Semantic AI in Marketing: Boosting Campaign Success
Imagine a marketing team that knows exactly what customers want before they even express it. That’s the power of Semantic AI, a technology that’s changing how brands connect with their audiences. By using natural language processing and machine learning, Semantic AI is transforming how marketers understand and engage with customers.
With AI-powered marketing projected to generate over $15 trillion for the global economy by 2030, Semantic AI is leading this change. Unlike traditional marketing tools, Semantic AI understands context, nuance, and meaning behind customer interactions, enabling personalized experiences at scale.
Think of Semantic AI as your marketing team’s interpreter, translating customer behavior into actionable insights and crafting messages that resonate. Whether analyzing social media sentiment, optimizing email campaigns, or predicting customer needs, Semantic AI turns casual browsers into loyal customers.
This article explores the applications of Semantic AI in marketing—from enhancing customer engagement to improving targeting precision. We’ll discuss the benefits businesses are experiencing and share strategies for integrating this technology into your marketing toolkit. Discover how Semantic AI is setting new standards for marketing excellence.
Understanding Semantic AI
Semantic AI transforms how brands connect with their audiences by understanding the meaning and context behind human language, enabling more intelligent and targeted marketing approaches.
At its core, Semantic AI works like a skilled interpreter. It comprehends the deeper meaning and relationships between words. When a customer searches for “lightweight laptop for travel,” Semantic AI understands they’re looking for portable computing solutions, even if product descriptions don’t use those exact terms. This contextual understanding allows marketers to deliver more relevant content and recommendations.
The real power of Semantic AI lies in its ability to analyze vast amounts of customer data and extract meaningful patterns. According to McKinsey & Company, companies using AI-powered personalization see a sales lift of 10-30% compared to traditional approaches. By processing everything from social media interactions to purchase histories, Semantic AI helps marketers understand not just what customers are saying, but what they truly want.
Major brands are leveraging this technology effectively. Sephora, for example, uses Semantic AI to analyze customer reviews and social media comments to understand product sentiment and identify emerging beauty trends. This deep understanding enables them to create highly targeted marketing campaigns that speak directly to customer needs and preferences.
Perhaps most impressively, Semantic AI excels at predicting future customer behavior by identifying subtle patterns in data. It can spot early indicators of changing consumer preferences or emerging market trends, allowing marketers to proactively adjust their strategies. For instance, when analyzing social media conversations, Semantic AI might detect growing interest in sustainable products before it becomes a mainstream trend, giving brands an early opportunity to align their messaging.
The future of marketing lies in understanding not just what customers say, but what they mean. Semantic AI bridges this gap by bringing human-like comprehension to digital interactions.
McKinsey Digital Report, 2023
In practice, this means marketers can move beyond broad demographic targeting to create truly personalized campaigns that resonate with individual customers. Whether it’s crafting email subject lines that drive higher open rates or designing social media ads that generate more engagement, Semantic AI provides the insights needed to make every marketing touchpoint more effective and meaningful.
Applications of Semantic AI in Marketing
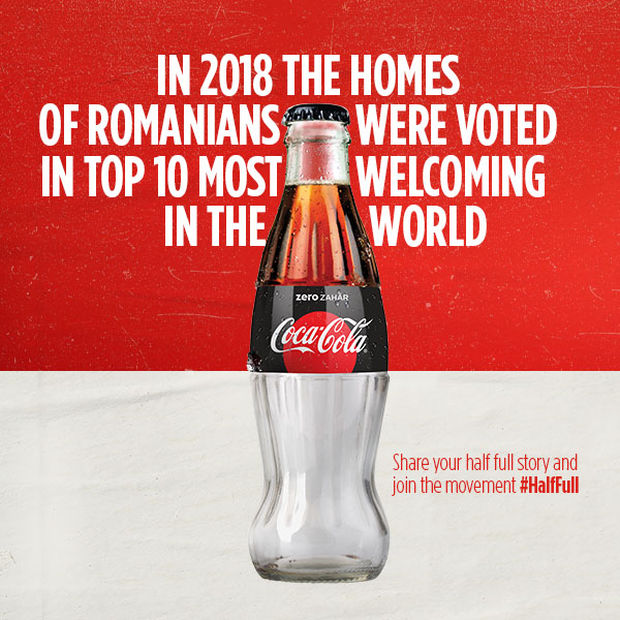
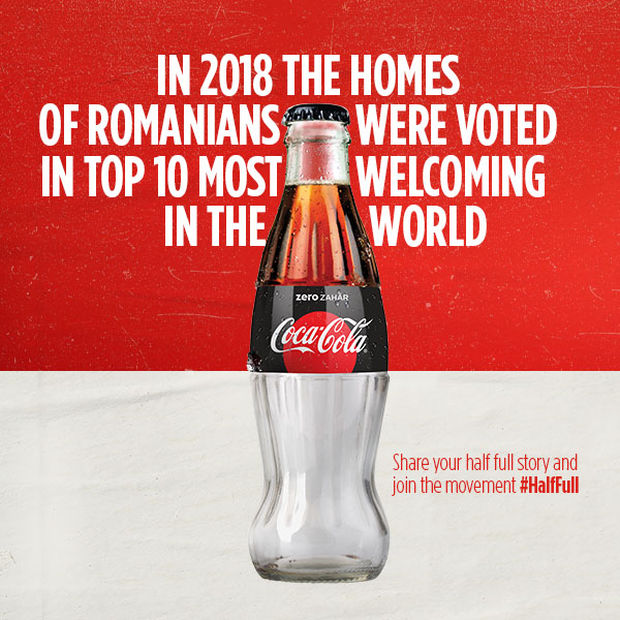
Marketing has entered a transformative era where semantic AI technologies are enhancing how brands connect with their audiences. By processing and understanding natural language with unprecedented accuracy, semantic AI enables marketers to create more intelligent, personalized, and effective campaigns.
AI-powered ad campaign optimization is at the forefront of this evolution. Recent research shows that predictive analytics are now used for sophisticated lead scoring and campaign optimization, aiding marketers in making data-driven decisions about their advertising strategies. This capability allows for real-time adjustments to targeting parameters and creative elements, significantly improving campaign performance.
Personalization has become increasingly sophisticated through semantic AI’s ability to understand customer context and intent. Rather than relying on basic demographic data, AI systems now analyze behavioral patterns, preferences, and historical interactions to deliver truly customized content experiences. The technology examines customer data across multiple touchpoints, creating comprehensive profiles that enable marketers to deliver relevant messages at precisely the right moment.
With the rapid advancement of artificial intelligence technology, the advertising industry is at a crossroads of new opportunities and challenges, particularly in areas of targeting, personalization, content creation, and ad optimization.
Journal of Advertising Research
Customer experience has seen remarkable improvements through the implementation of intelligent chatbots and sentiment analysis tools. These AI-powered systems can now understand complex customer queries, provide contextually relevant responses, and even detect emotional undertones in customer communications. This enhanced understanding allows brands to deliver more empathetic and effective customer service while gathering valuable insights about customer satisfaction and needs.
Marketing teams are discovering that semantic AI’s impact extends beyond just automation – it fundamentally changes how they understand and respond to customer needs. By processing vast amounts of unstructured data from social media, customer reviews, and support interactions, these systems can identify emerging trends and sentiment shifts that would be impossible to detect manually, enabling proactive strategy adjustments and more nuanced customer engagement approaches.
Benefits of Semantic AI for Marketers
Semantic AI represents a significant advancement in how marketers understand and engage with their audiences. By processing vast amounts of customer data with contextual understanding, this technology transforms raw information into actionable insights that drive meaningful results.
One of the most compelling advantages of Semantic AI lies in its ability to decode customer preferences with unprecedented precision. Unlike traditional analytics that simply track clicks and views, Semantic AI comprehends the subtle nuances of customer behavior, helping marketers craft hyper-personalized campaigns that truly resonate. Through advanced natural language processing and machine learning algorithms, marketers can now understand not just what customers do, but why they do it.
Campaign management becomes remarkably more efficient with Semantic AI at the helm. The technology automatically analyzes campaign performance, adjusts targeting parameters in real-time, and optimizes resource allocation across channels. This level of automation eliminates countless hours of manual analysis and adjustment, allowing marketing teams to focus on strategic initiatives rather than getting bogged down in data analysis.
Semantic AI proves its worth through intelligent resource utilization. The technology identifies which marketing activities generate the highest returns and automatically reallocates resources to maximize results. By understanding that certain customer segments respond better to specific message types or channels, Semantic AI can help marketers achieve significantly higher conversion rates while reducing wasteful spending.
Marketing teams also benefit from Semantic AI’s ability to predict emerging trends and customer needs. By analyzing patterns in customer interactions, social media conversations, and market data, the technology can alert marketers to opportunities before they become obvious to competitors. This predictive capability gives organizations a crucial competitive edge in today’s market.
With its ability to analyze data in real-time and automate routine tasks, semantic AI can free up resources and allow businesses to focus on more strategic initiatives.
Dezzai Research Report
Perhaps most importantly, Semantic AI enhances decision-making confidence by providing clear, contextual insights rather than just raw data. Marketers can now base their strategies on deep understanding rather than gut feelings, leading to more consistent success in their campaigns and better long-term relationships with customers.
Aspect | Traditional Analytics | Semantic AI |
---|---|---|
Nature | Static | Dynamic |
Data Handling | Manual data sorting and basic statistical software | Automates data collection, cleaning, and analysis |
Response Time | Days or weeks to answer new questions | Real-time analysis and responses |
Analysis Focus | Answers ‘what’ questions | Answers ‘why’ and ‘how’ questions |
Scalability | Limited by manual processes | Scales effortlessly with data volume |
Predictive Capabilities | Limited to basic statistical methods | Uses advanced algorithms for accurate predictions |
Bias | Influenced by human hypotheses and biases | Produces unbiased answers from exhaustive data testing |
Challenges in Implementing Semantic AI
Organizations aiming to harness Semantic AI face significant challenges beyond technical implementations. The promise of intelligent systems that understand context and meaning comes with complex hurdles that require careful consideration.
Data privacy concerns are a major challenge in implementing Semantic AI. These systems need vast amounts of training data to build comprehensive knowledge graphs, requiring a balance between data utility and protection. Integrating sensitive business information and personal data into semantic networks raises critical questions about data governance and compliance with evolving privacy regulations.
Technical Infrastructure and Expertise Requirements
Implementing Semantic AI demands a robust technical foundation that many organizations struggle to establish. High-performance computing resources, specialized software tools, and sophisticated data storage solutions are just the beginning of the necessary infrastructure investment.
Finding and retaining talent is another significant obstacle. The field requires data scientists and engineers with a unique blend of skills—from traditional machine learning expertise to specialized knowledge in natural language processing and knowledge representation. This talent scarcity often leads to prolonged implementation timelines and increased operational costs.
Most organizations underestimate the complexity of preparing their existing data for semantic analysis. Cleaning, standardizing, and annotating data requires significant manual effort and expertise. Without proper data preparation, Semantic AI systems may produce unreliable or inconsistent results.
Integration with legacy systems poses additional technical challenges. Many enterprises operate with decades-old infrastructure not designed to support modern AI applications. Creating seamless connections between these systems and new semantic technologies often requires extensive customization and careful architectural planning.
The rapid evolution of semantic technologies means today’s solutions might quickly become outdated. Organizations must maintain flexibility in their implementation approaches while ensuring their investments remain valuable as the technology landscape continues to shift.
Cost and Resource Management
The financial implications of implementing Semantic AI extend beyond initial setup costs. Ongoing maintenance, system updates, and the need for continuous training and refinement of models create a significant operational burden that organizations must carefully consider.
Cloud computing costs for processing and storing vast amounts of semantic data can quickly escalate. Organizations often struggle to accurately forecast these expenses, leading to budget overruns and delayed implementation timelines.
The need for specialized tools and software licenses adds another layer of complexity to the cost structure. Many enterprise-grade semantic analysis tools require substantial licensing fees, making it crucial for organizations to carefully evaluate their return on investment.
Training and upskilling existing staff represents another hidden cost. Even with experienced technical teams, the unique requirements of Semantic AI often necessitate additional training programs and workshops to ensure effective implementation and maintenance.
Resource allocation becomes particularly challenging when organizations need to balance Semantic AI initiatives with other digital transformation projects. The comprehensive nature of semantic technologies often requires dedicated teams and sustained focus, which can strain already limited IT resources.
Best Practices for Integrating Semantic AI
Clean data management forms the bedrock of successful Semantic AI integration. Recent industry analysis shows that organizations failing to maintain high-quality data face up to 20% higher operational costs and significantly reduced AI model performance.
Data scientists must implement rigorous data cleaning protocols before feeding information into Semantic AI systems. This includes standardizing formats, removing duplicates, and ensuring consistent labeling across datasets. Regular data audits help identify and correct quality issues before they impact model performance.
Step | Description |
---|---|
Define Data Cleaning Objectives | Assess raw data and identify requirements or desired output to focus on specific parts of the data, saving time and resources. |
Eliminate Duplicate or Irrelevant Values | Locate and remove repetitive or irrelevant data to achieve a more optimized dataset. |
Correct Structural Flaws | Fix structural errors such as misspellings, incorrect word usage, improper naming conventions, and capitalization mistakes. |
Remove Data Outliers | Identify and remove unusual values that differ greatly from existing values in the dataset to maintain data accuracy. |
Restore Any Missing Data | Scan the dataset thoroughly for any missing values, blank spaces, or empty cells and restore them. |
Ensure Data Validity | Perform a final quality check on the dataset to ensure its authenticity, consistency, and structure. |
Perform Detailed Data Profiling | Examine the dataset’s completeness, quality, and structure to discover trends, anomalies, and problems with data quality. |
Leverage Automated Data Cleaning Tools | Use automated data cleaning solutions to save time and introduce regular data cleansing into the workflow. |
Continuously Monitor and Improve Processes | Regularly review and improve data cleaning practices to maintain precise, consistent, and high-quality data. |
Ensure Continued Data Governance | Establish a strong data governance architecture to ensure data quality over time, including policies and procedures for data entry, updates, and retrieval. |
Continuous model training represents another crucial element for optimal Semantic AI integration. Marketing teams should establish feedback loops that allow models to learn from new data and adapt to changing market conditions. This iterative approach helps maintain model accuracy and prevents performance degradation over time.
The success of Semantic AI heavily depends on the quality of the data it processes. Implementing advanced semantic mapping techniques can be a game changer when dealing with structured data.
Illumex AI Research
Cross-functional collaboration between data scientists and marketing teams is essential for maximizing Semantic AI’s potential. Data scientists bring technical expertise in model development and optimization, while marketers contribute vital domain knowledge and understanding of customer behavior. This partnership ensures AI solutions align with business objectives and deliver actionable insights.
Organizations should also establish clear governance frameworks to maintain data integrity and security. This includes implementing automated quality checks, defining access controls, and ensuring compliance with relevant regulations. Regular monitoring and evaluation help identify areas for improvement and maintain high standards of data management.
Conclusion and Future of Semantic AI in Marketing
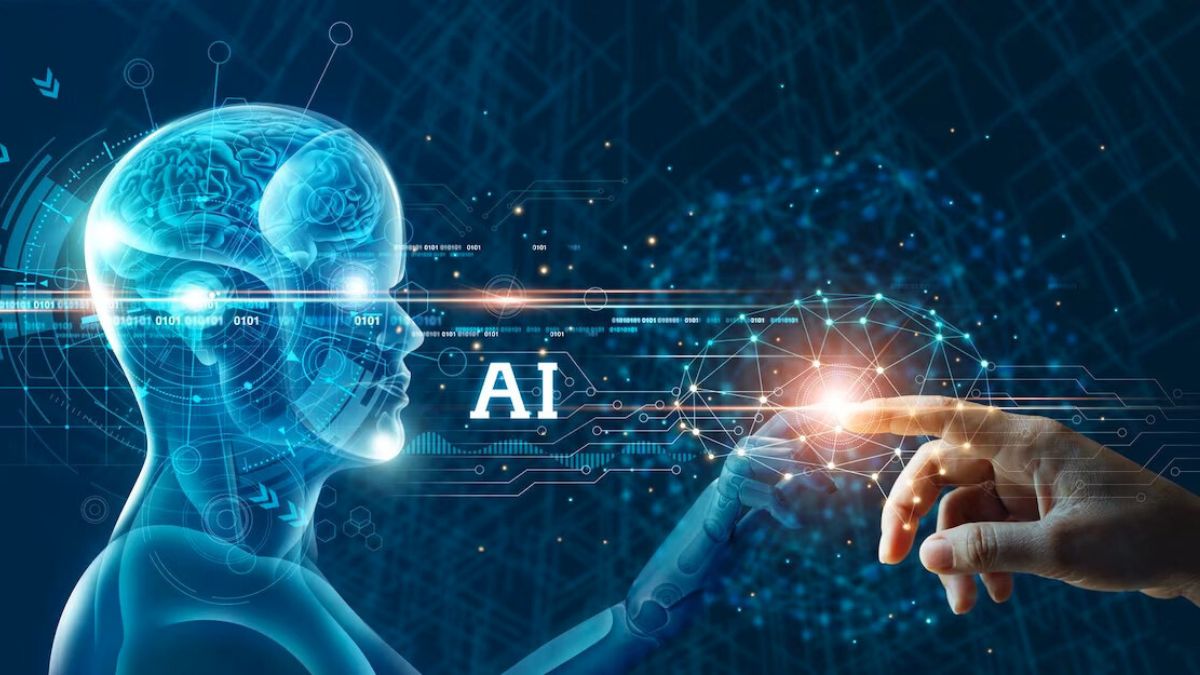
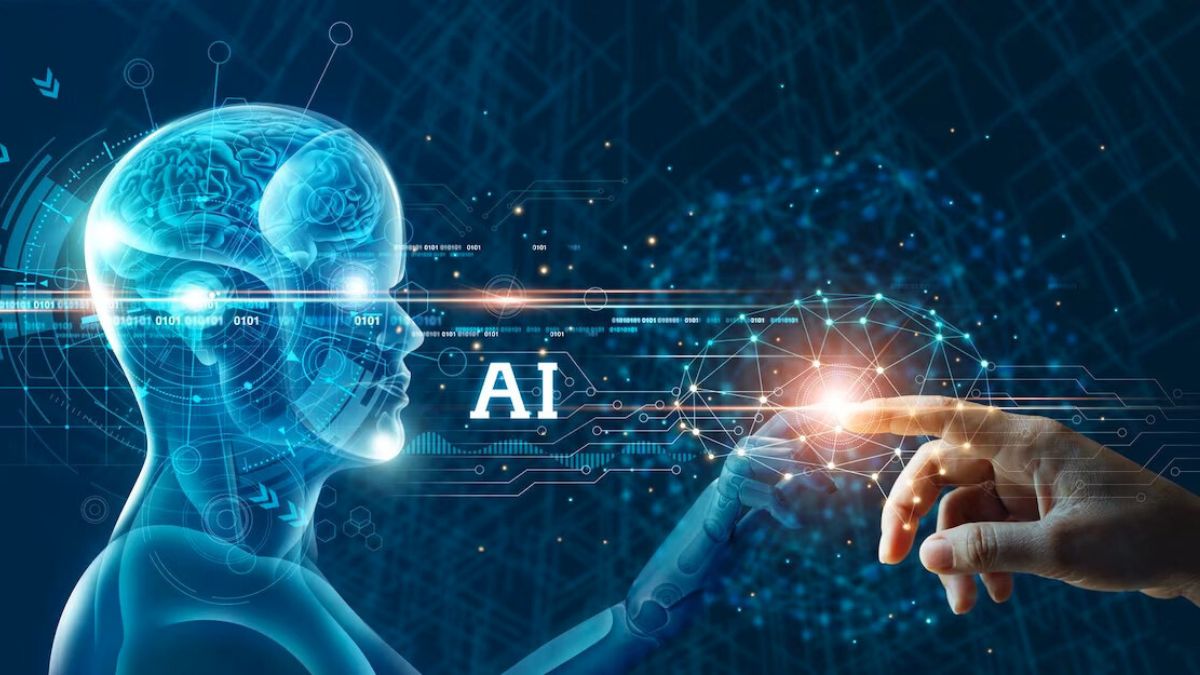
A figure with a transparent head reaching out to a hand. – Via smythos.com
The transformative potential of Semantic AI in marketing stands at a pivotal moment. As organizations increasingly recognize its value, the technology continues to evolve, offering unprecedented opportunities for personalized customer engagement and data-driven decision making. Research indicates that AI-powered marketing solutions are projected to grow from $6.48 billion in 2021 to $40.09 billion by 2026, highlighting the technology’s expanding influence.
The journey ahead requires marketers to thoughtfully address implementation challenges while embracing innovative solutions. By focusing on ethical AI practices and maintaining transparent data governance, organizations can build trust while leveraging advanced capabilities like predictive analytics and automated campaign optimization. This balanced approach ensures sustainable growth while protecting consumer privacy and maintaining brand integrity.
Companies like SmythOS are pioneering the next wave of marketing transformation by simplifying AI integration through specialized tools and platforms. Their approach to AI orchestration enables businesses of all sizes to harness sophisticated marketing capabilities without requiring extensive technical expertise. By providing visual builders and enterprise-grade security, such platforms democratize access to powerful AI marketing tools while ensuring responsible implementation.
The future of Semantic AI in marketing lies not just in automation, but in creating more meaningful and personalized customer experiences. As systems become more sophisticated in understanding consumer intent and behavior patterns, marketers can expect even more precise targeting and engagement opportunities. This evolution will empower brands to deliver more relevant content and services while optimizing resource allocation.
Looking ahead, success in this AI-driven landscape will depend on organizations’ ability to blend human creativity with artificial intelligence capabilities. By embracing these technological advances while maintaining focus on authentic human connections, marketers can unlock new levels of performance and innovation in their campaigns. The key lies in viewing Semantic AI not as a replacement for human expertise, but as a powerful tool for amplifying marketing effectiveness and driving sustainable growth.
Last updated:
Disclaimer: The information presented in this article is for general informational purposes only and is provided as is. While we strive to keep the content up-to-date and accurate, we make no representations or warranties of any kind, express or implied, about the completeness, accuracy, reliability, suitability, or availability of the information contained in this article.
Any reliance you place on such information is strictly at your own risk. We reserve the right to make additions, deletions, or modifications to the contents of this article at any time without prior notice.
In no event will we be liable for any loss or damage including without limitation, indirect or consequential loss or damage, or any loss or damage whatsoever arising from loss of data, profits, or any other loss not specified herein arising out of, or in connection with, the use of this article.
Despite our best efforts, this article may contain oversights, errors, or omissions. If you notice any inaccuracies or have concerns about the content, please report them through our content feedback form. Your input helps us maintain the quality and reliability of our information.