Agent-based Modeling in Energy Systems
Picture a virtual world where thousands of decision-makers, from homeowners installing solar panels to power plant operators managing electricity production, interact and shape our energy future. This is the realm of agent-based modeling (ABM), a transformative approach that’s optimizing complex energy systems.
Through ABM, researchers can simulate how different players in the energy sector make choices and interact in ways that affect the entire system. As noted in recent energy research, ABM is valuable for modeling consumer behavior in renewable energy adoption and analyzing market dynamics in detail.
ABM’s power lies in its ability to capture the ripple effects of individual decisions across the energy landscape. Whether predicting how new solar incentives might affect adoption rates or understanding how electric vehicle charging patterns could impact grid stability, ABM provides insights that traditional modeling approaches can’t match.
In this guide, we’ll explore how ABM is transforming energy system planning and operation. You’ll discover the key benefits of this modeling approach, understand its practical applications, and learn about the challenges developers face and strategies for overcoming them. For energy professionals and developers looking to harness the power of ABM, this article offers a roadmap to implementing these sophisticated modeling techniques effectively.
The energy sector stands at a critical juncture, facing challenges from renewable integration to grid modernization. Agent-based modeling emerges as a vital lens to better understand and shape our energy future. Let’s dive into how this approach is helping create more efficient, sustainable, and resilient energy systems.
The Role of Agent-based Modeling in Energy Transition
Agent-based modeling (ABM) has emerged as a powerful tool for understanding the complex dynamics of energy transitions. Unlike traditional forecasting methods, ABM excels at capturing the intricate interactions between consumers, utilities, policymakers, and market forces that shape our evolving energy landscape.
ABM helps stakeholders predict how different groups will respond to new energy policies and technologies. For example, when studying the adoption of residential solar panels, ABM can simulate how homeowners influence their neighbors’ decisions through social networks and information sharing. These insights prove invaluable for utilities and policymakers working to accelerate renewable energy adoption.
One of ABM’s most significant advantages is its ability to model consumer behavior realistically. As recent research has shown, the methodology can account for diverse factors influencing energy choices – from financial considerations to social dynamics and environmental values. This granular understanding helps policymakers design more effective incentives and programs.
The technology’s predictive capabilities extend beyond individual choices to system-wide impacts. When evaluating potential policies, ABM can reveal unexpected consequences and feedback loops that simpler models might miss. For instance, it can demonstrate how consumer adoption of electric vehicles might affect grid stability, electricity prices, and overall emission reductions.
Policy planning particularly benefits from ABM’s scenario-testing capabilities. Rather than relying on broad assumptions, decision-makers can experiment with different policy combinations in a virtual environment. This approach has already helped several jurisdictions optimize their renewable energy incentives and grid modernization strategies.
The greatest potential contribution of ABM to energy transition studies lies in its practical application to decision-making in policy and planning.
Paula Hansen, Energy Research & Social Science
Beyond policy design, ABM serves as an essential tool for utilities and energy companies navigating the transition. The technology helps them understand changing consumer preferences, predict adoption rates of new technologies, and plan infrastructure investments accordingly. This strategic foresight proves crucial as the energy sector becomes increasingly decentralized and consumer-driven.
Applications of ABM in Energy System Analysis
Agent-based modeling (ABM) has emerged as a powerful tool for understanding complex energy systems. Unlike traditional modeling approaches, ABM can simulate the intricate interactions between various components of the power grid while accounting for human behavior and market forces that shape energy consumption patterns.
The application of ABM in electricity demand analysis allows researchers to model how individual consumers, businesses, and industries respond to pricing signals and incentives. By simulating these behaviors at a granular level, utilities and policymakers can better predict peak demand periods and develop more effective demand response programs.
ABM offers unique insights into the adoption and integration of renewable energy technologies in distributed generation. Research has shown that ABM serves as an excellent testbed for analyzing new paradigms in smart grids, particularly in understanding how homeowners and businesses decide to invest in solar panels or other renewable energy systems.
Grid stability analysis through ABM helps operators understand how multiple distributed energy resources affect the overall system reliability. These models simulate various scenarios, from sudden changes in renewable energy output to equipment failures, allowing grid operators to develop more robust contingency plans. ABM and simulation techniques have gained significant recognition in studying electricity systems and markets, particularly as power grids become increasingly complex and decentralized.
Market dynamics play a crucial role in energy system modeling. ABM can simulate how different market participants – from large power plants to small-scale producers – interact and make decisions based on economic incentives. These simulations help policymakers design more effective market mechanisms and subsidies to encourage renewable energy adoption.
Challenges and Limitations of Agent-based Models
Agent-based models (ABMs) have emerged as powerful tools for simulating complex systems, yet they face several significant hurdles that researchers must navigate. Data quality is a primary challenge, as ABMs require extensive, accurate data to initialize agents and validate model outcomes. Collecting sufficiently detailed real-world data about individual behaviors and interactions often proves difficult and resource-intensive.
The computational demands of ABMs present another major obstacle. As researchers have noted, these models can strain even advanced computing systems, especially when simulating large populations or complex interaction networks. Running multiple iterations for validation requires substantial processing power and time, making high-performance computing resources essential yet not always accessible to all research teams.
Model validation, verifying that an ABM accurately represents the real-world system it aims to simulate, is perhaps the most challenging aspect. Traditional validation techniques often fall short when applied to ABMs due to their inherent complexity and stochastic nature. Researchers must develop and employ comprehensive validation approaches that can account for emergent behaviors and non-linear interactions between agents.
Solutions to these challenges are emerging but require significant investment and collaboration. Cloud computing platforms can help address computational limitations, while advances in data collection methods, including sensor networks and digital traces, are improving data quality. The development of standardized validation frameworks and tools is also helping researchers better verify their models.
The field continues to evolve as practitioners work to overcome these obstacles. Success requires not just technological advancement but also methodological innovation—finding new ways to collect relevant data, optimize computational efficiency, and validate complex models. As these challenges are addressed, ABMs will become even more valuable tools for understanding and predicting complex system behaviors.
Case Studies of ABM in Energy Systems
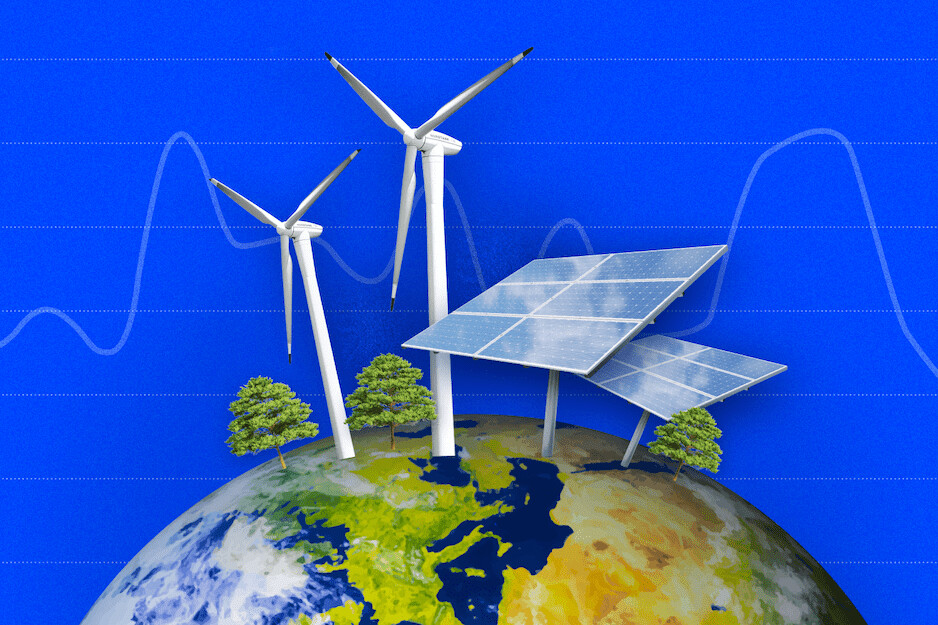
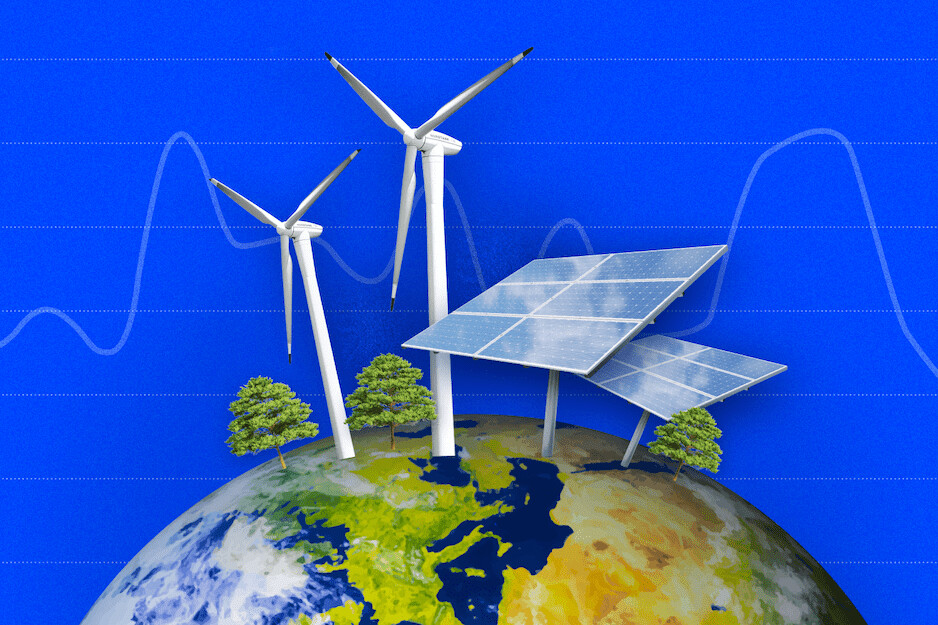
Agent-based modeling (ABM) has emerged as a powerful tool for analyzing complex energy systems, with several real-world applications demonstrating its effectiveness across various scenarios. Let’s explore some compelling case studies that showcase ABM’s practical impact.
Researchers have successfully used ABM to analyze how community energy systems develop and operate. These models have helped planners understand how different participants – from residential consumers to industrial users – interact within energy communities, leading to more effective system designs and better economic outcomes. Studies show community electricity cost savings of up to 42% when using ABM-optimized configurations.
Country | Electricity Cost Savings |
---|---|
Sweden | 66% |
Finland | 47.9% |
Annual Savings (2022 vs 2021) | From 37.8% to 41.2% |
The transportation sector offers another fascinating application of ABM. When studying electric vehicle adoption patterns, researchers employ these models to simulate how charging infrastructure deployment affects adoption rates. The models account for diverse factors including driver behavior, charging station availability, and grid capacity constraints. This comprehensive approach helps city planners and utilities optimize charging infrastructure placement for maximum impact. Energy storage solutions represent a particularly innovative application of ABM.
In urban settings, models have helped determine optimal battery sizing and placement by simulating various scenarios of energy demand and supply. One notable study revealed that properly configured energy storage systems could increase community self-sufficiency rates from 35% to 54% when combined with solar installations.
ABM’s versatility becomes evident in how it handles multi-stakeholder scenarios. The models can simultaneously account for individual household decisions, utility company strategies, and regulatory requirements – something traditional modeling approaches struggle to achieve. This capability has proven especially valuable when designing incentive programs and policy frameworks.
Beyond individual technologies, ABM has demonstrated remarkable effectiveness in analyzing integrated energy systems. Research shows these models can successfully simulate the complex interactions between renewable generation, storage systems, and flexible demand – providing insights that help optimize overall system performance and reliability.
Leveraging SmythOS for ABM Implementation
Agent-based modeling has emerged as a powerful tool for simulating complex energy systems, and SmythOS provides an innovative platform specifically designed to streamline ABM implementation. SmythOS offers a visual builder interface that transforms the traditionally code-heavy process of developing agent-based models into an intuitive, drag-and-drop experience.
The platform’s built-in monitoring capabilities set it apart by offering real-time visualization of agent interactions. Technical teams can pause simulations at any point, inspect individual agents, and modify parameters on the fly to observe immediate effects on model behavior. This level of granular control enables rapid prototyping and efficient debugging of complex energy system models.
Integration capabilities form another cornerstone of SmythOS’s value proposition. The platform seamlessly connects with external tools and data sources through its comprehensive API framework, enabling energy system modelers to incorporate real-world data streams and existing infrastructure into their simulations. This interoperability ensures models remain grounded in practical applications while maintaining flexibility for future expansions.
SmythOS truly shines in scalability. The platform’s architecture automatically handles resource management and load balancing, allowing models to grow from simple prototypes to complex enterprise-wide simulations without performance degradation. This scalability proves especially valuable for energy systems, where interactions between numerous agents – from individual smart meters to power plants – must be modeled simultaneously.
Enterprise security features provide the foundation for confidently deploying agent-based models in production environments. SmythOS implements robust access controls, encryption protocols, and audit trails to protect sensitive energy system data and model configurations. These security measures make it suitable for utilities and energy companies working with critical infrastructure simulations.
The platform’s visual approach and reusable components make it possible to build and iterate on complex models in a fraction of the time it would take with traditional methods.
By combining intuitive model development tools with enterprise-grade capabilities, SmythOS enables organizations to harness the full potential of agent-based modeling for energy system optimization. Whether simulating smart grid behavior, renewable energy integration, or demand response programs, the platform provides the foundation for building sophisticated models that drive real-world insights.
Future Directions for ABM in Energy Systems
As Agent-Based Modeling (ABM) evolves, advancements in data integration, model accuracy, and computational efficiency promise to transform energy system optimization. Future ABM platforms will seamlessly incorporate data from smart meters, grid sensors, and renewable energy sources, creating comprehensive models that capture the intricate relationships between energy production, distribution, and consumption patterns. As studies have demonstrated, this enhanced data synthesis will enable a nuanced understanding of socio-technical energy transition dynamics.
Model accuracy will improve through refined agent behavior algorithms and the inclusion of real-world complexity factors. Advanced validation techniques will ensure models represent actual energy system dynamics, from individual consumer choices to grid-level interactions, making ABM a reliable tool for policy planning and infrastructure development.
Breakthroughs in computational efficiency will expand ABM applications’ scope and scale. Optimized algorithms and parallel processing capabilities will allow future models to handle larger datasets and more complex scenarios, delivering faster results. This enhanced processing power will enable real-time analysis and dynamic adaptation of energy systems to changing conditions.
These advancements will strengthen ABM’s role in energy system optimization by providing deeper insights into the interplay between technical infrastructure, human behavior, and environmental factors. ABM will continue to drive innovation in sustainable energy transitions and support the development of more resilient and efficient energy systems.
Last updated:
Disclaimer: The information presented in this article is for general informational purposes only and is provided as is. While we strive to keep the content up-to-date and accurate, we make no representations or warranties of any kind, express or implied, about the completeness, accuracy, reliability, suitability, or availability of the information contained in this article.
Any reliance you place on such information is strictly at your own risk. We reserve the right to make additions, deletions, or modifications to the contents of this article at any time without prior notice.
In no event will we be liable for any loss or damage including without limitation, indirect or consequential loss or damage, or any loss or damage whatsoever arising from loss of data, profits, or any other loss not specified herein arising out of, or in connection with, the use of this article.
Despite our best efforts, this article may contain oversights, errors, or omissions. If you notice any inaccuracies or have concerns about the content, please report them through our content feedback form. Your input helps us maintain the quality and reliability of our information.