Revolutionizing Technology with AI and Machine Learning
Imagine a world where machines diagnose diseases, drive cars, and compose symphonies. This is the reality of artificial intelligence (AI) and machine learning shaping our world today. Although these terms are often used interchangeably, they represent different concepts.
AI encompasses the broader vision of machines that mimic human intelligence and decision-making. It’s the master blueprint for creating systems that reason, understand language, and solve complex problems. Machine learning, on the other hand, is a specific approach within AI that enables computers to learn and improve from experience without explicit programming for every task.
These technologies complement each other. While AI provides the framework for intelligent behavior, machine learning offers mechanisms for machines to get smarter over time through data analysis and pattern recognition. It’s like the difference between having a brain (AI) and the process of learning and growing (machine learning).
Exploring this technological frontier reveals how these innovations are transforming industries from healthcare to finance, changing how we work, and creating possibilities once limited to imagination. Whether you’re a business leader aiming to leverage these technologies or simply curious about their impact, understanding the nuances between AI and machine learning is crucial in today’s rapidly evolving digital landscape.
Understanding Artificial Intelligence
Artificial intelligence (AI) has evolved from science fiction into an integral part of our daily lives, fundamentally changing how we interact with technology. AI aims to replicate the cognitive capabilities of the human brain, from processing complex information to making nuanced decisions in real-time.
AI’s true power lies in emulating core human cognitive functions. Just as our brains analyze visual inputs, process language, and solve multifaceted problems, AI systems perform sophisticated pattern recognition, natural language processing, and complex problem-solving tasks. For instance, when you ask Siri to set a reminder, multiple AI processes work together—converting your speech to text, understanding your intent, and executing the appropriate action.
AI is highly visible in customer service, where automated chatbots engage in natural conversations with users. These systems don’t just follow pre-programmed scripts—they learn from interactions, becoming more adept at understanding context and providing relevant responses over time. According to Forbes, AI-powered virtual assistants have transformed customer support by providing instant, 24/7 responses while continuously improving through machine learning.
Beyond these examples, AI’s cognitive capabilities extend into more complex domains. In healthcare, AI systems analyze medical images with remarkable accuracy, often detecting subtle patterns that might escape human observation. In financial services, AI algorithms process vast amounts of data to identify fraudulent transactions in real-time, demonstrating their ability to make swift, accurate decisions based on complex criteria.
AI systems now demonstrate adaptive learning—they don’t just follow static rules but can modify their behavior based on new information, much like human learning. This capability enables them to tackle increasingly sophisticated challenges, from composing music to driving cars, showcasing the extraordinary potential of artificial intelligence to enhance and augment human capabilities across countless domains.
What is Machine Learning?
Machine learning is a branch of artificial intelligence that enables computers to learn and evolve without explicit programming. It allows systems to recognize intricate patterns within vast amounts of data, making predictions and decisions with increasing accuracy over time.
Unlike traditional software that follows rigid rules, machine learning systems develop their own understanding through experience. When exposed to new data, these systems analyze and adapt, continuously refining their ability to identify trends and generate insights. This self-improving capability makes machine learning valuable for tasks that are too complex for conventional programming approaches.
Machine learning can handle both structured and unstructured data. Whether processing numerical datasets, analyzing images, or interpreting natural language, these systems can extract meaningful patterns that might be invisible to human observers. For example, a machine learning model might analyze millions of financial transactions to detect fraudulent activities or study medical images to identify potential health concerns with precision.
One of the most fascinating aspects of machine learning is its iterative nature. As IBM explains, these systems use data and algorithms to imitate the way humans learn, gradually improving their accuracy through experience. The more data a machine learning system processes, the better it becomes at making accurate predictions and decisions.
The practical applications of machine learning extend across many industries. From powering recommendation systems that suggest your next favorite movie to enabling autonomous vehicles to navigate complex environments, machine learning algorithms drive innovation in ways that were once confined to science fiction. These systems can process and analyze data at scales far beyond human capability, opening new possibilities for scientific discovery, business optimization, and technological advancement.
Applications of AI and Machine Learning
Artificial intelligence and machine learning are reshaping critical industries, delivering capabilities once confined to science fiction. In healthcare, AI-powered diagnostic tools are enhancing patient care. Studies show that AI solutions are improving efficiency while reducing costs across multiple sectors, marking a new era in technological advancement.
The healthcare sector exemplifies AI’s transformative power. Machine learning algorithms analyze medical images with remarkable precision, helping radiologists detect diseases earlier and with greater accuracy. Smart systems monitor patient vital signs in real-time, alerting medical staff to potential complications before they become critical. These innovations have improved countless lives through faster, more accurate diagnoses.
In the financial sector, AI systems process vast amounts of transaction data to detect fraudulent activities in real-time. Banks and investment firms leverage machine learning models to assess credit risks, optimize trading strategies, and provide personalized financial advice. This technological evolution has made financial services more secure and accessible while enabling institutions to make data-driven decisions with unprecedented speed.
Sector | Examples of AI Adoption | Risks | Future Prospects |
---|---|---|---|
Financial Services | Fraud detection, investment decisions, automated document processing | Bias, privacy breaches, cybersecurity threats | Continuous oversight, alignment with industry standards |
Healthcare | Scientific discovery, informed decision-making, administrative task automation | Switchover disruption, AI hallucinations, privacy breaches | Careful change management, digital adoption platforms |
Retail | Inventory tracking, personalized recommendations, dynamic pricing | Potential for mass unemployment, shift from creative human work | Identifying right opportunities, multi-site infrastructure investment |
Manufacturing has undergone its own AI revolution. Smart factories now employ machine learning algorithms to predict equipment maintenance needs, preventing costly breakdowns. Production lines utilize AI-powered quality control systems that can spot defects invisible to the human eye, ensuring higher product quality and reduced waste. These advancements have led to significant improvements in operational efficiency and output quality.
The impact of these technologies extends beyond operational improvements. AI and machine learning are creating new possibilities for innovation and growth. From predictive analytics that forecast market trends to natural language processing systems that enhance customer service, these technologies are becoming indispensable tools for businesses aiming to maintain competitive advantages in their industries.
Advantages of AI and Machine Learning
In a data-driven business landscape, sophisticated tools are essential for processing vast amounts of information. AI and machine learning have emerged as transformative technologies that offer concrete advantages for organizations. Let’s explore the key benefits that make these technologies indispensable for modern enterprises.
The most immediate advantage of AI and machine learning is their unparalleled ability to enhance operational efficiency. According to research shared by Deloitte, organizations implementing AI see improvements in product enhancement, internal operations optimization, and external operations streamlining. These systems work tirelessly around the clock, processing data volumes that would overwhelm human analysts.
Decision-making receives a dramatic boost through AI and machine learning capabilities. These technologies excel at identifying complex patterns and correlations within massive datasets that human analysts might miss. By leveraging historical data and real-time inputs, AI systems can predict trends and potential outcomes with remarkable accuracy, enabling leaders to make more informed strategic choices.
Cost reduction represents another significant benefit of AI implementation. By automating routine tasks and optimizing resource allocation, organizations can substantially lower operational expenses. AI systems can predict maintenance needs, streamline supply chains, and minimize waste through precise inventory management. The technology’s ability to prevent costly errors and optimize processes creates lasting financial benefits.
AI and machine learning transform how organizations derive actionable insights from their data. These technologies don’t just process information faster – they uncover subtle patterns and relationships that drive meaningful business intelligence. Companies can better understand customer behavior, market trends, and operational inefficiencies through advanced analytics powered by AI.
The impact of AI extends beyond mere automation – it’s about augmenting human capabilities and enabling better strategic decisions through data-driven insights.
Dr. Stylianos Kampakis, CEO of The Data Scientist
Organizations implementing AI-driven solutions also benefit from improved accuracy and consistency in their operations. Unlike human operators who may tire or make inconsistent judgments, AI systems apply the same rigorous analysis to every task, every time. This reliability proves especially valuable in quality control, risk assessment, and compliance monitoring.
Challenges and Ethical Considerations
As artificial intelligence and machine learning technologies reshape our world, they bring forth complex challenges that demand careful consideration. At the forefront of these concerns lies data privacy – a critical issue as AI systems process vast amounts of personal information. Recent analysis shows that AI systems handle extensive personal data, raising serious questions about collection methods, storage security, and algorithmic usage patterns.
The ethical implications of AI extend beyond privacy concerns into the realm of algorithmic bias. When AI systems are trained on biased or incomplete datasets, they risk perpetuating and amplifying existing societal inequalities. This manifests in various ways, from unfair hiring practices to discriminatory lending decisions, highlighting the need for rigorous testing and validation protocols.
Transparency and accountability present another significant challenge. AI systems, particularly those employing deep learning, often operate as “black boxes,” making decisions that are difficult for humans to interpret or explain. This opacity becomes particularly problematic when these systems are deployed in critical areas such as healthcare, criminal justice, or financial services, where the reasoning behind decisions must be clear and justifiable.
Addressing these challenges requires a multi-faceted approach. Organizations must implement robust frameworks for responsible AI development, incorporating ethical guidelines from the earliest stages of design. This includes establishing clear protocols for data collection and usage, implementing strong security measures, and ensuring transparency in AI decision-making processes.
Perhaps most crucially, the advancement of AI technology must be balanced with societal interests. This means developing comprehensive regulatory frameworks that protect individual rights while fostering innovation. Industry leaders and policymakers must collaborate to create standards that ensure AI systems are developed and deployed responsibly, with clear accountability measures in place.
One of the top ethical issues with AI is privacy and data protection. AI systems generally handle vast amounts of personal data, and thus concerns surrounding the methods used to collect, store, and use this data by AI algorithms remain.
DATAVERSITY
The path forward requires continuous monitoring and evaluation of AI systems’ impacts on society. Regular audits of AI algorithms for bias, ongoing assessment of privacy protections, and transparent reporting of AI outcomes are essential components of responsible innovation. Only through such diligent oversight can we ensure that AI advancement serves the collective good while minimizing potential harms.
Leveraging SmythOS for AI Development
SmythOS transforms traditional AI development through its innovative visual workflow builder, making complex knowledge graph integration and agent creation accessible to both technical and non-technical teams. Unlike conventional platforms that demand extensive coding expertise, SmythOS enables organizations to build sophisticated AI applications through an intuitive drag-and-drop interface.
The platform’s standout feature is its comprehensive debugging environment, which provides unprecedented visibility into AI operations. The built-in visual debugger allows developers to examine workflows in real-time, enabling quick identification and resolution of issues. This approach significantly reduces development time while ensuring the accuracy of data connections and relationships within the system.
Enterprise organizations particularly benefit from SmythOS’s seamless integration with major graph databases. According to Stanford’s AI Lab research, these integrations enable real-time analysis of multifaceted relationships between entities, allowing businesses to derive actionable insights from their interconnected data. The platform’s support for established graph databases ensures compatibility with existing infrastructure while providing the flexibility to scale as organizational needs evolve.
The platform streamlines AI development through its intelligent process agents, which handle much of the heavy lifting in knowledge graph creation and management. These agents automatically pull information from various sources and organize it into meaningful connections, reducing the time and effort required to build and maintain complex AI systems while minimizing the potential for human error.
Perhaps most impressively, SmythOS maintains enterprise-grade security throughout its operations. The platform implements stringent security measures to protect sensitive data and knowledge bases while enabling seamless integration with existing enterprise systems. This makes SmythOS particularly valuable for organizations handling confidential information within their AI infrastructure.
Conclusion and Future Prospects
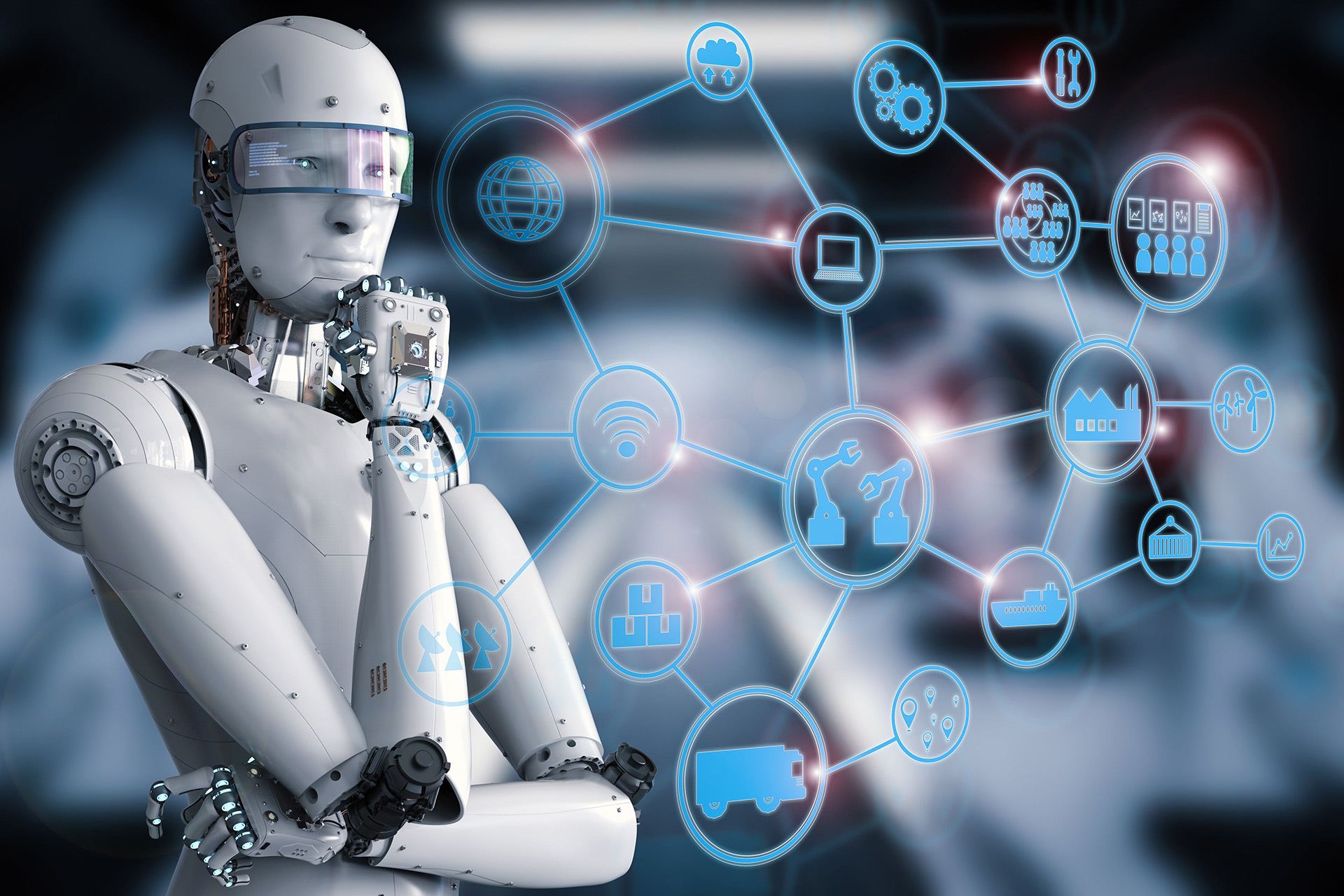
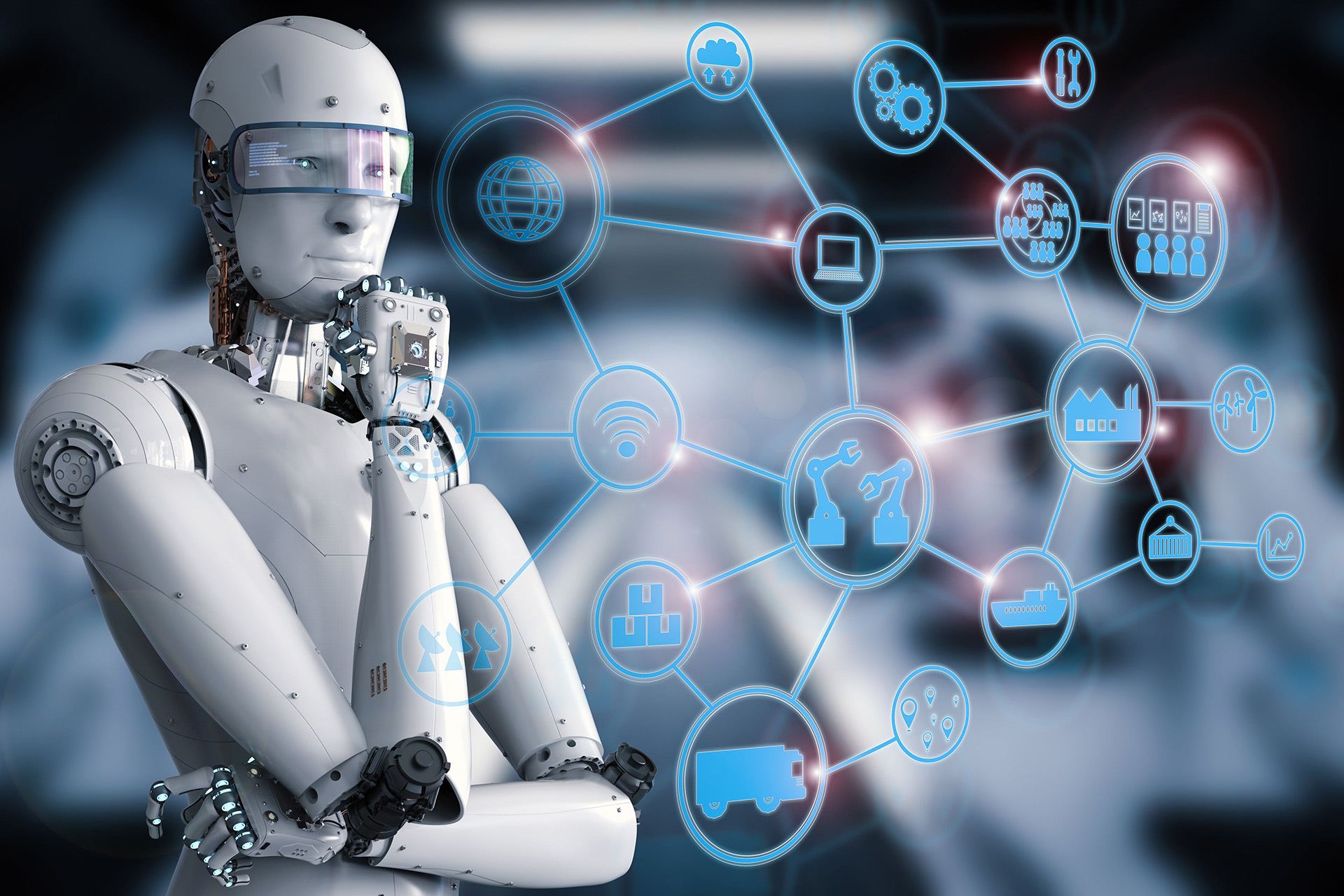
A robot contemplating technology and connectivity. – Via cio.com
The future of artificial intelligence and machine learning stands at a transformative threshold, poised to revolutionize industries in ways previously unimagined. Recent developments show these technologies are already enhancing efficiency and decision-making across sectors, from healthcare to manufacturing. The rapid evolution of AI capabilities, particularly in natural language processing and autonomous systems, signals an exciting trajectory ahead.
Significant refinements in AI technologies will make them even more integral to daily operations. According to recent industry analysis, the integration of quantum computing with AI could dramatically reduce computational time and resource requirements, enabling more sophisticated and efficient AI systems.
The emergence of multi-agent systems and specialized AI collaborators represents another frontier in this evolution. Rather than relying on monolithic AI models, the future points toward networks of specialized agents working in concert to solve complex problems. SmythOS exemplifies this forward-thinking approach, providing a comprehensive platform for orchestrating AI agents that can adapt and scale to meet evolving business needs.
As these technologies mature, we’ll likely witness a shift toward more intuitive and accessible AI implementations. Organizations that embrace these advancements while maintaining a focus on ethical considerations and human-centric design will be best positioned to thrive in this new era. The key to success lies not just in adopting AI, but in thoughtfully integrating it to augment human capabilities rather than replace them.
The journey ahead promises continued innovation and discovery in the field of artificial intelligence. While challenges remain, particularly around ethics and governance, the potential benefits of these advancing technologies far outweigh the obstacles. Through careful development and implementation, AI and machine learning will undoubtedly shape a more efficient, intelligent, and connected future for us all.
Last updated:
Disclaimer: The information presented in this article is for general informational purposes only and is provided as is. While we strive to keep the content up-to-date and accurate, we make no representations or warranties of any kind, express or implied, about the completeness, accuracy, reliability, suitability, or availability of the information contained in this article.
Any reliance you place on such information is strictly at your own risk. We reserve the right to make additions, deletions, or modifications to the contents of this article at any time without prior notice.
In no event will we be liable for any loss or damage including without limitation, indirect or consequential loss or damage, or any loss or damage whatsoever arising from loss of data, profits, or any other loss not specified herein arising out of, or in connection with, the use of this article.
Despite our best efforts, this article may contain oversights, errors, or omissions. If you notice any inaccuracies or have concerns about the content, please report them through our content feedback form. Your input helps us maintain the quality and reliability of our information.