Agent-Based Modeling in Policy Analysis
Ever wonder how policymakers can predict the ripple effects of their decisions across millions of individuals? Enter agent-based modeling, a transformative approach reshaping our understanding of public policy.
Like a sophisticated chess simulation predicting countless moves and outcomes, agent-based modeling allows analysts to create virtual worlds where digital entities—representing everything from citizens to organizations—interact and respond to policy changes. This powerful computational technique has become an essential tool for policymakers seeking to understand the complex web of human behavior and societal dynamics.
According to research published in the Proceedings of the National Academy of Sciences, agent-based modeling excels at revealing how different factors—from retail density to price information to individual characteristics—work together to shape real-world outcomes across diverse contexts and populations.
This approach is particularly fascinating because it captures the emergence of unexpected patterns and behaviors that often elude traditional analytical methods. Rather than relying on oversimplified assumptions about human behavior, agent-based models embrace the messy reality of how individuals and organizations interact, adapt, and evolve in response to policy interventions.
In the following sections, we’ll explore how agent-based modeling has revolutionized policy analysis, examine its core principles, and discover why it’s become an indispensable tool for understanding complex adaptive systems.
Basic Concepts of Agent-Based Modeling
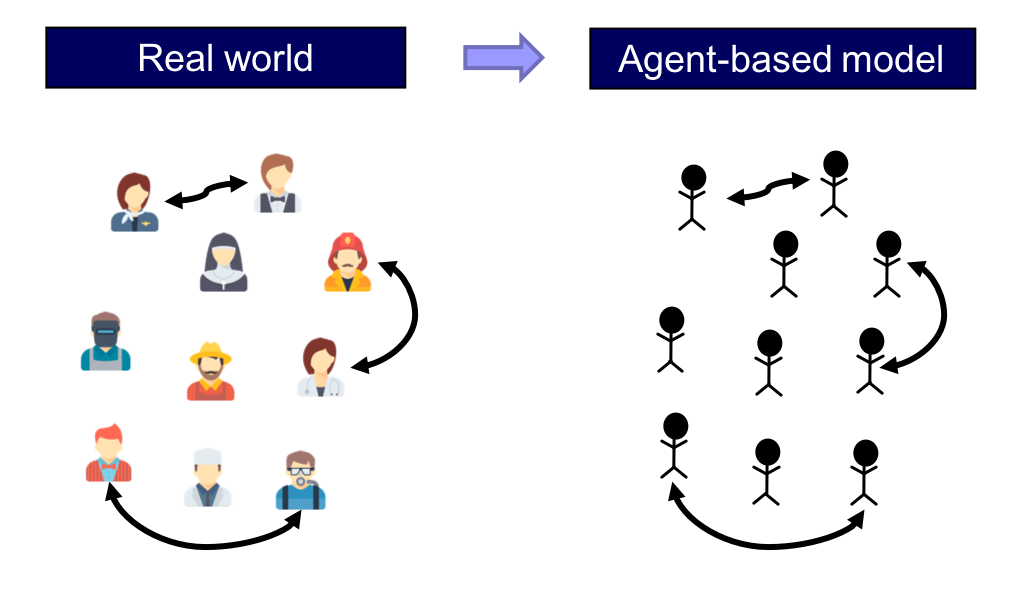
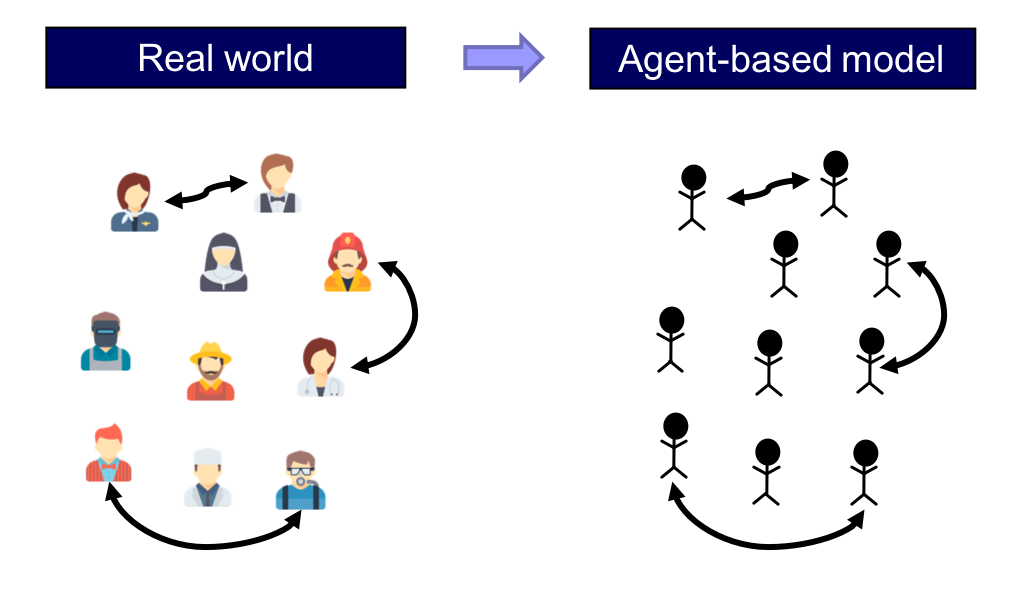
Agent-based modeling represents a powerful approach to understanding complex systems by focusing on how individual components, called agents, interact and influence each other. These models operate like sophisticated social simulations where autonomous agents – whether they represent people, animals, organizations, or even molecules – make decisions and take actions based on their own rules and current circumstances.
Each agent in the system possesses unique characteristics and behaviors, much like how different people have distinct personalities and decision-making patterns. These agents function autonomously within their environment, making independent choices without central control. For example, in a model simulating customer behavior in a shopping mall, individual agents might represent shoppers with different preferences, budgets, and shopping strategies.
One of the most fascinating aspects of agent-based modeling is how agents interact with both their environment and each other. Just as people adjust their behavior based on those around them, agents respond to changes in their surroundings and modify their actions accordingly. These interactions can be as simple as maintaining personal space in a crowd simulation or as complex as negotiating prices in a market model.
The rules governing agent behavior form another crucial component of these models. These rules can range from basic ‘if-then’ statements to sophisticated algorithms that incorporate learning and adaptation. For instance, in a traffic simulation, agents (vehicles) might follow rules about maintaining safe distances, obeying speed limits, and responding to traffic signals while interacting with other vehicles on the road.
What makes agent-based modeling particularly powerful is its ability to reveal emergent behavior – patterns and phenomena that arise naturally from the collective interactions of agents but weren’t explicitly programmed into the model. An excellent example is how simple rules governing individual agents can lead to complex flock formations in bird migration models or the emergence of traffic jams in vehicle simulations.
The practical applications of agent-based modeling span numerous fields. In epidemiology, these models help predict disease spread by simulating how people interact and transmit infections. In economics, they’re used to understand market dynamics by modeling the behavior of buyers, sellers, and other market participants. Urban planners use them to optimize city designs by simulating how residents might utilize different spaces and transportation systems.
While traditional modeling approaches often rely on aggregate equations and averages, agent-based modeling captures the rich diversity and individual variations that exist in real-world systems. This granular approach allows researchers and analysts to explore ‘what-if’ scenarios and test different interventions before implementing them in the real world, making it an invaluable tool for decision-makers across various domains.
Applications of Agent-Based Modeling in Policy Analysis
Agent-based modeling (ABM) has emerged as a powerful analytical tool for policymakers seeking to understand complex social, economic, and environmental systems. By simulating the interactions of autonomous agents within these systems, ABMs provide unique insights into how different policies might affect real-world outcomes.
In economic policy design, ABMs have proven particularly valuable for analyzing financial markets and monetary policy. According to research from Dawid and Neugart, agent-based simulations help evaluate policy measures in complex economic environments where traditional equilibrium models fall short. These models can capture emergent phenomena like market crashes or banking crises that often elude conventional analytical approaches.
Phenomenon | Description |
---|---|
Formation of Industrial Clusters | Local agglomerations of industries, often high-tech, forming due to spatial-economic factors. |
Prices | Market prices emerging from the interactions of buyers and sellers. |
Common Currency | The development of a shared currency system among different regions or countries. |
Industry Standards | Standards that emerge within industries to ensure compatibility and efficiency. |
Cultural Norms | Social behaviors and norms that arise and solidify within communities. |
Language | Shared communication systems that develop within societies. |
Segregated Neighborhoods | Patterns of residential segregation based on socioeconomic factors. |
International Trade Patterns | Complex global trade relationships formed by the interactions of multiple economic agents. |
Environmental policy represents another crucial application area. For instance, researchers have used ABMs to study watershed management policies in agricultural regions. A notable example is the Smoky Hill River Watershed case study, where scientists developed a model integrating hydrological, ecological, and social factors to evaluate conservation policies. The model revealed that cultural factors significantly influence policy support, and opportunities for policy acceptance often emerge immediately following extreme environmental events.
The versatility of ABMs extends to social policy interventions as well. These models help policymakers understand how individual behaviors aggregate to create societal outcomes. For example, models examining population dynamics and resource consumption patterns have helped shape sustainable development policies by illustrating the complex interplay between human decisions and environmental impacts.
One of the most powerful aspects of agent-based modeling is its ability to test policy interventions before implementation in the real world. This allows policymakers to identify potential unintended consequences and refine their approaches. Through careful calibration with real-world data, these models can simulate the effects of different policy scenarios while accounting for the complex interactions between economic, social, and environmental factors.
Agent-based models are digital laboratories in which you can test hypotheses, capture emergent and highly non-linear phenomena, and design interventions. By specifying the behaviour of individual agents and the rules of an environment in which they interact, ABMs allow the study of complex systems with emergent phenomena.
Sharan Agrawal, Cambridge University Technical Report
The effectiveness of ABM in policy analysis stems from its bottom-up approach to modeling complex systems. Rather than relying on simplified assumptions about aggregate behavior, these models capture the heterogeneity of individual agents and their interactions. This granular level of detail provides policymakers with richer insights into how their interventions might play out across different segments of society.
Challenges and Limitations of Agent-Based Models
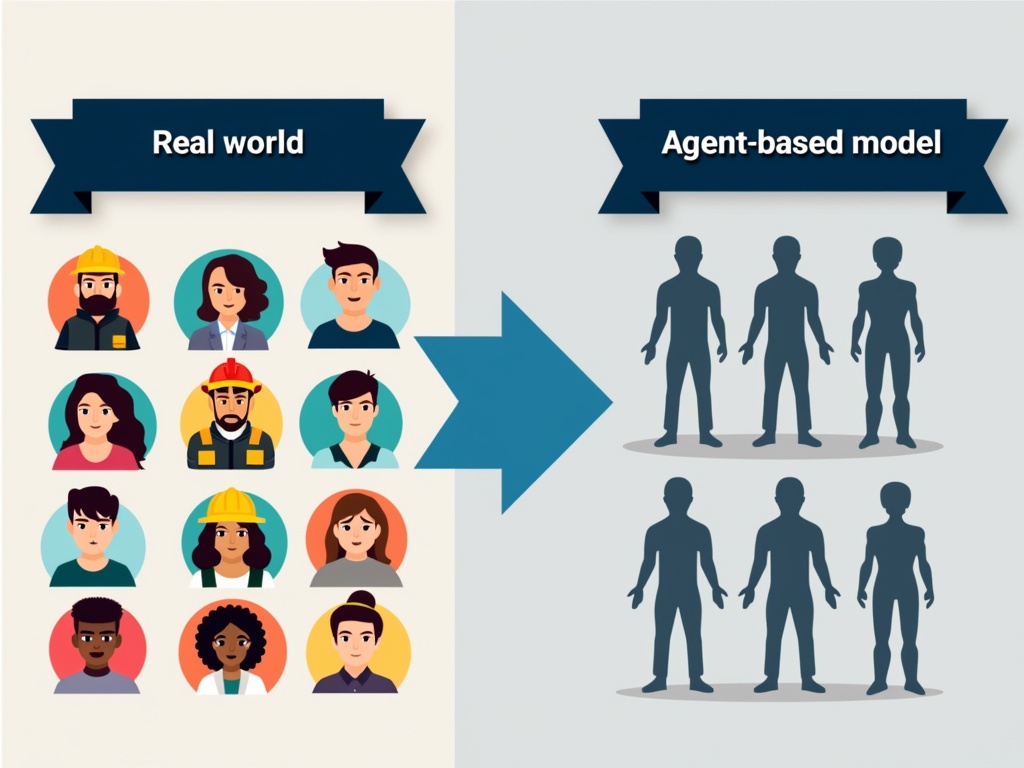
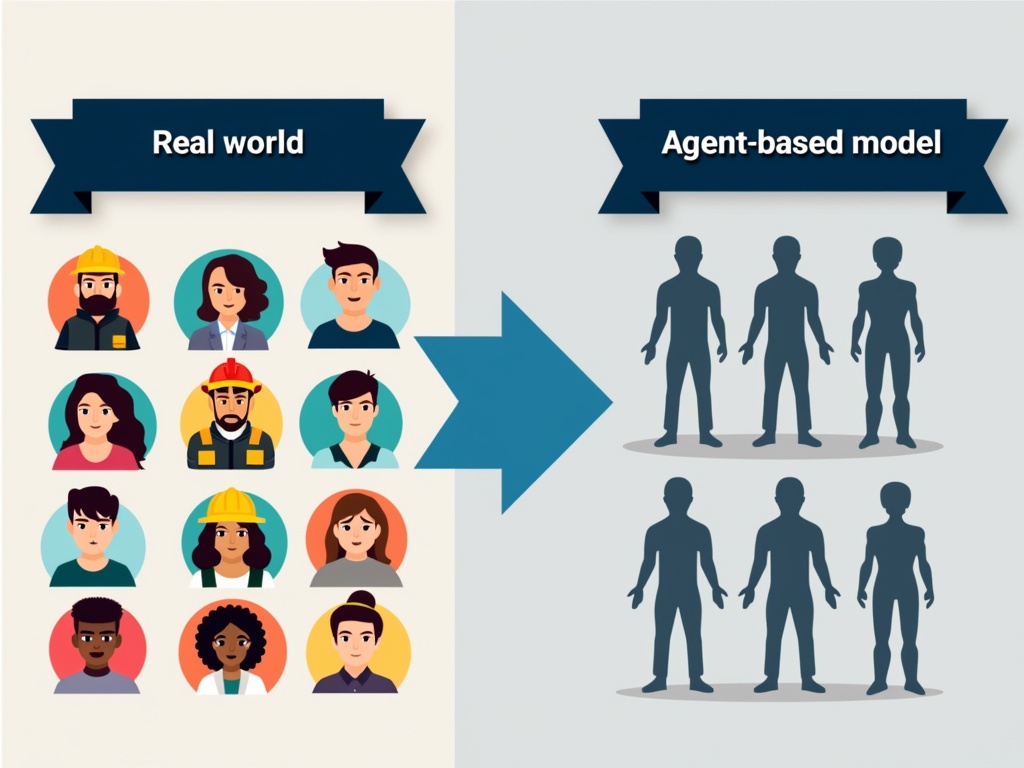
Infographic of real world to agent-based model transition.
Despite their immense potential, agent-based models (ABMs) face significant challenges that researchers and practitioners must navigate carefully. Complex computational demands often strain even modern computing resources, particularly when simulating large-scale systems with numerous interacting agents. For instance, modeling a city’s transportation network with millions of individual commuters can overwhelm standard computing infrastructure, requiring careful optimization of model parameters and selective simplification of agent behaviors.
Data quality emerges as another critical hurdle in ABM implementation. As research has shown, these models are particularly sensitive to input data quality, with even small inaccuracies potentially cascading into significant deviations in model outcomes. The challenge intensifies when dealing with real-world systems where complete, accurate data about individual agent behaviors may be unavailable or prohibitively expensive to collect.
The complexity of ABMs also introduces validation challenges that set them apart from traditional modeling approaches. Unlike simpler models, ABMs generate emergent behaviors and feedback loops that can be difficult to verify against real-world data. This validation becomes especially challenging when modeling human behavior, where individual decision-making processes may not follow predictable patterns and can vary significantly across different contexts and cultures.
Interdisciplinary collaboration, while essential for developing comprehensive models, presents its own set of challenges. Different disciplines often approach problems with varying methodologies and terminologies, making effective communication and integration of expertise difficult. For example, when developing an ABM for urban planning, social scientists, computer scientists, and urban planners must align their perspectives and methodologies to create an effective model.
To address these challenges, researchers are developing several promising strategies. High-performance computing clusters and parallel processing techniques help manage computational complexity, while machine learning algorithms assist in handling data quality issues by filling gaps and identifying patterns in incomplete datasets. Standardized validation frameworks and documentation protocols are emerging to ensure model reliability and reproducibility across different applications.
The implementation of hybrid modeling approaches, combining ABMs with other simulation techniques, offers another pathway forward. These hybrid solutions can help balance the need for detailed agent-level simulation with computational efficiency. For instance, some researchers successfully employ a combination of agent-based and equation-based models, using detailed agent simulation only for critical system components while modeling other aspects with simpler approaches.
Moving forward, the key to advancing ABM capabilities lies in fostering stronger collaboration across disciplines and developing more sophisticated tools for model validation and verification. As computing power continues to increase and new methodologies emerge, many of today’s limitations may become less constraining, opening up new possibilities for complex system modeling and analysis.
Future Directions in Agent-Based Policy Modeling
Agent-based modeling for policy analysis is undergoing significant transformation, driven by breakthroughs in artificial intelligence and computational capabilities. These advancements are reshaping how we understand and predict complex societal dynamics, offering more nuanced and accurate policy development opportunities.
The integration of generative AI with agent-based models represents a promising development. These enhanced models can simulate more realistic and adaptive agent behaviors, leading to sophisticated predictions of policy outcomes. This combination of AI and traditional modeling approaches enables policymakers to explore scenarios with unprecedented depth and accuracy.
Computational advances are dramatically expanding the scope and scale of agent-based simulations. Today’s models can process millions of individual agents simultaneously, each making complex decisions that mirror real-world behaviors. This increase in processing power means policy analysts can now model entire economic systems, from individual households to global markets, with remarkable fidelity.
The evolution of multi-agent reinforcement learning represents another frontier in policy modeling. These systems can now learn optimal strategies through repeated interactions, mimicking how real populations adapt to policy changes over time. This capability is valuable for testing policies in domains where historical data is limited or where novel situations need to be explored.
Modern agent-based models are becoming increasingly interdisciplinary, incorporating insights from behavioral economics, psychology, and social network theory. This integration helps create more realistic simulations of human behavior, moving beyond purely rational economic actors to include emotional, social, and cognitive factors that influence policy outcomes.
The validity of inferences drawn from ABM explorations depends on the quality of the ABM agents’ behavioral models
IEEE Xplore
Looking ahead, we can expect even more sophisticated applications of these tools. The development of explainable AI techniques promises to make complex model outputs more interpretable for policymakers, while advances in data collection and processing will enable real-time policy adjustments based on continuous feedback from agent-based simulations.
Leveraging SmythOS for Agent-Based Policy Modeling
Policy modeling demands sophisticated tools that can handle complex interactions and adaptable scenarios. SmythOS stands out as an innovative platform that transforms how researchers and policymakers approach agent-based modeling, making the intricate process of policy simulation more accessible and efficient.
At its core, SmythOS excels through its comprehensive built-in monitoring capabilities. This feature allows policy analysts to track agent behaviors and system performance in real-time, providing crucial insights into how different policy scenarios might play out in the real world. As reported by VentureBeat, SmythOS empowers users across organizations to leverage AI capabilities without requiring years of specialized expertise.
The platform’s seamless API integration capabilities set it apart in the field of agent-based modeling. By connecting effortlessly with external data sources and services, SmythOS enables policy modelers to incorporate real-world data streams into their simulations. This integration creates more realistic and dynamic models that better reflect the complexities of policy implementation in actual environments.
One of SmythOS’s most powerful features is its automatic scaling functionality. As policy models grow in complexity or require additional computational resources, the platform intelligently adjusts its capacity to maintain optimal performance. This scalability ensures that even the most sophisticated policy simulations run smoothly, without the technical bottlenecks that often plague traditional modeling approaches.
The visual debugging environment of SmythOS deserves special mention for its contribution to policy modeling. This intuitive interface allows researchers to visualize agent interactions and identify potential issues quickly, significantly reducing the time required to validate and refine policy models. The ability to observe and adjust model parameters in real-time makes it easier to test different policy scenarios and their potential outcomes.
Whether analyzing economic policies, social programs, or environmental regulations, SmythOS provides the robust infrastructure needed to create, test, and deploy agent-based models effectively. Its combination of powerful features and user-friendly design makes it an invaluable tool for policy professionals seeking to understand the complex implications of their decisions.
Conclusion and Future Prospects
The transformative potential of agent-based modeling in policy analysis stands at a crucial inflection point. These sophisticated systems have demonstrated remarkable capabilities in unraveling the complexities of social and economic systems, offering policymakers unprecedented insights for decision-making.
By leveraging advanced platforms like SmythOS, organizations can now navigate intricate policy landscapes with greater precision and confidence. Looking toward the horizon, the integration of artificial intelligence technologies with agent-based modeling promises to revolutionize how we approach complex policy challenges.
The evolution from current modeling approaches to more sophisticated AI-driven systems will enable increasingly nuanced simulations of human behavior and system dynamics, leading to more accurate and actionable policy recommendations. Future advancements in computational capabilities will undoubtedly expand the scope and scale of agent-based modeling.
As processing power grows and algorithms become more sophisticated, policymakers will be able to simulate increasingly complex scenarios with greater precision, leading to more effective and targeted policy interventions.
The convergence of AI technologies and agent-based modeling represents more than just a technological advancement – it signifies a fundamental shift in how we understand and shape policy outcomes. This synthesis will enable policymakers to better anticipate the ripple effects of their decisions across interconnected systems, ultimately leading to more resilient and adaptable policy frameworks.
While challenges remain in fully realizing the potential of agent-based modeling, the path forward is clear. Through continued innovation and the thoughtful integration of emerging technologies, we stand poised to enter a new era of evidence-based policymaking that better serves the complex needs of our evolving society.
Last updated:
Disclaimer: The information presented in this article is for general informational purposes only and is provided as is. While we strive to keep the content up-to-date and accurate, we make no representations or warranties of any kind, express or implied, about the completeness, accuracy, reliability, suitability, or availability of the information contained in this article.
Any reliance you place on such information is strictly at your own risk. We reserve the right to make additions, deletions, or modifications to the contents of this article at any time without prior notice.
In no event will we be liable for any loss or damage including without limitation, indirect or consequential loss or damage, or any loss or damage whatsoever arising from loss of data, profits, or any other loss not specified herein arising out of, or in connection with, the use of this article.
Despite our best efforts, this article may contain oversights, errors, or omissions. If you notice any inaccuracies or have concerns about the content, please report them through our content feedback form. Your input helps us maintain the quality and reliability of our information.