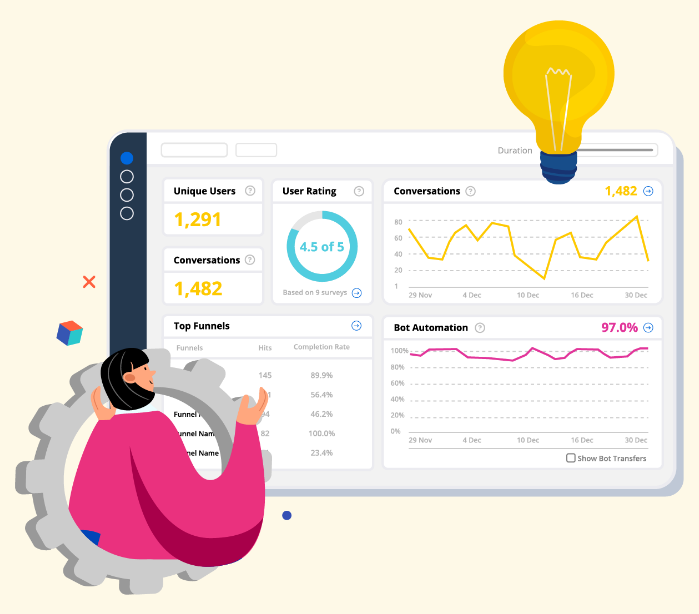
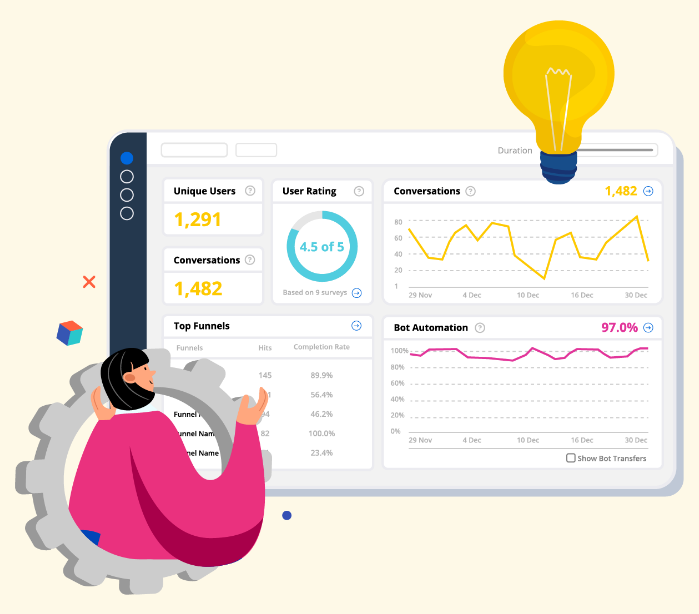
Chatbots have become indispensable tools for businesses seeking to enhance customer engagement and streamline operations. But how do we know if these virtual assistants are truly making an impact? Enter chatbot analytics – the key to unlocking valuable insights about your bot’s performance and user interactions.
Chatbot analytics involves the meticulous collection and analysis of data generated from user interactions with chatbots. Think of it as a health check-up for your digital helper, providing vital signs that indicate how well it’s performing its job. By diving into these metrics, businesses can fine-tune their chatbots, ensuring they are not just functional but truly exceptional in serving customer needs.
Decoding User Engagement
One of the cornerstone metrics in chatbot analytics is user engagement. This isn’t just about how many people chat with your bot – it’s about the quality of those interactions. Are users finding value in the conversation, or are they quickly bouncing away in frustration?
To gauge user engagement, we look at several key indicators:
- Session duration: How long are users chatting with the bot?
- Message volume: How many messages are exchanged in a typical conversation?
- Retention rate: Are users coming back for more chats?
For example, if your chatbot is helping customers track their orders, a high retention rate might indicate that users find the service helpful and return to check on multiple orders. On the flip side, if most conversations end after just one or two messages, it could signal that users aren’t getting the information they need.
Navigating the Conversation Flow
Another crucial aspect of chatbot analytics is understanding the conversation flow. This is all about how smoothly users move through the chat, from their initial query to resolution. A well-designed conversation flow feels natural and intuitive, much like chatting with a knowledgeable friend.
Key metrics for assessing conversation flow include:
- Goal completion rate: How often do users successfully achieve their objectives?
- Fallback rate: How frequently does the bot fail to understand user queries?
- Conversation steps: How many interactions does it take to resolve a user’s issue?
Imagine you’re using a chatbot to book a flight. A smooth conversation flow might look like this: you state your destination, the bot offers date options, you select one, and voila – you’re booked! If, instead, you find yourself repeating information or hitting dead ends, that’s a sign the conversation flow needs work.
The Power of Data Analysis
At its core, chatbot analytics is all about turning raw data into actionable insights. By crunching the numbers on user interactions, businesses can uncover patterns and trends that might otherwise go unnoticed.
For instance, data analysis might reveal that users frequently ask about a product feature that isn’t well-explained on your website. Armed with this information, you could update your site content or train your chatbot to provide more detailed responses on this topic.
Moreover, advanced analytics tools can perform sentiment analysis, gauging the emotional tone of user messages. This can be invaluable for identifying pain points in the customer journey and addressing them proactively.
Putting It All Together
By leveraging chatbot analytics, businesses can create more intelligent, responsive, and user-friendly bots. It’s not just about collecting data – it’s about using those insights to continuously refine and improve the chatbot experience.
Remember, the goal isn’t to replace human interaction entirely, but to complement it. A well-optimized chatbot can handle routine queries efficiently, freeing up human agents to tackle more complex issues. This synergy, guided by data-driven insights, is the key to delivering exceptional customer service in the digital age.
As we continue to navigate the evolving landscape of AI and customer engagement, one thing is clear: chatbot analytics will play an increasingly crucial role in shaping the future of digital interactions. By embracing these tools and insights, businesses can ensure their chatbots aren’t just keeping up with user expectations – they’re exceeding them.
Key Metrics to Track
To harness the power of your chatbot and ensure it delivers value, monitor its performance carefully. Here are the critical metrics that can determine your chatbot’s success.
Total Interactions: The Pulse of Your Chatbot
Imagine you’ve just launched a new chatbot for your e-commerce site. How do you know if it’s being used? Enter the total interactions metric. This number shows how many conversations your chatbot is having, giving you an overview of its reach and adoption.
For instance, if your chatbot logged 10,000 interactions last month but increased to 15,000 this month, that’s a sign of growing user engagement. However, if you notice a sudden drop, it might be time to investigate – perhaps there’s a technical glitch or your bot’s responses aren’t effective.
Average Chat Duration: Quality Time with Your Users
Next is average chat duration. This metric is like the Goldilocks of chatbot analytics – you want it to be just right. Too short, and your bot might not be providing enough value. Too long, and it could be struggling to resolve queries efficiently.
Let’s say your customer service chatbot averages 2-minute conversations. That might be perfect for quick inquiries. But if you’re seeing 10-minute averages for simple questions like “What are your store hours?”, it’s a red flag that your bot’s conversational flow needs streamlining.
Goal Completion Rate: The Bottom Line
This metric tells you how often your chatbot helps users achieve their objectives, whether that’s making a purchase, finding information, or resolving an issue.
A high goal completion rate is the holy grail of chatbot performance. For example, if your bot is designed to help users book appointments, and 80% of conversations end with a successful booking, you’re on the right track. But if only 30% of users are making it to the finish line, it’s time to optimize that conversation flow.
Remember, a chatbot that can’t help users complete their goals is like a GPS that can’t get you to your destination – frustrating and ultimately useless.
User Engagement: Beyond the Numbers
Tracking user engagement involves looking at several indicators that paint a picture of how users interact with your bot. This includes metrics like the number of messages per conversation, repeat usage, and user ratings.
For instance, if users are consistently engaging in multi-message conversations and coming back to use the bot again, that’s a strong sign your chatbot is providing value. On the flip side, if you’re seeing a lot of one-message interactions or low repeat usage, it might be time to reassess your bot’s conversational design or the value it’s offering.
The Power of Continuous Improvement
Tracking these metrics isn’t a one-time task. It’s an ongoing process of monitoring, analyzing, and optimizing. By keeping a close eye on these key performance indicators, you can continually refine your chatbot’s performance, ensuring it remains an effective tool for engaging users and driving business results.
A well-optimized chatbot isn’t just a cost-saver – it’s a powerful asset that can enhance customer experience, drive conversions, and give your business a competitive edge. So dive into the data and make your chatbot the best it can be!
Analyzing User Sentiment
Understanding the emotional nuances of user responses is crucial in chatbot interactions. User sentiment analysis allows businesses to decode customer emotions and tailor their chatbot responses accordingly. This approach goes beyond mere keyword recognition, delving into the complexity of human emotions expressed through text.
Imagine a customer reaching out to a chatbot, frustrated after a failed attempt to return a product. Without sentiment analysis, the chatbot might respond with a generic, ‘How may I assist you today?’ But with sentiment analysis, it could detect the user’s frustration and respond with, ‘I’m sorry to hear you’re having trouble. Let’s get this sorted out quickly for you.’ This empathetic response can dramatically shift the tone of the interaction.
How Sentiment Analysis Works
Sentiment analysis employs natural language processing (NLP) and machine learning algorithms to categorize text as positive, negative, or neutral. It’s about understanding the intensity and context of emotions. Here’s a simplified breakdown of the process:
- Text Preprocessing: The user’s input is cleaned and normalized, removing irrelevant characters and standardizing the format.
- Tokenization: The text is broken down into individual words or phrases.
- Feature Extraction: Key elements that might indicate sentiment are identified, such as adjectives, adverbs, and emoticons.
- Classification: The extracted features are run through a trained model that classifies the overall sentiment.
- Output: The analysis produces a sentiment score, often ranging from -1 (very negative) to 1 (very positive).
For instance, the statement ‘This product is absolutely fantastic!’ would likely receive a high positive score, while ‘I’ve been waiting for hours and still no response’ would register as negative.
Benefits of Sentiment Analysis in Chatbot Interactions
Implementing sentiment analysis in chatbot systems offers several benefits that can enhance user satisfaction:
- Personalized Responses: Chatbots can adjust their tone and content based on the user’s emotional state, creating a more empathetic interaction.
- Improved Issue Resolution: By identifying negative sentiment early, chatbots can escalate issues to human agents more quickly, preventing frustration from escalating.
- Enhanced Customer Insights: Aggregated sentiment data provides valuable insights into overall customer satisfaction and areas for improvement.
- Proactive Support: Chatbots can offer additional assistance or resources when they detect uncertainty or confusion in user responses.
- Brand Reputation Management: By addressing negative sentiments promptly, companies can mitigate potential PR issues before they spiral.
Practical Applications
Consider an e-commerce chatbot interacting with a customer:
Customer: ‘I’ve been trying to track my order for days, and it’s still not here. This is ridiculous!’
Frustrated e-commerce customer
Without sentiment analysis, the chatbot might respond with a standard tracking information request. However, with sentiment analysis, it could respond:
‘I’m truly sorry for the inconvenience you’re experiencing. I can sense your frustration, and I want to help resolve this immediately. Let me look into the status of your order right away and explore expedited options for you.’
This response acknowledges the customer’s emotions, potentially diffusing the situation and leading to a more positive outcome.
Challenges and Considerations
While sentiment analysis offers potential, it’s not without challenges. Sarcasm, cultural nuances, and context-dependent phrases can sometimes confuse even sophisticated algorithms. For instance, the phrase ‘Great, just what I needed!’ could be genuinely positive or deeply sarcastic, depending on the context.
Moreover, ethical considerations come into play. Companies must be transparent about their use of sentiment analysis and ensure they’re not crossing privacy boundaries. It’s a balance between providing personalized service and respecting user privacy.
As we continue to refine sentiment analysis technologies, the future of chatbot interactions looks bright. By understanding and responding to user emotions in real-time, businesses can create more meaningful, satisfying, and human-like conversational experiences. The key lies in continuous learning, ethical implementation, and always keeping the user’s needs at the forefront of technological advancements.
Improving Chatbot Accuracy
Chatbot accuracy is essential for seamless human-computer interaction. When a chatbot consistently understands and responds correctly to user queries, it boosts user satisfaction and builds trust in the AI system. Here are some cutting-edge methods to enhance chatbot accuracy, focusing on refining Natural Language Processing (NLP) algorithms and leveraging diverse training datasets.
Refining NLP Algorithms: The Brain of Your Chatbot
At the heart of every chatbot lies its NLP engine, which interprets human language and formulates responses. To boost accuracy, developers are constantly fine-tuning these algorithms. One promising approach involves incorporating context awareness into NLP models. This allows chatbots to understand not just the literal meaning of words, but also the nuances and intent behind them.
For instance, consider the query “How do I reset my password?” A basic chatbot might provide a generic response about password resets. However, an advanced NLP algorithm could recognize if this user has attempted resets before, tailoring its response accordingly. This contextual understanding significantly improves the accuracy and relevance of chatbot responses.
Another breakthrough in NLP refinement is the use of transfer learning. This technique allows chatbots to leverage knowledge gained from one task to improve performance on another. Imagine a chatbot trained on general customer service queries suddenly needing to handle technical support questions. Transfer learning enables it to adapt more quickly and accurately to this new domain.
The quality of your training data matters more than its quantity. Large volumes of incorrect data can be just as problematic as insufficient data.
Insights from ServisBot
Diverse Training Datasets: Fuel for Accuracy
Just as a human learns from diverse experiences, a chatbot’s accuracy improves with exposure to varied training data. However, it’s not just about quantity – quality and diversity are paramount. Here’s why:
1. Real-world representation: By incorporating a wide range of user inputs, including colloquialisms, typos, and various phrasing styles, chatbots become adept at handling real-world conversations. This diversity helps bridge the gap between controlled testing environments and actual user interactions.
2. Reducing bias: Diverse datasets help minimize biases that can creep into AI systems. For example, if a chatbot is trained primarily on data from one demographic, it may struggle to understand or accurately respond to users from different backgrounds.
3. Handling edge cases: Unusual or complex queries often reveal a chatbot’s limitations. By including these edge cases in training data, developers can significantly enhance the chatbot’s ability to handle unexpected inputs accurately.
A fascinating approach to diversifying datasets is the use of adversarial examples. These are inputs specifically designed to challenge the chatbot’s understanding. By exposing the AI to these tricky scenarios during training, it becomes more robust and accurate in real-world applications.
Actionable Tips for Improving Chatbot Accuracy
For those looking to enhance their chatbot’s performance, here are some practical steps:
1. Continuous learning: Implement a feedback loop where the chatbot learns from each interaction. This allows for ongoing improvement based on real user data.
2. Regular audits: Periodically review your chatbot’s performance. Look for patterns in misunderstood queries or inaccurate responses and use these insights to refine your NLP models and training data.
3. User-centric design: Involve actual users in your testing process. Their feedback can provide invaluable insights into areas where accuracy needs improvement.
4. Hybrid approaches: Consider combining rule-based systems with machine learning models. This can provide a safety net for handling common queries while allowing for the flexibility to tackle more complex interactions.
5. Semantic understanding: Invest in tools that can detect semantic overlap within your chatbot’s intents. This helps in identifying and resolving confusion points, leading to more accurate responses.
By focusing on these areas, businesses can significantly enhance their chatbots’ accuracy, leading to better user experiences and more efficient customer service operations. Remember, the goal isn’t to create a chatbot that passes the Turing test, but one that consistently and accurately meets your users’ needs.
Leveraging Predictive Analytics
Predictive analytics is emerging as a game-changing technology for chatbots, enhancing user interactions significantly. By leveraging data and advanced algorithms, chatbots can now anticipate user needs before they’re expressed, creating a more intuitive experience.
Predictive analytics in chatbots involves analyzing historical data to identify patterns and make informed guesses about future user behavior. This goes beyond keyword matching to understand the context and intent behind user queries. For example, if a user frequently asks about order status in the evenings, a predictive chatbot might proactively offer shipping updates at that time.
Integrating predictive models into chatbots personalizes interactions. By examining past purchases, browsing history, and demographic information, chatbots can tailor responses to each user. Imagine a retail chatbot suggesting products based on what similar customers have purchased and enjoyed.
Predictive analytics also enhances the efficiency of chatbot interactions. By anticipating common follow-up questions or potential issues, chatbots can provide comprehensive initial responses, reducing the need for back-and-forth exchanges. This saves time for users and reduces the load on human customer service representatives.
The impact of predictive analytics on user experience is profound. When chatbots seem to understand users, it leads to increased engagement and satisfaction. For businesses, this translates to higher conversion rates, improved customer loyalty, and valuable insights into consumer behavior.
Practical Applications of Predictive Analytics in Chatbots
Here are some ways predictive analytics enhances chatbot functionality:
Proactive Customer Service: Chatbots can identify potential issues based on usage patterns and offer solutions preemptively. For instance, a telecom chatbot might detect unusual data usage and suggest a plan upgrade before the user experiences overage charges.
Intelligent Product Recommendations: E-commerce chatbots can analyze browsing behavior, purchase history, and current trends to suggest products users are likely to buy. This targeted approach can boost sales and improve the shopping experience.
Dynamic Conversation Flows: Predictive models allow chatbots to adjust their conversation paths in real-time based on user responses and behaviors. This creates more natural, context-aware interactions.
Sentiment Analysis: By analyzing the tone and content of user messages, chatbots can gauge emotional states and adjust responses accordingly. This empathetic approach can de-escalate tense situations and provide more satisfying resolutions.
Looking ahead, the integration of predictive analytics in chatbots will become even more sophisticated. With advancements in machine learning and natural language processing, chatbots will become increasingly adept at understanding and anticipating human needs, blurring the line between artificial and human intelligence in customer service interactions.
Predictive analytics is not just about answering questions faster; it’s about knowing what questions to ask and when to ask them. It’s the difference between a chatbot that responds and one that truly understands.
Leveraging predictive analytics in chatbots represents a significant leap forward in artificial intelligence and customer service technology. By anticipating user needs, personalizing interactions, and continuously learning from each engagement, chatbots equipped with predictive capabilities are setting new standards for efficiency and user satisfaction.
As this technology evolves, businesses embracing these intelligent chatbots will be at the forefront of customer experience innovation, reaping the rewards of increased loyalty and operational efficiency.
Conclusion and SmythOS Benefits
Chatbot analytics are crucial for enhancing the performance and user experience of AI-driven conversational agents. Robust analytics tools allow developers to fine-tune their chatbots, leading to better functionality and higher user satisfaction rates. In this context, SmythOS stands out as a transformative platform for chatbot development and optimization.
SmythOS offers comprehensive built-in monitoring and logging capabilities, enabling developers to track performance metrics in real-time. This facilitates the quick identification of bottlenecks and rapid adjustments to chatbot behavior, ensuring continuous improvement and successful deployments. The platform’s visual workflow builder converts complex AI logic into an intuitive, drag-and-drop interface, allowing developers to create sophisticated decision-making processes easily.
Beyond analytics, SmythOS accelerates the entire development lifecycle for autonomous agents. Tasks that once took weeks can now be completed in days or even hours, allowing businesses to iterate on their chatbot solutions rapidly. This efficiency saves time and resources and keeps companies at the forefront of AI innovation.
For developers aiming to create intelligent, scalable chatbot solutions, SmythOS offers unparalleled advantages. Its focus on enterprise-grade security, seamless API integrations, and user-friendly interface makes it an ideal choice for businesses across various industries. By using SmythOS, developers can unlock the full potential of chatbot technology, delivering exceptional user experiences while staying ahead in the evolving world of conversational AI.
SmythOS: Where advanced chatbot development meets simplicity and security. Build smarter, deploy faster, and manage with confidence.
As the demand for sophisticated AI agents grows, platforms like SmythOS become essential. By democratizing access to advanced AI capabilities, SmythOS empowers developers to bring their visionary chatbot concepts to life. The future of conversational AI is bright, and with SmythOS, you’re well-equipped to shape it.
Last updated:
Disclaimer: The information presented in this article is for general informational purposes only and is provided as is. While we strive to keep the content up-to-date and accurate, we make no representations or warranties of any kind, express or implied, about the completeness, accuracy, reliability, suitability, or availability of the information contained in this article.
Any reliance you place on such information is strictly at your own risk. We reserve the right to make additions, deletions, or modifications to the contents of this article at any time without prior notice.
In no event will we be liable for any loss or damage including without limitation, indirect or consequential loss or damage, or any loss or damage whatsoever arising from loss of data, profits, or any other loss not specified herein arising out of, or in connection with, the use of this article.
Despite our best efforts, this article may contain oversights, errors, or omissions. If you notice any inaccuracies or have concerns about the content, please report them through our content feedback form. Your input helps us maintain the quality and reliability of our information.