Table of Contents
Introduction
Are you looking for the perfect AI development platform to bring your projects to life? With so many options available, it can be overwhelming to choose the right one. That’s why we’re here to help you compare two popular platforms: Adala vs LangChain.
In this article, we’ll dive into the features, capabilities, and target audiences of these platforms, so you can make an informed decision. Whether you’re a software developer, engineer, or data scientist, this comparison will provide valuable insights to enhance your AI development journey. Let’s explore Adala and LangChain and discover the perfect fit for your needs.
A Comprehensive Overview of Adala’s Offering
Adala’s unique features include everything from data labelling to text summarization, making it a versatile tool for a variety of tasks. Its agents have problem-solving capacities and are capable of interacting with users, particularly data scientists, through Python notebooks. This offers a user-friendly interface and effective communication protocols.
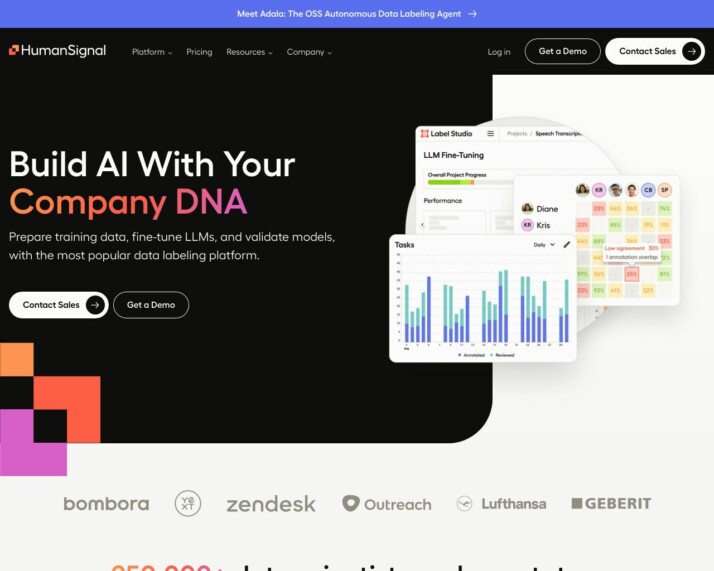
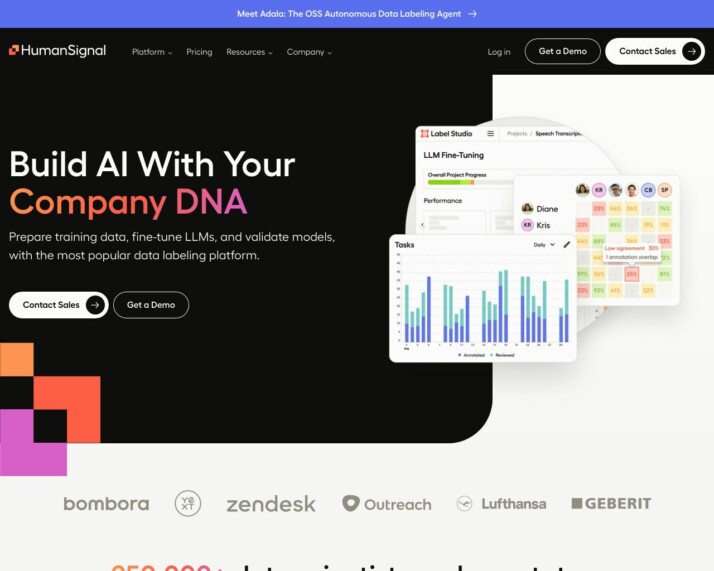
This Adala overview wouldn’t be complete without mentioning its target audience. The platform is designed for AI Engineers who want to build effective AI solutions without getting into the complexities of machine learning algorithms, and Machine Learning Researchers who want a testing ground for new techniques and methods.
Adala’s ability to process text-based data implies that it can support plain text file analysis and processing. However, there is currently no mention of support for image, audio, or video data processing.
Adala’s vision is to create reliable AI solutions that combine both AI and human input. It has a modular architecture that encourages contributions from the community, and its goal is to offer effective and cost-efficient data labelling solutions. Yet, there’s no mention of special security protocols like SSL/TLS for data, OAuth for third-party authentication, or IP-based access control.
An Overview of LangChain: Offering, Target Audience, and Vision
Welcome to our LangChain overview. LangChain is a tool that helps create smart computer programs, like chatbots. It came into the world as an open-source project in 2022. Open-source means anyone can use or change it.
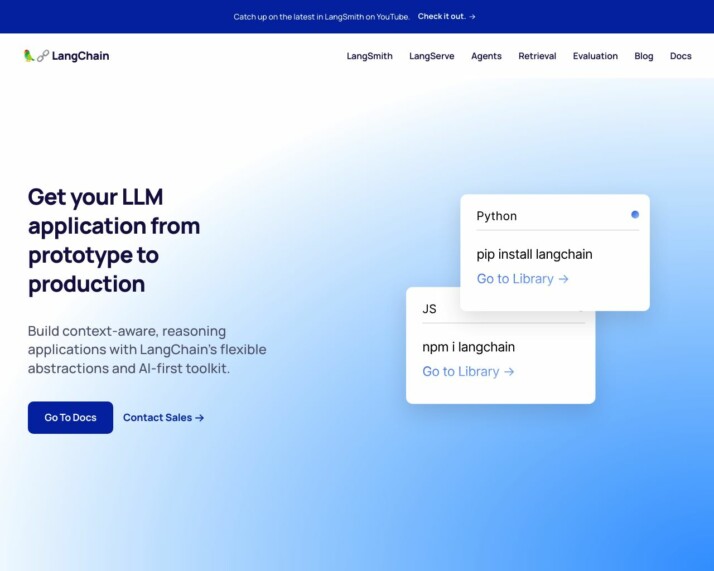
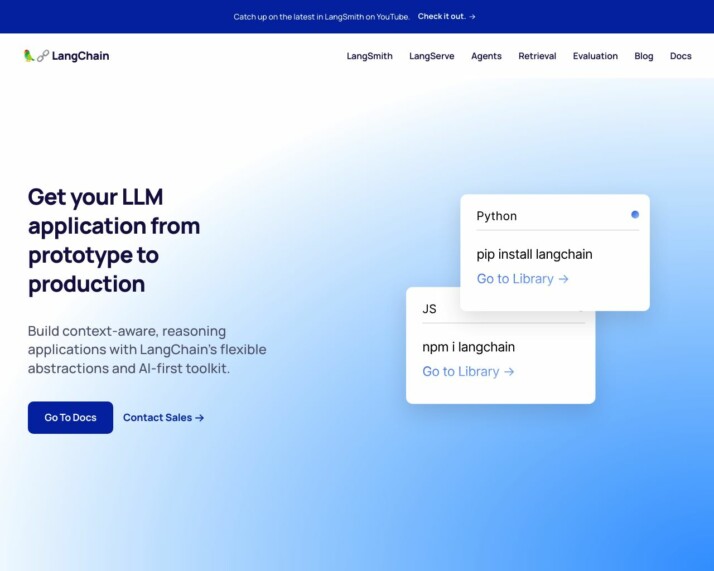
Now, let’s talk about LangChain’s offerings. Here’s what makes LangChain special:
- It can be used for different things – from building chatbots to analyzing data.
- Its design is like a box of Legos. You can use what’s already there or create something new based on your needs.
- It makes creating AI applications easier. AI or Artificial Intelligence is when a computer program can think and learn.
- It has several parts/modules for creating effective NLP applications. NLP or Natural Language Processing is when a computer program understands and responds to human language.
LangChain was made by Harrison Chase and Ankush Gola. They wanted to link LLMs (Large Language Models) with other data sources to create and make NLP applications better. Their mission is to make developing such applications easier and more efficient for programmers experienced in Python, JavaScript, or TypeScript.
LangChain’s target audience includes those who create computer programs and those who work with big data. Big data is a large amount of data that can be analyzed for patterns and trends.
One important point to note is that LangChain does not offer a cloud hosting option for its agents and does not offer distinct development and production environments for AI agent deployment. However, it supports memory capabilities and problem-solving capacities, and allows human-AI interaction, among other features.
In summary, LangChain stands out for its application development, libraries, and a vision focused on making the creation of AI and NLP applications simpler and more effective.
Talking about the LangChain vision, its end goal is to make it easy for experienced programmers to create and enhance AI-based applications. It aims to contribute to software development by offering a flexible and versatile platform.
Indeed, by doing so, LangChain is poised to make a significant impact in the world of AI and language-based applications. It gives developers the tools they need to succeed and continues to evolve according to their needs and the needs of the rapidly changing tech industry. Stay tuned to see what exciting LangChain shipped products will come next on this fascinating journey!
Finally, please note that while LangChain has several standout features, it does not provide visual builders, no-code editors, autonomous agents or multi-modal interactions. However, it indeed offers a logic-gate in workflows and deployment of AI agents as APIs, among other special features.
Feature Comparison: Adala vs LangChain vs SmythOS
This feature comparison provides an in-depth analysis of Adala, LangChain, and SmythOS, presenting head-to-head results of their capabilities, with a sharp focus on core competencies. We critically evaluate how these platforms perform across various metrics, highlighting elements that can contribute to ease of use and functionality.
Features | Adala | LangChain | SmythOS |
---|---|---|---|
Hosted Agents (Dev, Production) | ❌ | ❌ | ✅ |
Environments (Dev, Production) | ❌ | ❌ | ✅ |
Visual Builder | ❌ | ❌ | ✅ |
No-Code Editor | ❌ | ❌ | ✅ |
Memory & Context | ✅ | ✅ | ✅ |
Autonomous Agents | ❌ | ❌ | ✅ |
Explainability and Transparency | ❌ | ✅ | ✅ |
Debug Mode | ❌ | ✅ | ✅ |
Multimodal | ❌ | ❌ | ✅ |
Problem Solving Capabilities | ✅ | ✅ | ✅ |
In our comparison, it’s clear that there are differences in the features provided by Adala and LangChain. While both offer impressive Problem Solving Capabilities and a good hold on Memory & Context, LangChain stands out with additional features like Explainability and Transparency, and Debug Mode. Add to this, LangChain’s integration capabilities give it a strategic leverage, not found in the offerings of Adala. This can greatly impact the end user’s experience and could pose expanded possibilities for complex project needs.
Contrarily, Adala’s offers simplicity, which might appeal to users seeking straightforward, no-frills solutions. However, in a head-to-head competition, the resourcefulness of LangChain seems to outshine Adala. Both tools have distinct strengths, and the choice between Adala and LangChain would ultimately depend on the specific use case of the end user.
Adala vs LangChain: Understanding the Intended Audience
When it comes to the intended audience for Adala and LangChain, it’s important to understand who the end-users are and how these frameworks cater to their needs. Let’s explore the target audience and the features and applications that make these frameworks unique.
- Adala’s Target Audience: Adala is primarily designed for AI engineers and machine learning researchers. It provides a platform for building production-level AI agent systems, making it suitable for professionals who want to develop sophisticated AI solutions without diving deep into the complexities of machine learning algorithms.
- LangChain’s Target Audience: The intended audience for LangChain primarily includes software developers, software engineers, and data scientists experienced in programming languages such as Python, JavaScript, or TypeScript. These professionals are the primary end-users of the product as the features and applications of LangChain cater to their needs in developing advanced applications powered by large language models (LLMs).
The Features and Applications of Adala and LangChain
Let’s explore how the features and potential applications of Adala and LangChain align with the needs of their respective target audiences:
- Adala:
- Adala offers a platform for AI engineers to build production-level AI agent systems. Its main advantage is the abstraction of low-level machine learning tasks, making it easier for engineers to focus on developing sophisticated AI solutions without getting lost in complex algorithms.
- Machine learning researchers can benefit from Adala’s environment, which enables experimentation with complex problem decomposition and causal reasoning. This provides a solid base for testing and refining new methodologies and techniques in the field of AI and machine learning.
- LangChain:
- LangChain is renowned for its wide application range, making it suitable for a diverse range of applications such as retrieval-augmented generation, analyzing structured data, and building chatbots. This versatility demonstrates its capacity to cater to different AI-driven development needs.
- One of the key features of LangChain is its modular design. It offers composable tools and integrations that are easy to use and customize, making it accessible for developers to either use the existing chains or build new ones based on their specific requirements.
- LangChain simplifies the process of creating generative AI applications, particularly in the realm of natural language processing (NLP). It organizes large data volumes for easy access, which is crucial for advanced NLP applications.
- LangChain includes innovative modules for effective NLP apps, such as model interaction, data connection and retrieval, chains, and agents. These modules are designed to ensure the smooth operation and integration of multiple components or LLMs, necessary for effective NLP applications.
Conclusion
While Adala and LangChain have their own unique features and applications, SmythOS outshines them when it comes to its comprehensive and user-friendly approach.
SmythOS provides a seamless integration of AI capabilities, making it accessible to a wide range of users, including both developers and non-technical professionals.
Its intuitive interface allows for easy collaboration and efficient development of AI-driven solutions. With its robust support for various runtime configurations and advanced features, SmythOS is the preferred choice for those seeking a powerful and user-friendly AI framework.
Last updated:
Disclaimer: The information presented in this article is for general informational purposes only and is provided as is. While we strive to keep the content up-to-date and accurate, we make no representations or warranties of any kind, express or implied, about the completeness, accuracy, reliability, suitability, or availability of the information contained in this article.
Any reliance you place on such information is strictly at your own risk. We reserve the right to make additions, deletions, or modifications to the contents of this article at any time without prior notice.
In no event will we be liable for any loss or damage including without limitation, indirect or consequential loss or damage, or any loss or damage whatsoever arising from loss of data, profits, or any other loss not specified herein arising out of, or in connection with, the use of this article.
Despite our best efforts, this article may contain oversights, errors, or omissions. If you notice any inaccuracies or have concerns about the content, please report them through our content feedback form. Your input helps us maintain the quality and reliability of our information.
Alexander De Ridder
Co-Founder, Visionary, and CTO at SmythOS. Alexander crafts AI tools and solutions for enterprises and the web. He is a smart creative, a builder of amazing things. He loves to study “how” and “why” humans and AI make decisions.
Explore All Comparison Articles
Decisions vs. Sola: AI Workflow Automation Showdown
AI-powered workflow automation platforms revolutionize how businesses streamline operations and boost productivity. This comparison explores Decisions vs. Sola, and SmythOS,…
DeepOpinion vs. Sola: Comparing AI Automation Platforms
AI-powered automation platforms revolutionize business operations, but choosing the right solution can be challenging. This comparison of DeepOpinion vs. Sola,…
DevGPT vs. Sola: AI-Powered Development Tools Compared
AI-powered development tools revolutionize software creation, offering unprecedented efficiency and capabilities. This comprehensive review compares DevGPT vs. Sola, and SmythOS,…
Fine AI vs. Sola: Comparing AI Automation Tools
AI-powered automation transforms software development and business workflows, offering unprecedented efficiency and innovation. Fine AI vs. Sola present distinct approaches…
FlowiseAI vs. Sola: Comparing AI Automation Platforms
AI-powered automation revolutionizes business operations, driving efficiency and innovation across industries. FlowiseAI vs. Sola offer distinct approaches to harness this…
Gooey AI vs. Sola: AI-Powered Automation Platforms Compared
AI-powered automation platforms revolutionize how businesses streamline operations and enhance productivity. This comparison delves into Gooey AI vs. Sola, two…