Knowledge Graphs in Data Analytics: Unlocking Insights
Did you know that enterprises using knowledge graphs in their AI implementations reduce technical debt by 75% compared to those that don’t? This approach to data management is transforming how organizations derive meaning from their vast information landscapes.
Think of knowledge graphs as the digital equivalent of how our brains connect information—linking people, places, events, and concepts in an intricate web of relationships. Unlike traditional databases that store data in rigid tables, knowledge graphs create rich, interconnected networks that mirror how humans naturally process information.
According to Gartner’s analysis, the semantic layer of knowledge graphs makes data interpretation more intuitive for analytics leaders while enabling AI algorithms to leverage information for sophisticated analysis and operational use cases.
The semantic layer of the knowledge graph makes it more intuitive and easy to interpret, making the analysis easy for D&A leaders. It adds depth and meaning to the data usage and content graph, allowing AI/ML algorithms to use the information for analytics and other operational use cases.
Gartner
As organizations grapple with increasingly complex data environments, knowledge graphs have emerged as the foundation for modern analytics. They break down data silos, enable contextual understanding, and provide the semantic richness necessary for advanced AI applications. The global graph database market, currently valued at $2.12 billion, is projected to reach $10.3 billion by 2032—a testament to their growing importance in the data analytics landscape.
This guide explores how knowledge graphs are revolutionizing data analytics, examines their key benefits, and explains why they’ve become indispensable for organizations seeking to extract maximum value from their data assets.
Benefits of Knowledge Graphs in Analytics
Modern organizations face a challenge: making sense of vast amounts of disconnected data. Knowledge graphs have emerged as a solution, creating an intelligent web of information that transforms how businesses analyze and utilize their data assets.
According to industry research, organizations implementing knowledge graphs have seen up to a 300% improvement in their AI model accuracy. This enhancement stems from the graphs’ unique ability to provide rich contextual information and validated relationships between data points, effectively reducing errors in AI-generated insights.
One of the most powerful benefits of knowledge graphs is their ability to unify fragmented data sources. Rather than struggling with isolated information silos, businesses can now create comprehensive views of their data landscape. For example, when a financial institution connects customer profiles, transaction histories, and market data through a knowledge graph, they can quickly identify patterns that may indicate new investment opportunities or potential fraud risks.
Enhanced Decision-Making Capabilities
Knowledge graphs revolutionize the decision-making process by providing leaders with complete context rather than fragmented insights. A study by McKinsey revealed that even leading firms waste 5-10% of employee time on non-value-added tasks due to poor data governance—a challenge that knowledge graphs directly address.
By representing information in an interconnected network, knowledge graphs enable decision-makers to understand the ripple effects of their choices across the organization. When a manufacturing company considers changing suppliers, for instance, the knowledge graph can instantly reveal all potential impacts on production schedules, inventory levels, and customer commitments.
The real power lies in the graphs’ ability to uncover non-obvious relationships that might be missed in traditional analysis. These hidden connections often lead to breakthrough insights that can drive competitive advantage.
Improved Data Governance and Quality
Knowledge graphs serve as powerful tools for maintaining data consistency and quality across large organizations. By creating a single source of truth, they eliminate the confusion and inefficiencies caused by conflicting definitions and duplicate information.
Real-world implementations have shown that knowledge graphs can significantly reduce the time spent on data validation and reconciliation. Organizations report spending less time questioning data accuracy and more time acting on insights.
Knowledge graphs transform raw data into actionable intelligence by providing context and relationships that make information meaningful and trustworthy.
The impact on data governance is particularly noteworthy in regulated industries. Financial institutions and healthcare providers use knowledge graphs to ensure compliance while maintaining the flexibility to adapt to changing regulatory requirements.
Advanced Analytical Capabilities
The integration of knowledge graphs with modern analytics tools has opened new frontiers in data analysis. Organizations can now perform complex queries that would be impossible with traditional database systems, enabling deeper insights and more accurate predictions.
For instance, pharmaceutical companies leverage knowledge graphs to accelerate drug discovery by analyzing relationships between chemical compounds, biological targets, and disease mechanisms. This interconnected approach has led to breakthroughs in identifying new applications for existing medications.
Perhaps most impressively, knowledge graphs enhance the accuracy of AI and machine learning models by providing them with rich contextual information. This results in more reliable predictions and recommendations, leading to better business outcomes.
Techniques for Building Robust Knowledge Graphs
Knowledge graphs have transformed how organizations structure and understand complex data relationships. By combining semantic modeling, machine learning, and data linking techniques, modern knowledge graphs create powerful frameworks for knowledge representation and reasoning.
Semantic modeling provides the foundational architecture for representing information relationships. A semantic data model acts as a schema, defining how entities and their relationships should be structured within the knowledge graph. This approach ensures that data maintains consistent meaning and context across the entire graph structure.
Data linking serves as a crucial bridge between disparate information sources. When building knowledge graphs, organizations must carefully connect related entities while maintaining data integrity. For example, modern knowledge graph platforms employ sophisticated entity resolution techniques to identify and merge duplicate entries, ensuring data consistency throughout the network.
Machine learning algorithms enhance knowledge graph construction by automating pattern recognition and relationship inference. These systems can identify hidden connections between entities, predict missing links, and validate existing relationships. This capability proves particularly valuable when dealing with large-scale datasets where manual curation becomes impractical.
The semantic layer of the knowledge graph makes it more intuitive and easy to interpret, making the analysis easy for D&A leaders. It adds depth and meaning to the data usage and content graph, allowing AI/ML algorithms to use the information for analytics and other operational use cases.
Mark Beyer, Distinguished VP Analyst at Gartner
The implementation process typically begins with data preprocessing, where raw information is cleaned and standardized. Organizations must address data quality issues like inconsistent formatting, missing values, and duplicate entries before incorporation into the knowledge graph. This foundational step ensures the resulting graph provides reliable insights.
Quality validation represents another critical aspect of knowledge graph construction. Regular audits help maintain data integrity by checking for logical consistency, relationship accuracy, and semantic coherence. This ongoing process helps identify and correct potential issues before they impact downstream applications.
Advanced knowledge graph platforms now incorporate visual debugging tools that allow developers to examine and validate relationships in real-time. This capability streamlines the construction process by making it easier to identify and resolve issues during development, rather than after deployment.
Enterprise security considerations must also factor into knowledge graph construction. Organizations need to implement robust access controls and data governance frameworks to protect sensitive information while still enabling appropriate data sharing and collaboration across teams.
By carefully combining these techniques and best practices, organizations can build knowledge graphs that serve as powerful tools for understanding and leveraging their data assets. The resulting structures provide the foundation for advanced analytics, improved decision-making, and innovative AI applications.
Applications of Knowledge Graphs in Various Industries
Knowledge graphs have transformed how organizations process and derive value from complex data relationships across diverse sectors. Their ability to map intricate connections between different data points has made them indispensable tools for modern enterprises.
In healthcare, knowledge graphs are improving patient care by connecting vital medical information in meaningful ways. For example, doctors can quickly visualize relationships between symptoms, diseases, treatments, and patient histories through comprehensive knowledge networks. A study by Mayo Clinic demonstrates how these connections enable healthcare professionals to better understand disease etiology, improve diagnoses, and discover new drug interactions.
The financial sector leverages knowledge graphs for sophisticated fraud detection and risk management. By analyzing patterns in transaction data and customer behavior, banks can identify suspicious activities that might otherwise go unnoticed. For instance, knowledge graphs help map relationships between accounts, transactions, and entities to detect coordinated fraud attempts with unprecedented accuracy.
In telecommunications, knowledge graphs assist in network optimization and customer service enhancement. Telecom providers use these systems to understand the complex relationships between network infrastructure, service quality, and customer experience. This enables them to predict potential network issues before they affect service delivery and personalize customer interactions based on comprehensive user profiles.
E-commerce platforms employ knowledge graphs to create sophisticated recommendation systems. Rather than simply suggesting products based on previous purchases, these systems understand deeper connections between products, customer preferences, and shopping behaviors. This results in more accurate and personalized shopping experiences that drive customer engagement and satisfaction.
The media and entertainment industry utilizes knowledge graphs to enhance content discovery and user engagement. Streaming services like Netflix analyze viewing patterns, genre preferences, and content relationships to provide highly personalized recommendations. These systems understand not just what content users consume, but also the underlying elements that make certain content appealing to specific audiences.
In manufacturing, knowledge graphs help optimize supply chains and production processes by mapping relationships between suppliers, materials, production schedules, and quality metrics. This enables manufacturers to identify potential bottlenecks, improve efficiency, and maintain consistent product quality across complex global operations.
The versatility of knowledge graphs extends to research and development across industries. Scientists and researchers use these tools to discover new connections in their fields, whether it’s identifying potential drug interactions in pharmaceuticals or understanding complex market dynamics in economic research.
Overcoming Challenges in Knowledge Graph Implementation
Data integration stands as perhaps the most formidable obstacle in knowledge graph implementation. Organizations frequently struggle with consolidating information from diverse sources, each with its own format, schema, and vocabulary. For instance, merging data from CRM systems, marketing platforms, and analytics tools requires careful reconciliation of different data models while maintaining semantic consistency.
Schema alignment presents another critical challenge that demands attention. When integrating multiple data sources, organizations must map heterogeneous data to a consistent model. As implementation specialists noted, translating between different schemas while preserving meaning requires sophisticated entity resolution techniques and standardized knowledge representations.
Data quality emerges as a persistent concern that can significantly impact the effectiveness of knowledge graphs. Poor data quality leads to erroneous outputs and flawed insights, potentially undermining the entire system’s utility. Organizations must implement rigorous validation processes and establish clear governance policies defining data quality standards, update frequencies, and responsibility assignments.
Scalability challenges intensify as knowledge graphs expand to handle billions of nodes and relationships while maintaining query performance. The technical complexity increases when knowledge graphs need to process real-time updates and concurrent access from multiple applications. This requires sophisticated architectural decisions and careful infrastructure planning to ensure sustainable growth.
Challenges and Solutions in Knowledge Graph Implementation
To address these challenges effectively, organizations should adopt a phased implementation approach. Start with a small dataset to experiment and validate the knowledge graph’s design. This allows quick identification of issues and understanding of the ontology’s effectiveness. Once the model proves accurate, gradually expand to incorporate the full scope of data while maintaining strict quality controls and governance standards.
Continuous monitoring and refinement of the integration process is crucial for long-term success. As new data sources emerge and existing ones evolve, the integration framework must adapt while maintaining the knowledge graph’s coherence. Regular audits help ensure integrated data remains accurate and valuable for downstream applications. The cost of technical infrastructure for a knowledge graph is minimal compared to the overwhelming cost of managing the cottage industry of silos and proprietary approaches many established organizations use.
Success in knowledge graph implementation ultimately depends on establishing clear data governance policies, investing in proper planning and architecture, and building internal expertise over time. Organizations should view implementation as an iterative journey rather than a destination, with continuous improvement and adaptation as central principles.
Future Trends and Innovations in Knowledge Graphs
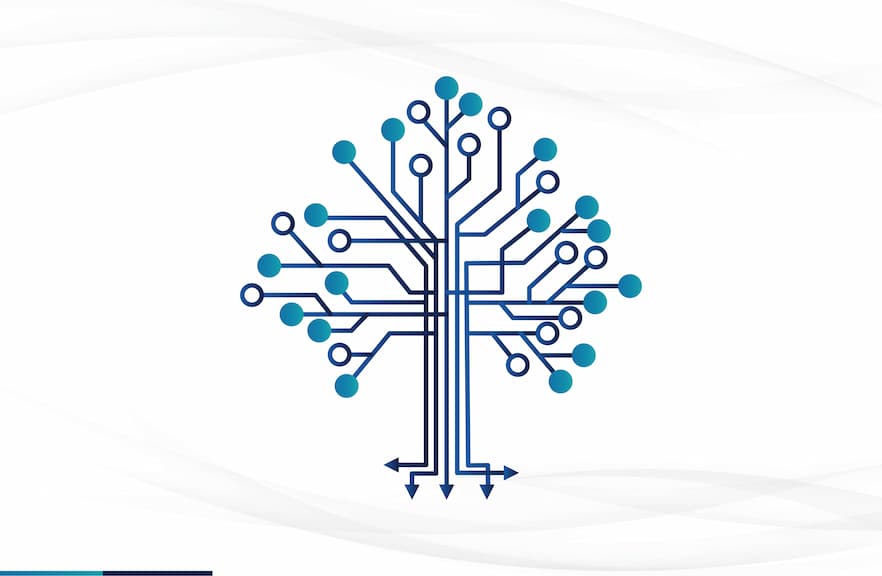
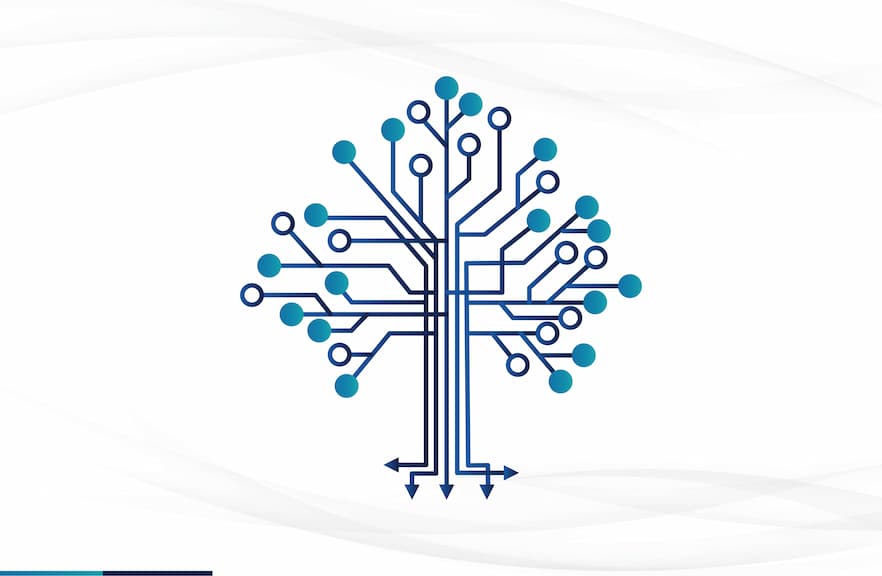
Knowledge graph technology stands at a transformative era, with innovations reshaping how organizations manage and utilize complex data relationships. Nearly half of data professionals are leveraging machine learning to extract deeper insights from their knowledge graphs.
The integration of AI and knowledge graphs represents a significant advancement. Machine learning algorithms are becoming increasingly sophisticated at building and maintaining knowledge graphs, automatically drawing connections between data points that human analysts might miss. This relationship enables more accurate predictions and enhanced decision-making capabilities across industries.
Large Language Models (LLMs) are changing how we interact with knowledge graphs. These AI systems can now leverage knowledge graphs to provide contextually accurate information with verifiable sources, marking a crucial step toward more trustworthy and explainable AI systems.
In healthcare, knowledge graphs are evolving to handle increasingly complex biomedical data relationships. These systems can now map intricate interactions between drugs, diseases, and genetic markers, enabling more precise treatment recommendations and accelerating drug discovery.
Knowledge graphs will play a crucial role in the development of the Semantic Web, enabling more meaningful and efficient data sharing and discovery across the Internet.
Hassan Chafi, Senior Director at Oracle Labs
Enterprise adoption of knowledge graphs is accelerating, particularly in manufacturing and retail sectors. Companies are using this technology to create digital twins of their operations, enabling real-time monitoring and optimization of complex systems. This trend is expected to grow as organizations seek more sophisticated ways to manage their data ecosystems.
Another emerging trend is the development of federated knowledge graphs, allowing organizations to maintain separate but interconnected knowledge bases. This approach addresses privacy concerns while enabling collaborative data sharing across organizational boundaries. Finance and healthcare industries are leading this adoption, particularly for sensitive data management.
Looking ahead, knowledge graphs will become increasingly crucial for smart city initiatives and IoT applications. By connecting vast networks of sensors and devices, these systems will enable better urban planning, traffic management, and energy optimization. The ability to process and analyze real-time data streams while maintaining complex relationship models will be essential for future smart infrastructure.
Leveraging SmythOS for Knowledge Graph Development
SmythOS transforms the traditionally complex process of knowledge graph development into an intuitive, streamlined workflow through its comprehensive visual builder interface. Unlike conventional approaches that require extensive coding expertise, SmythOS empowers both technical and non-technical teams to create sophisticated knowledge graph applications using simple drag-and-drop interactions.
The platform’s standout feature is its robust debugging environment, which provides real-time insights into knowledge graph operations. Developers can visually trace data flows, examine relationship mappings, and quickly identify potential issues, significantly reducing the time and effort typically required for troubleshooting complex graph structures. This visual debugging approach makes it easier to validate data connections and ensure proper knowledge graph construction.
Enterprise organizations benefit significantly from SmythOS’s built-in security infrastructure. The platform implements rigorous security measures to protect sensitive knowledge bases while maintaining seamless integration capabilities with existing enterprise systems. This enterprise-grade security makes SmythOS particularly valuable for organizations handling confidential information within their knowledge graphs.
SmythOS further streamlines knowledge graph development through its automated process agents. These intelligent components handle much of the heavy lifting in data ingestion and organization, automatically pulling information from various sources and establishing meaningful connections. This automation drastically reduces the potential for human error while accelerating the development process.
SmythOS isn’t just another AI tool. It’s transforming how we approach AI debugging. The future of AI development is here, and it’s visual, intuitive, and incredibly powerful.
The platform’s extensive library of reusable components accelerates knowledge graph development by providing pre-built blocks that integrate seamlessly into workflows. This allows developers to focus on customizing and optimizing their knowledge graphs rather than building basic functionality from scratch, enabling faster deployment and iteration of graph-based applications.
Through its combination of visual workflows, robust debugging tools, and enterprise security features, SmythOS creates an environment where organizations can harness the full power of knowledge graphs without getting bogged down in technical complexities. This approach makes advanced knowledge graph development accessible to a broader range of teams while maintaining the sophistication required for enterprise-scale applications.
Conclusion and Future Directions
Knowledge graphs have emerged as a transformative force in data analytics, fundamentally changing how organizations understand and utilize complex information networks. Their ability to create structured, interconnected representations of data has proven invaluable across diverse applications, from enhancing search capabilities to powering sophisticated AI decision-making processes.
The integration of knowledge graphs with emerging technologies like large language models represents a particularly promising frontier. By combining the structured nature of knowledge graphs with the natural language capabilities of LLMs, organizations can develop more sophisticated systems that better understand context and relationships within data while maintaining factual accuracy. This synthesis enables more nuanced analysis and decision-making capabilities than either technology could achieve alone.
SmythOS stands at the forefront of this evolution, offering a robust platform that simplifies knowledge graph development through its innovative visual workflow builder and comprehensive debugging capabilities. The platform’s enterprise-grade security features and seamless integration capabilities with major graph databases ensure organizations can confidently build and scale their knowledge graph implementations while protecting sensitive information.
Looking ahead, knowledge graphs will continue to evolve through several key advancements. Self-improving systems that automatically update their structure based on new information are emerging, while graph neural networks enable more sophisticated analysis of complex relationships. These developments, combined with improved standardization and interoperability frameworks, will further expand the utility and accessibility of knowledge graph technology.
As organizations continue generating exponentially more data, the need for sophisticated tools to make sense of complex information landscapes becomes increasingly critical. Knowledge graphs, supported by platforms like SmythOS, provide the foundation for meeting this challenge, enabling enterprises to extract meaningful insights and drive innovation across sectors.
Last updated:
Disclaimer: The information presented in this article is for general informational purposes only and is provided as is. While we strive to keep the content up-to-date and accurate, we make no representations or warranties of any kind, express or implied, about the completeness, accuracy, reliability, suitability, or availability of the information contained in this article.
Any reliance you place on such information is strictly at your own risk. We reserve the right to make additions, deletions, or modifications to the contents of this article at any time without prior notice.
In no event will we be liable for any loss or damage including without limitation, indirect or consequential loss or damage, or any loss or damage whatsoever arising from loss of data, profits, or any other loss not specified herein arising out of, or in connection with, the use of this article.
Despite our best efforts, this article may contain oversights, errors, or omissions. If you notice any inaccuracies or have concerns about the content, please report them through our content feedback form. Your input helps us maintain the quality and reliability of our information.