Machine Learning in Recruitment: Transforming Talent Acquisition
Picture walking into your office on Monday morning to find hundreds of job applications waiting in your inbox. Overwhelming, right? Now imagine having an intelligent assistant that could instantly identify the most promising candidates, eliminate bias from hiring decisions, and accelerate your entire recruitment process. This isn’t science fiction – it’s the reality of machine learning in modern recruitment.
Business leaders are increasingly embracing artificial intelligence to gain a competitive edge in talent acquisition. From automating repetitive tasks to uncovering insights about candidate fit, machine learning is reshaping how organizations attract, evaluate, and hire top talent.
But what exactly can machine learning do for your recruitment process? Think of it as having a tireless assistant that can screen resumes 24/7, predict which candidates are most likely to succeed in a role, and even help write more effective job descriptions. The technology is particularly transformative for companies handling high volumes of applications, where manual screening would be practically impossible.
However, this powerful technology isn’t without its challenges. While machine learning promises to make hiring faster and more objective, questions about algorithmic bias, candidate privacy, and the balance between automation and human judgment deserve careful consideration. Understanding both the potential and pitfalls of machine learning in recruitment has become essential for modern hiring professionals.
This guide explores how machine learning is revolutionizing various aspects of recruitment, examines the real benefits and risks of implementation, and shares practical strategies for leveraging this technology effectively in your hiring process. Whether you’re just starting to explore AI-powered recruitment tools or looking to optimize your existing processes, you’ll discover actionable insights to enhance your talent acquisition strategy.
Benefits of Machine Learning in Recruitment
The recruitment landscape has transformed due to machine learning technology. According to a Glassdoor study, corporate job openings receive an average of 250 applications each, overwhelming traditional manual screening processes.
Machine learning algorithms power intelligent screening systems that analyze thousands of resumes in minutes, accelerating the candidate evaluation process. By automating repetitive tasks like resume screening and initial outreach, recruiters can focus on strategic priorities like relationship building and cultural fit assessment.
AI-powered recruitment tools help reduce unconscious bias in hiring. Instead of relying on subjective human judgments influenced by factors like candidate names or educational backgrounds, these systems evaluate prospects based on skills, experience, and job-relevant qualifications. This objectivity promotes more inclusive and equitable hiring practices.
The benefits extend to candidate matching as well. Advanced algorithms can analyze not just keywords but the context and meaning in resumes and job descriptions. This semantic understanding enables more accurate matching between open roles and qualified candidates. The system can even predict which candidates are most likely to succeed in specific positions based on historical hiring data patterns.
Machine learning promises to accelerate every stage of the recruitment cycle, quickly writing job descriptions, screening resumes, assessing candidates, scheduling assessments and interviews, and making informed hiring decisions.
Vervoe Research Report
For recruiters overwhelmed by applications, machine learning delivers efficiency. The technology can pre-screen candidates, handle routine communications, and surface the most promising prospects while maintaining consistency and reducing bias. This allows talent acquisition teams to build high-performing, diverse organizations more effectively.
Application of Machine Learning in Recruitment Processes
The recruitment landscape has transformed dramatically with machine learning technologies streamlining previously time-consuming processes. From initial job postings to final candidate selection, AI-powered solutions are enhancing how organizations attract and evaluate talent.
Resume screening, traditionally one of the most labor-intensive aspects of recruitment, has been particularly enhanced by machine learning algorithms. For example, one study found that machine learning tools can reduce resume screening time by up to 70% while improving the quality of candidate matches. These systems go beyond simple keyword matching to understand context and identify qualified candidates who might be overlooked by traditional methods.
In job advertising, machine learning algorithms analyze vast amounts of data to optimize job postings and target them to the most relevant candidates. The technology considers factors like past successful hires, candidate engagement rates, and market trends to refine ad placement and content. This targeted approach not only improves the quality of applications but also reduces recruitment marketing costs.
Candidate assessment has also been transformed through AI-driven solutions. Rather than relying solely on subjective human judgment, machine learning tools can evaluate candidates’ skills, experience, and potential fit through sophisticated pattern recognition. This data-driven approach helps eliminate unconscious bias and ensures more consistent evaluation across all applicants.
Personalized outreach has taken on new dimensions with machine learning capabilities. AI systems can analyze candidate profiles and interaction data to craft customized messages that resonate with specific talent pools. This level of personalization has proven particularly effective in engaging passive candidates who might not actively be seeking new opportunities.
Machine learning algorithms often learn what hiring success looks like from historical recruitment data, which means organizations must carefully monitor for potential biases in their training data.
Laura Ross, Recruitment Technology Specialist
Workforce planning has become more precise and predictive thanks to machine learning. These systems analyze historical employee data, market trends, and business forecasts to help organizations anticipate future talent needs. This proactive approach enables companies to build talent pipelines before critical gaps emerge.
Application | Description |
---|---|
Job Advertising | Analyzes data to optimize job postings and target relevant candidates, improving application quality and reducing costs. |
Resume Screening | Automates the analysis of resumes, reducing screening time by up to 70% and improving candidate match quality. |
Candidate Assessment | Evaluates skills, experience, and potential fit through pattern recognition, reducing bias and ensuring consistent evaluations. |
Candidate Engagement | Uses AI to personalize outreach and communication, improving engagement with passive candidates and enhancing the candidate experience. |
Workforce Planning | Analyzes historical data and market trends to predict future talent needs, enabling proactive talent pipeline building. |
However, it’s crucial to note that while machine learning brings powerful capabilities to recruitment, human oversight remains essential. The most successful implementations combine AI efficiency with human expertise, creating a balanced approach that leverages technology while maintaining the personal touch necessary in recruitment.
Overcoming Challenges and Risks
Machine learning enhances recruitment, but it faces significant challenges that require careful consideration. Addressing these issues ensures AI remains a valuable tool in hiring rather than a source of problems.
Data bias is a major concern when implementing AI in recruitment. Amazon’s experimental AI hiring tool showed bias against women candidates due to historical hiring data reflecting past discrimination. To combat this, organizations must prioritize diverse training data and regular algorithm audits to prevent perpetuating existing prejudices.
According to Workable’s AI in Hiring survey, 40% of hiring team managers identify bias as a major challenge when using AI in recruitment, while 37.2% express concerns about data privacy. These statistics underscore the importance of implementing robust safeguards.
The lack of human judgment presents another significant challenge. While AI excels at processing vast amounts of data, it struggles to evaluate soft skills, cultural fit, and interpersonal dynamics. Organizations must maintain a balanced approach where AI augments rather than replaces human decision-making. This hybrid model allows recruiters to leverage AI’s efficiency while preserving the crucial human elements of hiring.
Privacy concerns loom large as AI systems collect and analyze extensive candidate data. Organizations must establish clear data protection policies that comply with regulations like GDPR and maintain transparency about how candidate information is used. Regular audits of data handling practices help ensure ongoing compliance and build trust with candidates.
Implementing Best Practices for Fair AI Hiring
To ensure ethical and effective use of machine learning in recruitment, organizations should implement several key strategies. First, establish clear guidelines for AI implementation, including regular bias testing and validation of algorithms against diverse candidate pools.
Creating a DEI (Diversity, Equity, and Inclusion) dashboard helps monitor the impact of AI on hiring decisions. This tool tracks key metrics like gender and ethnic diversity in candidate selection, allowing organizations to identify and address any emerging patterns of bias quickly.
Regular assessment of AI systems proves crucial for maintaining fairness. Organizations should conduct quarterly audits of their AI recruitment tools, analyzing outcomes to ensure they align with diversity goals and ethical hiring practices. This proactive approach helps catch and correct potential issues before they impact hiring decisions.
External expert involvement adds another layer of accountability. Independent auditors can provide unbiased evaluations of AI systems and suggest improvements. Their outside perspective often identifies blind spots that internal teams might miss.
The one strategy that helped us best reduce the impact of AI bias when hiring new candidates was implementing quarterly audits and reviews of our AI systems. The results have been impressive because we have increased the number of diverse hires.
John Xie, CEO at Taskade
Transparency in AI decision-making processes builds trust with candidates and helps ensure fairness. Organizations should clearly communicate how AI tools are used in their hiring process and provide candidates with information about how their data will be handled and protected.
Best Practices for Implementing Machine Learning in Recruitment
The transformative power of machine learning in recruitment brings both opportunities and responsibilities. Modern hiring teams are discovering that successful implementation requires a careful balance of technological innovation and human wisdom. Here are essential practices to ensure ML systems enhance rather than compromise the hiring process.
Building Representative Data Foundations
The quality of machine learning outputs directly reflects the data used to train these systems. Organizations must prioritize using diverse, comprehensive data sources that represent all qualified candidate segments. This means gathering recruitment data from varied channels, industries, and demographic groups to prevent algorithmic bias.
According to a recent industry analysis, AI systems are only as unbiased as the data they’re trained on. Historical hiring data often contains inherent biases that can skew results if not carefully curated. Smart organizations actively audit their training data sets to identify and remove potential sources of discrimination.
Consider supplementing traditional recruitment data with information from multiple sources like skills assessments, behavioral interviews, and performance metrics. This creates a more holistic view of candidate potential while reducing reliance on potentially biased historical patterns.
Regular data quality assessments help ensure the system continues to make fair, accurate recommendations as your talent pool evolves. This might involve quarterly audits of hiring outcomes and periodic reviews of data collection methods.
The most successful implementations typically establish clear data governance frameworks that specify how recruitment information should be collected, validated, and maintained over time.
Component | Description |
---|---|
Data Policies and Standards | Regulations governing data collection, storage, management, and usage within the organization. Ensures consistency and compliance across data-related activities. |
Data Stewardship | Assigns responsibility for specific data sets to individuals who ensure accuracy, integrity, and proper usage of data. |
Data Quality Management | Processes like data validation, cleansing, and regular audits to maintain high data quality standards. |
Data Security and Privacy | Measures to protect sensitive information from unauthorized access and ensure compliance with privacy regulations. |
Data Lifecycle Management | Oversees data from its creation to disposal, ensuring proper management at every stage. |
Continuous Algorithm Refinement
Machine learning systems require ongoing optimization to remain effective. Smart organizations treat their recruitment algorithms as living systems needing regular testing, monitoring, and refinement based on real-world results.
Start by establishing clear performance metrics that align with your hiring goals. Track key indicators like time-to-hire, quality of hire, and diversity outcomes to gauge algorithm effectiveness. Use these insights to make targeted improvements to the system.
Pay special attention to edge cases and unexpected results that could indicate potential blind spots in your algorithms. Sometimes the most valuable insights come from examining why the system made unusual recommendations.
Remember that refinement isn’t just about technical optimization. It’s equally important to ensure the algorithms continue to reflect your organization’s evolving hiring criteria and values.
Create feedback loops between hiring managers, recruiters, and your ML team to capture qualitative insights about system performance. This human perspective is invaluable for identifying areas needing adjustment.
Maintaining Human Oversight
While machine learning can dramatically improve recruitment efficiency, human judgment remains essential. The most effective implementations establish clear processes for human oversight and intervention throughout the hiring journey.
The biggest ethical challenge in AI recruitment is ensuring that it doesn’t perpetuate biases. AI is not made the sole decision-maker. It’s part of a broader, human-led process.
Zephyr Chan, Founder and Growth Marketer, Better Marketer
Define specific checkpoints where human recruiters should review and validate ML recommendations. This might include final candidate selections, compensation decisions, or situations where the system flags unusual patterns.
Train your recruitment team to effectively collaborate with ML systems. Help them understand both the capabilities and limitations of the technology so they can provide meaningful oversight.
Establish clear escalation paths for situations where human judgment determines an algorithm’s recommendation may be incorrect or biased. The ability to thoughtfully override automated decisions is crucial for maintaining hiring quality.
Remember that the goal is to augment, not replace, human expertise. The most successful implementations find ways to combine the pattern-recognition capabilities of ML with the nuanced understanding that only human recruiters can provide.
Future Trends in Machine Learning for Recruitment
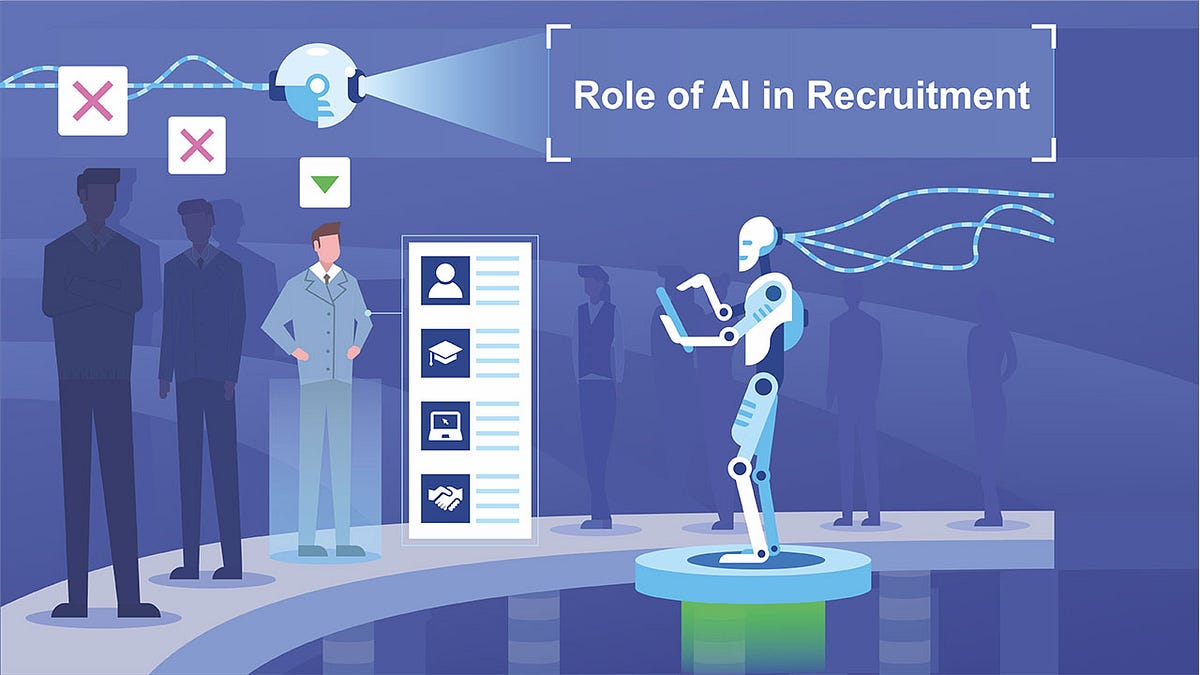
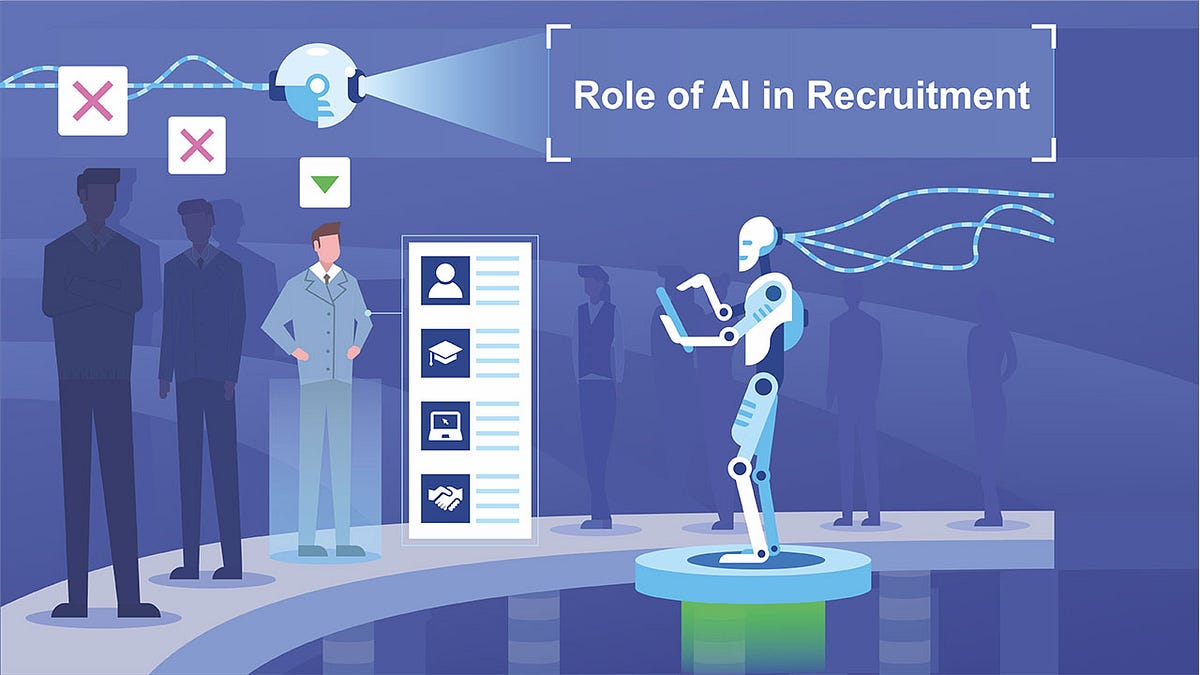
The recruitment landscape is transforming with artificial intelligence and machine learning innovations. According to McKinsey’s 2024 Technology Outlook, investment in AI recruitment technologies surged sevenfold in 2023, signaling a fundamental shift in how organizations discover and engage talent.
No-code machine learning platforms are democratizing access to sophisticated recruitment tools, allowing HR teams to harness AI without extensive technical expertise. These interfaces enable recruiters to build and deploy predictive models that assess candidate fit, forecast retention rates, and automate repetitive screening tasks – all without writing a single line of code.
Predictive analytics is revolutionizing hiring decisions. By analyzing vast amounts of historical recruitment data, systems can now forecast a candidate’s likelihood of success, cultural fit, and long-term potential. This data-driven approach helps eliminate gut-based decisions while significantly reducing time-to-hire and improving quality of hire.
AI is transforming candidate engagement through hyper-personalization. Smart chatbots engage candidates 24/7, answering questions and providing updates throughout the recruitment journey. AI-powered tools analyze candidate behavior patterns to deliver tailored content and job recommendations, creating a more engaging and candidate-centric experience.
The integration of machine learning in recruitment isn’t just about automation – it’s about augmentation. As John Vlastelica, CEO at Recruiting Toolbox notes, The best recruiters must be great at building relationships and creating high-touch candidate experiences that will help them rise above the bots.
The human element remains critical, with AI handling repetitive tasks while recruiters focus on building meaningful connections.
Looking ahead, we can expect even more sophisticated AI applications in recruitment, from sentiment analysis during video interviews to predictive models that gauge cultural alignment through natural language processing. The future of recruitment is increasingly automated but also more human-centered and personalized than ever before.
Conclusion: Leveraging Machine Learning for Recruitment Success
The transformative impact of machine learning on modern recruitment cannot be understated. By automating critical processes like resume screening and candidate matching, organizations are discovering unprecedented efficiency gains while expanding their talent pools.
The data-driven approach enabled by AI not only accelerates hiring timelines but also helps eliminate unconscious biases that have historically limited workplace diversity. What makes this technological shift particularly compelling is its ability to handle high-volume recruitment while maintaining personalized candidate experiences.
As research has demonstrated, machine learning tools can simultaneously evaluate thousands of applications while ensuring each candidate receives fair and thorough consideration – a feat impossible through traditional methods.
Most importantly, AI-powered solutions are proving to be instrumental in creating more inclusive workplaces. By emphasizing skills and qualifications rather than demographic factors, machine learning helps organizations tap into diverse talent pools that they may have previously overlooked. This increased objectivity in candidate evaluation marks a significant advancement in establishing equitable hiring practices.
For companies looking to modernize their recruitment processes, SmythOS provides a comprehensive suite of machine learning tools designed to streamline hiring. With robust features for automated screening, intelligent candidate matching, and bias mitigation, organizations can transform their recruitment strategies while still maintaining the essential human touch in hiring decisions.
The future of recruitment lies in the thoughtful integration of machine learning technologies. By embracing these innovations today, organizations can build stronger, more diverse teams while significantly reducing time-to-hire and recruitment costs.
Last updated:
Disclaimer: The information presented in this article is for general informational purposes only and is provided as is. While we strive to keep the content up-to-date and accurate, we make no representations or warranties of any kind, express or implied, about the completeness, accuracy, reliability, suitability, or availability of the information contained in this article.
Any reliance you place on such information is strictly at your own risk. We reserve the right to make additions, deletions, or modifications to the contents of this article at any time without prior notice.
In no event will we be liable for any loss or damage including without limitation, indirect or consequential loss or damage, or any loss or damage whatsoever arising from loss of data, profits, or any other loss not specified herein arising out of, or in connection with, the use of this article.
Despite our best efforts, this article may contain oversights, errors, or omissions. If you notice any inaccuracies or have concerns about the content, please report them through our content feedback form. Your input helps us maintain the quality and reliability of our information.