Generative AI Solutions: Transforming Industries
Machines now create, innovate, and solve complex problems alongside humans. Generative AI solutions have transformed business operations across healthcare, finance, and manufacturing sectors.
Generative AI produces new content – text, images, music, and product designs – while developing novel ideas and solutions independently. A recent study projects its economic impact at $2.6-4.4 trillion annually, equivalent to the UK’s entire economy.
Across industries, generative AI delivers tangible benefits:
- Healthcare: Accelerating drug discovery and personalizing treatment plans
- Manufacturing: Designing efficient products and predicting equipment failures
- Marketing: Creating personalized content at scale
- Finance: Developing smarter investment strategies
Beyond financial gains, generative AI enhances business efficiency. Product design cycles have halved, while AI assistants provide instant customer support.
Successful implementation requires a measured approach: start with specific problems, validate solutions, and maintain human oversight throughout the process.
The following sections explore how generative AI transforms industries and provides strategic guidance for businesses seeking competitive advantage through AI adoption.
Applications of Generative AI in Healthcare
Generative AI advances healthcare through three key innovations: accelerated drug discovery, enhanced medical imaging, and personalized treatments. These breakthroughs improve patient outcomes and streamline healthcare delivery with unprecedented efficiency.
AI models analyze vast molecular datasets to predict compound interactions with biological targets, transforming drug discovery. Insilico Medicine exemplifies this advancement, using AI platforms to design targeted drugs for cancer and fibrosis treatment, significantly reducing traditional development timelines.
AI algorithms enhance diagnostic precision by analyzing MRI and CT scans to detect abnormalities human observers might miss. Advanced AI tools reduce image noise for clearer scans, improving diagnostic accuracy while minimizing patient discomfort and radiation exposure.
AI systems analyze genetic profiles, medical histories, and lifestyle factors to create personalized treatment strategies. Patients with chronic conditions receive care plans optimized for their specific needs, leading to better treatment outcomes and fewer side effects.
Benefit | Description |
---|---|
Tailored Treatment for Individual Needs | Personalized treatment plans are customized to fit an individual’s unique medical history, lifestyle factors, and health objectives, ensuring more effective and safer outcomes. |
Prevention and Early Detection of Diseases | These plans prioritize preventative healthcare, focusing on identifying potential risks and taking proactive steps to prevent illnesses before they occur. |
Improved Patient Engagement and Satisfaction | Patients are more likely to be engaged and satisfied with their healthcare when actively involved in their treatment plans, fostering trust and confidence. |
Cost-Effective and Efficient Healthcare | By predicting which treatments will work best for specific patients, personalized treatment plans can avoid the trial and error often associated with traditional medicine, leading to more efficient and cost-effective care. |
AI streamlines healthcare operations by automating administrative tasks and optimizing workflows. AI-powered chatbots handle routine inquiries, schedule appointments, and assist with initial diagnoses, allowing healthcare professionals to focus on patient care.
Success in healthcare AI requires addressing data privacy and algorithmic bias challenges. Ethical implementation remains essential, prioritizing patient well-being and equitable care access. This technology promises more efficient, personalized, and effective healthcare delivery.
Optimizing Manufacturing with Generative AI
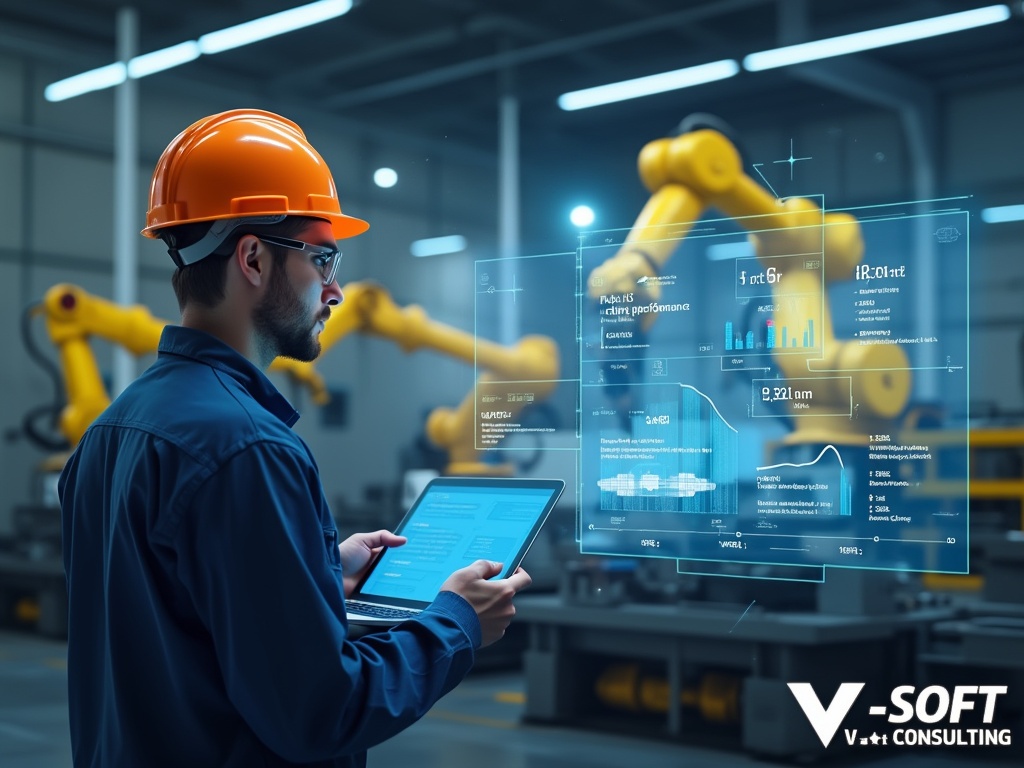
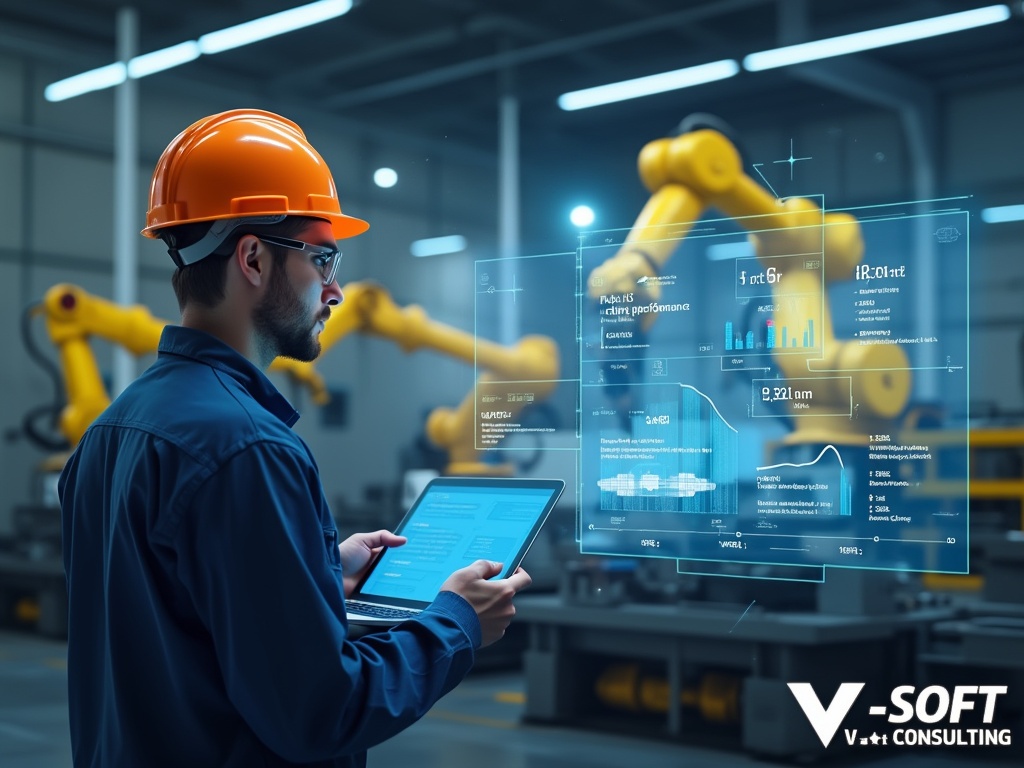
Generative AI enhances manufacturing processes and drives operational efficiency. This technology streamlines product design, predicts equipment failures, and delivers significant cost savings.
Advanced Product Design
Engineers harness AI to generate multiple design options by inputting specific parameters and constraints. Airbus demonstrates this capability, creating aircraft partition designs 45% lighter than conventional versions through AI optimization.
AI accelerates the design process while producing innovative solutions. The technology explores numerous iterations to create products with improved strength, reduced weight, and lower production costs.
Smart Predictive Maintenance
AI analyzes sensor data from manufacturing equipment to forecast potential failures before they occur. GE employs AI algorithms to detect patterns and predict equipment issues through sensor and historical data analysis. This enables optimal maintenance scheduling, minimizes downtime, and extends machinery lifespan.
Industry | Application | Benefits |
---|---|---|
Manufacturing | Analyzing sensor data from machines to predict failures | Reduces downtime, increases equipment lifespan |
Energy | Monitoring power plants, wind turbines, and electrical grids | Optimizes energy production, reduces blackout risks |
Healthcare | Ensuring continuous operation of medical equipment | Minimizes downtime, improves patient care |
Transportation | Predicting maintenance needs for trains, planes, and automobiles | Enhances safety, reduces operational costs |
Oil and Gas | Monitoring drilling equipment, pipelines, and refining processes | Ensures integrity, optimizes maintenance schedules |
Agriculture | Scheduling maintenance for farming equipment | Reduces downtime, increases crop yields |
Aviation | Predicting maintenance for aircraft engines and components | Reduces flight disruptions, ensures safety |
Telecommunications | Predicting and preventing network equipment failures | Ensures reliable connectivity |
Supply Chain Optimization
AI transforms supply chain management through enhanced visibility and control. The technology analyzes historical data, market trends, and real-time information to generate accurate demand forecasts and optimize inventory.
Walmart uses AI to predict customer demand and manage stock levels effectively. This approach ensures product availability while reducing excess inventory, leading to cost savings and improved customer satisfaction.
AI’s impact extends throughout manufacturing operations, from production scheduling to quality control. Manufacturers who adopt this technology gain competitive advantages through increased productivity and reduced operational costs.
The technology’s potential continues to expand, offering early adopters significant advantages in efficiency, innovation, and market competitiveness.
Enhancing Financial Services with Generative AI
Generative AI transforms financial services through enhanced efficiency, personalization, and security. Financial institutions now streamline operations, from document processing to fraud detection, creating a more responsive and secure banking environment.
Financial institutions use generative AI to analyze and extract critical information from financial documents, contracts, and regulatory filings. This automation accelerates workflows and reduces human error, improving accuracy and compliance.
AI-powered chatbots and virtual assistants now handle complex customer interactions with natural language processing capabilities. These systems provide personalized financial advice, process transactions, and anticipate customer needs based on financial history and behavior patterns.
Advanced Fraud Detection
AI models analyze vast datasets in real-time to detect fraud, surpassing traditional rule-based systems. JPMorgan Chase reports their AI system cut false positives by 50% while boosting fraud detection effectiveness by 25%.
Metric | Improvement | Impact |
---|---|---|
Fraud Transaction Decline Rate | 58% | Reduction in lost sales, increased revenue |
Fraud Value Decline Rate | 25% | Incremental $74M in revenue |
Fraud Detection Rate | 141% | $13.2M in loss avoidance |
False Positive Rate | 50% reduction | Enhanced customer trust, reduced disruptions |
These AI systems continuously learn and adapt to new fraud patterns, protecting financial institutions while maintaining customer trust through reduced false positives.
Impact and Future Outlook
Banks now offer innovative products through AI-powered solutions, including robo-advisors that democratize sophisticated investment strategies for all investors, not just high-net-worth individuals.
The combination of generative AI with blockchain and quantum computing promises enhanced security, precise risk assessment, and innovative financial products. However, success depends on addressing data privacy concerns and maintaining ethical AI practices to realize this technology’s full potential.
Best Practices for Implementing Generative AI
Successful generative AI implementation requires a strategic focus on data quality, ethical considerations, and continuous monitoring. Organizations that prioritize these areas can maximize AI’s potential while maintaining accuracy and fairness.
Ensure High-Quality Data
High-quality data forms the bedrock of effective generative AI systems. Poor data leads to biased or inaccurate results that can undermine the entire AI infrastructure. Organizations should:
Implement data governance policies with clear quality standards and processes. Deploy automated tools to cleanse, validate, and monitor data. Keep datasets current through regular updates and maintenance.
AI expert Andrew Ng, founder of DeepLearning.AI, notes, “If 80 percent of our work is data preparation, then ensuring data quality is the most critical task for a machine learning team.”
Prioritize Ethical Considerations
Building trust requires strong ethical foundations in generative AI development. Organizations must:
Create comprehensive AI guidelines covering ethical principles, data privacy, and bias mitigation. Build a culture that encourages open dialogue about AI risks and benefits. Update policies regularly to match evolving ethical standards and regulations.
A Chief AI Officer can provide essential oversight and promote organization-wide awareness of ethical AI practices.
Implement Continuous Monitoring
AI systems need constant attention to maintain peak performance. Organizations should:
Set up real-time monitoring of AI outputs and performance metrics. Schedule regular audits to catch and fix biases or inaccuracies. Create feedback loops with users and stakeholders for ongoing improvement.
Adaptability and vigilance help organizations navigate the rapidly evolving AI landscape.
Ensure Transparency and Accountability
Trust in AI systems depends on operational transparency. Organizations must:
Document and share AI decision-making processes with stakeholders. Use explainable AI techniques to clarify how outputs are generated. Define clear responsibility lines for AI-driven decisions.
These best practices help organizations implement generative AI responsibly while prioritizing fairness and accuracy. Success requires ongoing commitment to learning, improvement, and ethical deployment.
Addressing Challenges and Risks of Generative AI
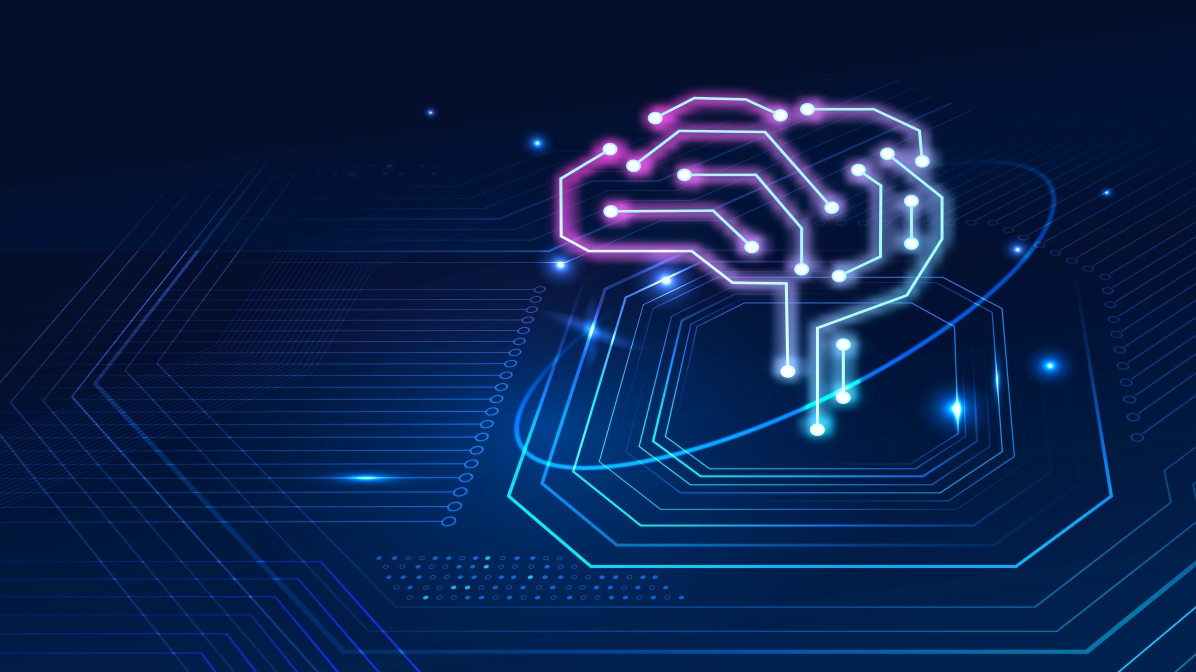
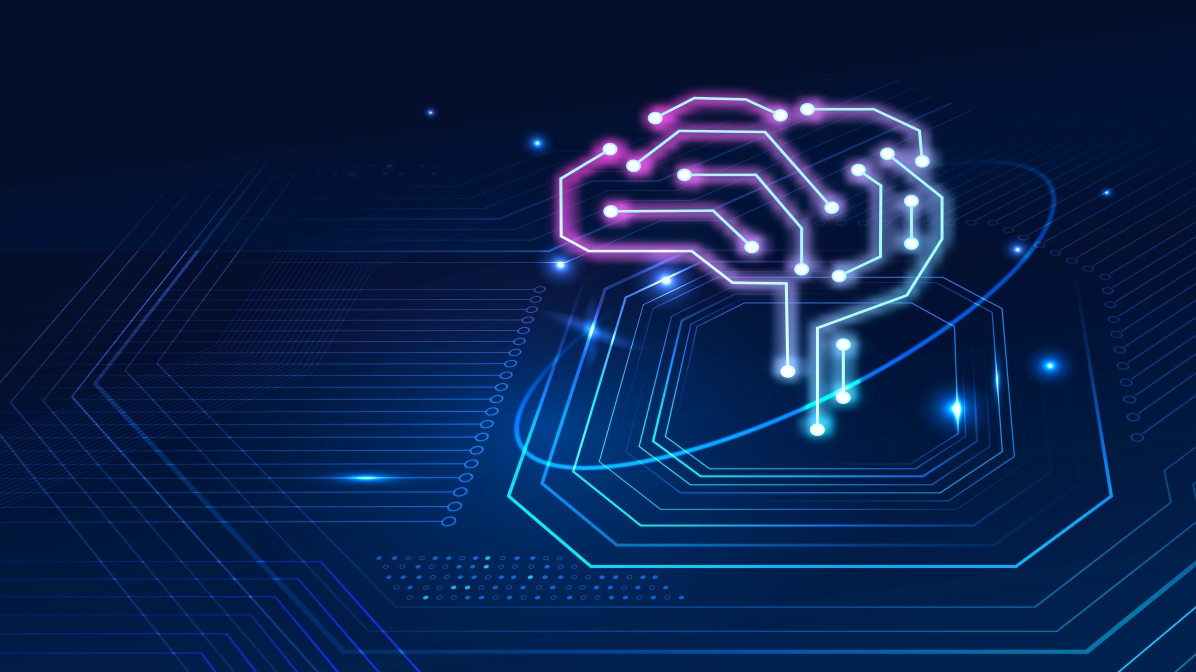
Stylized brain symbolizing AI and data for bias mitigation. – Via website-files.com
Generative AI presents significant challenges alongside its transformative capabilities. Organizations must address these challenges systematically to maximize benefits while minimizing risks.
Bias in AI Systems
AI models can perpetuate societal biases through their training data. Research shows text-to-image generators often associate beauty with lighter skin tones, while language models display gender stereotypes in career-related contexts.
Organizations combat bias by incorporating diverse training datasets and conducting regular bias assessments. This systematic approach helps create more equitable AI outputs.
Security Vulnerabilities
Advanced AI capabilities bring heightened security concerns. Bad actors can exploit these systems to create deepfakes, generate malicious code, or launch sophisticated misinformation campaigns.
Effective security requires robust authentication systems, advanced detection algorithms, and clear usage policies. Collaboration between AI developers, security experts, and policymakers strengthens protective measures.
Security Risk | Description |
---|---|
Deepfake Generation | Creation of highly realistic fake videos, images, or audio recordings that can lead to identity theft, financial fraud, or political instability. |
Automated Phishing Attacks | AI-generated phishing emails that mimic writing styles and personas to improve the effectiveness of social engineering attacks. |
Malicious Code Generation | Use of AI to write new and undetectable malicious code, pushing cybersecurity specialists into overdrive. |
Social Engineering | AI-powered attacks that create hyper-personalized and effective social engineering schemes, including voice phishing and catfishing. |
Adversarial Attacks on AI Systems | Generating inputs to trick AI systems into making incorrect outputs or decisions, undermining trust in AI-powered security systems. |
Data Poisoning | Altering training data to subvert AI behavior, potentially introducing vulnerabilities in AI-generated outputs. |
Model Theft and Reverse Engineering | Theft of AI models for creating competing systems or finding and exploiting vulnerabilities in AI-powered systems. |
AI-Generated Disinformation Campaigns | Creation of misleading content at scale, influencing public opinion and causing market panics. |
Privacy Leaks in AI Outputs | Unintentional exposure of private data in AI-generated outputs due to poor training data management. |
Overreliance on AI-Generated Content | Risk of spreading inaccuracies or biases by relying too heavily on AI-generated content without human verification. |
Model Transparency
The complexity of AI models creates challenges for transparency and accountability, particularly in healthcare and finance. Clear data collection practices and informed consent protocols help protect privacy while building trust.
Mitigation Strategies
- Diverse Training Data: Build representative datasets to reduce bias
- Regular Evaluations: Monitor and address emerging vulnerabilities
- Interdisciplinary Collaboration: Partner with experts across fields
- Ethical Guidelines: Implement clear frameworks for AI development
- User Education: Train users on AI capabilities and limitations
These strategies help organizations harness AI’s potential while building safer, more equitable systems. Success requires ongoing vigilance and commitment to responsible AI development.
Future Trends and Innovations in Generative AI
Generative AI advances rapidly, transforming how we work, create, and interact with technology. Key trends are shaping the next wave of AI innovation.
Multimodal Applications: Breaking Boundaries
Multimodal AI systems now seamlessly process and generate content across text, image, audio, and video simultaneously. Speak your product idea, and AI creates a 3D model, marketing copy, and brand-aligned jingle instantly.
Recent research shows multimodal AI redefining user interfaces for more natural, rich interactions.
Current Applications | Future Applications |
---|---|
Digital marketing: Crafting immersive, tailored content combining text, visuals, and sound. | Creating seamless, integrated multimodal systems that process and generate content across text, image, audio, and video simultaneously. |
Healthcare: Analyzing clinical notes, radiology images, lab results, and genetic information for accurate diagnoses and personalized treatment plans. | AI that can generate personalized treatment plans based on a patient’s genetic makeup, lifestyle, and real-time health data. |
Customer service: Chatbots that understand tone of voice, analyze facial expressions, and respond with appropriate verbal and visual cues. | AI agents that can draft contracts, conduct research, and predict case outcomes with remarkable accuracy in the legal sector. |
Education: Adapting to individual learning styles with a mix of text explanations, visual diagrams, interactive simulations, and audio guides. | AI that can describe a product idea verbally, generate a 3D model, marketing copy, and a jingle all aligned with a brand. |
Retail: Using purchase history, images, comments, and live browsing patterns to identify products to offer. | Hyper-personalized experiences in e-commerce, analyzing browsing history, purchase patterns, and mood for tailored recommendations. |
Hyper-Personalization: AI That Truly Knows You
AI systems now leverage data to deliver precisely tailored experiences. E-commerce platforms analyze browsing patterns and purchase history to create deeply personalized recommendations. Healthcare applications generate treatment plans based on individual genetic profiles and lifestyle data.
Seamless Integration into Business Processes
AI augments daily business operations across industries. Legal teams use AI to draft contracts and predict case outcomes. Manufacturing systems optimize supply chains and design innovative products based on market trends. The focus remains on enhancing human capabilities rather than replacing them.
Ethical Considerations and Responsible AI
Built-in safeguards against bias, enhanced privacy protections, and transparent decision-making processes mark the future of AI development. Explainable AI systems articulate their reasoning clearly, building trust and accountability in critical business and social applications.
The transformative potential of generative AI spans every sector. Success requires balancing innovation with ethical responsibility, ensuring these powerful tools benefit humanity while minimizing risks.
Conclusion and Strategic Recommendations
Generative AI holds transformative potential across industries, requiring a thoughtful, strategic approach to harness its power responsibly. Organizations must balance innovation with ethical considerations to unlock AI’s capabilities effectively.
Businesses should establish clear, measurable objectives aligned with strategic goals to implement generative AI successfully. Setting well-defined targets provides direction, whether the aim is boosting productivity, enhancing customer experiences, or driving innovation.
A robust ethical framework forms the cornerstone of responsible AI implementation. This framework addresses potential biases in training data, ensures transparency in AI-generated outputs, and implements strong data privacy and security measures. As Forbes notes, neglecting ethical implications creates significant business risks.
Organizations must invest in workforce development, creating an AI-fluent culture where employees understand AI’s capabilities and limitations. This human-AI collaboration drives meaningful innovation and value creation.
Continuous monitoring through regular audits and feedback loops helps identify issues and refine systems. AI implementation succeeds through iterative improvement rather than one-time deployment.
Strategic, ethically-grounded AI adoption positions businesses at the forefront of technological advancement. Success belongs to those who harness AI’s potential while navigating its complexities with wisdom and foresight.
Last updated:
Disclaimer: The information presented in this article is for general informational purposes only and is provided as is. While we strive to keep the content up-to-date and accurate, we make no representations or warranties of any kind, express or implied, about the completeness, accuracy, reliability, suitability, or availability of the information contained in this article.
Any reliance you place on such information is strictly at your own risk. We reserve the right to make additions, deletions, or modifications to the contents of this article at any time without prior notice.
In no event will we be liable for any loss or damage including without limitation, indirect or consequential loss or damage, or any loss or damage whatsoever arising from loss of data, profits, or any other loss not specified herein arising out of, or in connection with, the use of this article.
Despite our best efforts, this article may contain oversights, errors, or omissions. If you notice any inaccuracies or have concerns about the content, please report them through our content feedback form. Your input helps us maintain the quality and reliability of our information.