Agent-Based Modeling in Psychology
What if you could create an entire artificial world to test your psychological theories? That’s exactly what agent-based modeling (ABM) allows researchers to do, transforming our understanding of human behavior and social dynamics. This computational method has become a vital tool for psychologists aiming to connect individual actions with collective outcomes.
Unlike traditional research methods that struggle to capture complex social interactions, ABM offers unparalleled control and scale. By programming virtual agents to follow simple behavioral rules, researchers can observe how group phenomena emerge, from the formation of social cliques to the spread of cultural beliefs. As noted by Columbia University researchers, these simulations help us understand the intricate interplay between people, places, and time.
Have you ever wondered why minor individual preferences can lead to significant societal patterns? ABM provides insights into these dynamics, allowing psychologists to simulate thousands of interactions and track their effects across virtual populations. The implications for social psychology are profound, offering new ways to study conformity, prejudice, cooperation, and competition.
This exploration of agent-based modeling in psychology will uncover how this methodology works, examine its transformative applications in social psychology research, and reveal the advantages it holds over conventional methods. Whether you’re a researcher seeking new tools or simply curious about how individual behaviors scale to create social phenomena, you’ll discover how ABM is reshaping our understanding of human psychology.
By the end of this article, you’ll understand how this approach bridges the gap between individual behaviors and social outcomes—a challenge that has long puzzled psychological researchers. Explore the future of psychological science through the lens of agent-based modeling.
Understanding Agent-Based Modeling
Agent-based modeling (ABM) simulates complex systems by focusing on individual actors, or agents, within a system. It’s like a virtual laboratory where researchers can observe how individual decisions and interactions lead to larger patterns and outcomes.
ABM creates virtual agents representing real-world entities, such as people, organizations, animals, or particles. These agents operate within a controlled digital environment based on predefined rules governing their behavior and interactions. Each agent can make autonomous decisions, adapt to changing conditions, and interact with their environment and other agents, mirroring real-world behaviors.
The power of agent-based modeling lies in its ability to reveal emergent phenomena—patterns and behaviors that arise naturally from interactions between agents but wouldn’t be obvious by looking at individual components. As noted in research published in the Oxford Bibliographies, these models excel at capturing complex patterns that emerge from local interactions between autonomous entities.
The fundamental components of an agent-based model include:
- Agents with unique characteristics and decision-making capabilities
- An environment where agents operate and interact
- Rules governing agent behavior and interactions
- Methods for agents to perceive and respond to their surroundings
Component | Description |
---|---|
Agents | Entities with unique characteristics and decision-making capabilities |
Environment | The digital space where agents operate and interact |
Rules | Guidelines that govern agent behavior and interactions |
Perception and Response | Methods for agents to perceive and respond to their surroundings |
ABM’s flexibility in modeling both simple and highly complex systems makes it valuable. Traditional modeling approaches often rely on aggregate behaviors and averages, but agent-based models capture the diversity of individual behaviors and their cumulative effects on the system as a whole.
Consider a model simulating crowd behavior during an emergency evacuation. Each agent (person) might have unique characteristics like walking speed, risk tolerance, and familiarity with exits. Their individual decisions—whether to follow others, which exit to choose, when to start moving—collectively create realistic crowd dynamics that would be difficult to predict using conventional methods.
Agent-based modeling allows us to understand how simple rules at the individual level can generate complex patterns at the system level, providing insights that would be impossible to obtain through other means.
Charles M. Macal, Director of the Center for Complex Adaptive Agent Systems Simulation
By breaking down complex phenomena into understandable components and rules, agent-based modeling provides researchers and practitioners with powerful tools for exploring scenarios, testing hypotheses, and making more informed decisions across various fields—from epidemiology to economics, urban planning to environmental science.
Applications of ABM in Social Psychology
Agent-based modeling has emerged as a powerful tool for studying the intricate dynamics of human social behavior. Researchers harness ABM’s capabilities to simulate how individual actions and choices aggregate into larger social patterns and phenomena, much like how a few raindrops can eventually form a mighty river. One fascinating application is in studying group formation and social identity. Rather than requiring explicit social identities to exist first, recent research demonstrates that stable groups can emerge simply through basic human tendencies like reciprocity (cooperating with those who have helped us) and transitivity (sharing our network’s social preferences). This reveals how complex social structures can arise from surprisingly simple individual behaviors.
In the realm of cooperation and competition, ABM has provided groundbreaking insights into how collaborative behaviors emerge and sustain themselves in populations. By simulating repeated social interactions under different conditions, researchers have found that simple strategies like “tit-for-tat”—starting with cooperation and then mirroring the other’s previous choice—can foster stable cooperation even in challenging environments.
The modeling of social influence and opinion dynamics represents another powerful application. ABM simulations demonstrate how individual beliefs and attitudes spread through social networks, revealing the conditions under which minority views can overtake majorities or how polarization can emerge from initially moderate positions. This helps explain real-world phenomena like the viral spread of social movements or the formation of echo chambers.
Perhaps most remarkably, ABM allows researchers to explore counterfactual scenarios that would be impossible or unethical to test in real life. For instance, simulations can reveal how different intervention strategies might affect the spread of harmful behaviors through social networks, or how changes in organizational structures could impact group performance and innovation. Beyond theoretical insights, these applications have practical implications for addressing real-world challenges. From designing more effective public health campaigns to developing strategies for reducing social conflict, ABM provides a virtual laboratory for testing and refining social interventions before implementing them at scale.
Advantages of ABM Over Traditional Methods
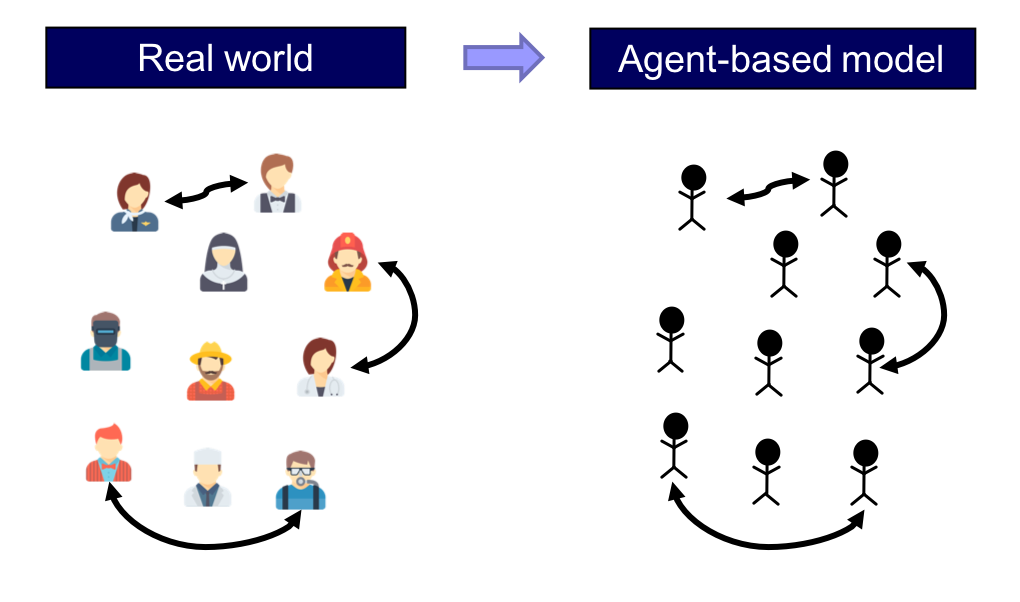
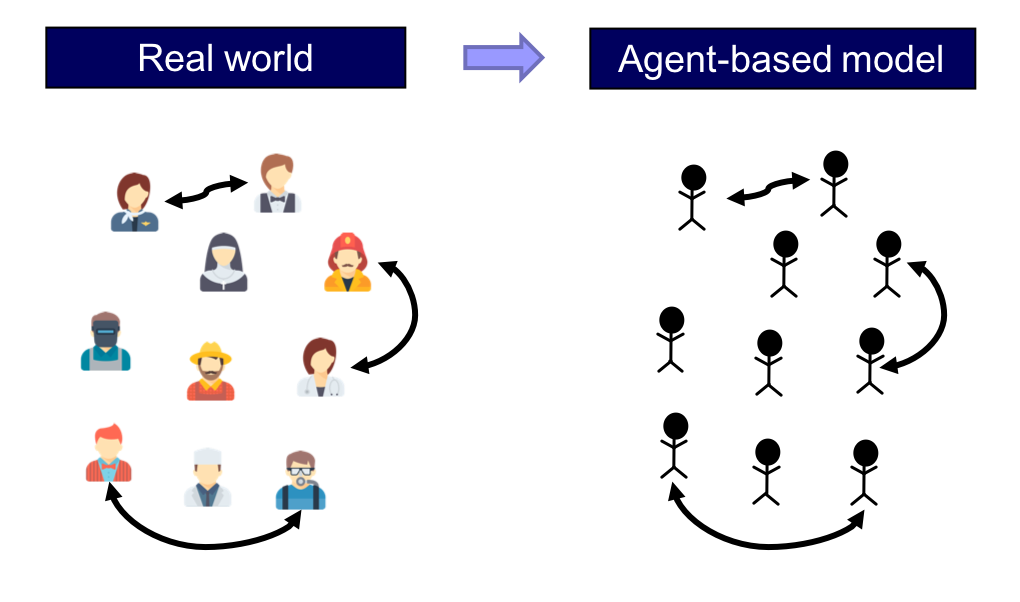
Agent-based modeling (ABM) represents a powerful advancement over traditional research methodologies, offering unprecedented control in studying complex social and behavioral systems. Unlike conventional statistical approaches that often treat populations as homogeneous groups, ABM allows researchers to adjust and examine individual-level variables with remarkable precision while observing how these changes ripple through the entire system.
One of ABM’s most significant advantages is its ability to simulate massive populations while maintaining granular control over individual agents. Traditional methods often struggle to account for the actions and interactions of thousands or millions of discrete entities. In contrast, ABM can model populations of virtually any size, with each agent possessing unique characteristics and decision-making capabilities.
As noted in a recent study, this scalability makes ABM particularly valuable for studying phenomena like disease transmission or social behavior patterns across large populations.
Criteria | ABM | Traditional Methods |
---|---|---|
Targeting Approach | Focuses on individual agents and their interactions | Uses aggregate behaviors and averages |
Data Integration | Incorporates both quantitative and qualitative data | Primarily uses quantitative data |
Emergent Phenomena | Captures complex, non-linear patterns | Focuses on linear relationships |
Scalability | Can model large populations with unique agent characteristics | Struggles with large, heterogeneous populations |
Flexibility | Highly adaptable to different scenarios | Limited adaptability |
Experimental Potential | Allows for extensive virtual experimentation | Limited to observational data |
The observation of emergent behaviors sets ABM apart from conventional research methods. While traditional approaches typically focus on linear relationships between variables, ABM captures the complex, non-linear patterns that emerge from countless individual interactions. These emergent properties – collective behaviors that cannot be predicted by studying individual components in isolation – provide crucial insights into phenomena like market dynamics, social movements, or disease outbreaks.
Another compelling advantage is ABM’s capacity to incorporate both quantitative and qualitative data into its models. Traditional methods often struggle to integrate diverse data types, but ABM can seamlessly combine numerical data with behavioral rules, social norms, and environmental factors. This flexibility allows researchers to create more comprehensive and realistic models of complex systems.
The experimental potential of ABM also surpasses traditional methods. Researchers can run countless simulations with different parameters, testing hypotheses and interventions in a virtual environment before implementing them in the real world. This capability is especially valuable when studying situations where real-world experimentation would be impractical, unethical, or impossible.
Furthermore, ABM excels at handling spatial and temporal dynamics that traditional methods find challenging to model. The ability to simulate how agents move through and interact with their environment over time provides insights into everything from urban development patterns to the spread of innovations through social networks.
Perhaps most importantly, ABM enables researchers to study systemic feedback loops and adaptive behaviors – critical features of complex systems that traditional methods often overlook or oversimplify. By modeling how agents learn from and react to changes in their environment, ABM captures the dynamic, evolving nature of real-world systems more accurately than static analytical approaches.
Challenges in Implementing ABM
Agent-based modeling offers powerful capabilities for simulating complex systems, but several significant challenges can impede its successful implementation. The computational demands of ABM present one of the most pressing concerns, particularly when simulating systems with millions of agents. For instance, modeling just one cubic centimeter of tissue requires simulating 108-109 cells, pushing the limits of current computing capabilities.
Computational complexity escalates dramatically as more agents and interactions are added to the model. As recent research shows, the computational cost of testing numerical stability in multi-scale models can become prohibitive. This challenge is particularly acute when attempting to optimize resource allocation or validate model behavior across different scales.
Data availability poses another significant hurdle. ABM requires detailed information about agent behaviors, interaction rules, and environmental conditions to create realistic simulations. However, obtaining comprehensive real-world data to inform these parameters often proves difficult. The challenge intensifies when dealing with complex systems where data collection may be impractical or ethically constrained.
Model validation emerges as a critical challenge that demands careful attention. Unlike simpler modeling approaches, validating ABMs requires confirming the accuracy of both individual agent behaviors and emergent system-level patterns. This dual requirement makes validation particularly complex, as researchers must verify that the model accurately represents both micro-level interactions and macro-level outcomes.
Despite these challenges, several promising solutions are emerging. Cloud computing and parallel processing technologies are helping address computational limitations by distributing simulations across multiple processors. Additionally, hybrid modeling approaches that combine ABM with other simulation techniques can help reduce computational overhead while maintaining model accuracy.
To tackle data availability issues, researchers are increasingly turning to innovative data collection methods and synthetic data generation techniques. Machine learning algorithms can help fill data gaps by inferring agent behaviors from limited available data. These approaches, while not perfect, provide practical workarounds for scenarios where complete data sets are unavailable.
For validation challenges, standardized frameworks and best practices are being developed to ensure model reliability. These include rigorous testing protocols, sensitivity analyses, and documentation standards that make validation more systematic and reproducible. Cross-validation with multiple data sources and expert review processes help strengthen confidence in model results.
Future Directions in ABM
Agent-based modeling is undergoing a profound transformation in psychological research, driven by advances in computational capabilities. Modern processors can simulate millions of interactions simultaneously, allowing researchers to model increasingly complex social and cognitive phenomena with remarkable precision.
A major breakthrough lies in the integration of sophisticated data analytics with ABM frameworks. Researchers can now feed real-world behavioral data directly into their models, creating what some experts call “digital twins” of human psychological processes. For instance, recent applications show how ABM can simulate intricate social dynamics and collective behavior patterns that were previously impossible to model.
The emergence of more nuanced modeling approaches is reshaping our understanding of human cognition and behavior. Modern ABMs can now account for emotional states, cognitive biases, and even cultural influences. These enhanced models offer unprecedented insights into how individual psychological processes scale up to create complex social phenomena.
Another exciting development is the growing synergy between ABM and other cutting-edge technologies. Machine learning algorithms are being incorporated to make agents more adaptive and responsive, while virtual reality platforms provide new ways to validate model predictions against human behavior in controlled environments.
Perhaps most promising is the expansion of ABM into diverse psychological domains. From clinical psychology, where models help predict treatment outcomes, to educational psychology, where they simulate learning environments, ABM is proving invaluable across the field. These applications demonstrate how agent-based approaches can bridge the gap between individual psychological processes and broader social outcomes.
Looking ahead, the integration of neurobiological data with ABM frameworks may soon allow researchers to create models that span multiple levels of analysis, from neural circuits to social networks. This multi-scale modeling approach could revolutionize our understanding of how brain activity relates to complex social behaviors.
The future of psychological research lies not just in observing behavior, but in creating sophisticated digital laboratories where we can explore the full complexity of human psychology.
Adapted from contemporary ABM researchers
Leveraging SmythOS for ABM Development
SmythOS transforms the complex process of agent-based modeling into a streamlined, intuitive experience through its comprehensive development platform. The platform’s visual builder eliminates the need for extensive coding expertise, allowing researchers and developers to construct sophisticated ABMs using a drag-and-drop interface. Built-in monitoring capabilities provide real-time insights into model performance. Developers can track message exchange rates between agents, resource utilization patterns, and task completion metrics, enabling quick identification and resolution of potential bottlenecks. This visibility proves invaluable when scaling up models to handle thousands of interacting agents.
Integration challenges often plague ABM implementations, but SmythOS addresses this through its seamless API connection framework. The platform can interface with virtually any external service or data source, significantly expanding the capabilities of agent-based models. Whether pulling real-time market data for financial simulations or connecting to IoT sensors for environmental modeling, SmythOS handles the technical complexity of these integrations.
SmythOS is not just a tool; it’s a game-changer for agent-based modeling. Its visual approach and reusable components make it possible to build and iterate on complex models in a fraction of the time it would take with traditional methods.
The visual debugging environment sets SmythOS apart from conventional ABM tools. This feature transforms complex agent interactions and system flows into clear, understandable visual representations. Developers can pause simulations at any point, inspect individual agents, and modify parameters on the fly to see immediate effects on model behavior. This visual approach accelerates the development process and opens doors to more creative and efficient solutions in ABM architecture.
For those new to ABM development, SmythOS offers an extensive library of reusable components. These pre-built elements handle common agent behaviors and environmental factors, allowing developers to focus on the unique aspects of their models rather than rebuilding basic functionality. The modular nature of these components also promotes rapid prototyping and iterative refinement of models.
Conclusion: The Growing Importance of ABM
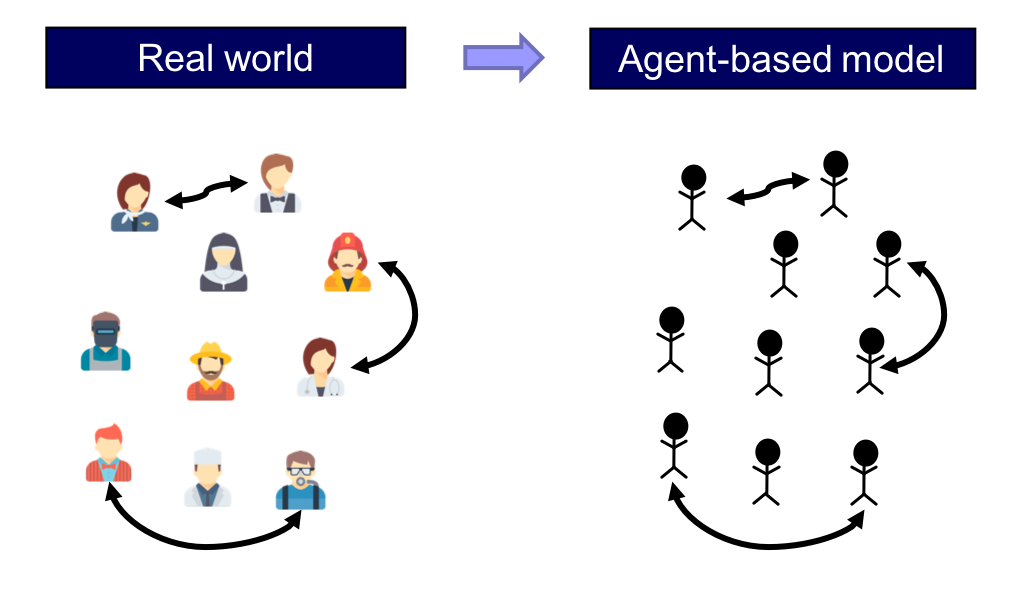
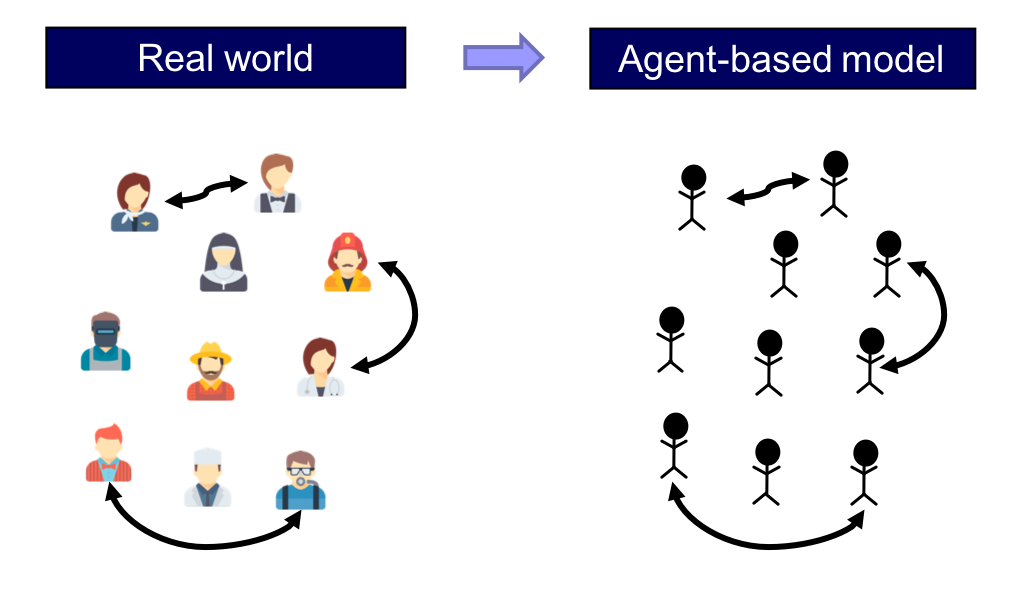
Real-world agents transitioning in an agent-based model. – Via ncsu.edu
Agent-based modeling has emerged as a transformative force in psychological research, offering unprecedented capabilities for studying complex human behaviors and social interactions. Through its ability to simulate intricate scenarios with precise control, ABM provides researchers with a powerful lens to examine phenomena that were previously difficult to study in traditional laboratory settings. As noted in a comprehensive review in Social Psychological and Personality Science, ABM’s unique strength lies in revealing how individual behaviors aggregate into often-surprising collective phenomena.
The evolution of ABM technology marks a significant turning point in psychological research methodology. Where traditional approaches often struggled to capture the dynamic nature of human behavior, ABM excels at modeling the intricate dance of social interactions, cognitive processes, and behavioral adaptations. This technological advancement enables researchers to conduct virtual experiments with unprecedented scale and precision, leading to discoveries that might otherwise remain hidden in the complexity of human psychology.
Looking ahead, ABM’s role in psychological research will likely expand as computing power grows and modeling techniques become more sophisticated. The ability to simulate complex social dynamics, test theoretical frameworks, and generate new hypotheses positions ABM as an invaluable tool for future psychological investigations. These capabilities become increasingly crucial as psychology grapples with understanding human behavior in an increasingly interconnected and complex world.
The accessibility of ABM technology continues to improve, breaking down traditional barriers to entry. Modern platforms streamline the process of creating and deploying agent-based models, democratizing access to this powerful research tool. This accessibility ensures that a broader range of researchers can contribute to our understanding of human psychology, potentially accelerating the pace of discovery in the field.
As we stand at the intersection of psychology and technology, the future of ABM in psychological research appears remarkably promising. Its ability to bridge the gap between theoretical models and empirical observations, combined with increasing technological sophistication, suggests that ABM will continue to uncover new insights into the complexities of human behavior and social interaction for years to come.
Last updated:
Disclaimer: The information presented in this article is for general informational purposes only and is provided as is. While we strive to keep the content up-to-date and accurate, we make no representations or warranties of any kind, express or implied, about the completeness, accuracy, reliability, suitability, or availability of the information contained in this article.
Any reliance you place on such information is strictly at your own risk. We reserve the right to make additions, deletions, or modifications to the contents of this article at any time without prior notice.
In no event will we be liable for any loss or damage including without limitation, indirect or consequential loss or damage, or any loss or damage whatsoever arising from loss of data, profits, or any other loss not specified herein arising out of, or in connection with, the use of this article.
Despite our best efforts, this article may contain oversights, errors, or omissions. If you notice any inaccuracies or have concerns about the content, please report them through our content feedback form. Your input helps us maintain the quality and reliability of our information.