Transforming Innovation with Generative AI
Imagine creating an entire symphony, writing a novel, or designing architectural blueprints with the help of artificial intelligence. This is no longer science fiction. Generative artificial intelligence (AI) has emerged as one of the most transformative technologies of our time, capable of creating original content by learning from vast amounts of existing data.
From generating human-like writing and stunning artwork to composing music and writing software code, these AI systems are reshaping creativity and productivity. Unlike traditional AI that analyzes or categorizes information, generative AI builds something entirely new, learning patterns from millions of examples to produce fresh, original content.
What makes this technology remarkable is its ability to understand context and nuance. When you interact with a generative AI system, you are engaging with a model that can grasp subtle meanings and generate contextually appropriate content. This breakthrough has profound implications for industries ranging from healthcare and drug discovery to creative arts and software development.
However, generative AI brings both extraordinary opportunities and significant challenges. Questions about accuracy, bias, copyright, and ethical use loom large as these systems become more sophisticated and widespread. How do we ensure AI-generated content remains truthful and authentic? What happens when AI can perfectly mimic human creativity?
As we enter this new era, one thing is clear: generative AI is not just another technological innovation. It represents a fundamental shift in how we create, innovate, and solve problems, promising to unlock human potential in ways we are only beginning to understand.
Understanding Generative AI Models
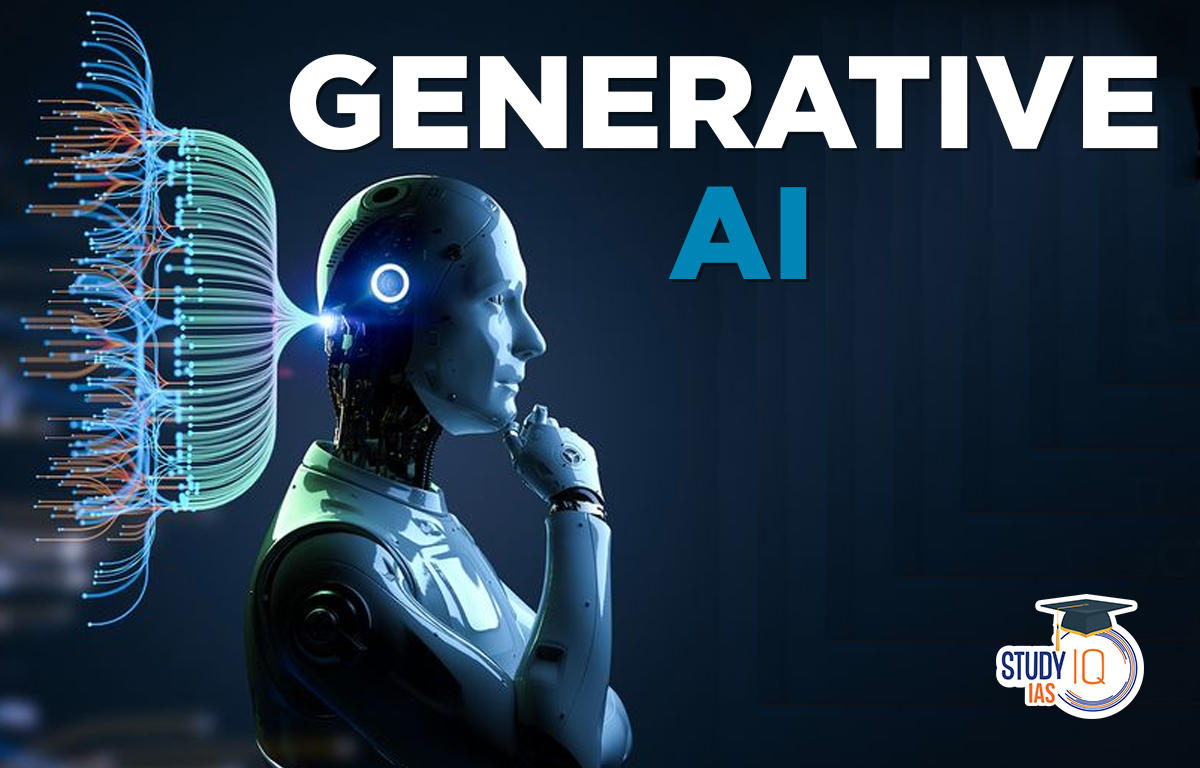
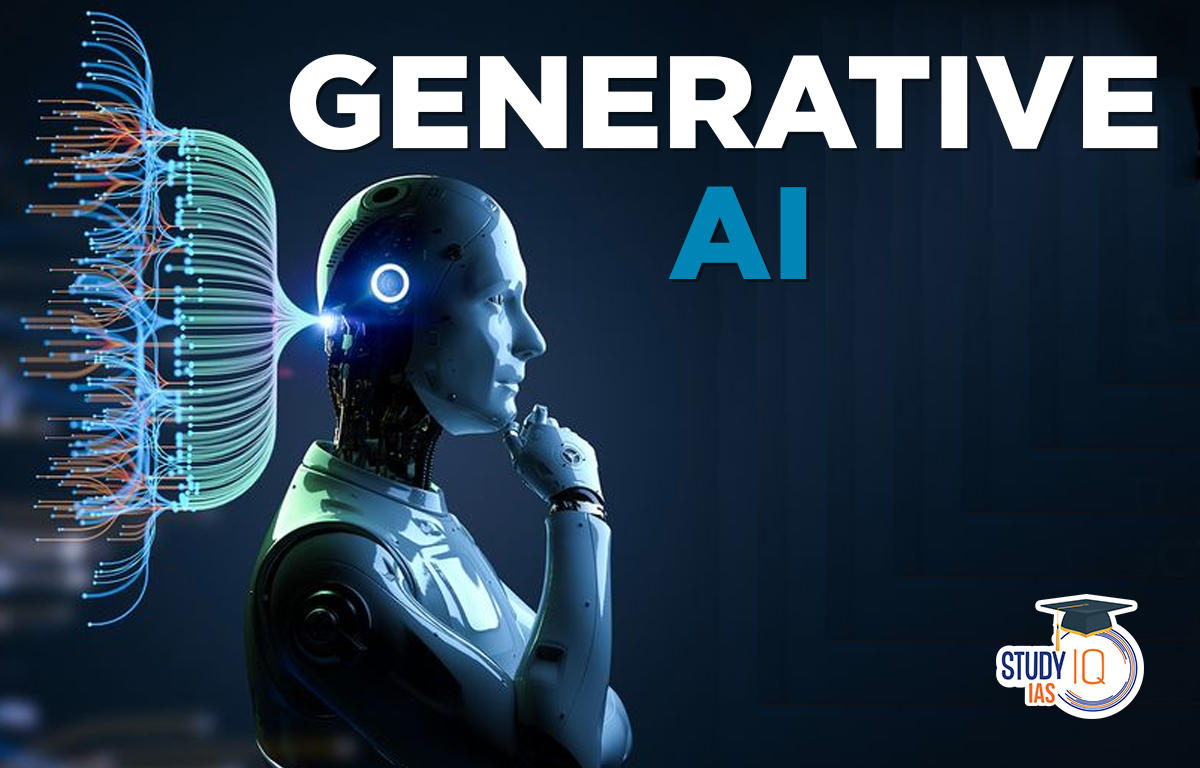
The AI landscape has fundamentally shifted with the emergence of sophisticated generative models that can create remarkably human-like content. These powerful systems – particularly GANs, VAEs, and transformers – represent distinct approaches to teaching machines how to generate new data.
At their core, Generative Adversarial Networks (GANs) operate like an artistic competition between two neural networks. The generator network attempts to create convincing content while the discriminator network acts as a critic, learning to distinguish real examples from generated ones. Through this back-and-forth process, GANs become remarkably adept at producing highly realistic images and other media.
Variational Autoencoders (VAEs) take a different approach by learning to compress and reconstruct data through an encoding-decoding process. While they may not match GANs’ impressive visual outputs, VAEs excel at capturing the underlying structure of data in a way that enables controlled generation of new examples. This makes them particularly valuable for tasks requiring precise manipulation of content characteristics.
Transformers have revolutionized natural language processing through their ability to understand context and relationships in sequential data. By employing a mechanism called self-attention, these models can generate coherent text while maintaining consistency across long passages. This capability has enabled breakthroughs in chatbots, translation, and other language-based applications.
The generator transforms random noise into artificial data, such as synthetic images, trying to match the distribution of the real data. This makes GANs highly specialized unsupervised learning models as they can generate highly realistic videos or images without requiring labelled training data.
Hyqoo AI Research
Each of these architectures has unique strengths – GANs for photorealistic imagery, VAEs for structured content generation, and transformers for natural language. Together, they form the foundation of modern generative AI, enabling machines to create increasingly sophisticated content across multiple domains. Understanding their individual capabilities and limitations is crucial for developers working to advance this rapidly evolving field.
Applications of Generative AI
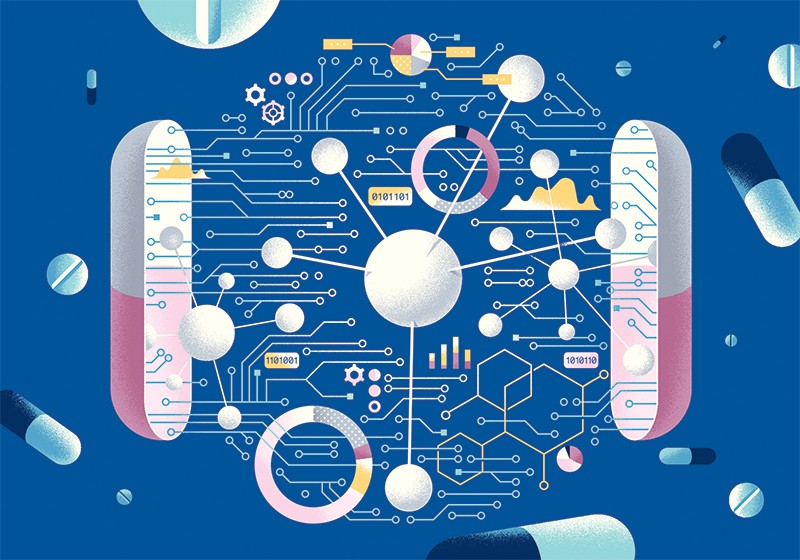
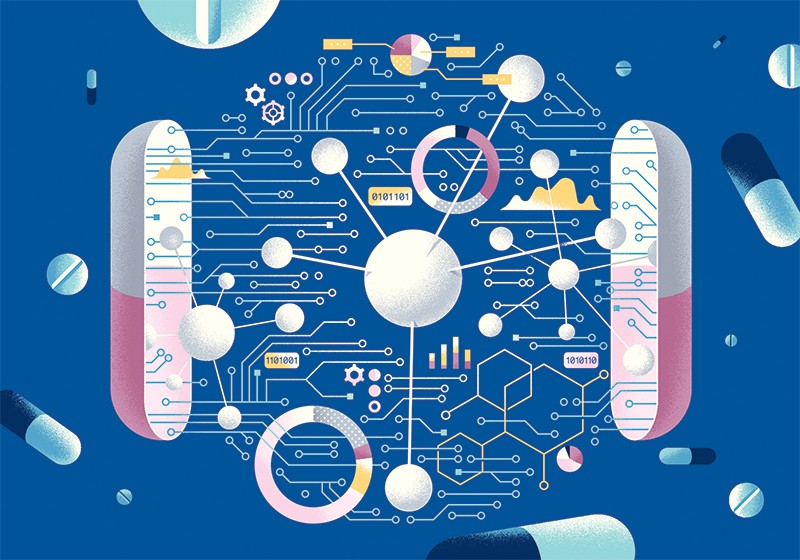
Exploring AI impact in pharmaceutical outcomes. – Via creative-biostructure.com
Generative AI has emerged as a transformative technology reshaping multiple industries through its ability to create original content and solve complex problems. From creating stunning artwork to accelerating drug discovery, its applications are diverse and impactful.
In the creative realm, generative AI enables artists and designers to explore new artistic possibilities. Tools like DALL-E and Midjourney produce original artworks, designs, and visual content based on text descriptions, helping artists enhance their creative processes. These systems learn from vast databases of artwork to generate new pieces that can serve as inspiration or finished products.
The software development industry has seen significant productivity gains through generative AI applications. Advanced AI systems can now generate functional code, accelerate debugging processes, and assist in application architecture design. Developers use these tools to automate routine coding tasks, allowing them to focus on more complex problem-solving and innovation.
In healthcare, generative AI is revolutionizing drug discovery and development. Rather than testing countless compounds manually, researchers use AI to generate and evaluate potential drug candidates rapidly. These systems analyze molecular structures and predict their properties, dramatically reducing the time and cost of bringing new medications to market. The technology also generates synthetic medical data for research while preserving patient privacy.
Entertainment and media industries leverage generative AI to create immersive experiences. Game developers use AI to generate realistic environments, character behaviors, and storylines. Content creators employ AI tools to produce and edit videos, create special effects, and generate personalized content for audiences.
Beyond content creation, generative AI proves invaluable in data augmentation across industries. It helps address data scarcity by creating synthetic datasets for training other AI models, crucial in fields like autonomous vehicles and medical imaging where real-world data may be limited or difficult to obtain.
Benefits and Challenges of Generative AI
Artificial intelligence is at a pivotal moment, particularly with its generative capabilities. Studies from MIT Sloan reveal that when properly implemented, generative AI can boost worker productivity by up to 40% compared to traditional methods. This improvement stems from AI’s ability to accelerate idea generation, draft persuasive content, and streamline complex workflows.
The advantages extend beyond efficiency gains. Harvard Business Review reports that generative AI excels at augmenting human creativity, helping overcome traditional innovation barriers by facilitating divergent thinking and supporting idea refinement. For knowledge workers, this means faster iteration cycles and enhanced ability to explore creative solutions.
However, the implementation of generative AI isn’t without significant hurdles. When used outside its capabilities, performance can drop by 19 percentage points – a reminder that AI isn’t a universal solution. Organizations must navigate what researchers call the “jagged technological frontier,” where AI’s abilities have clear but often invisible boundaries.
Ethical considerations pose another critical challenge. The technology’s potential to perpetuate existing biases or create new ones demands careful oversight. Security risks also loom large, particularly when AI systems handle sensitive data or make crucial decisions. These concerns actively shape how companies approach AI implementation.
The impact on the labor market is perhaps most pressing. While generative AI promises increased productivity, it also raises concerns about job displacement and the changing nature of work. This transformation requires organizations to focus on upskilling their workforce and creating new roles that leverage AI’s capabilities while maintaining human oversight.
Managers and workers need to collectively develop new expectations and work practices to ensure that any work done in collaboration with generative AI meets the values, goals, and standards of their key stakeholders
Kate Kellogg, MIT Sloan
Success with generative AI demands a balanced approach. Organizations must establish clear accountability frameworks, invest in proper training, and encourage role reconfiguration. The technology’s potential is immense, but realizing its benefits while mitigating risks requires thoughtful implementation and ongoing assessment of its impact on both productivity and ethical considerations.
Future Directions in Generative AI
Generative AI is poised for a transformative leap. Unlike basic text and image generators, next-generation AI systems are evolving into sophisticated agents capable of reasoning, collaborating, and making dynamic decisions. According to a recent RSM survey, 78% of executives already use AI in some capacity, signaling the technology’s growing maturity and adoption.
The dawn of AI agentification represents perhaps the most exciting frontier. These systems are becoming interconnected specialists that can autonomously handle complex tasks like supply chain management and predictive maintenance. This evolution promises to fundamentally reshape business operations, enabling unprecedented levels of automation and decision-making capabilities.
Personalized content generation is experiencing remarkable advances through improved contextual understanding. Future AI systems will move beyond simple customization to deliver deeply resonant, individualized experiences across industries. From healthcare providers generating tailored treatment plans to entertainment platforms creating custom content, AI will transform how we interact with and consume information.
Human-AI collaboration stands to become more intuitive and productive as these systems develop enhanced reasoning capabilities. Rather than replacing human creativity, AI will augment it, providing new tools for ideation, problem-solving, and innovation. This symbiotic relationship between human insight and machine capability represents a key focus area for ongoing research and development.
However, realizing this potential requires addressing significant challenges. Current research emphasizes improving AI’s reliability, reducing bias, and ensuring ethical deployment. As these systems become more autonomous, establishing proper governance frameworks and maintaining transparency in decision-making processes remains crucial.
The future of generative AI lies in fostering human-AI collaboration to push creative boundaries while addressing the technical and ethical challenges that come with increased autonomy.
Capgemini TechnoVision Report 2024
While obstacles remain, the trajectory is clear—generative AI is evolving from a set of isolated tools into an interconnected ecosystem that will transform how we work, create, and solve problems. Through continued research and responsible development, we stand to unlock unprecedented possibilities in human-AI cooperation and automated innovation.
Conclusion and SmythOS’s Role
Generative AI stands poised to transform industries, from healthcare diagnostics to creative content production. As organizations harness this technology, the challenge lies in responsible and effective implementation.
The potential impact is significant, with market projections approaching $970 billion by 2032. From automating routine tasks to enhancing human creativity, generative AI boosts operational efficiency and drives innovation. In healthcare, it revolutionizes diagnosis and treatment planning. In financial services, it transforms risk assessment and fraud detection. These applications represent just the beginning.
The key to unlocking this potential lies in having the right tools and infrastructure. Platforms like SmythOS play a crucial role, offering robust integration capabilities with over 300,000 apps, APIs, and data sources. By providing comprehensive debugging toolkits and visual workflow builders, such platforms reduce the complexity of implementing generative AI solutions.
The journey toward widespread AI adoption comes with challenges. Organizations must address data security, privacy, and ethical implementation. Success requires a balanced approach that prioritizes responsible development while maintaining the agility to innovate and adapt.
Looking to the future, organizations that thrive will effectively orchestrate multiple AI agents while maintaining enterprise-grade security and transparency. The transformation brought by generative AI is about reimagining how we work, create, and solve problems in an AI-enhanced world.
Last updated:
Disclaimer: The information presented in this article is for general informational purposes only and is provided as is. While we strive to keep the content up-to-date and accurate, we make no representations or warranties of any kind, express or implied, about the completeness, accuracy, reliability, suitability, or availability of the information contained in this article.
Any reliance you place on such information is strictly at your own risk. We reserve the right to make additions, deletions, or modifications to the contents of this article at any time without prior notice.
In no event will we be liable for any loss or damage including without limitation, indirect or consequential loss or damage, or any loss or damage whatsoever arising from loss of data, profits, or any other loss not specified herein arising out of, or in connection with, the use of this article.
Despite our best efforts, this article may contain oversights, errors, or omissions. If you notice any inaccuracies or have concerns about the content, please report them through our content feedback form. Your input helps us maintain the quality and reliability of our information.
We're working on creating new articles and expanding our coverage - new content coming soon!