Amazon AI: Innovations and Impact
Did you know that your favorite Alexa device is just the tip of Amazon’s artificial intelligence iceberg? Beyond the familiar voice assistant responding to millions of daily queries, Amazon has quietly transformed how we shop, interact with technology, and conduct business through its AI innovations.
Every time you browse Amazon’s store, AI works invisibly in the background—analyzing billions of interactions, predicting your preferences, and personalizing your experience. According to Amazon Science, the company’s AI systems are becoming increasingly self-aware and self-learning, capable of understanding complex customer intent and automating warehouse operations.
From empowering small businesses with AI-driven insights to transforming how we interact with smart devices, Amazon’s artificial intelligence innovations touch nearly every aspect of modern commerce and technology. The retail giant’s machine learning models now process vast amounts of data to predict shopping patterns, optimize supply chains, and help third-party sellers thrive on the platform.
This article pulls back the curtain on Amazon’s most impactful AI advancements—exploring how Alexa has evolved from a simple voice assistant into a sophisticated AI companion, examining the machine learning systems revolutionizing e-commerce, and revealing how Amazon’s latest generative AI tools are creating new possibilities for both sellers and shoppers. Discover how one company’s relentless AI innovation is reshaping the future of how we live, work, and shop.
The Evolution of Alexa
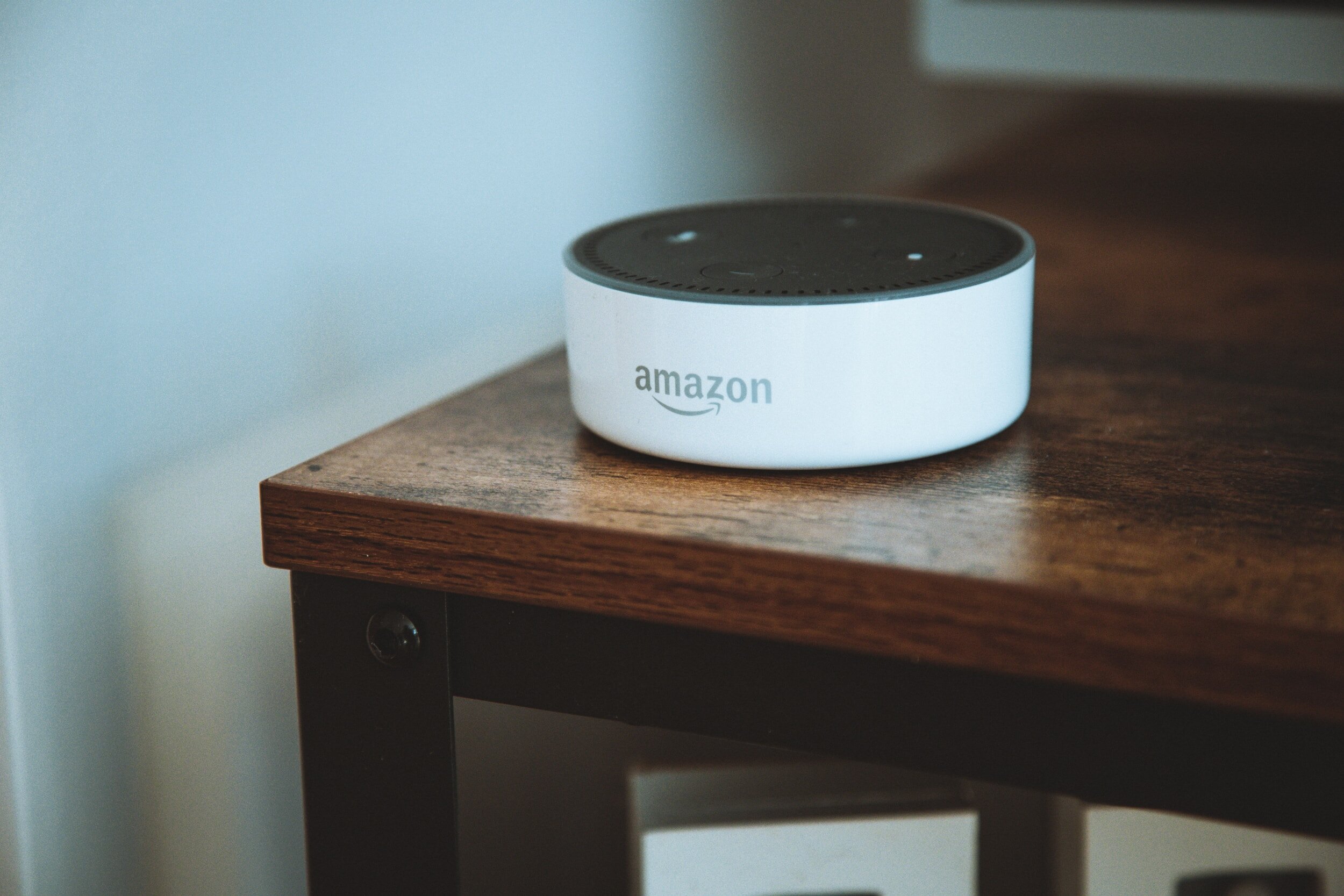
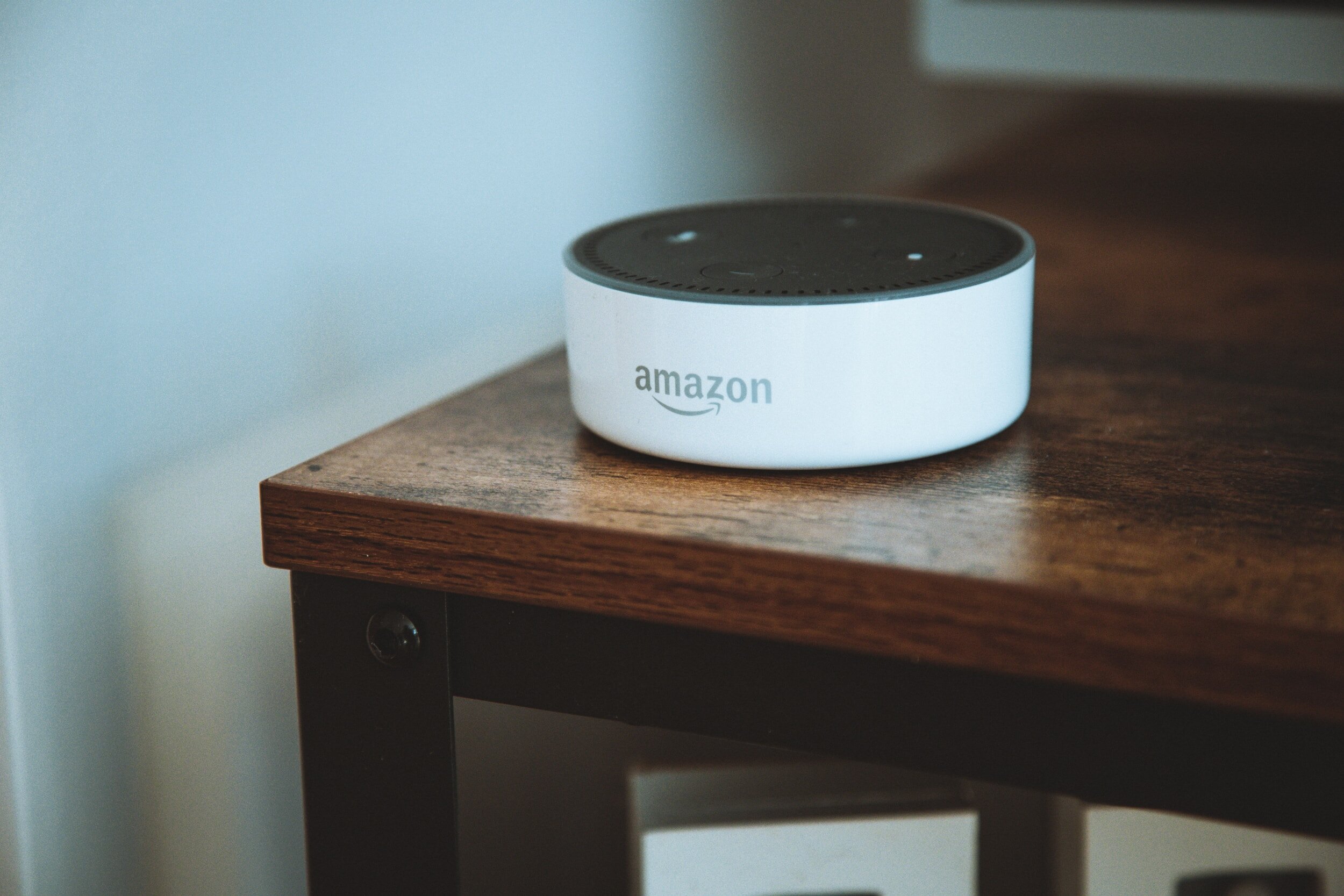
Ten years after its launch, Amazon’s Alexa has transformed from a simple voice assistant into one of the most ubiquitous AI platforms, now present in over 100 million homes across 400 million devices. While it began with basic functions like setting timers and playing music, Alexa’s journey reflects the broader evolution of voice-activated AI technology.
Early versions of Alexa focused primarily on fundamental tasks – controlling smart home devices, playing media, and responding to basic queries. As improvements in natural language understanding (NLU) emerged, the assistant gained the ability to interpret context and intent with greater accuracy, enabling more natural conversations.
Year | Milestone |
---|---|
2014 | Launch of Amazon Echo with Alexa |
2015 | Introduction of third-party skills and Alexa Skills Kit (ASK) |
2016 | Launch of Alexa Prize competition |
2017 | Introduction of Alexa for Business |
2018 | Launch of Alexa Hunches for smart home |
2019 | Introduction of self-learning capabilities |
2020 | Launch of Alexa Guard for home security |
2021 | Expansion of Alexa’s natural turn-taking abilities |
2022 | Development of Alexa’s voice mimicry feature |
The technological backbone supporting Alexa has undergone significant transformation. Modern iterations leverage advanced conversational AI to process multi-turn dialogues without losing context – a dramatic improvement over the rigid, command-based interactions of earlier versions. This evolution allows users to speak more naturally, as they would with another person.
Yet Alexa’s journey hasn’t been without challenges. Despite its widespread adoption, the platform initially struggled to expand beyond basic functionalities, with many users primarily employing it for simple tasks like timers and music control. Amazon’s recent integration of generative AI technology marks a pivotal shift, promising to unlock more sophisticated conversational capabilities and deeper understanding of user intent.
Looking ahead, Amazon is reimagining Alexa’s future through the lens of generative AI, aiming to deliver the kind of natural, contextual conversations that users have long desired. These advancements could finally bridge the gap between the assistant’s current capabilities and the seamless, human-like interactions that were part of its original vision.
We’ve always thought of Alexa as an evolving service, and we’ve been continuously improving it since the day we introduced it in 2014
Amazon
Machine Learning Models at Amazon
Amazon stands at the forefront of machine learning innovation, leveraging sophisticated algorithms to transform how millions of customers shop and receive their purchases. Amazon’s machine learning infrastructure processes vast amounts of data to make intelligent decisions at an unprecedented scale.
The company’s recommendation system is a cornerstone of its machine learning implementation. Using deep learning algorithms and collaborative filtering, Amazon analyzes historical purchase data, browsing patterns, and user preferences to generate personalized product suggestions. This system has proven remarkably effective, driving approximately 35% of total company sales through tailored recommendations.
In logistics, Amazon’s Supply Chain Optimization Technologies (SCOT) team has developed groundbreaking solutions that coordinate the movement of hundreds of millions of products daily. Their multi-echelon system, introduced in 2020, uses predictive analytics to optimize inventory levels across multiple fulfillment centers while accounting for varying delivery speeds and demand uncertainty.
The impact of these machine learning models extends beyond efficiency gains. In 2020 alone, Amazon’s AI-driven logistics systems generated $1.6 billion in transportation and logistics cost savings while reducing CO2 emissions by one million tons. These achievements demonstrate how machine learning can boost operational efficiency and environmental sustainability.
Another innovative application is the Packaging Decision Engine (PDE), an AI model that optimizes packaging decisions for millions of items daily. By analyzing product dimensions, fragility, and shipping requirements, this system has helped Amazon eliminate over two million tons of packaging material globally since 2015. The model exemplifies how machine learning can solve complex operational challenges while delivering tangible environmental benefits.
At SCOT, using science and technology to optimize the supply chain is not just an enabler, it’s our core focus.
Ashish Agiwal, Vice President, Fulfillment Optimization
Amazon’s machine learning models continue to evolve, with new applications emerging across its vast operations network. From forecasting demand patterns to optimizing delivery routes, these systems work together to create a more efficient and responsive retail ecosystem that benefits both the company and its customers.
Generative AI: Transforming Content Creation
Generative AI is transforming how businesses create and manage content at scale. By harnessing sophisticated machine learning algorithms and deep learning capabilities, these AI systems can now autonomously generate high-quality content, from product descriptions to personalized recommendations.
At the forefront of this change, generative AI tools have become increasingly versatile, enabling sellers to automate tasks like writing product listings and creating marketing copy. This automation speeds up the content creation process, maintains consistency across thousands of product pages, and reduces human error.
One of the most significant impacts has been in personalization. AI systems analyze vast amounts of customer data to generate tailored product recommendations, dynamic pricing suggestions, and customized marketing messages. These personalized experiences help customers find relevant products more quickly while increasing engagement and conversion rates for sellers.
Beyond basic automation, generative AI demonstrates remarkable capabilities in understanding context and maintaining brand voice. The technology can adapt its output to match specific tone guidelines, industry terminology, and marketing objectives. This flexibility allows businesses to scale their content operations without sacrificing quality or brand consistency.
The efficiency gains are particularly noteworthy. What once took content teams days or weeks to produce can now be generated in hours or minutes. This acceleration enables businesses to respond more quickly to market changes, launch new products faster, and maintain fresh, updated content across their digital presence.
Generative AI has the potential to become a general-purpose technology, transforming how we approach content creation and customer engagement across all industries.
Master of Code Global
However, successful implementation requires a balanced approach. While AI excels at generating initial drafts and handling repetitive content tasks, human oversight remains crucial for ensuring accuracy, maintaining brand voice, and adding creative nuances that resonate with target audiences. The most effective strategies combine AI’s efficiency with human expertise to deliver optimal results.
AI-Powered Seller Tools
Amazon is enhancing the seller experience with Project Amelia, an AI assistant that acts as a personalized business advisor for merchants. Built using Amazon Bedrock technology, this tool combines broad market knowledge with specialized Amazon selling expertise to deliver tailored insights and actionable recommendations.
Project Amelia excels in three areas that directly impact seller success. First, it functions as an always-available knowledge base, providing instant, accurate answers to specific questions about selling on Amazon. Whether preparing for the holiday season or navigating complex regulations, Amelia offers clear, reliable guidance drawn from authoritative sources.
The AI assistant’s second strength lies in its ability to deliver real-time business intelligence. Sellers can ask questions like “How is my business doing?” to receive comprehensive analyses of their sales performance, traffic metrics, and year-over-year comparisons. This data-driven approach enables merchants to make informed decisions quickly and identify growth opportunities.
Time Period | Metric | Value |
---|---|---|
Daily | Sales Revenue | $500,000 |
Daily | Website Traffic | 1,000,000 visits |
Weekly | Customer Retention Rate | 85% |
Monthly | Inventory Turnover | 12 times |
Yearly | Revenue Growth | 20% |
Perhaps most impressively, Project Amelia will soon introduce automated issue resolution capabilities. The system will diagnose problems, investigate discrepancies, and in many cases, take corrective action on behalf of sellers. For instance, if inventory numbers don’t align with shipment records, Amelia can investigate the disparity and coordinate with support teams to resolve the issue.
The practical impact of these AI tools extends beyond convenience. By automating routine tasks and providing strategic insights, sellers can redirect their time and resources toward core business activities like product development and customer service. Early beta users report significant improvements in operational efficiency and reduced time spent on administrative tasks.
While Project Amelia is currently available to select U.S. sellers, Amazon plans to expand access progressively, including international markets and multiple language support. This rollout strategy ensures the system can learn and evolve based on real-world interactions, continuously improving its ability to serve as a trusted advisor to the seller community.
For independent sellers, managing a successful global selling business can require navigating a number of complex and challenging areas. Project Amelia makes it easier than ever for sellers to manage and grow their businesses.
Mary Beth Westmoreland, Vice President, Worldwide Selling Partner Experience
Security and Ethical Considerations
As artificial intelligence reshapes enterprise technology, organizations must balance innovation with robust security measures and ethical guidelines. Amazon’s approach to AI security demonstrates how companies can implement safeguards while maximizing AI’s transformative potential.
At the foundation of secure AI implementation lies vulnerability management. According to AWS security documentation, organizations must protect three critical components: inputs, models, and outputs. This includes guarding against threats like prompt injection, data poisoning, and model inversion that could compromise system integrity.
Data protection takes center stage in ethical AI deployment. Companies must implement strict controls over sensitive information, ensuring data is encrypted both in transit and at rest. This includes careful consideration of data tokenization processes and implementing robust monitoring systems to detect potential breaches or unauthorized access patterns.
Human oversight remains crucial for responsible AI implementation. Organizations should maintain a careful balance between automation and human judgment, particularly in high-stakes decision-making scenarios. This might involve human-in-the-loop processes for critical decisions or human-over-the-loop approaches where humans retain ultimate authority.
It is important to remember that responsible AI is not the exclusive jurisdiction of a single entity or group; it is a collaborative effort that requires the active involvement and commitment of all stakeholders involved in the AI ecosystem.
AWS Enterprise Strategy Blog
Privacy considerations extend beyond basic data protection. Organizations must adhere to various regulations like GDPR and CCPA, conducting regular Privacy Impact Assessments (PIAs) to ensure compliance. This includes implementing mechanisms for data subject rights, such as the right to erasure and maintaining transparent data processing practices.
Regular security testing and evaluation form another crucial component of responsible AI deployment. This includes conducting threat modeling specific to AI systems, performing regular penetration testing, and maintaining comprehensive audit trails. Organizations should also establish clear incident response procedures for AI-related security events.
Conclusion: The Future of AI at Amazon
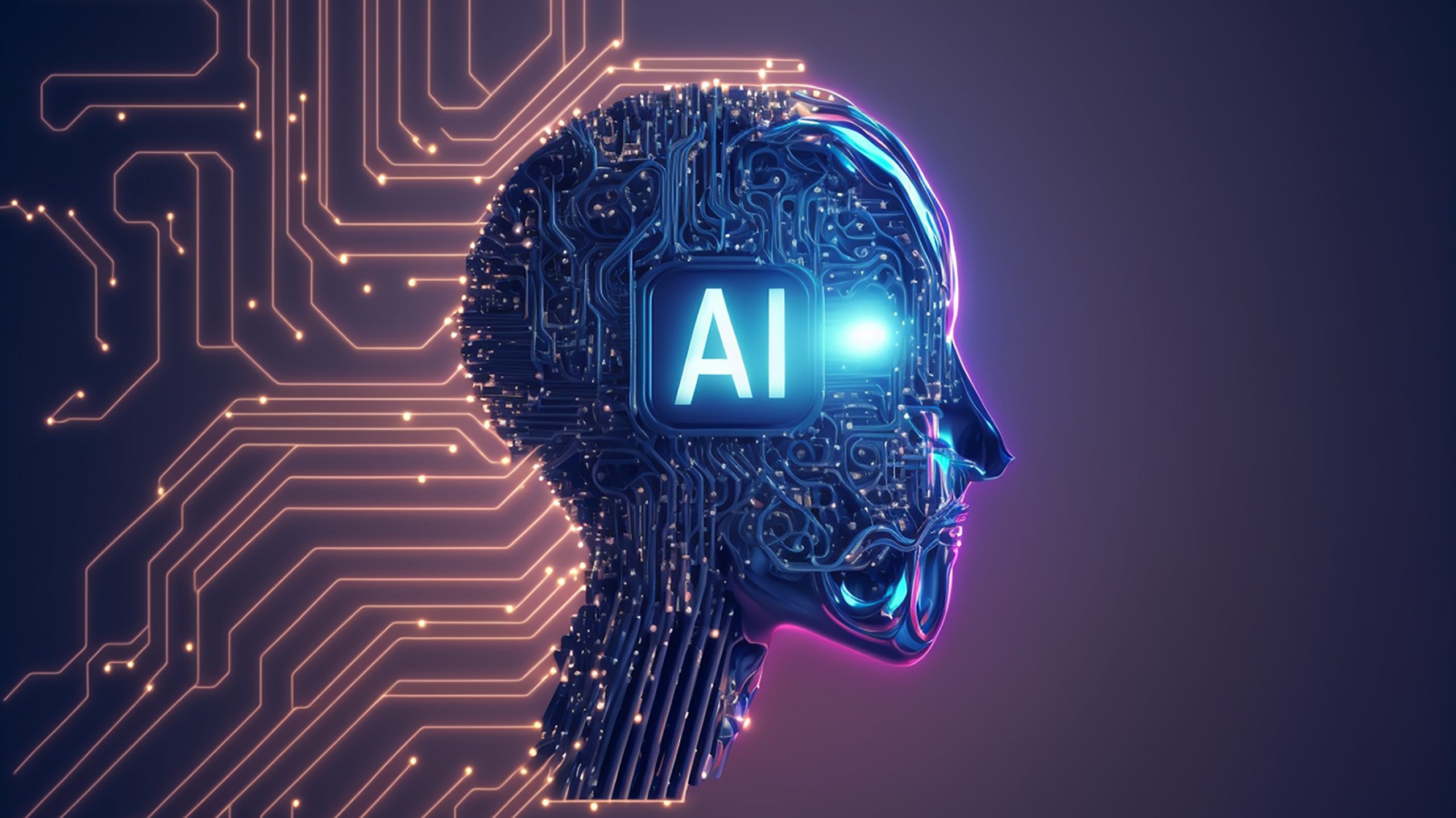
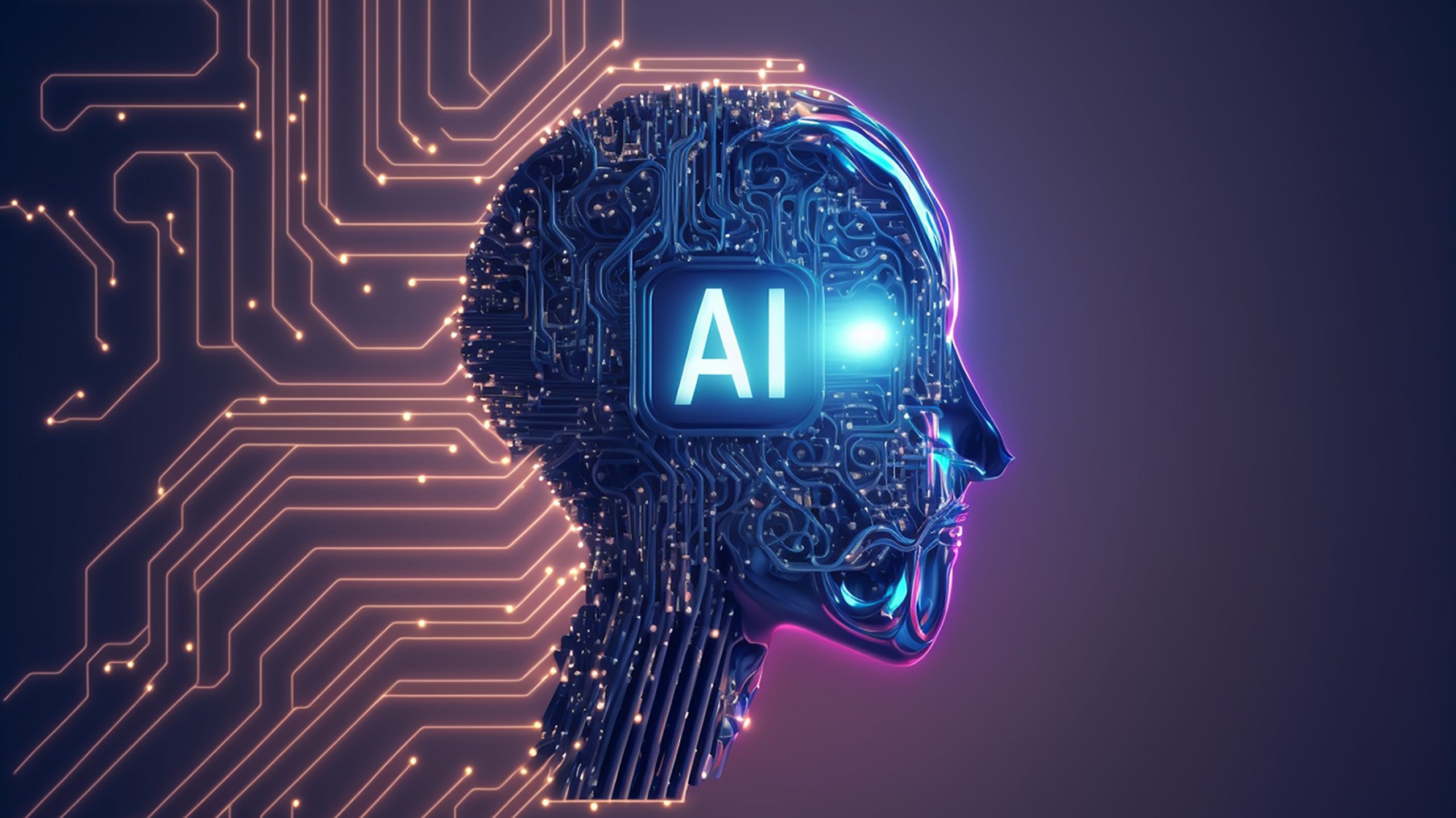
Digital head design featuring intricate circuits and letters. – Via marktechpost.com
Amazon’s relentless pursuit of AI innovation continues to reshape how we interact with technology. Through groundbreaking initiatives like their commitment to provide AI skills training to 2 million people by 2025, Amazon demonstrates its dedication to democratizing artificial intelligence while pushing technological boundaries.
The company’s vision goes well beyond its current accomplishments in personalized shopping and autonomous delivery systems. Their strategic investments in generative AI, robotics, and machine learning indicate a future where AI is increasingly integrated into daily commerce and consumer experiences. From AI-powered image generation for advertisers to intelligent inspection systems for delivery vans, Amazon’s innovations consistently bridge the gap between advanced technology and practical applications.
Looking ahead, Amazon’s AI roadmap suggests a future where technology becomes more seamless and less intrusive, yet more impactful. The integration of AI into services such as product recommendations, fraud detection, and supply chain optimization is expected to become more sophisticated, providing experiences that feel natural and intuitive while operating complex AI systems behind the scenes.
What makes this AI-driven future particularly exciting is its potential to democratize access to powerful tools and capabilities. Through platforms like SmythOS, businesses of all sizes can utilize similar AI capabilities, creating custom solutions with visual debugging tools and enterprise-grade security features. This democratization ensures that the benefits of AI advancements extend beyond major corporations, empowering smaller enterprises and developers.
As we stand at this technological frontier, one thing is clear: Amazon’s AI initiatives aim not only to improve business metrics but also to fundamentally transform how we live, work, and interact with technology. The future of AI at Amazon promises not just incremental improvements, but revolutionary changes that will continue to surprise and delight users while setting new standards for what is possible in the realm of artificial intelligence.
Last updated:
Disclaimer: The information presented in this article is for general informational purposes only and is provided as is. While we strive to keep the content up-to-date and accurate, we make no representations or warranties of any kind, express or implied, about the completeness, accuracy, reliability, suitability, or availability of the information contained in this article.
Any reliance you place on such information is strictly at your own risk. We reserve the right to make additions, deletions, or modifications to the contents of this article at any time without prior notice.
In no event will we be liable for any loss or damage including without limitation, indirect or consequential loss or damage, or any loss or damage whatsoever arising from loss of data, profits, or any other loss not specified herein arising out of, or in connection with, the use of this article.
Despite our best efforts, this article may contain oversights, errors, or omissions. If you notice any inaccuracies or have concerns about the content, please report them through our content feedback form. Your input helps us maintain the quality and reliability of our information.