What is Generative AI vs AI: Key Differences and Applications Explained
The world of artificial intelligence stands at a fascinating crossroads. Traditional AI has quietly powered our daily digital experiences for years through search engines and fraud detection systems. Meanwhile, generative AI has captured global attention with its ability to create, imagine, and innovate in surprisingly human-like ways.
Picture two distinct branches of the same technological tree: traditional AI operates like a meticulous librarian, excelling at organizing, analyzing, and retrieving information based on specific rules. In contrast, generative AI functions more like an artist, capable of creating entirely new content – from poetry and paintings to code and conversations – by learning patterns from vast amounts of data.
As noted in a recent analysis by Blue Prism, companies implementing generative AI alongside traditional systems have seen remarkable results, with some businesses reporting 60% faster response times to customer inquiries. This dramatic improvement hints at the transformative potential when both forms of AI work in concert.
Yet these technologies serve fundamentally different purposes. Traditional AI shines in performing specific, well-defined tasks with consistent precision – think spam detection or product recommendations. Generative AI pushes the boundaries of what machines can do by creating new content, solving open-ended problems, and even engaging in creative endeavors previously thought to be exclusively human domains.
Exploring the distinct capabilities, challenges, and real-world applications of both traditional and generative AI, one thing becomes clear: we are witnessing not just an evolution in technology, but a revolution in how machines can complement and enhance human potential. The question isn’t which type of AI is superior – it’s how we can harness the unique strengths of each to shape a more innovative and efficient future.
Understanding Traditional AI
Traditional AI, commonly known as narrow AI, represents a focused branch of artificial intelligence designed to excel at specific, predefined tasks. Unlike more advanced forms of AI, these systems operate within carefully defined boundaries using established rules and algorithms.
Consider your email’s spam filter—a perfect example of traditional AI in action. It methodically scans incoming messages, applying predetermined criteria to identify and isolate unwanted emails. According to research, Google’s TensorFlow AI blocks over 100 million spam messages daily, demonstrating the remarkable efficiency of these narrowly-focused systems.
Voice assistants like Siri and Alexa showcase another practical application of traditional AI. These digital helpers excel at understanding and responding to specific voice commands, from setting alarms to answering basic questions. However, they can only perform tasks they’ve been explicitly programmed to handle—they won’t suddenly develop the ability to write poetry or compose music.
Streaming services like Netflix heavily rely on traditional AI through their recommendation engines. These systems analyze your viewing history and preferences to suggest content you might enjoy. While impressive in their ability to process vast amounts of user data, these engines remain confined to their singular purpose of content recommendation.
Application | Description |
---|---|
Speech Recognition | AI systems like Siri and Alexa understand and interpret human speech, allowing devices to perform tasks based on voice commands. This technology is used in virtual assistants, healthcare, customer service, and accessibility for people with disabilities. |
Image Recognition | AI systems can recognize objects, faces, and patterns in images with remarkable accuracy. This technology is used in autonomous vehicles, security systems, and medical imaging to help detect and diagnose diseases faster and more accurately. |
Natural Language Processing (NLP) | NLP enables computers to understand and generate human language. Applications include chatbots, language translation, sentiment analysis, and content recommendation systems. |
Facial Recognition | Used in security systems, social media platforms, and mobile devices for user authentication, AI algorithms recognize and analyze faces to identify individuals. |
Recommendation Systems | Used by e-commerce websites, streaming services, and social media platforms to provide personalized recommendations based on user preferences and behavior. |
Medical Diagnosis | AI algorithms analyze medical images to detect patterns and assist doctors in diagnosing diseases like cancer, identifying abnormalities, and improving patient outcomes. |
Autonomous Vehicles | AI plays a vital role in self-driving cars by processing sensor data, making decisions, and navigating the environment in real-time to ensure passenger safety and efficient transportation. |
The beauty of traditional AI lies in its predictability and reliability within its defined scope. Unlike more advanced AI systems that aim to be generalists, traditional AI systems are specialists, focusing on doing one thing exceptionally well. This specialized nature makes them particularly valuable for businesses seeking to automate specific tasks or enhance particular aspects of their operations.
Introduction to Generative AI
Imagine asking a computer to paint like Van Gogh, compose a symphony in the style of Mozart, or write poetry that captures the essence of Maya Angelou. Just a decade ago, this might have seemed like science fiction. Today, it is reality, thanks to generative AI—a breakthrough reshaping our understanding of artificial intelligence.
Unlike traditional AI systems that analyze and categorize existing information, generative AI can create entirely new content. This technology leverages sophisticated architectures like Generative Adversarial Networks (GANs), where two neural networks engage in an intricate dance—one creating content while the other evaluates its authenticity.
The capabilities of generative AI extend far beyond simple mimicry. When trained on vast datasets of human-created works, these systems can generate original images that capture subtle nuances of artistic styles, compose musical pieces that evoke genuine emotion, and craft written content that flows with natural eloquence. This technology can understand and implement abstract concepts, not just follow predetermined rules.
Consider how a generative AI system approaches the task of creating an image of a ‘happy dog playing in autumn leaves.’ It doesn’t just paste together existing pictures—it understands the concepts of ‘happiness,’ ‘playfulness,’ ‘dogs,’ and ‘autumn,’ then creates an entirely original image that combines these elements in a coherent and often surprising way. This level of creative synthesis was previously thought to be uniquely human.
The impact of this technology extends into practical applications across numerous fields. Architects use it to generate innovative building designs, scientists employ it to visualize complex molecular structures, and artists harness it as a tool for expanding their creative horizons. As these systems evolve, they are not replacing human creativity but augmenting it, opening up new possibilities for collaboration between human ingenuity and artificial intelligence.
Functional Differences
Traditional AI and generative AI represent two distinct approaches to artificial intelligence, each serving fundamentally different purposes. Traditional AI excels at analyzing existing data to make predictions and decisions within clearly defined boundaries. It’s like having a highly skilled analyst who can process vast amounts of information but can only work with what already exists.
In contrast, generative AI takes a more proactive approach, capable of creating entirely new content using learned patterns. Think of it as having a creative artist who can produce original works based on their understanding of various styles and techniques. This fundamental distinction shapes how these technologies are applied across different industries.
Traditional AI’s functionality centers on pattern recognition and data analysis, making it particularly effective for tasks like fraud detection, diagnostic systems, and predictive maintenance. These systems follow predetermined rules and algorithms, ensuring consistent and reliable outputs. For example, when a traditional AI system analyzes medical images, it specifically looks for known patterns that indicate certain conditions, providing doctors with data-driven insights.
Generative AI, however, pushes beyond analysis into creation. Rather than simply identifying patterns, it uses its understanding of data relationships to generate new, original content. This capability enables it to write stories, create artwork, compose music, or even develop new product designs. Unlike its traditional counterpart, generative AI can produce multiple unique outputs from the same input, demonstrating a level of creativity previously unseen in artificial intelligence.
Aspect | Traditional AI | Generative AI |
---|---|---|
Primary Function | Analyzes, classifies, or predicts based on data | Generates new content resembling training data |
Examples | Spam detection, Image recognition | Text generators, GANs |
Flexibility | Rigid, focused on predefined tasks | Highly flexible in terms of output |
Training Data | Can work with smaller, task-specific data | Needs large, diverse datasets |
Use Cases | Classification, prediction, decision-making | Content creation, creative tasks |
Challenges | Lacks creativity, data-dependent | Resource-intensive, difficult to interpret |
Traditional AI is reactive – focused on processing and analyzing data to provide predictions or insights. In contrast, generative AI is proactive – capable of creating something new using learned data patterns.
MIT Open Learning
The implications of these functional differences are profound. While traditional AI continues to revolutionize data-driven decision-making processes, generative AI is opening new frontiers in creative industries and innovation. Together, they represent complementary approaches that are reshaping how we interact with technology and pushing the boundaries of what machines can accomplish.
Applications in Various Domains
Artificial intelligence has evolved far beyond theoretical research, delivering tangible value across countless industries. From healthcare facilities to agricultural fields, AI systems are reshaping how we work, live, and solve complex challenges.
In healthcare, AI demonstrates remarkable versatility through sophisticated diagnostic tools and automation systems. Platforms like Google’s DeepMind Health give doctors crucial early warnings about life-threatening conditions, while AI-powered imaging analysis accelerates the detection of diseases with unprecedented accuracy.
The agricultural sector has witnessed a technological revolution through AI integration. Precision farming systems now leverage computer vision and machine learning to analyze soil conditions, optimize crop yields, and automate harvesting processes. These innovations help farmers make data-driven decisions while significantly reducing resource waste.
In the retail and e-commerce landscape, AI powers sophisticated recommendation engines and inventory management systems. Major platforms like Amazon harness AI to personalize shopping experiences, with their recommendation engines driving up to 35% of total revenue. Meanwhile, brands like H&M use machine learning to analyze store receipts and returns, optimizing inventory levels and reducing overstock by up to 40%.
The financial sector relies heavily on AI for risk assessment, fraud detection, and automated trading. Companies like PayPal have partnered with Deep Instinct to combat fraud through deep learning, while robo-advisors democratize wealth management through algorithm-driven portfolio optimization.
Perhaps most transformatively, generative AI is revolutionizing creative industries. These systems can now produce high-quality text, images, and designs, augmenting human creativity rather than replacing it. From assisting writers with content creation to helping designers iterate through concepts, generative AI tools are becoming indispensable creative partners.
Education has also embraced AI’s potential, with platforms like Carnegie Learning offering personalized tutoring experiences. These systems adapt to individual learning styles and progress, providing targeted support when students struggle while maintaining appropriate challenges for advancement.
As artificial intelligence continues to mature, its applications grow increasingly sophisticated and nuanced. The key to successful AI implementation lies not in replacing human expertise, but in augmenting and enhancing our capabilities across every domain.
Challenges and Risks
AI systems face distinct challenges that can impact their reliability and effectiveness. Traditional AI models heavily depend on data quality for accurate results. When trained on incomplete, outdated, or biased datasets, these systems may perpetuate existing prejudices or make flawed decisions that affect critical applications like healthcare diagnostics or financial assessments.
Generative AI presents an even more complex set of challenges. According to research on AI systems, these models can produce content that exhibits systematic gender and racial biases, potentially reinforcing harmful stereotypes. The sophisticated nature of generative AI makes its outputs particularly concerning; they can create misleading content that appears highly credible, complete with fabricated citations and authoritative-sounding language.
Content authenticity poses another significant risk. Generative AI models excel at mimicking human-like writing patterns and can enhance details in ways that make false information seem more plausible. They often communicate uncertainties and limitations strategically, which paradoxically increases their perceived credibility rather than diminishing it.
The scalability of these technologies amplifies these concerns. Traditional AI’s limitations might affect individual decisions, but generative AI can rapidly produce volumes of misleading content that floods information channels. This capability threatens to create an environment where distinguishing truth from AI-generated falsehoods becomes increasingly challenging.
Organizations must implement robust oversight mechanisms to address these challenges. This includes regular audits of training data for traditional AI systems and developing sophisticated detection tools for identifying AI-generated content. Human expertise remains crucial; no AI system should operate without meaningful human supervision and ethical guidelines that prioritize transparency and accountability.
The ability of generative AI to create seemingly credible but potentially misleading content represents one of the most significant challenges in AI development today.
Dr. Amit Joshi, AI Ethics Researcher
Leveraging SmythOS for AI Development
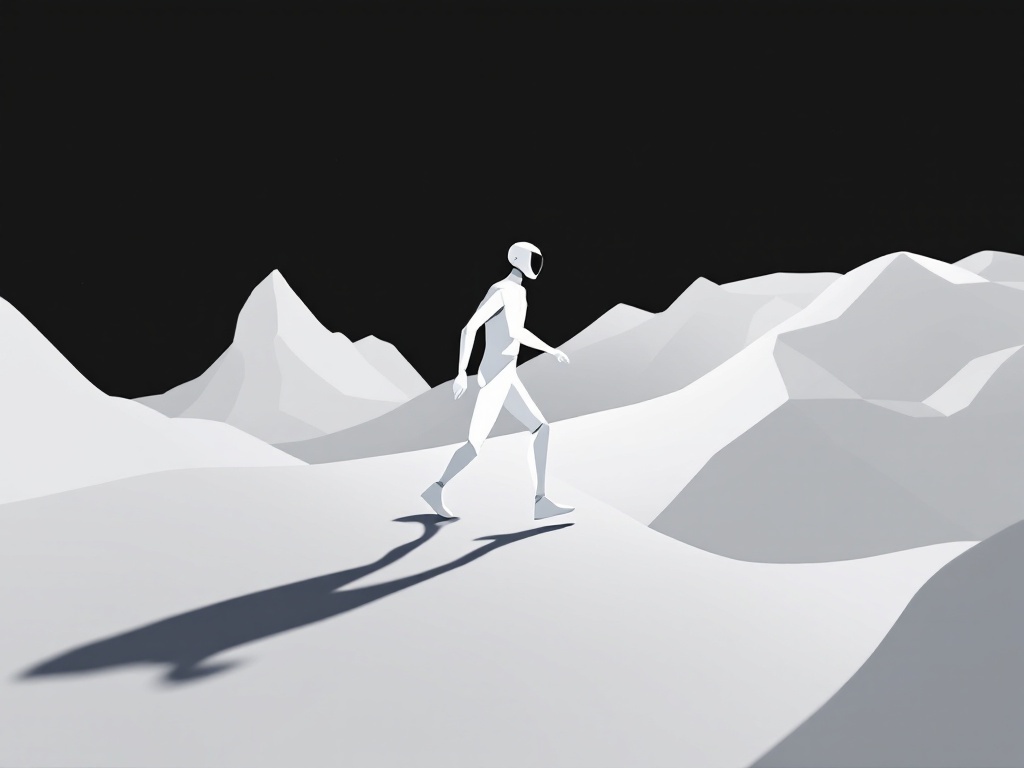
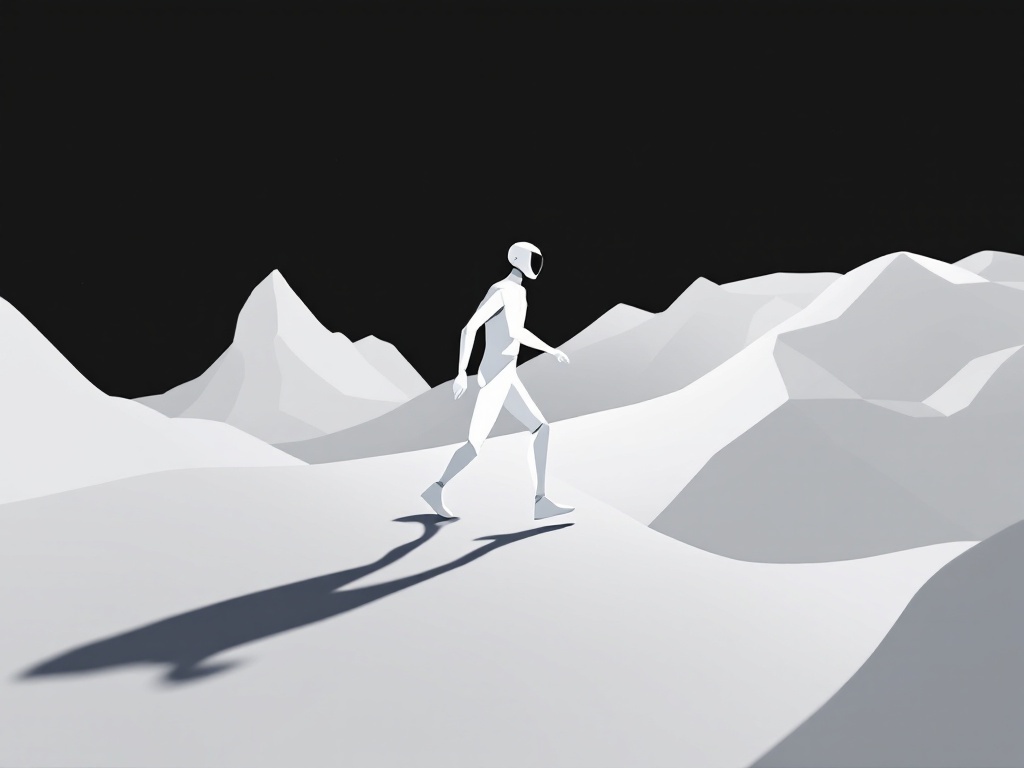
AI development often resembles navigating a maze of tools and frameworks, but SmythOS transforms this landscape with an intuitive approach that streamlines how teams build and deploy AI solutions. Through its visual builder interface, developers can create sophisticated intelligent agents without getting lost in code—a refreshing departure from traditional development methods that often bog teams down in technical complexities.
At the heart of SmythOS’s capabilities lies its robust integration with major graph databases, enabling teams to handle intricate data relationships with unprecedented ease. This seamless connection to graph databases allows for complex relationship mapping and knowledge representation, essential features for building intelligent systems that can understand context and relationships within data. The platform’s visual workflow builder allows developers to map these relationships intuitively, significantly reducing the time typically spent on manual coding and configuration.
Perhaps most impressively, SmythOS tackles one of AI development’s most persistent challenges: debugging. Traditional AI debugging often feels like searching for a needle in a haystack, but SmythOS’s robust debugging tools provide real-time insights into model behavior and performance. This accessibility enables teams across an organization to leverage AI capabilities without requiring specialized expertise.
For teams handling complex data relationships, SmythOS offers unparalleled advantages in orchestration capabilities. The platform seamlessly combines various AI models, tools, workflows, and data sources into a cohesive system that scales effortlessly. This integration is particularly valuable for organizations dealing with interconnected data systems where traditional development approaches often fall short.
Security remains paramount in enterprise AI development, and SmythOS delivers with comprehensive enterprise-grade features. Teams can focus on innovation rather than worrying about data protection, thanks to built-in security measures including data encryption and OAuth integration. This comprehensive approach to security doesn’t come at the cost of flexibility—SmythOS maintains its user-friendly nature while ensuring robust protection of sensitive information.
SmythOS transforms complex AI development into an intuitive process through its visual workflow builder, making sophisticated AI solutions accessible to teams regardless of their technical expertise.
Thomas Sobolik, Machine Learning Engineer
Conclusion and Future Prospects
The artificial intelligence landscape stands at a fascinating crossroads where traditional AI’s analytical precision meets generative AI’s creative potential. Drawing from decades of development, traditional AI continues to excel in structured tasks, offering reliability and transparency that businesses depend on for critical operations like fraud detection and predictive analytics. Meanwhile, generative AI pushes boundaries with its ability to create, innovate, and adapt to complex scenarios.
What’s particularly exciting is how these two branches of AI are beginning to converge. Forward-thinking enterprises are discovering that the real power lies not in choosing between them, but in leveraging their complementary strengths. Traditional AI’s rule-based efficiency perfectly complements generative AI’s creative flexibility, opening new possibilities for innovation across industries.
The future trajectory appears increasingly clear – successful AI implementation will require a sophisticated blend of both technologies. Organizations that master this integration will be best positioned to tackle complex challenges, from personalized customer experiences to breakthrough product development. This synergy promises to unlock unprecedented levels of automation, creativity, and problem-solving capabilities.
As we look ahead, the emphasis will likely shift toward developing more sophisticated hybrid systems that combine traditional AI’s reliability with generative AI’s adaptability. These integrated solutions will need to address current challenges around ethics, transparency, and resource optimization while maintaining high standards of accuracy and efficiency.
The key to success in this evolving landscape lies in understanding each technology’s strengths and implementing them strategically. While traditional AI will continue to form the backbone of mission-critical operations, generative AI will increasingly augment these systems with creative problem-solving capabilities, leading to more comprehensive and powerful AI solutions that can tackle increasingly complex real-world challenges.
Last updated:
Disclaimer: The information presented in this article is for general informational purposes only and is provided as is. While we strive to keep the content up-to-date and accurate, we make no representations or warranties of any kind, express or implied, about the completeness, accuracy, reliability, suitability, or availability of the information contained in this article.
Any reliance you place on such information is strictly at your own risk. We reserve the right to make additions, deletions, or modifications to the contents of this article at any time without prior notice.
In no event will we be liable for any loss or damage including without limitation, indirect or consequential loss or damage, or any loss or damage whatsoever arising from loss of data, profits, or any other loss not specified herein arising out of, or in connection with, the use of this article.
Despite our best efforts, this article may contain oversights, errors, or omissions. If you notice any inaccuracies or have concerns about the content, please report them through our content feedback form. Your input helps us maintain the quality and reliability of our information.