Generative AI Jobs: Navigating the Future of Work
The AI revolution is not just changing how we work; it’s transforming what work means. Recent research from Indeed’s Hiring Lab reveals a significant 1,848% increase in generative AI job postings throughout 2023, indicating a major shift in career opportunities.
For data scientists and enterprise architects, this transformation presents a unique opportunity. Industry experts suggest those in the top 20% of roles impacted by generative AI will gain remarkable advantages in efficiency, productivity, and career value.
Imagine a world where AI researchers craft algorithms for novel solutions, machine learning engineers build adaptive systems, and data scientists use generative models to unlock unprecedented insights. These scenarios are today’s reality.
This moment is extraordinary because we’re not just enhancing existing roles. New positions like AI prompt engineers, generative AI ethicists, and synthetic data specialists are emerging—roles that didn’t exist months ago.
As we navigate this new frontier, you’ll learn how these evolving positions are reshaping the tech landscape and how you can be at the forefront of this transformation. The future of work is here, and it’s more exciting than we imagined.
The Rise of AI Researchers in Gen AI
AI researchers are the driving force behind groundbreaking developments in generative artificial intelligence, pioneering sophisticated algorithms that expand possibilities. They blend expertise in mathematics, computer science, and specialized domains to craft increasingly capable AI models.
At major research institutions and tech companies, AI researchers tackle complex challenges in areas like large language models and computer vision. For instance, researchers at Cornell University have established crucial frameworks for developing responsible generative AI systems that maintain research integrity while advancing innovation.
Beyond model architecture, these scientists focus on making AI systems more reliable and efficient. They employ novel approaches like few-shot learning and transfer learning to enhance model performance with less training data. This work translates into more resource-efficient and sustainable AI development practices.
A key contribution of AI researchers lies in identifying innovative real-world applications. They collaborate across disciplines to adapt generative AI for specialized tasks—from accelerating drug discovery to enhancing creative workflows in design and content creation. This practical focus helps organizations realize concrete productivity gains.
The impact of their work extends beyond technical achievements. AI researchers also address crucial questions around model safety, bias mitigation, and ethical deployment. Their findings shape best practices and governance frameworks that ensure the responsible advancement of generative AI technology.
AI researchers are not just building models—they are architecting the future of human-AI collaboration through rigorous scientific inquiry and responsible innovation.
Guy Doron, Senior Insight Solutions Lead at Bayer Pharmaceuticals
As generative AI rapidly evolves, researchers remain essential for bridging the gap between cutting-edge capabilities and practical applications. Their work enables organizations to harness AI’s potential while addressing important considerations around safety, fairness, and societal impact.
Machine Learning Engineers: The Architects of AI
Machine learning engineers are the vital link between AI concepts and practical business solutions. These professionals use their technical skills and strategic insight to tackle complex business challenges through AI.
They are responsible for developing and deploying scalable machine learning systems. Collaborating with data scientists and business stakeholders, ML engineers create efficient data pipelines for processing large datasets.
A key part of their role is implementing MLOps best practices to ensure AI models are reliable in production. This involves setting up automated monitoring, version control for code and data, and clear deployment protocols.
Building Robust AI Infrastructure
AI project success relies on the infrastructure supporting it. ML engineers design systems to handle the computational demands of training and deploying models at scale.
They must balance performance, cost, and maintainability, optimizing resources while keeping models aligned with business needs.
Decisions in the architecture phase greatly affect a project’s success. Engineers consider data pipeline efficiency, model serving, and system monitoring.
Ensuring Production Excellence
Transitioning AI models to production requires careful testing and validation to ensure consistent performance in real-world scenarios.
Once deployed, continuous monitoring is crucial. Engineers set up systems to track model performance, detect issues, and trigger alerts if metrics deviate from expectations.
The success of machine learning models in production depends heavily on the robustness of the underlying infrastructure and monitoring systems.
The role combines software engineering and machine learning expertise. ML engineers must keep up with evolving tools, frameworks, and best practices in both fields.
Data Scientists: Driving Insights with Gen AI
Data scientists have emerged as key players in the AI-driven world. Their ability to extract meaningful patterns from vast datasets is crucial for building and deploying generative AI systems that create everything from text to synthetic data.
At companies like Salesforce, data scientists working on Einstein meticulously structure and organize data streams to ensure their large language models produce reliable, high-quality outputs. This foundational work is essential for the success of sophisticated AI models.
These specialists use advanced analytics techniques to clean, prepare, and transform raw data into formats that generative AI can learn from effectively. Their work prevents the ‘Garbage In, Garbage Out’ principle, where poor quality data leads to unreliable AI outputs.
Data scientists are transforming how organizations derive value from their data through predictive analytics. By combining traditional statistical methods with generative AI capabilities, they create synthetic datasets for testing and develop more accurate forecasting models.
Beyond technical analysis, modern data scientists serve as translators between complex AI systems and business stakeholders. They craft data stories that help leaders understand patterns and trends, making AI-driven insights accessible and actionable.
Advances in AI make data management a high priority, while 92% say that the need for trustworthy data is higher than ever
The State of Data and Analytics Report 2023
Their impact spans industries, from healthcare, where they generate synthetic patient data for research, to finance, where they develop fraud detection models. Their expertise ensures that generative AI tools remain grounded in reliable information.
As organizations increasingly rely on generative AI for innovation, data scientists continue to evolve their techniques. They develop new methods for data augmentation, automated reporting, and knowledge extraction that will shape the next generation of AI applications.
Exploring the Role of Algorithm Engineers
Algorithm engineers form the backbone of generative AI development, crafting the complex computational systems that power today’s most advanced AI technologies. Their core responsibility involves designing and implementing efficient algorithms that balance performance with ethical considerations.
These specialists focus on creating algorithms that not only solve complex computational problems but also ensure compliance with evolving AI regulations and standards. A key part of their role involves implementing ethical AI development principles directly into the algorithmic design process.
Algorithm engineers must carefully consider transparency and fairness when developing AI systems. They work to eliminate potential biases in their algorithms while maintaining high performance standards. This delicate balance requires deep technical expertise combined with a thorough understanding of ethical implications.
These professionals implement safeguards and monitoring systems within their algorithms. This includes building in audit trails, establishing clear documentation processes, and creating mechanisms for human oversight of AI decision-making.
The role extends beyond pure technical implementation to include collaboration with ethics boards and compliance teams. Engineers must regularly participate in ethical reviews and adjust their algorithms based on feedback to ensure responsible AI development.
One of the most critical aspects of algorithm engineering involves testing and validation. Engineers must rigorously evaluate their algorithms not just for technical performance, but also for potential unintended consequences and ethical concerns.
As AI technology continues to evolve, algorithm engineers increasingly focus on explainability and interpretability. They develop methods to make complex AI decision-making processes more transparent and understandable to both technical and non-technical stakeholders.
The field also demands continuous learning and adaptation. Algorithm engineers must stay current with both technical advancements and emerging ethical frameworks to ensure their work meets the highest standards of responsible AI development.
It is essential to treat collected data as biased or incomplete until it has been tested and validated. Concrete measures must be taken to address this concern.
Ethics by Design for Artificial Intelligence, 2024
Success in this role requires a unique combination of technical expertise and ethical awareness. Engineers must consistently evaluate how their algorithms might impact different user groups and society as a whole.
Challenge | Description | Solution |
---|---|---|
Time Complexity | Time taken by an algorithm as a function of input size. | Design efficient algorithms to manage large datasets. |
Space Complexity | Amount of memory used by an algorithm. | Balance memory usage with performance. |
Scalability | Ability to handle increasing input sizes and data types. | Design algorithms that scale effectively. |
Stability | Maintaining relative order of equal input elements. | Ensure stability for data integrity. |
Adaptability | Ability to adjust to different situations and requirements. | Design flexible algorithms for broader applicability. |
Implementation Complexity | Balancing simplicity and effectiveness. | Strive for simple and effective algorithms. |
Trade-offs and Constraints | Decisions between time, space complexity, stability, and adaptability. | Understand problem constraints to guide decisions. |
Enhancing Development with SmythOS
SmythOS enhances AI development with its intuitive visual workflow builder, turning complex agent creation into a streamlined process akin to building with digital blocks. Its drag-and-drop interface removes coding barriers, allowing both technical and non-technical users to develop sophisticated AI solutions.
Central to SmythOS’s development environment is its comprehensive debugging toolkit, enabling technical teams to identify and resolve issues in real-time, significantly shortening development cycles from months to weeks. This visibility ensures AI systems operate reliably while maintaining enterprise-grade standards.
For organizations embarking on AI initiatives, SmythOS enables the creation of reusable components that can be shared across different projects and departments, reducing redundant development efforts and ensuring consistency in AI deployment throughout the enterprise.
The platform’s visual debugging environment offers unprecedented insight into AI agent behavior and performance. Teams can monitor, test, and refine their AI workflows in real-time, ensuring optimal functionality and reliability before deployment.
SmythOS transforms enterprise AI development by combining powerful capabilities with intuitive design. Its comprehensive toolkit empowers organizations to build, test, and deploy sophisticated AI solutions while maintaining full control over their systems.
Conclusion: Preparing for the Future of Generative AI Jobs
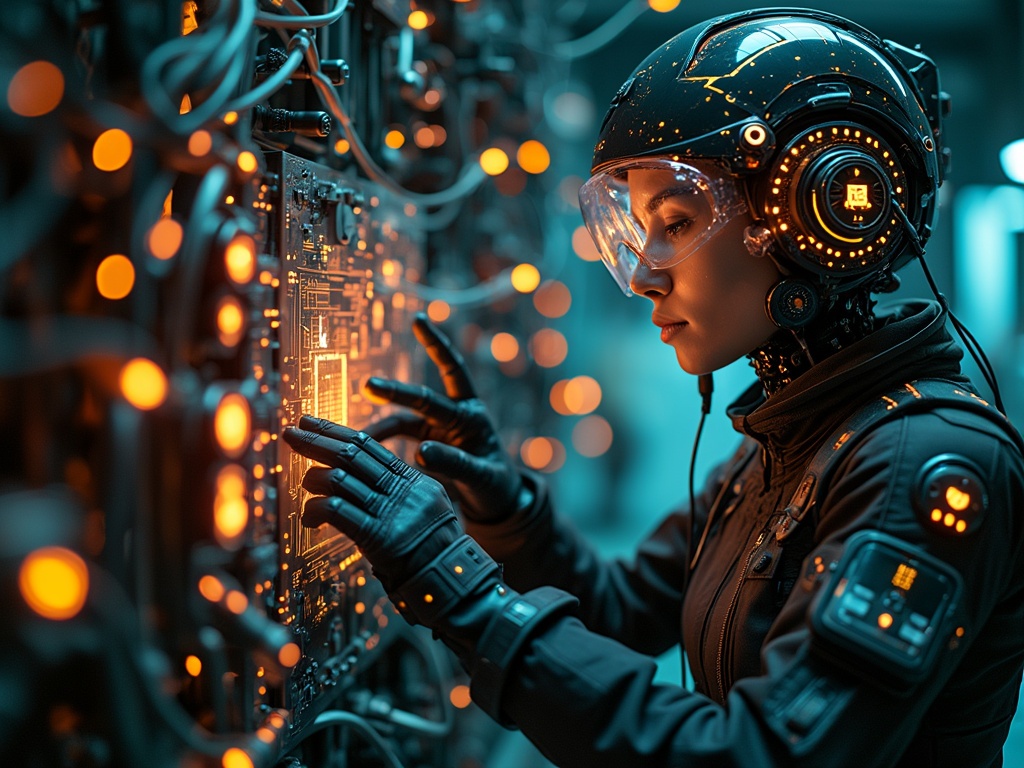
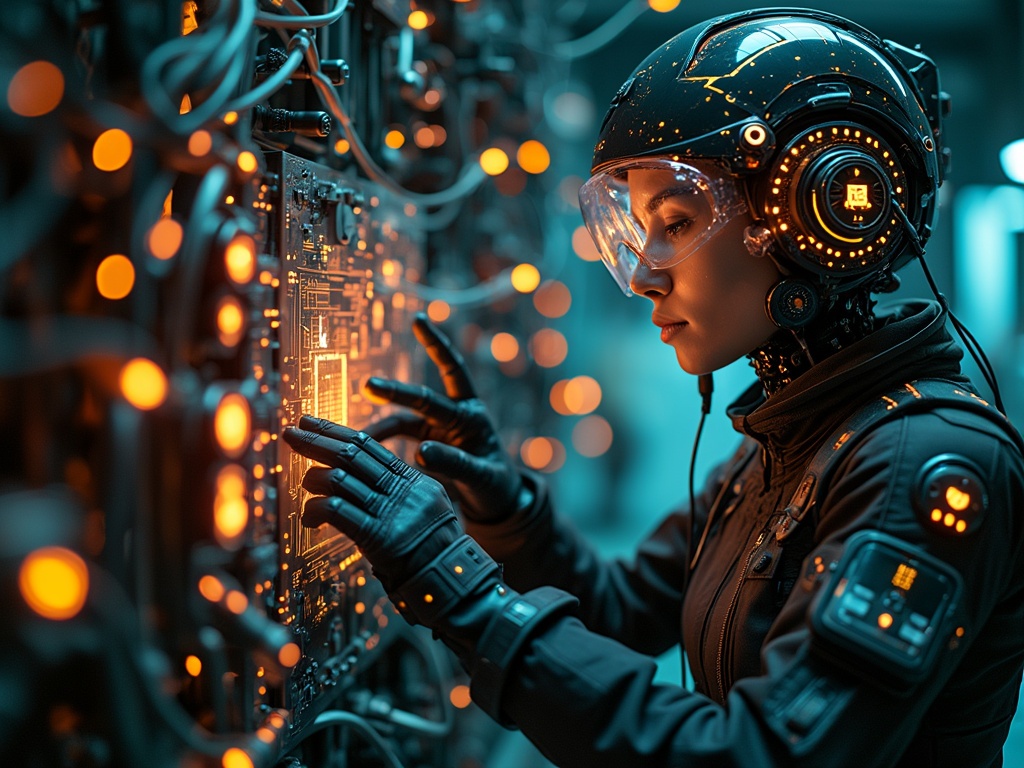
Generative AI is reshaping traditional roles and creating new opportunities.
Recent analysis from Brookings suggests over 30% of workers could see at least half their tasks disrupted by this technology, while 85% may experience some level of task transformation. This transformation is not following historical patterns of technological disruption. Instead of primarily affecting routine manual labor, generative AI is set to revolutionize knowledge work and creative professions, from law and marketing to healthcare and education.
Organizations must prioritize upskilling initiatives and embrace emerging tools like collaborative AI platforms that enhance rather than replace human capabilities. Successful transitions will likely come from businesses that view AI as a complement to human creativity and emotional intelligence. Worker preparation and engagement are essential components of this evolution.
Employees need to become active participants in shaping how AI transforms their roles. This includes developing both technical literacy and uniquely human skills that AI cannot replicate. The key to thriving in this AI-enhanced future lies not in resistance but in strategic adaptation. Organizations that invest in their workforce while thoughtfully implementing AI tools position themselves to capitalize on unprecedented opportunities for innovation and growth.
Last updated:
Disclaimer: The information presented in this article is for general informational purposes only and is provided as is. While we strive to keep the content up-to-date and accurate, we make no representations or warranties of any kind, express or implied, about the completeness, accuracy, reliability, suitability, or availability of the information contained in this article.
Any reliance you place on such information is strictly at your own risk. We reserve the right to make additions, deletions, or modifications to the contents of this article at any time without prior notice.
In no event will we be liable for any loss or damage including without limitation, indirect or consequential loss or damage, or any loss or damage whatsoever arising from loss of data, profits, or any other loss not specified herein arising out of, or in connection with, the use of this article.
Despite our best efforts, this article may contain oversights, errors, or omissions. If you notice any inaccuracies or have concerns about the content, please report them through our content feedback form. Your input helps us maintain the quality and reliability of our information.