Agent-based Modeling in Finance: Revolutionizing Market Simulations and Risk Management
Financial markets hold countless untold stories of complex interactions between banks, traders, and institutions, each making decisions that ripple through the system in often unexpected ways. This intricate dance of market participants has historically been difficult to model and predict, but agent-based modeling (ABM) is changing that reality.
ABM can be seen as a sophisticated simulation where every market participant, from major banks to individual traders, is represented by an autonomous digital agent making independent decisions. These agents interact and adapt their behaviors based on changing market conditions, much like their real-world counterparts. This approach is particularly powerful because it reveals how seemingly simple individual actions can combine to create complex market-wide phenomena.
According to recent research in risk management, agent-based models have proven especially valuable for identifying systemic risks that traditional models often miss. Rather than assuming all market participants behave identically, ABM embraces the reality of heterogeneous agents—some following trends, others trading on fundamentals, and still others adapting their strategies as markets evolve.
The applications of this methodology are remarkably diverse. From predicting the cascading effects of bank failures to understanding housing market dynamics, ABM offers unprecedented insight into market behavior. Most importantly, it helps us grasp how individual decisions aggregate into market-wide movements and potential systemic risks.
ABM is particularly relevant today because of its predictive power. By modeling the complex interplay of diverse market participants, it can anticipate market impacts and identify potential vulnerabilities before they manifest in the real world. This proactive capability is transforming how financial institutions and regulators approach risk management and policy decisions.
Key Concepts of Agent-Based Modeling
Agent-based modeling (ABM) offers a robust method for understanding complex systems by focusing on three core elements: agent heterogeneity, interaction rules, and dynamic adaptation. But what do these elements mean for modeling real-world systems?
Agent heterogeneity refers to the natural diversity seen in financial markets. Just as investors have different risk tolerances, resources, and strategies, ABM allows us to create agents with varying characteristics and behaviors. As noted in research on model performance, this heterogeneity can manifest through different agent characteristics (like income levels) and distinct decision-making rules.
The interaction rules form the backbone of how agents engage with each other and their environment. In financial systems, these rules might dictate how traders respond to market signals, how information flows between market participants, or how prices adjust based on supply and demand. These rules create the complex web of relationships that drive market dynamics.
Dynamic adaptation represents how agents learn and evolve over time based on their experiences and changing conditions. For example, traders might adjust their strategies based on past performance or market conditions. This adaptive behavior helps explain how financial markets can shift dramatically as participants collectively respond to new information or changing circumstances.
Unlike traditional economic models that often rely on assumptions of rational, identical actors, ABM embraces the messiness of real-world behavior. Through these three key elements, ABM can capture the emergence of complex market behaviors – from sudden price swings to the formation of trading patterns – that often puzzle conventional economic analysis.
The power of ABM lies in its ability to simulate how these individual-level behaviors and interactions aggregate into system-wide patterns. Whether modeling financial crises, market bubbles, or the impact of new regulations, ABM provides insights into how complex market behaviors emerge from the bottom up.
Agent-based modeling is not just another economic tool – it represents a fundamental shift in how we understand complex systems, allowing us to capture the rich tapestry of behaviors and interactions that drive real-world markets.
Rai & Henry, Nature Climate Change
Applications in Financial Markets
Agent-based modeling (ABM) has emerged as a powerful tool for understanding and predicting complex dynamics in financial markets. By simulating interactions between diverse market participants – from individual traders to large institutional investors – ABM provides valuable insights into price movements and market behavior that traditional analytical methods often miss.
One significant application of ABM in finance is predicting market volatility and price movements. Recent research has demonstrated how adaptive agents that switch between fundamentalist and chartist behaviors can effectively model market dynamics and forecast potential price changes. These models are particularly valuable when simulating how markets react to new information or sudden changes in trading patterns.
ABM also serves as a critical tool for examining the impact of regulatory policies on financial markets. Rather than relying on broad assumptions, regulators can use agent-based simulations to test how different policy interventions might affect market stability and systemic risk. This approach allows policymakers to identify potential unintended consequences before implementing new regulations.
The ability to assess systemic risks represents another transformative application of ABM in finance. Studies have shown that ABM can effectively model how financial distress propagates through networks of interconnected institutions, helping identify potential sources of systemic risk before they materialize into full-blown crises.
Market trend forecasting through ABM has proven particularly valuable during periods of high uncertainty. By simulating multiple scenarios with varying agent behaviors and market conditions, analysts can generate more robust predictions about potential market trajectories. This capability becomes especially important during market stress periods when traditional forecasting methods may break down.
The true power of agent-based modeling lies in its ability to capture the complex, nonlinear interactions that drive financial markets – something that conventional models often struggle to achieve.
Amanda M. Y. Chu, Researcher at The Education University of Hong Kong
Most importantly, ABM helps market participants better understand the feedback loops and cascading effects that can amplify market movements. By modeling how different types of traders respond to and influence each other’s behavior, ABM provides crucial insights into the mechanisms behind market crashes and rallies.
Overcoming Modeling Challenges
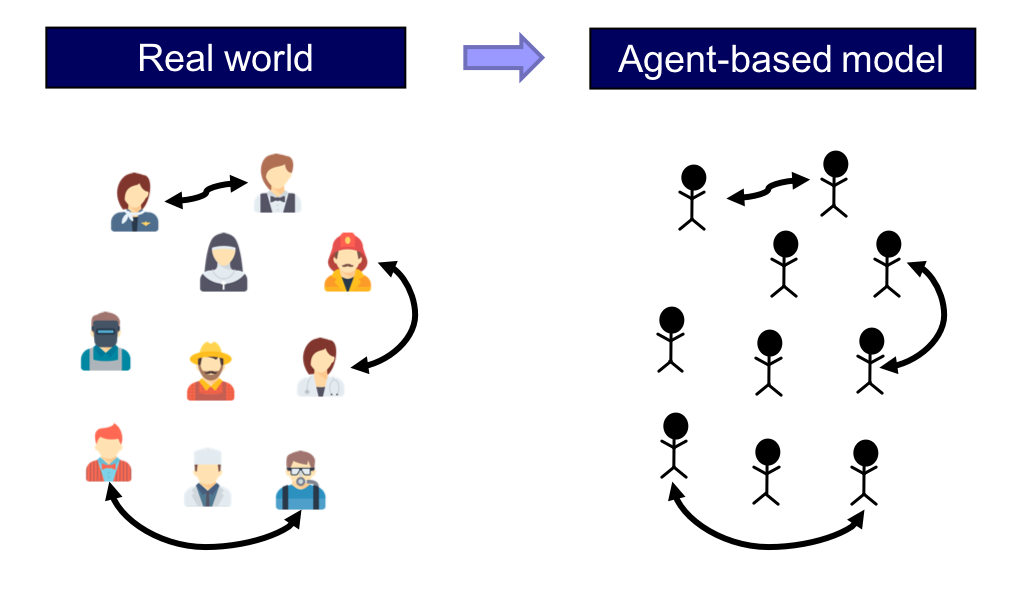
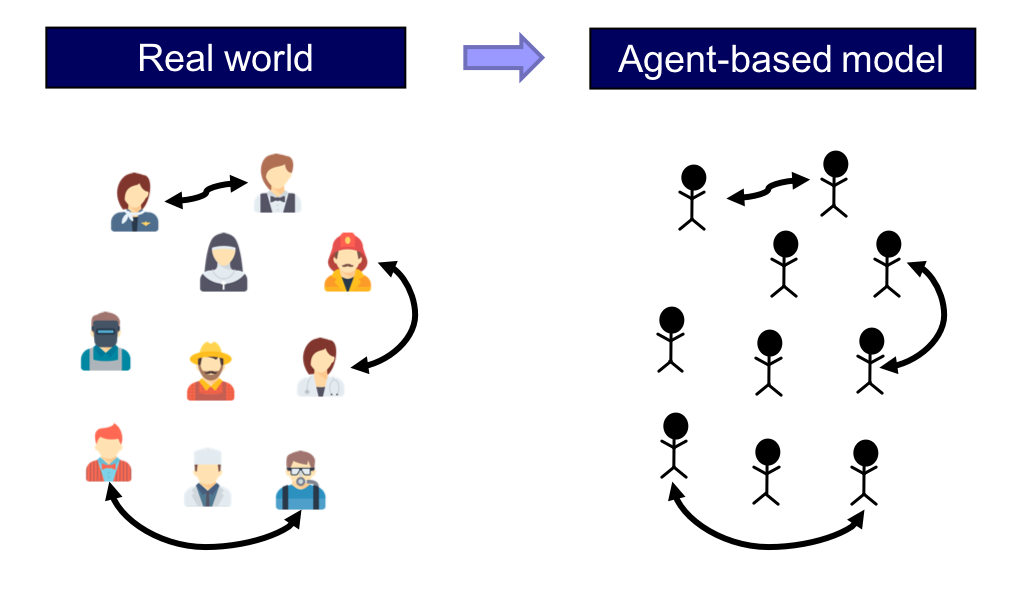
Agent-based modeling (ABM) in finance presents unique hurdles that demand careful consideration and systematic approaches. The complexity of financial markets, with their interconnected participants and evolving dynamics, requires sophisticated modeling techniques that can capture these intricate relationships while maintaining accuracy.
Data integration stands as a primary challenge in developing effective ABMs. Financial institutions must synthesize information from multiple sources, including market data feeds, trading records, and economic indicators. As noted by research published in JSTOR, agent-based models must overcome limitations of traditional economic models when dealing with financial markets, particularly in capturing complex interactions between different market participants.
Bias mitigation represents another critical challenge. Models can inadvertently incorporate various biases, from selection bias in historical data to confirmation bias in assumption setting. Teams must implement rigorous validation protocols and cross-checking mechanisms to ensure their models remain objective and reliable. This includes regular backtesting against historical data and performing sensitivity analyses to understand how different assumptions impact model outcomes.
Interdisciplinary collaboration emerges as a crucial element in addressing these challenges. Successful ABM development requires input from multiple experts – quantitative analysts who understand the mathematical foundations, domain experts who grasp market dynamics, and software engineers who can implement efficient computing solutions. This collaborative approach helps ensure that models capture both the technical and practical aspects of financial markets.
Computational constraints also pose significant challenges. Complex ABMs often require substantial processing power to simulate thousands of agents interacting simultaneously. Organizations must balance model sophistication with practical limitations, often requiring innovative solutions in model design and implementation. Teams frequently need to optimize their code and leverage advanced computing infrastructure to maintain model performance without sacrificing accuracy.
Documentation and model transparency present ongoing challenges that require careful attention. Clear documentation of assumptions, methodologies, and validation results helps ensure models remain maintainable and trustworthy over time. Teams must establish robust documentation practices that allow other stakeholders to understand and validate the model’s behavior.
Despite these challenges, organizations can implement several practical strategies to enhance model accuracy. Regular calibration against real market data, implementing systematic testing procedures, and maintaining flexible model architectures that can adapt to changing market conditions all contribute to more reliable results. The key lies in developing systematic approaches to address each challenge while maintaining a balance between model complexity and practical usability.
The Role of SmythOS in ABM Development
Finance’s complex ecosystem demands sophisticated agent-based models (ABMs) that can adapt and scale seamlessly. SmythOS transforms this challenging landscape with its comprehensive suite of development tools, making ABM creation and deployment more accessible than ever before.
SmythOS features an intuitive visual workflow builder that empowers developers to construct complex ABM logic without deep coding. This drag-and-drop interface accelerates development cycles while ensuring model integrity, allowing financial institutions to rapidly prototype and iterate their agent-based systems.
Built-in monitoring capabilities set SmythOS apart in the financial technology sphere. The platform provides real-time insights into agent behaviors and system performance, enabling teams to identify and address potential issues before they impact operations. This proactive approach to system health maintains the reliability crucial for financial applications.
Security and scalability form the cornerstone of SmythOS’s design philosophy. The platform’s robust architecture ensures that as models grow in complexity and scale, performance remains consistent while maintaining stringent security protocols essential for financial data protection.
Integration capabilities further enhance SmythOS’s value proposition. The platform seamlessly connects with existing financial systems through standardized APIs, eliminating the traditional barriers between ABM implementation and legacy infrastructure. This interoperability ensures that organizations can leverage their existing investments while embracing advanced modeling techniques.
SmythOS is not just a tool; it’s a game-changer for agent-based modeling. Its visual approach and reusable components make it possible to build and iterate on complex models in a fraction of the time it would take with traditional methods.
The comprehensive logging system provides an unbroken chain of model behavior documentation, essential for audit trails and regulatory compliance in financial environments. This detailed record-keeping enables teams to trace decisions, optimize performance, and demonstrate model reliability to stakeholders.
Future Trends in Agent-Based Modeling
The convergence of artificial intelligence and machine learning with agent-based modeling heralds a transformative era in financial forecasting.
Recent research from the Institute for New Economic Thinking suggests that these technologies are revolutionizing how we understand and predict market behaviors, offering unprecedented accuracy in financial analysis. Sophisticated AI-powered agents now possess the capability to process vast amounts of market data in real-time, identifying patterns and correlations that human analysts might miss.
These advanced models adapt dynamically to changing market conditions, learning from each interaction to refine their predictive capabilities. The integration of deep learning algorithms enables these agents to develop an increasingly nuanced understanding of complex market dynamics.
What makes this evolution particularly exciting is the potential for multi-agent systems to simulate entire market ecosystems with remarkable precision. These systems can model thousands of individual market participants, each with their own decision-making patterns and risk preferences, creating a more realistic representation of market behavior. This granular level of simulation helps identify potential market risks and opportunities that traditional modeling approaches might overlook.
One of the most promising developments is the emergence of hybrid models that combine the adaptability of AI with the structured approach of traditional financial analysis. These models don’t just predict market movements; they provide detailed insights into the underlying factors driving those changes.
For instance, they can simultaneously analyze macroeconomic indicators, company fundamentals, and market sentiment to generate more comprehensive forecasts. Looking ahead, we will likely see the development of increasingly autonomous trading systems that can not only predict market trends but also execute complex trading strategies with minimal human intervention.
However, this automation won’t eliminate the need for human oversight; instead, it will elevate the role of financial professionals to focus more on strategy development and risk management rather than routine analysis. The fusion of AI and agent-based modeling represents a quantum leap in our ability to understand and navigate financial markets. We are moving from simple prediction to true market intelligence.
Perhaps most intriguingly, these advancements in agent-based modeling could help prevent financial crises by identifying systemic risks before they manifest. By simulating various market scenarios and stress conditions, these models can help regulators and financial institutions better prepare for potential market disruptions, ultimately contributing to a more stable and resilient financial system.
Conclusion and Implications
Agent-based modeling has emerged as a transformative force in financial markets, offering unprecedented capabilities for understanding complex market dynamics and predicting potential risks. Through detailed simulations of individual market participants, ABMs illuminate how micro-level interactions cascade into macro-level market phenomena, providing crucial insights for risk management and policy decisions.
The integration of advanced AI capabilities through platforms like SmythOS’s visual debugging environment has revolutionized how researchers and practitioners develop and deploy these models. This technological advancement ensures greater efficiency and robustness in model development, reducing the traditional barriers to creating sophisticated financial simulations.
The practical implications of these developments are far-reaching. Financial institutions can now better anticipate market movements and potential crisis scenarios, while regulators gain powerful tools for testing policy interventions before implementation. This enhanced predictive capability represents a significant step forward in maintaining market stability and protecting against systemic risks.
Looking ahead, the convergence of ABMs with artificial intelligence promises even more sophisticated modeling capabilities. As computing power increases and machine learning algorithms become more refined, future models will likely capture market dynamics with unprecedented accuracy and granularity. This evolution will enable more nuanced risk assessment and better-informed decision-making across the financial sector.
The financial modeling landscape stands at an exciting crossroads where traditional economic theory meets cutting-edge technology. By combining the structural insights of agent-based modeling with the analytical power of AI, we’re entering an era of more sophisticated, reliable, and actionable market analysis – one that could fundamentally transform how we understand and interact with financial markets.
Last updated:
Disclaimer: The information presented in this article is for general informational purposes only and is provided as is. While we strive to keep the content up-to-date and accurate, we make no representations or warranties of any kind, express or implied, about the completeness, accuracy, reliability, suitability, or availability of the information contained in this article.
Any reliance you place on such information is strictly at your own risk. We reserve the right to make additions, deletions, or modifications to the contents of this article at any time without prior notice.
In no event will we be liable for any loss or damage including without limitation, indirect or consequential loss or damage, or any loss or damage whatsoever arising from loss of data, profits, or any other loss not specified herein arising out of, or in connection with, the use of this article.
Despite our best efforts, this article may contain oversights, errors, or omissions. If you notice any inaccuracies or have concerns about the content, please report them through our content feedback form. Your input helps us maintain the quality and reliability of our information.