Agent-Based Modeling in Healthcare
Imagine a world where we can predict how diseases spread, test different healthcare policies, and optimize hospital operations before implementing them in real life. This is the powerful reality of agent-based modeling (ABM) in healthcare today.
Healthcare systems are incredibly complex, involving countless interactions between patients, medical staff, resources, and policies. Traditional analysis methods often fall short of capturing these intricate dynamics. Agent-based modeling offers a transformative approach to understanding and improving healthcare delivery.
Think of agent-based modeling as a sophisticated simulation where individual ‘agents’—whether they are doctors, nurses, patients, or even entire hospital departments—interact with each other according to specific rules. These interactions create patterns and outcomes that help us understand how real healthcare systems work and how we can make them better.
Agent-based modeling has made inroads into substantive disciplines. Over the past decade, public health research has embraced social simulation as a method to better understand the population effects of interventions, to model interactions between individual and socio-economic effects on health, and to understand the role of social influence in health behaviors.
Social Simulation Conference 2023
This article explores the fascinating world of agent-based modeling in healthcare. We’ll delve into its practical applications in improving patient care, examine the challenges healthcare professionals face when implementing these models, and look into the future of this transformative technology. Whether you are a healthcare administrator, medical researcher, or simply curious about how technology is changing healthcare, you will discover how ABM is reshaping the way we think about and deliver healthcare services.
Applications of Agent-Based Modeling in Public Health
Agent-based modeling (ABM) has emerged as a powerful computational approach for understanding complex public health challenges.
By simulating how individuals interact with each other and their environment according to predefined rules, ABMs offer unique insights that traditional statistical methods cannot capture. In infectious disease epidemiology, ABMs have proven particularly valuable for modeling disease transmission and evaluating control strategies.
For example, researchers have used ABMs to assess vaccination strategies for bioterrorism response, tuberculosis control measures, and targeted antiviral prophylaxis during influenza outbreaks. These models can simulate how diseases spread through populations while accounting for individual behaviors and environmental factors. Beyond infectious diseases, ABMs have been instrumental in studying health behaviors and lifestyle interventions.
Researchers employ these models to examine how social networks and environmental factors influence behaviors like physical activity, dietary choices, and smoking. This approach helps identify which intervention strategies might be most effective by simulating different scenarios before implementing costly real-world programs.
One of the most powerful applications of ABMs in public health is policy assessment. These models allow researchers to conduct virtual experiments testing different intervention strategies in simulated populations.
For instance, ABMs can evaluate how changes in the built environment might affect walking behavior or how communication campaigns could influence vaccine uptake during an epidemic. The strength of ABMs lies in their ability to capture complex social dynamics and feedback loops that characterize many public health challenges. By modeling individual decision-making while accounting for social influences and environmental contexts, ABMs provide insights into how population-level health patterns emerge from individual behaviors and interactions.
Public health practitioners increasingly recognize ABMs as valuable tools for both research and planning. These models help bridge the gap between individual behavior and population health outcomes, offering a sophisticated approach to understanding and addressing public health challenges in our interconnected world.
Addressing Healthcare Workforce Dynamics with ABM
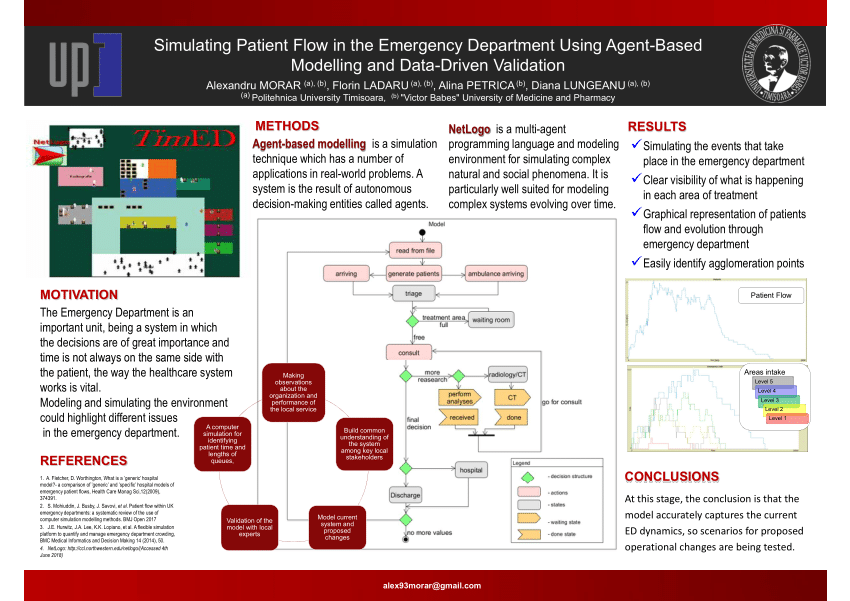
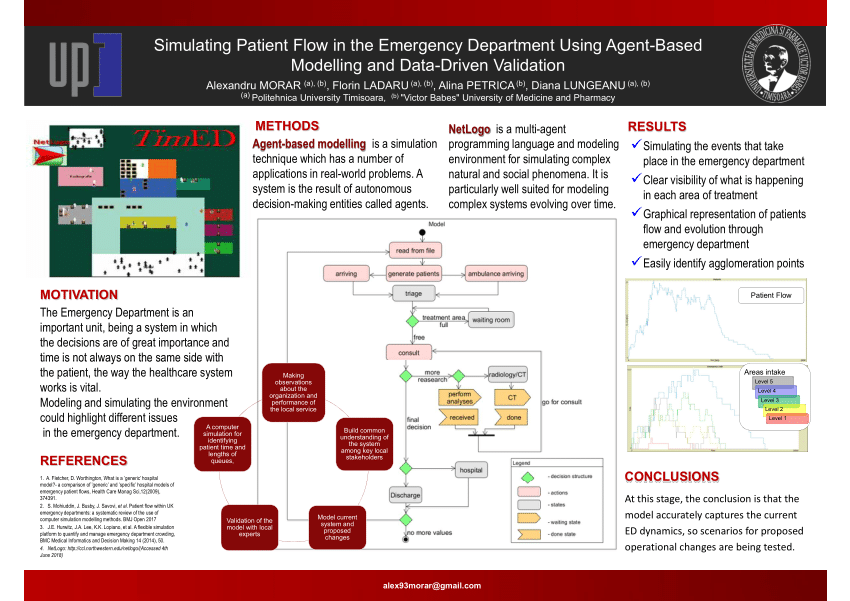
Agent-based modeling (ABM) has emerged as a powerful tool for healthcare administrators grappling with complex staffing and resource challenges. Unlike traditional planning approaches, ABM creates a virtual healthcare environment where individual staff members, patients, and resources interact in ways that mirror real-world dynamics.
The true strength of ABM lies in its ability to simulate realistic human behavior and decision-making within healthcare settings. When a nurse decides how to prioritize multiple patient needs or when a doctor determines the best allocation of their time between different departments, ABM can capture these intricate choices and their ripple effects throughout the system. According to a recent study, data-driven ABM simulations have demonstrated superior results in staff and resource allocation compared to conventional manual planning methods.
Healthcare facilities are using ABM to test various staffing scenarios before implementation. For instance, administrators can simulate how different nurse-to-patient ratios affect patient wait times, care quality, and staff workload. These virtual experiments help identify potential bottlenecks and inefficiencies without disrupting actual patient care or risking staff burnout.
Resource allocation becomes more precise with ABM’s predictive capabilities. The model can anticipate peak demand periods and suggest optimal distribution of medical equipment, beds, and specialized staff across departments. This proactive approach helps prevent the common scenario of having too many resources in one area while another department faces shortages.
Perhaps most importantly, ABM helps healthcare organizations build resilience into their workforce planning. By simulating unexpected scenarios – from sudden patient surges to staff absences – administrators can develop robust contingency plans. This capability proved especially valuable during the recent global health crisis, where hospitals needed to rapidly adjust their staffing models to meet unprecedented demands.
Modeling Chronic Disease Management Using ABM
Agent-based modeling (ABM) represents a powerful breakthrough in our ability to understand and manage complex chronic diseases. Unlike traditional statistical approaches that often oversimplify health dynamics, ABM captures the intricate web of factors that influence disease progression and treatment outcomes by simulating individual patient behaviors within larger healthcare systems.
Thanks to its sophisticated modeling capabilities, ABM has emerged as a promising systems science methodology that can integrate multiple layers of complexity – from individual patient decisions about medication adherence to population-level impacts of various interventions. This allows healthcare providers and policymakers to test different scenarios and interventions before implementing them in the real world.
One of ABM’s most valuable attributes is its ability to simulate how patients with chronic conditions like diabetes or heart disease respond to different treatment approaches over time. The model can account for various factors affecting patient behaviors, including social influences, economic constraints, and psychological factors – elements that traditional models often struggle to incorporate effectively.
Healthcare organizations can leverage ABM to design more effective disease management programs by understanding how different patient populations might respond to specific interventions. For instance, an ABM simulation might reveal that a diabetes management program would be more successful if it combined medication adherence support with peer group activities, rather than focusing on medication alone.
The practical applications of ABM extend beyond individual patient care to inform broader healthcare policy decisions. By simulating the long-term impacts of different policy interventions across diverse populations, decision-makers can better allocate resources and design programs that achieve optimal outcomes. This evidence-based approach helps healthcare systems move beyond one-size-fits-all solutions to develop more targeted and effective chronic disease management strategies.
Challenges in Implementing ABM in Healthcare
Healthcare organizations face several key hurdles when implementing agent-based modeling, despite its powerful potential for improving patient care and operational efficiency. Ensuring data quality is one of the most significant challenges. Healthcare systems generate vast amounts of complex patient data, but this information often contains inconsistencies, missing values, and variations in how it is recorded across different departments and facilities.
Model validation poses another crucial challenge. As noted by researchers in a recent PLOS ONE study, validating healthcare ABMs requires replicating hospital-specific conditions and developing new metrics for validating social-environmental network structures. Traditional validation methods often fall short when dealing with the complex interactions between patients, staff, and hospital environments.
The integration of ABMs into existing healthcare systems presents its own set of difficulties. Many hospitals operate with legacy systems that were not designed to accommodate advanced modeling tools. Healthcare professionals must also learn to interpret and trust the model’s outputs, which requires significant training and change management efforts.
Data privacy and security requirements add another layer of complexity. Healthcare organizations must ensure their ABM implementations comply with regulations like HIPAA while still maintaining enough detail in the data to produce meaningful insights. This balancing act between privacy and utility often requires sophisticated data anonymization techniques.
Resource constraints further complicate ABM implementation. Developing and maintaining accurate models demands substantial computational power, specialized expertise, and ongoing investment. Smaller healthcare facilities may struggle to allocate sufficient resources to support comprehensive ABM programs.
To address these challenges, healthcare organizations should consider implementing standardized data collection protocols, investing in robust validation frameworks, and adopting modular approaches that allow for gradual integration of ABM capabilities. Cross-institutional collaboration can also help share costs and expertise, making ABM more accessible to a broader range of healthcare providers.
Leveraging SmythOS for Advanced ABM Development
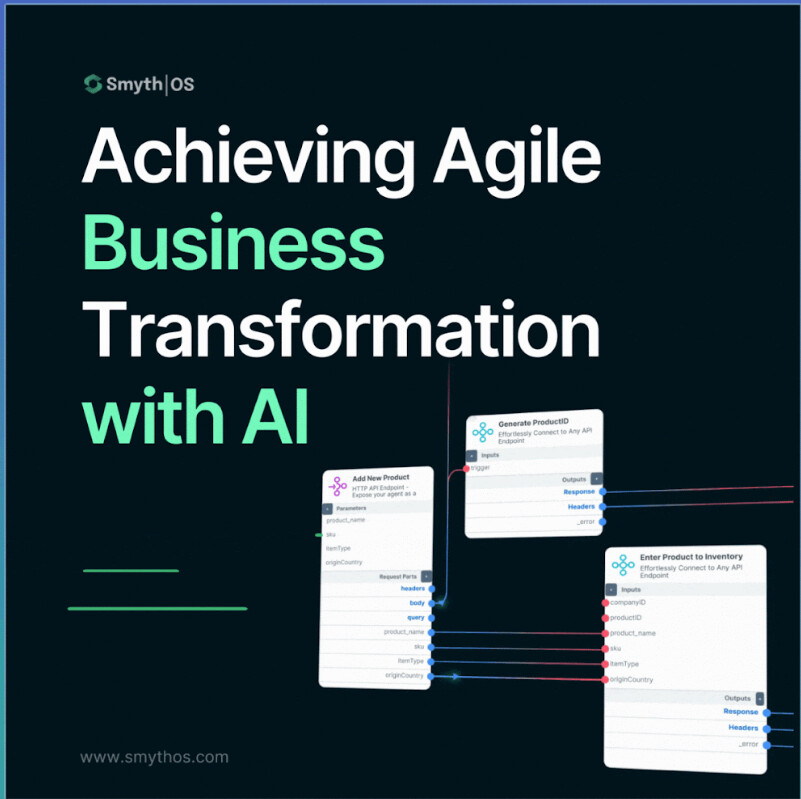
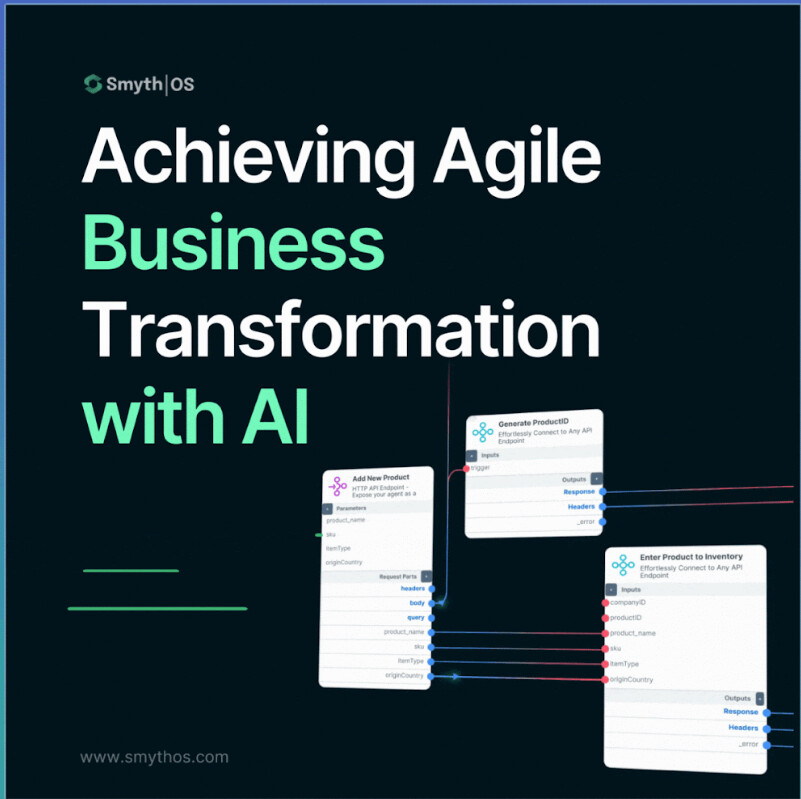
Agile transformation through interconnected API processes. – Via imgix.net
SmythOS revolutionizes Agent-Based Modeling (ABM) development through its sophisticated yet accessible platform design. At its core, the system provides developers with a visual workflow builder that transforms traditionally complex coding tasks into an intuitive drag-and-drop experience. This approach democratizes ABM development while maintaining the power needed for advanced applications.
The platform’s built-in monitoring capabilities set it apart from conventional development environments. Developers can track agent interactions in real-time, allowing for immediate insights into model behavior and performance. This feature proves invaluable when fine-tuning complex simulations, as it enables rapid identification and resolution of unexpected behaviors or performance bottlenecks.
Integration capabilities form another cornerstone of SmythOS’s ABM toolkit. The platform seamlessly connects with external APIs, data sources, and tools, allowing developers to enhance their models with real-world data streams and third-party services. This flexible integration framework enables the creation of more sophisticated and realistic simulations without requiring extensive custom coding.
The visual builder represents a significant advancement in ABM development efficiency. Rather than wrestling with complex code structures, developers can assemble their models using pre-built components and visual workflows. This approach not only accelerates the development process but also makes it more accessible to researchers and practitioners who may not have extensive programming backgrounds.
SmythOS’s approach to resource management further enhances the development experience. The platform handles automatic scaling and load balancing, ensuring that even complex models with thousands of agents run efficiently. This infrastructure management happens behind the scenes, allowing developers to focus on model design and behavior rather than technical implementation details.
SmythOS is not just a tool; it’s a game-changer for agent-based modeling. Its visual approach and reusable components make it possible to build and iterate on complex models in a fraction of the time it would take with traditional methods.
Future Directions in Agent-Based Modeling for Healthcare
Agent-based modeling (ABM) stands at an exciting crossroads in healthcare, with emerging capabilities that promise to transform how we understand and improve patient outcomes. The integration of real-time data streams represents a particularly promising frontier, enabling models to adapt and respond to changing healthcare conditions with unprecedented speed and accuracy.
Model validation techniques are evolving rapidly, responding to the growing need for robust and reliable simulations. As detailed in a comprehensive review in the Annual Review of Public Health, researchers are developing more sophisticated approaches to verify ABM results against empirical data, helping ensure these models provide actionable insights for healthcare decision-makers.
The expansion into personalized medicine represents one of the most promising applications of advanced ABM technologies. By simulating individual patient characteristics, genetic factors, and treatment responses, these models can help predict optimal therapeutic approaches for specific patients. This level of granularity in modeling marks a significant departure from traditional population-level analyses.
Integration of real-time data from electronic health records, wearable devices, and environmental sensors will dramatically enhance the predictive power of agent-based models. This continuous flow of information allows models to capture the dynamic nature of healthcare systems more accurately than ever before, enabling rapid response to emerging health challenges and evolving patient needs.
Looking ahead, the convergence of ABM with artificial intelligence and machine learning algorithms will unlock new possibilities for healthcare modeling. These hybrid approaches will enable more sophisticated analysis of complex healthcare interactions, from hospital operations to disease transmission patterns, while maintaining the interpretability that makes ABM so valuable for healthcare decision-making.
Last updated:
Disclaimer: The information presented in this article is for general informational purposes only and is provided as is. While we strive to keep the content up-to-date and accurate, we make no representations or warranties of any kind, express or implied, about the completeness, accuracy, reliability, suitability, or availability of the information contained in this article.
Any reliance you place on such information is strictly at your own risk. We reserve the right to make additions, deletions, or modifications to the contents of this article at any time without prior notice.
In no event will we be liable for any loss or damage including without limitation, indirect or consequential loss or damage, or any loss or damage whatsoever arising from loss of data, profits, or any other loss not specified herein arising out of, or in connection with, the use of this article.
Despite our best efforts, this article may contain oversights, errors, or omissions. If you notice any inaccuracies or have concerns about the content, please report them through our content feedback form. Your input helps us maintain the quality and reliability of our information.