Agent-Based Modeling in Urban Planning: Shaping Smarter Cities Through Simulation
Imagine a city where every decision about new housing developments, transit routes, and commercial zones is tested virtually before breaking ground. This vision is now becoming reality through agent-based modeling—a cutting-edge approach transforming how we plan and design our urban environments.
Traditional urban planning relies heavily on static projections and assumptions. In contrast, agent-based modeling brings cities to life in the digital realm by simulating the dynamic behaviors and interactions of thousands of individual actors—from residents choosing where to live, to businesses selecting optimal locations, to commuters navigating daily routes. As noted in recent research, this computational technique allows planners to witness how small-scale individual decisions combine to create large-scale urban patterns and phenomena.
Agent-based modeling is powerful for urban planning because it captures the true complexity of city life. Rather than treating a city as a collection of buildings and infrastructure, it models it as an intricate web of human behaviors and relationships. A resident’s choice of neighborhood isn’t just about house prices—it’s influenced by school quality, commute times, nearby amenities, and the decisions of other residents. These interlinked choices create ripple effects that reshape entire communities.
This article explores how agent-based modeling is transforming urban planning by enabling us to test different development scenarios, predict unintended consequences, and design cities that work better for actual humans.
Core Principles of Agent-Based Modeling
Agent-based modeling represents a powerful approach to understanding complex systems by focusing on their smallest building blocks – individual agents. It involves creating a virtual environment where each person, vehicle, or building is an independent decision-maker with its own set of rules and behaviors.
The foundation of agent-based modeling rests on three key principles. First, agents are autonomous entities that can make decisions independently, similar to how residents in a city choose their routes to work or where to shop. Each agent follows specific behavioral rules that guide their actions and responses to different situations.
Second, interactions between agents form the heart of these models. Just as people in cities constantly interact with each other and their environment – whether at crosswalks, in stores, or on public transit – agents in the model engage with one another based on predefined rules. These interactions can be direct, like two pedestrians avoiding collision, or indirect, such as how one person’s housing choice affects property values for others.
Third, and perhaps most fascinating, is the concept of emergent patterns. According to recent research, when multiple agents interact over time, they create large-scale patterns that weren’t explicitly programmed into the model. For example, simple rules about individual walking preferences can lead to the formation of spontaneous pedestrian lanes in crowded spaces.
In urban planning applications, these principles help professionals understand how individual decisions collectively shape city dynamics. By simulating thousands of households making independent housing choices, planners can observe how neighborhoods evolve, traffic patterns emerge, and public spaces are utilized. This bottom-up approach provides valuable insights that traditional top-down planning methods might miss.
The power of agent-based modeling lies in its ability to reveal unexpected consequences of planning decisions. When planners simulate how individuals might respond to a new transit line or zoning change, they can identify potential challenges or opportunities before implementing real-world changes. This makes it an invaluable tool for creating more resilient and livable urban environments.
Application Area | Description | Example |
---|---|---|
Traffic Flow Simulation | Allows planners to test various scenarios before implementing costly infrastructure changes. | Barcelona evaluating the impact of reducing lanes on Gran Via de les Corts Catalanes. |
Land Use Optimization | Simulates how different zoning policies might affect neighborhood development, from housing density to commercial space distribution. | Urban planners can account for resident preferences and economic factors. |
Social Behavior Simulation | Simulates individual decision-making processes influenced by neighbors, infrastructure, and personal preferences. | Assessing policy impacts like congestion pricing or new transit routes. |
Development Scenario Testing | Virtual urban laboratories for testing different combinations of housing, commercial spaces, and transportation networks to find optimal configurations. | Experimenting with scenarios to serve community needs better. |
Applications of Agent-Based Models in Urban Planning
Agent-based models have transformed how cities approach complex planning challenges. Traffic flow simulation, a prominent application, allows planners to test various scenarios before implementing costly infrastructure changes. For instance, when Barcelona needed to evaluate the impact of reducing lanes on Gran Via de les Corts Catalanes, researchers used agent-based models to analyze vehicle speeds, lane occupancy, and overall traffic patterns.
Beyond traffic management, these sophisticated tools excel at modeling land use optimization. Urban planners can simulate how different zoning policies might affect neighborhood development, from housing density to commercial space distribution. The models account for countless variables – from resident preferences to economic factors – providing insights that traditional planning methods often miss.
What makes agent-based models particularly powerful is their ability to capture social behaviors and interactions. Rather than treating populations as uniform blocks, these models simulate individual decision-making processes. A resident’s choice to walk, drive, or take public transit isn’t made in isolation – it’s influenced by neighbors, available infrastructure, and personal preferences.
The predictive capabilities of these models prove invaluable when assessing policy impacts. Before implementing congestion pricing or new transit routes, cities can simulate how residents might adapt their daily routines. This anticipatory approach helps identify potential issues and optimize solutions before real-world implementation.
Perhaps most importantly, agent-based models serve as virtual urban laboratories for testing development scenarios. Planners can experiment with different combinations of housing, commercial spaces, and transportation networks to find configurations that best serve community needs. This evidence-based approach reduces the risk of costly planning mistakes and helps build more resilient, livable cities.
Challenges in Implementing Agent-Based Models
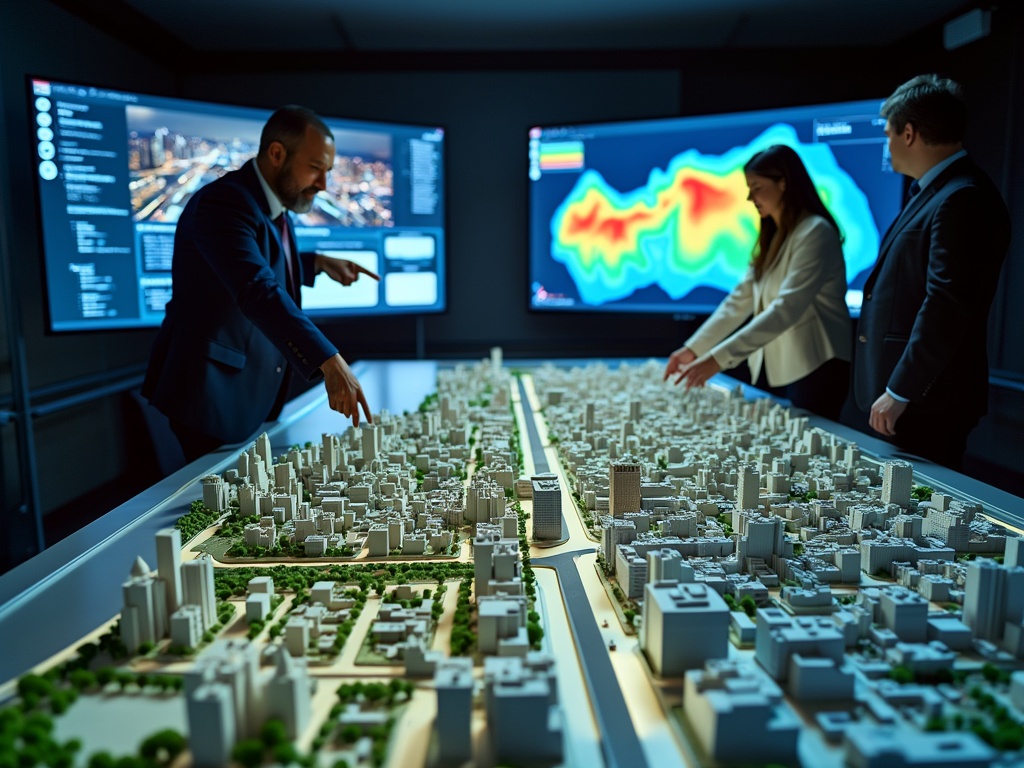
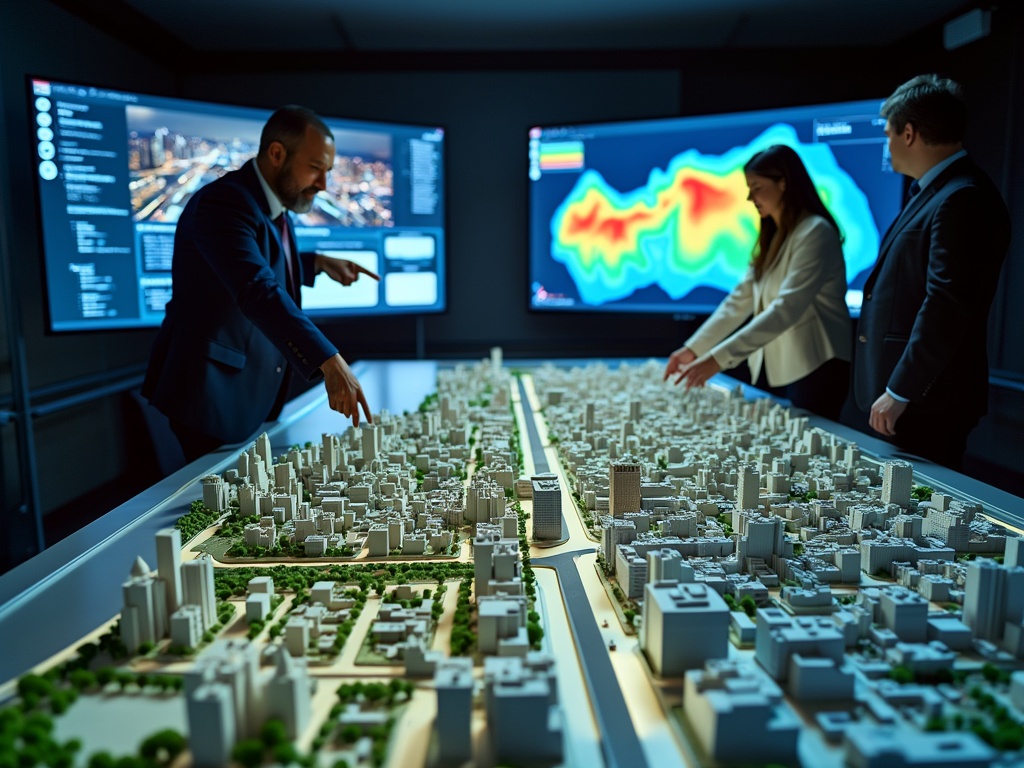
Agent-based modeling (ABM) is a powerful approach for simulating urban systems, yet its implementation comes with several significant hurdles. These challenges stem from the inherent complexity of modeling human behavior and urban environments at scale.
Data accuracy stands as a primary obstacle in ABM implementation. Models require extensive, high-quality datasets to accurately represent agent behaviors and environmental conditions. Recent research highlights that ABMs tend to be “data-hungry” and difficult to validate, particularly when modeling complex human decision-making processes in urban environments. The challenge intensifies with incomplete or inconsistent data sources, which can significantly impact the model’s reliability and predictive accuracy.
The computational demands of agent-based models present another formidable challenge. These models attempt to simulate thousands or even millions of individual agents interacting simultaneously, causing processing requirements to quickly become overwhelming. Traditional computing resources often struggle to handle the intensive calculations required for large-scale urban simulations, particularly when modeling complex behavioral rules and environmental interactions.
Simulation Type | Computational Requirement | Challenges |
---|---|---|
City-scale building energy simulation | High memory and processing power | Requires comprehensive computation process for a whole year |
Digital twins in city planning | Linear increase in computer time and memory | Complex geospatial applications |
Urban flood simulation | High performance computing facilities | Running thousands of model simulations |
Interdisciplinary collaboration emerges as both a necessity and a challenge in ABM implementation. Urban planning projects require expertise from multiple domains—from social scientists understanding human behavior to computer scientists managing technical implementation, and urban planners providing domain expertise. Coordinating these diverse perspectives and integrating their insights into a cohesive model demands careful management and clear communication channels.
The complexity of agent behavior modeling further compounds these challenges. Capturing realistic human decision-making processes requires sophisticated algorithms that can account for both rational and irrational choices. Traditional economic theories of benefit maximization often prove insufficient, necessitating more nuanced approaches that incorporate psychological and sociological factors.
Most agent-based models are not simply the computational implementation of a model or set of models previously developed in equation form. Rather, agent-based modeling often proceeds from agent taxonomy and flow diagrams, to pseudo code, and finally to software programs that can be executed.
Tesfatsion (2017)
To address these challenges, practitioners must adopt strategic approaches. This includes leveraging advanced computing technologies, developing robust data validation methods, and establishing clear frameworks for interdisciplinary collaboration.
Success in implementing agent-based models ultimately depends on carefully balancing these various elements while maintaining focus on the model’s intended purpose and practical utility.
Future Trends in Agent-Based Modeling for Urban Planning
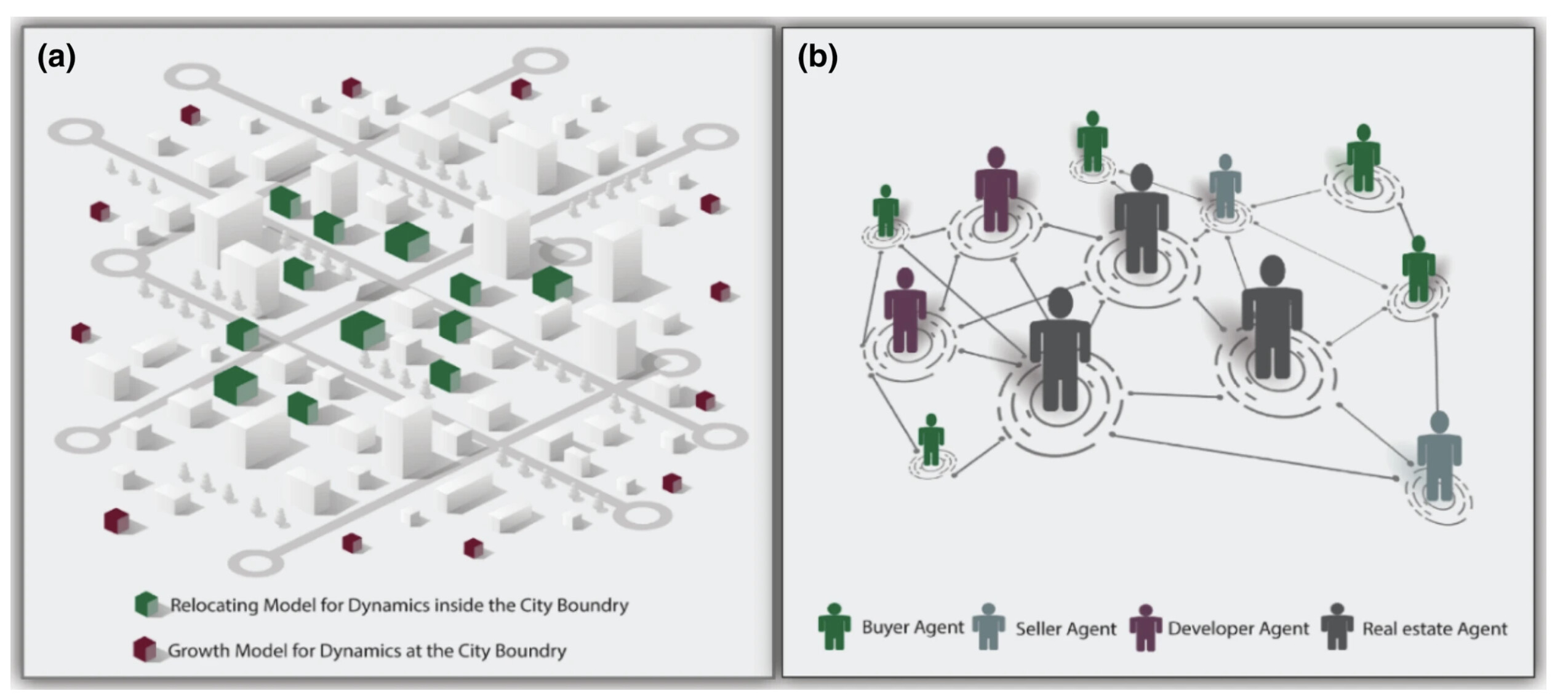
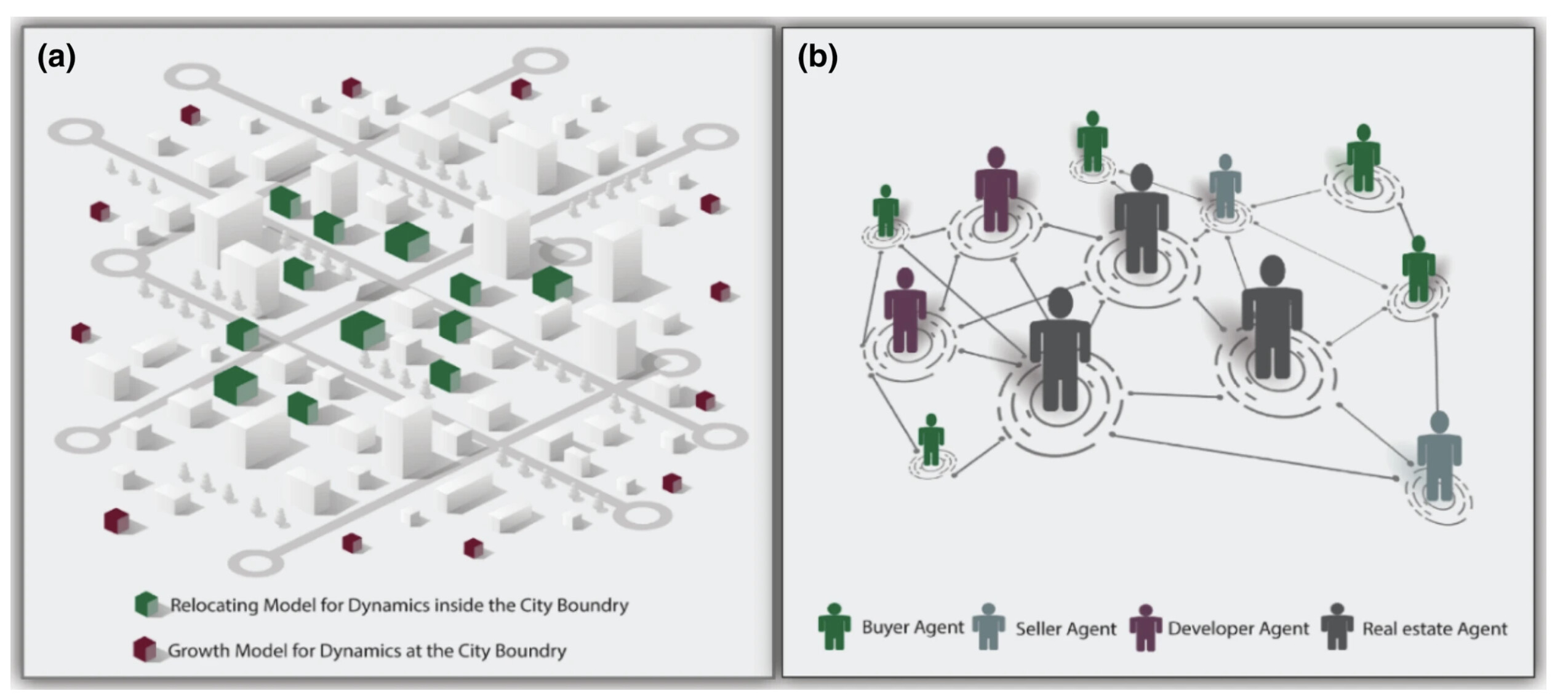
Exploring urban planning through dynamic models. – Via blogspot.com
Recent advances in artificial intelligence and data analytics are enhancing agent-based modeling (ABM) for urban planning applications. As recent research indicates, integrating machine learning algorithms with ABM has led to more sophisticated agent behaviors and better model calibration, marking a new era of intelligent system simulations.
Smart ABM systems can process vast amounts of real-time urban data, from traffic patterns to pedestrian movements. These models leverage advanced AI capabilities to simulate complex urban dynamics with unprecedented accuracy, helping planners make more informed decisions about infrastructure development and resource allocation.
Integrating digital twin technology with ABM represents another significant advancement. By creating virtual replicas of urban environments, planners can test various scenarios and interventions before implementing them in the real world. This capability is valuable for analyzing the potential impacts of major urban development projects on traffic flow, population density, and resource utilization.
Enhanced visualization techniques are transforming how urban planners interact with ABM outputs. Three-dimensional renderings and immersive virtual reality interfaces allow stakeholders to experience simulated urban environments firsthand, making it easier to identify potential issues and opportunities in proposed development plans.
Real-time data integration capabilities are perhaps the most transformative trend in urban planning ABM. Modern systems can continuously update their simulations with live data from IoT sensors, surveillance systems, and social media feeds, providing planners with dynamic, responsive models that reflect current urban conditions. This advancement enables more agile and adaptive urban planning strategies that can respond quickly to changing circumstances.
Leveraging SmythOS for Agent-Based Modeling
Urban planners tackling complex city development challenges need powerful tools to simulate and test their ideas before implementation. SmythOS emerges as a game-changing platform for building sophisticated agent-based models (ABMs) that can accurately represent urban dynamics and interactions.
At the core of SmythOS’s urban planning capabilities lies its intuitive visual workflow builder. This drag-and-drop interface transforms the traditionally code-heavy process of ABM development into an accessible experience, allowing urban planners to focus on model design rather than technical implementation. Planners can quickly prototype and iterate on their models, testing different scenarios for neighborhood development, traffic flow optimization, or public transportation networks.
The platform’s robust integration capabilities set it apart in the urban modeling landscape. SmythOS seamlessly connects with various data sources and APIs essential for urban planning, from demographic databases to real-time traffic data. This integration power enables models to incorporate diverse datasets, creating more accurate and comprehensive urban simulations.
SmythOS’s built-in monitoring system provides unprecedented visibility into model behavior. Urban planners can track key performance indicators in real-time, observing how different urban elements interact and influence each other. This immediate feedback loop allows for quick identification of potential issues in proposed urban developments before they manifest in the real world.
The visual debugging environment represents another crucial advantage for urban planners. When models produce unexpected results, SmythOS’s debugging tools allow planners to pause simulations, inspect individual agents (whether they represent people, vehicles, or buildings), and modify parameters on the fly. This granular control helps ensure model reliability and accuracy in predicting urban development outcomes.
SmythOS transforms the complexity of urban modeling into an intuitive process, enabling planners to create sophisticated simulations without getting bogged down in technical details.
The platform’s auto-scaling capabilities ensure that even large-scale urban models with thousands of agents run smoothly.
Feature | Benefit |
---|---|
Integration Capabilities | Seamlessly connects with various data sources and APIs essential for urban planning. |
Visual Workflow Builder | Transforms the traditionally code-heavy process of ABM development into an accessible experience. |
Real-Time Monitoring | Provides unprecedented visibility into model behavior, allowing for quick identification of potential issues. |
Visual Debugging Environment | Enables planners to inspect individual agents and modify parameters on the fly. |
Auto-Scaling Capabilities | Ensures that even large-scale urban models with thousands of agents run smoothly. |
Conclusion: The Road Ahead for Agent-Based Modeling
Agent-based modeling has emerged as a transformative force in urban planning, changing how we understand and shape our cities. By simulating individual behaviors and interactions, this approach reveals complex patterns that traditional methods often miss. Modeling intricate ecosystem dynamics, from traffic flows to social interactions, provides urban planners with unprecedented insights for decision-making.
As computational power grows, the potential for more sophisticated agent-based models expands. These advancements enable researchers and planners to tackle complex urban challenges, from optimizing public transportation networks to predicting the impact of climate change on city infrastructure. The future of urban planning lies in harnessing these capabilities to create more resilient, sustainable, and livable cities.
However, realizing the full potential of agent-based modeling requires overcoming several key challenges. High-quality data, robust validation methods, and efficient computational resources remain essential. Recent research has highlighted the importance of developing flexible frameworks that combine bottom-up approaches with participatory planning methods.
Looking ahead, integrating agent-based modeling with emerging technologies promises even more powerful urban planning solutions. Visual debugging environments and automated optimization tools are making these sophisticated models more accessible to planners and developers. SmythOS’s intuitive platform exemplifies this evolution, providing the necessary infrastructure to build and deploy complex agent-based models while maintaining the flexibility needed for urban planning applications.
As cities become increasingly complex, the role of agent-based modeling in urban planning will only grow in importance. By embracing these advanced modeling techniques and the tools that support them, urban planners can create more effective, data-driven solutions that shape the cities of tomorrow. The future of urban planning is about understanding and nurturing the intricate web of interactions that make cities truly live and breathe.
Last updated:
Disclaimer: The information presented in this article is for general informational purposes only and is provided as is. While we strive to keep the content up-to-date and accurate, we make no representations or warranties of any kind, express or implied, about the completeness, accuracy, reliability, suitability, or availability of the information contained in this article.
Any reliance you place on such information is strictly at your own risk. We reserve the right to make additions, deletions, or modifications to the contents of this article at any time without prior notice.
In no event will we be liable for any loss or damage including without limitation, indirect or consequential loss or damage, or any loss or damage whatsoever arising from loss of data, profits, or any other loss not specified herein arising out of, or in connection with, the use of this article.
Despite our best efforts, this article may contain oversights, errors, or omissions. If you notice any inaccuracies or have concerns about the content, please report them through our content feedback form. Your input helps us maintain the quality and reliability of our information.