When AI Was First Invented
Picture a warm summer day in 1956 at Dartmouth College, where a small group of visionaries gathered to explore an audacious question: Could machines actually think? This moment marked the official birth of Artificial Intelligence.
The journey began slightly earlier with British mathematician Alan Turing, who in 1950 posed a revolutionary question: “Can machines think?” His groundbreaking work laid the theoretical foundation for what would become one of humanity’s most transformative technologies.
At the historic Dartmouth Conference in 1956, computer scientist John McCarthy officially coined the term “Artificial Intelligence,” bringing together brilliant minds like Marvin Minsky, Norman Nash, and Claude Shannon to explore the fascinating possibilities of machine intelligence.
Every aspect of learning or any other feature of intelligence can in principle be so precisely described that a machine can be made to simulate it.
The Dartmouth Conference Proposal, 1956
In these early days, the pioneers believed they could crack the code of machine intelligence within a matter of years. While their timeline proved optimistic, their vision set in motion a technological revolution that continues to reshape our world in ways they could hardly have imagined.
Early Challenges in AI Development
Artificial intelligence’s journey began with optimism but soon faced challenges that shaped its evolution. Early AI systems from the 1950s and 1960s were limited to basic tasks due to fundamental constraints.
Computational power was a major hurdle. The era’s machines lacked the processing capabilities for complex AI operations, limiting researchers’ achievements. Even simple tasks like pattern recognition strained available hardware.
John McCarthy observed that early AI relied on rigid rules and symbolic logic, limiting systems’ ability to learn and adapt. This inflexibility made intuitive tasks challenging for AI.
The big mistake we made in artificial intelligence was in not appreciating the difficulty of the problems we were trying to solve.
Marvin Minsky
A critical obstacle was the scarcity of training data. Unlike today’s data-rich environment, early AI researchers had limited datasets, hindering effective learning and generalization.
Challenge | Description |
---|---|
Computational Power | Early machines lacked processing capabilities for complex AI operations, limiting achievements. |
Rule-Based Systems | AI systems were inflexible, relying heavily on rigid rules and symbolic logic. |
Data Scarcity | Limited access to large datasets hindered effective AI training and generalization. |
Human Cognition Complexity | Difficulty in replicating human-like reasoning and decision-making in machines. |
Algorithmic Limitations | Relied on basic rule-based approaches, failing in real-world problems. |
Hardware Constraints | Massive computational requirements exceeded available technology. |
The complexities of human cognition presented another challenge. Scientists struggled with replicating human-like reasoning and decision-making in machines. Early attempts at natural language processing and image recognition highlighted this gap.
These limitations created a gap between AI pioneers’ ambitious goals and technical feasibility. Simple tasks stretched early systems to their limits.
The lack of sophisticated algorithms hindered progress. Early AI’s rule-based approaches couldn’t handle real-world problem nuances, performing well in controlled environments but failing with unexpected situations.
Hardware constraints meant successful prototypes often couldn’t scale beyond labs. AI systems’ computational demands exceeded the era’s technology capabilities.
The AI Winter and Subsequent Revival
Artificial intelligence faced significant challenges in the late 20th century, known as the AI winter. The first occurred in the late 1960s and early 1970s when expectations exceeded the limits of available computing power and algorithms.
A second winter hit in the late 1980s when expert systems, despite initial excitement, proved inadequate for practical use. Many researchers avoided the term ‘AI,’ opting for ‘informatics’ or ‘machine learning’ to escape the negative connotations. Funding cuts led to the abandonment of numerous AI projects as disillusionment grew over its limitations.
However, by the mid-1990s, technological breakthroughs began to revive the field. Neural networks re-emerged with improved training techniques and enhanced computational capabilities. The internet provided access to large datasets, and machine learning algorithms advanced, particularly in pattern recognition and predictive analysis. These developments allowed AI to solve complex problems more accurately, such as logistics optimization and financial forecasting.
By the late 1990s, the AI winter ended as AI technologies began transforming industries, fueled by powerful hardware, refined algorithms, and big data.
Breakthrough | Description |
Machine Learning Algorithms | The rise of machine learning algorithms, such as decision trees and neural networks, allowed machines to learn from data and improve performance. |
Natural Language Processing (NLP) | Advancements in NLP enhanced machines’ ability to understand and generate human language, paving the way for applications like language translation and chatbots. |
Expert Systems | Widespread use of expert systems that emulated human decision-making, with applications in various industries like finance and healthcare. |
Neural Networks Resurgence | Improved training techniques and increased computational power led to a renaissance in neural network research. |
Internet Era | The rise of the internet provided unprecedented access to massive datasets, accelerating AI research and applications. |
AI’s Role in Modern Technology
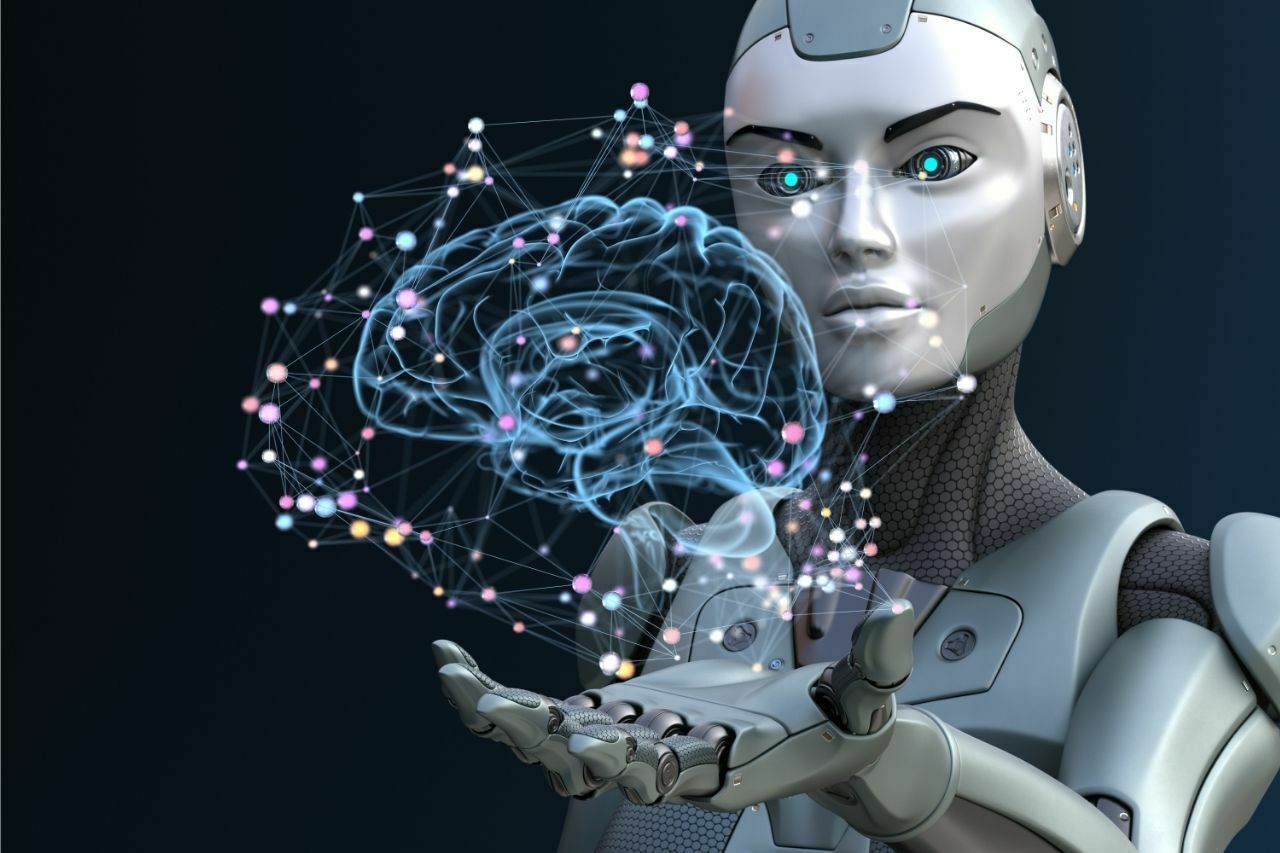
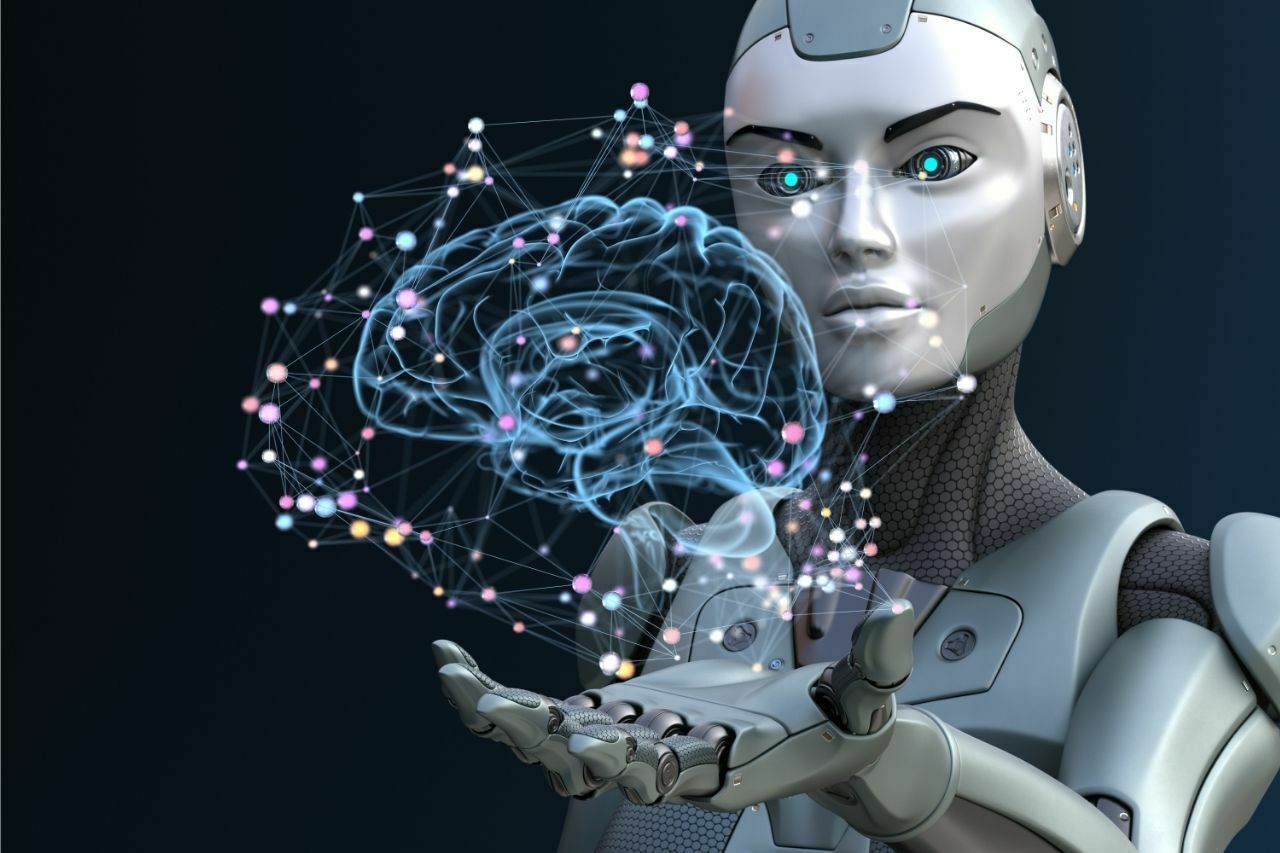
Artificial intelligence has transformed significantly due to innovations in deep learning and neural networks. These advances have enhanced AI’s capabilities beyond what was possible a decade ago.
Modern AI systems use sophisticated neural networks to process vast data, often trillions of data points, to recognize patterns and make decisions. According to Scientific American, deep learning has revolutionized how machines understand and interact with the world.
Virtual assistants like Siri and Alexa show AI’s growing sophistication in natural language processing. These systems now engage in natural conversations, schedule appointments, and control smart home devices accurately.
Deep learning has transformed computer vision applications. AI can analyze images and video streams in real-time, leading to breakthroughs in autonomous vehicles, medical diagnostics, and security systems.
Deep learning models now generalize knowledge across different domains. A single AI system can handle tasks from translating languages to generating creative content, showcasing unprecedented versatility.
Neural network capabilities have dramatically grown. What began as simple pattern recognition has evolved into systems capable of sophisticated reasoning and decision-making.
This evolution represents more than incremental improvements. It signals a fundamental shift in how machines process and understand information, opening new possibilities for human-AI collaboration across industries.
The Future of AI Development
Standing at the frontier of artificial intelligence, the path ahead promises advances in enhancing human capabilities and transforming complex processes. Recent breakthroughs in AI research, as highlighted by the Pew Research Center, suggest AI will amplify human effectiveness while introducing new opportunities for automation and innovation.
The next wave of AI development focuses on sophisticated multimodal systems that can process diverse types of data simultaneously. These advances enable AI to better understand and respond to human needs, from healthcare diagnostics to personalized education delivery. While the journey ahead includes addressing challenges around ethics, privacy, and responsible deployment, emerging frameworks and platforms are laying the groundwork for trustworthy AI implementation. This infrastructure helps organizations build AI solutions that balance innovation with accountability.
The acceleration of AI capabilities brings both excitement and responsibility. As autonomous systems become more advanced, ensuring alignment with human values and societal needs remains paramount. The future demands thoughtful development approaches that maximize benefits while managing potential risks. As we look toward tomorrow’s AI landscape, one thing is clear: the technology’s potential to enhance and empower humanity is immense. With continued focus on responsible innovation and human-centric design, AI development promises to unlock new realms of possibility that we are only beginning to imagine.
Last updated:
Disclaimer: The information presented in this article is for general informational purposes only and is provided as is. While we strive to keep the content up-to-date and accurate, we make no representations or warranties of any kind, express or implied, about the completeness, accuracy, reliability, suitability, or availability of the information contained in this article.
Any reliance you place on such information is strictly at your own risk. We reserve the right to make additions, deletions, or modifications to the contents of this article at any time without prior notice.
In no event will we be liable for any loss or damage including without limitation, indirect or consequential loss or damage, or any loss or damage whatsoever arising from loss of data, profits, or any other loss not specified herein arising out of, or in connection with, the use of this article.
Despite our best efforts, this article may contain oversights, errors, or omissions. If you notice any inaccuracies or have concerns about the content, please report them through our content feedback form. Your input helps us maintain the quality and reliability of our information.