What is Computer Vision: Understanding the Technology Behind AI Vision Systems
Self-driving cars navigate roads and smartphones unlock with facial recognition thanks to computer vision – a field of artificial intelligence that gives machines the ability to see and understand their surroundings.
Computer vision enables machines to process images and videos with superhuman speed and accuracy. These AI systems analyze visual data at massive scales, creating new possibilities across industries.
Medical diagnoses achieve pinpoint accuracy, smart cities manage traffic flow automatically, and robots navigate complex spaces with ease. Using artificial intelligence and deep learning, computer vision systems process visual information and make decisions instantly.
The technology enhances security through facial recognition and powers immersive augmented reality experiences. Computer vision represents more than technological advancement – it’s reshaping how we interact with machines and our environment.
This article explores computer vision’s core concepts, applications, and industry impact – from healthcare to entertainment. We’ll examine how this technology processes visual data to transform our world.
Computer vision is teaching machines to see the world as we do, but with the potential to perceive what we cannot. It’s not just about mimicking human vision; it’s about extending it.
How Does Computer Vision Work
Computers gain the ability to see and understand images through computer vision technology. Here’s how this process works.
Step 1: Capturing Images
Image acquisition begins when cameras and sensors convert light into digital information. This process captures photos, videos, or 3D images that computers can process. Self-driving cars demonstrate this by continuously photographing their surroundings.
Step 2: Image Enhancement
The computer enhances each image through preprocessing. It adjusts brightness, removes blur, and modifies size to highlight important details. Security systems use this to clarify footage of faces and objects.
Step 3: Feature Detection
The computer identifies key visual elements through feature extraction. It searches for distinct shapes, colors, and patterns – similar to spotting a cat by its ears, whiskers, and tail.
Step 4: Image Understanding
Algorithms called convolutional neural networks (CNNs) analyze the processed images. These networks learn from millions of examples to recognize objects and patterns. Factory systems use CNNs to identify defective products on assembly lines.
The Complete Process
These steps combine to enable powerful applications. Computer vision helps doctors detect diseases, guides robots through spaces, and verifies identities. As algorithms and computing power advance, its capabilities continue to grow.
Computer vision gives machines the power to see the world through our eyes, enhanced by digital precision.Dr. Fei-Fei Li, AI researcher
Step | Description |
---|---|
Image Acquisition | Capturing images or videos using cameras, scanners, or sensors. |
Preprocessing | Enhancing image quality through techniques like noise reduction and contrast enhancement. |
Feature Extraction | Identifying and isolating important features within the image. |
Interpretation and Analysis | Applying algorithms to classify objects, detect anomalies, and recognize patterns. |
Model Training | Training models using labeled data to learn and make predictions. |
Model Evaluation | Assessing model performance and fine-tuning for accuracy. |
Model Testing | Verifying model performance on unseen data. |
Model Deployment | Making the model available for use in a production environment. |
Monitoring and Maintenance | Continuously tracking model performance and retraining with updated data as needed. |
Key Applications of Computer Vision
Computer vision solves complex problems across industries through AI-powered image analysis. Here are four key applications transforming our world:
Healthcare Diagnostics
Medical imaging algorithms now detect diseases with remarkable precision. AI systems analyze X-rays, MRIs, and CT scans to spot early signs of cancer and other conditions. Studies show computer vision achieves 99% diagnostic accuracy, enabling timely interventions that save lives.
Advanced algorithms also detect retinal diseases and eye abnormalities with high precision, making eye care more accurate and less invasive.
Autonomous Vehicles
Self-driving cars use computer vision to navigate safely. Their cameras, LiDAR, and radar sensors collect environmental data while algorithms process it in real-time to identify objects and hazards.
The technology recognizes and tracks pedestrians, cyclists, and vehicles on the road. This capability significantly reduces accident risks.
Retail Analytics
Smart cameras analyze shopping patterns and optimize store layouts. Facial recognition helps identify customers for personalized service, while shelf-monitoring systems track inventory levels automatically.
These tools enhance both customer experience and operational efficiency in retail stores.
Security Systems
Advanced surveillance systems use computer vision to monitor spaces and detect unusual activity. The technology enables facial recognition at airports and helps prevent crime in smart cities.
Biometric authentication methods are becoming more sophisticated, improving security across physical and digital spaces.
The applications of computer vision grow as the technology evolves, creating new solutions for business and society.
From healthcare advances to safer transportation, computer vision makes our world smarter and more efficient. The technology continues to expand its capabilities, promising even greater benefits ahead.
Challenges and Risks in Computer Vision
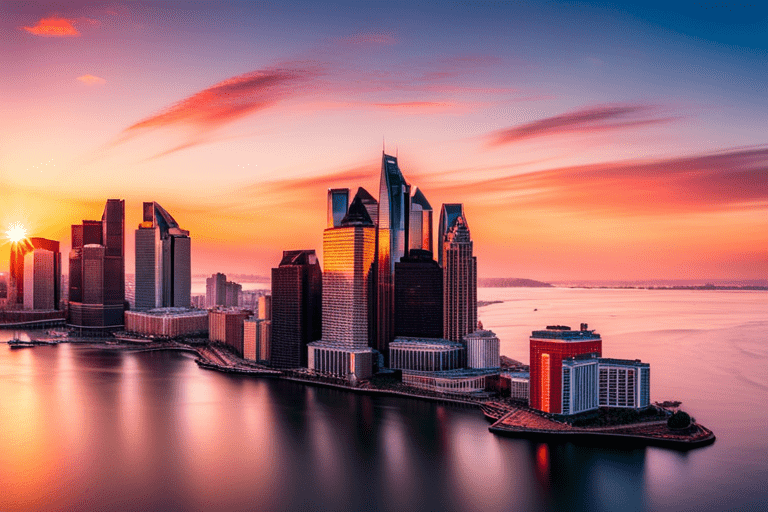
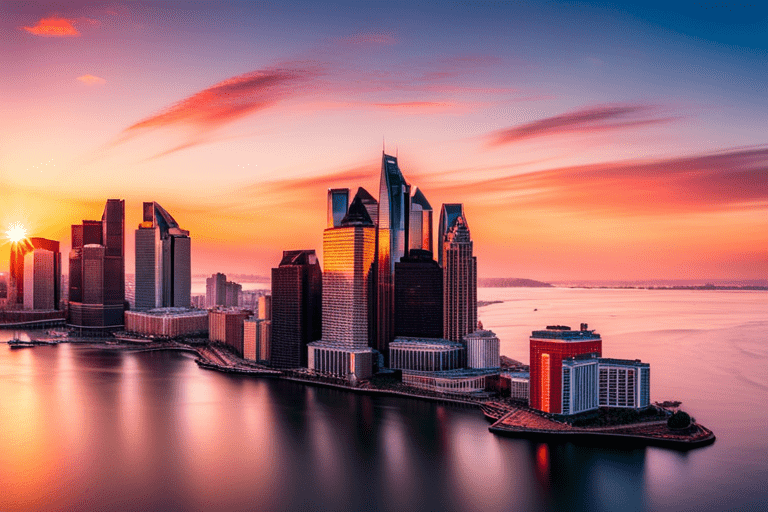
Modern skyscrapers reflect a beautiful sunset. – Via b-cdn.net
Despite significant advances, computer vision faces key challenges that affect its effectiveness and ethical implementation. These hurdles require careful consideration as the technology becomes more integrated into daily life.
Privacy Concerns
Data privacy stands at the forefront of computer vision challenges. These systems capture and analyze visual information containing sensitive personal data, raising crucial questions about consent and protection.
Facial recognition in public spaces exemplifies this concern. The technology tracks individuals without explicit permission, sparking debates about surveillance and freedom across many nations.
Companies must navigate strict privacy regulations while developing computer vision systems. Success depends on balancing technological advancement with personal privacy protection.
Training Data Bias
Bias in training data poses a significant challenge. The quality and diversity of data directly impact system performance and fairness.
Facial recognition systems often show reduced accuracy with darker skin tones and certain ethnic groups. This technical limitation creates ethical problems and reinforces social inequities.
Creating representative datasets and conducting regular bias audits helps address these issues. Regular testing ensures systems remain fair and accurate across all demographics.
Technical Limitations
Machines struggle to match human ability in processing complex visual information. Understanding partially hidden objects or unclear scenes remains challenging for computer vision systems.
Researchers develop advanced techniques to improve scene interpretation, but achieving human-level understanding requires significant advancement.
Ethical Framework
Computer vision’s growing capabilities demand strong ethical guidelines. While the technology enables medical breakthroughs, it also facilitates surveillance and deepfake creation.
Building ethical frameworks requires input from diverse stakeholders. Technologists, ethicists, policymakers, and the public must collaborate to ensure computer vision serves society’s best interests.
Future Trends in Computer Vision
Deep learning advances and growing computational power are transforming computer vision. These developments open new possibilities for how machines process and understand visual information.
Visual recognition accuracy continues to improve through innovative algorithms. Recent breakthroughs allow systems to detect subtle emotional expressions in faces, transforming mental health assessment and customer analytics. This precision enables applications that were previously impossible.
Computer vision models now understand and reason about what they see. This capability allows autonomous vehicles to anticipate pedestrian movements and medical systems to spot early disease indicators that could save lives.
New Industries Adopting Computer Vision
AI-powered drones use computer vision to revolutionize farming – detecting crop diseases and optimizing irrigation. Retail stores create checkout-free shopping experiences that change how customers buy products.
The combination of computer vision with augmented reality creates powerful new tools. Technicians can repair complex equipment guided by AR overlays that analyze machinery in real-time.
The future of computer vision is not just about seeing – it’s about understanding, predicting, and enhancing human capabilities in ways we’ve only begun to imagine.
Computer vision applications continue expanding – from enhanced public safety systems to immersive entertainment experiences. However, this technology must develop responsibly.
Privacy, ethics and bias remain key challenges as computer vision becomes more widespread. Success requires balancing innovation with societal values and needs.
Computer vision brings us closer to a world where machines can truly understand visual information. Our commitment to responsible development will help realize this technology’s full potential while protecting individual rights and promoting fairness.
Conclusion: The Impact of Computer Vision
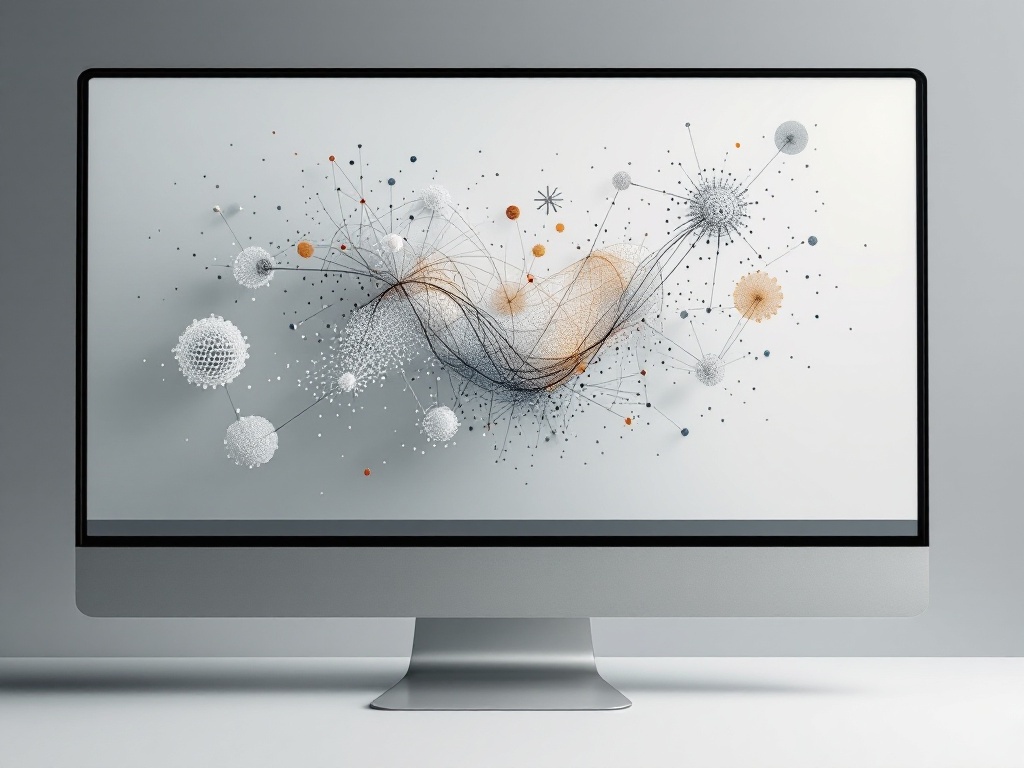
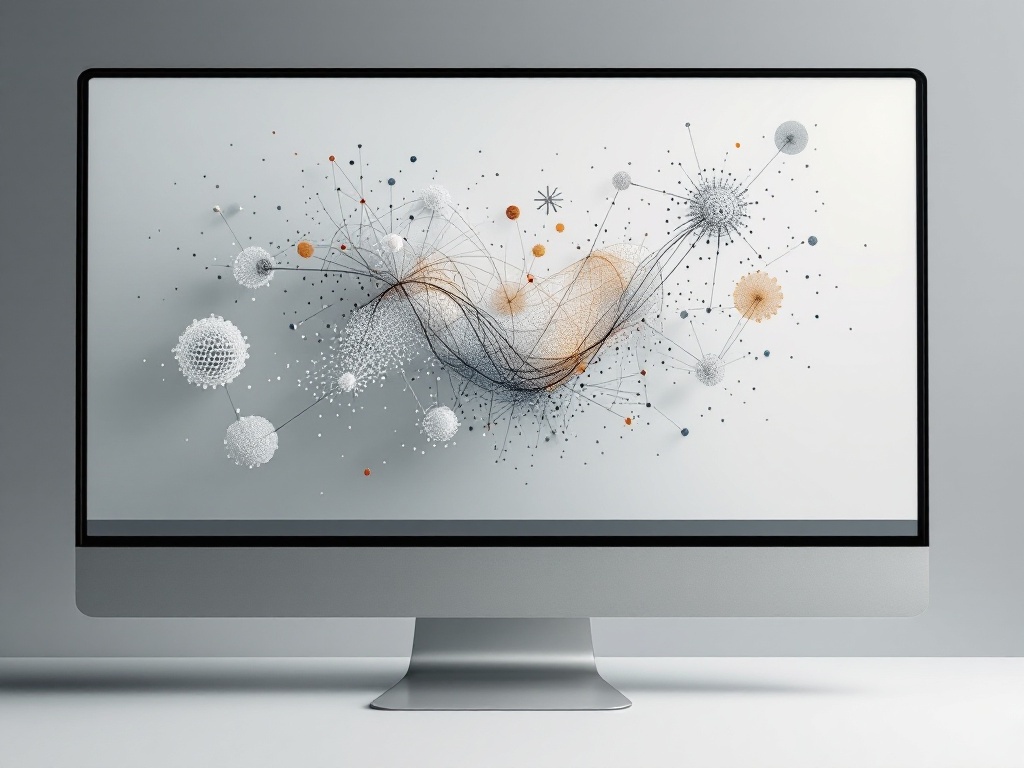
A dynamic visual display of ideas and connections. – Via smythos.com
Computer vision transforms how machines interpret and analyze visual data, bringing innovation to industries from public safety to retail. This technology drives efficiency gains and creates new possibilities for automation and analysis.
AI-powered systems match human experts in medical imaging diagnostics and enable real-time quality control in manufacturing. These advances streamline complex tasks and improve decision-making across sectors. The technology helps doctors detect conditions with high accuracy while allowing manufacturers to spot defects instantly.
Deep learning models and neural networks enhance computer vision’s capabilities daily. Machines now process visual information with increasing speed and precision, enabling advances in autonomous vehicles, smart cities, and personalized services. These improvements create opportunities for safer transportation and more efficient urban planning.
SmythOS provides the tools needed to build and deploy computer vision applications effectively. Their development platform helps organizations implement AI-driven visual intelligence solutions with integrated tools and seamless deployment options.
Computer vision’s impact extends beyond technical advancement. The technology changes how we interact with machines and understand our environment. Its applications continue to expand, promising ongoing benefits for business operations and daily life.
Last updated:
Disclaimer: The information presented in this article is for general informational purposes only and is provided as is. While we strive to keep the content up-to-date and accurate, we make no representations or warranties of any kind, express or implied, about the completeness, accuracy, reliability, suitability, or availability of the information contained in this article.
Any reliance you place on such information is strictly at your own risk. We reserve the right to make additions, deletions, or modifications to the contents of this article at any time without prior notice.
In no event will we be liable for any loss or damage including without limitation, indirect or consequential loss or damage, or any loss or damage whatsoever arising from loss of data, profits, or any other loss not specified herein arising out of, or in connection with, the use of this article.
Despite our best efforts, this article may contain oversights, errors, or omissions. If you notice any inaccuracies or have concerns about the content, please report them through our content feedback form. Your input helps us maintain the quality and reliability of our information.