Symbolic AI and AI Ethics: Ensuring Transparency and Accountability in Intelligent Systems
Artificial intelligence increasingly shapes critical decisions in healthcare and finance. Symbolic AI emerges as a promising approach to ensure ethical and transparent machine reasoning. By combining human-like logic with explicit rules, Symbolic AI provides a framework where AI systems can make decisions that are both powerful and accountable.
Unlike traditional black-box AI systems, Symbolic AI follows clearly defined logical rules that humans can understand and verify. This transparency proves invaluable in high-stakes environments like healthcare, where AI assists in critical tasks like drug discovery and treatment planning. When doctors and patients can understand how an AI system arrived at its recommendations, it builds the trust necessary for responsible adoption.
In the financial sector, where biased algorithms could unfairly deny loans or investment opportunities, Symbolic AI’s explicit reasoning helps identify and eliminate discriminatory patterns. By encoding ethical principles directly into the system’s rule set, organizations can ensure their AI makes decisions that are not only profitable but also fair and socially responsible.
However, implementing Symbolic AI comes with its own set of challenges. As AI systems grow more complex, balancing detailed logical rules with efficient processing becomes increasingly difficult. Additionally, organizations must carefully consider which ethical principles to encode and how to handle scenarios where different moral guidelines conflict.
The future of AI ethics likely lies in hybrid approaches that combine Symbolic AI’s transparency with the pattern-recognition capabilities of machine learning. By building systems that can both learn from data and follow explicit ethical guidelines, we can work toward AI that serves humanity’s needs while upholding our values.
Understanding Symbolic AI
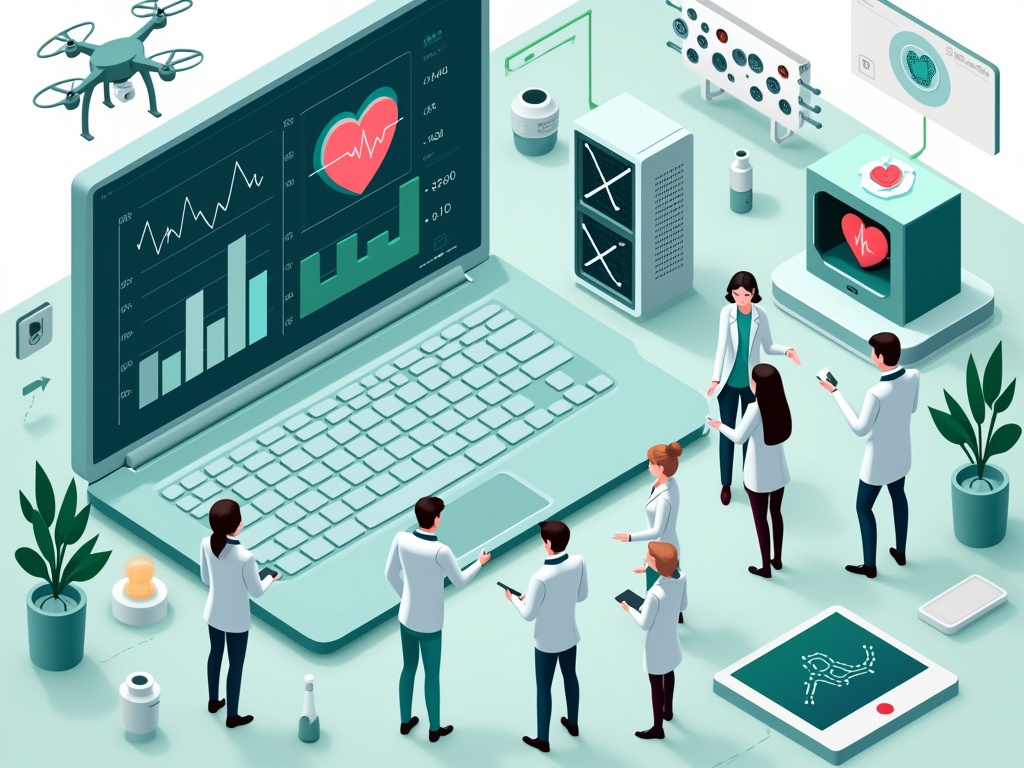
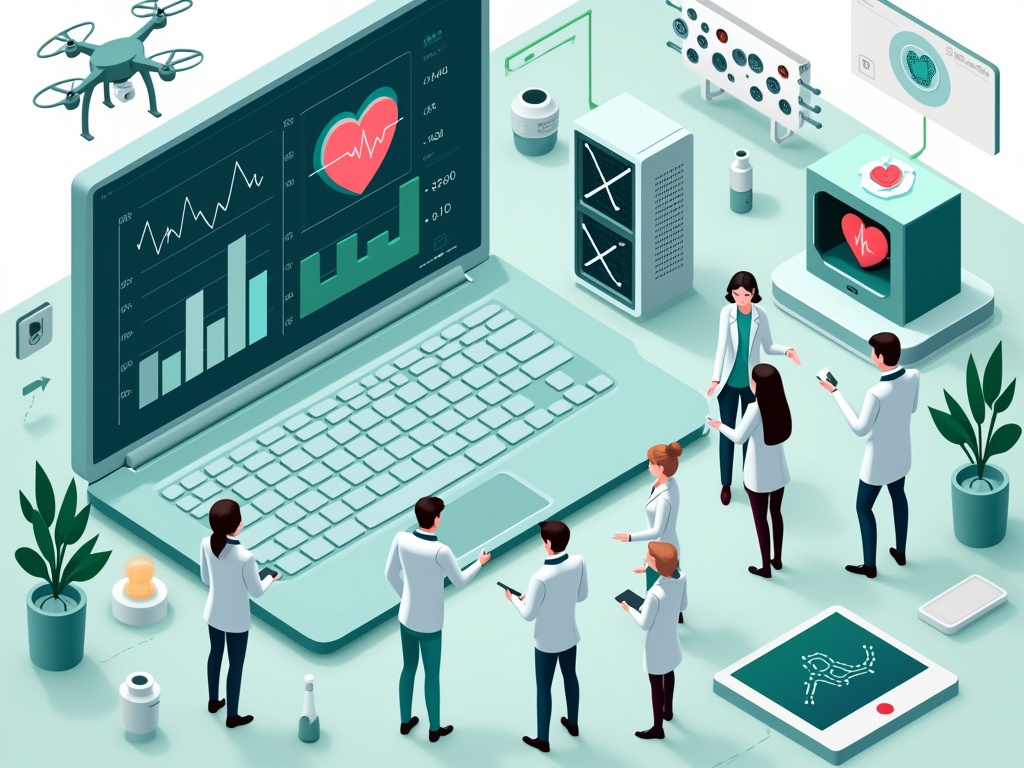
Symbolic AI represents a foundational approach to artificial intelligence that mirrors human reasoning by manipulating symbols and rules to solve complex problems. Unlike modern machine learning systems that learn from massive datasets, symbolic AI operates more like a meticulous librarian, carefully organizing and applying logical rules to reach conclusions.
At its core, symbolic AI excels at structured problem-solving through explicit knowledge representation. Picture a doctor’s diagnostic process: they consider symptoms, medical history, and established medical protocols to reach a diagnosis. Similarly, symbolic AI in healthcare uses carefully crafted rules and decision trees to help physicians make diagnostic decisions. For example, an expert system might follow logic like: ‘IF the patient has fever AND persistent cough AND difficulty breathing, THEN evaluate for pneumonia.’
The legal field offers another compelling example of symbolic AI’s strengths. Legal expert systems can navigate vast databases of case law and statutes using predefined rules that mirror legal reasoning. When attorneys need to research precedents for a complex case, these systems can systematically apply legal principles to identify relevant prior decisions, much like an experienced lawyer would, but at a much faster pace.
However, symbolic AI faces significant limitations that have influenced its evolution. Symbolic AI struggles to adapt to novel situations outside its predefined rule set, much like how a human expert can’t instantly master a completely new field. The approach also faces scalability challenges; as knowledge bases grow larger, managing and updating the complex web of rules becomes increasingly difficult.
Modern applications often use symbolic AI alongside other approaches to leverage its unique strengths while compensating for its limitations. For instance, some medical diagnostic systems combine symbolic AI’s logical reasoning with machine learning’s pattern recognition to provide more comprehensive analysis. This hybrid approach maintains the transparency and interpretability of symbolic reasoning while adding flexibility to handle less structured information.
What makes symbolic AI particularly valuable in critical domains like healthcare and law is its transparency. Unlike ‘black box’ AI systems, every step of symbolic AI’s reasoning process can be traced and verified. When a medical expert system recommends a diagnosis, it can explain its logic in terms that healthcare professionals can understand and validate.
Ethical Challenges in Symbolic AI
Symbolic AI systems offer transparency through their explicitly encoded rules and logical structures but face critical ethical challenges. Manual rule encoding introduces potential biases that can significantly impact decision-making processes.
Inherent bias in rule creation is a primary concern. When developers and domain experts encode knowledge and rules into symbolic AI systems, their unconscious biases, cultural assumptions, and limited perspectives can become embedded in the system’s decision-making framework. For instance, a symbolic AI system for medical diagnosis programmed primarily using research data from male patients may fail to recognize symptoms that present differently in female patients.
Transparency, often touted as a key advantage of symbolic AI, presents its own paradox. As noted in a recent study, complex logical structures in these systems can become so intricate that they create their own form of opacity. When rule systems expand to handle real-world complexity, the interaction between thousands of rules can create emergent behaviors that are difficult to predict or explain, despite each individual rule being technically transparent.
Accountability is another significant challenge. While symbolic AI’s decision-making process can be traced through its rule chains, assigning responsibility when errors occur becomes complicated. Should accountability lie with the experts who defined the rules, the developers who implemented them, or the organizations deploying these systems? This question is particularly crucial in high-stakes applications like autonomous vehicles or criminal justice, where decisions can have life-altering consequences.
Ensuring fairness in symbolic AI systems requires constant vigilance and regular auditing of encoded rules. Organizations must implement robust testing frameworks to identify potential biases and assess the impact of rule interactions on different demographic groups. This includes creating diverse teams for rule development and incorporating feedback from affected communities to ensure the system serves all users equitably.
The path forward requires balancing symbolic AI’s inherent interpretability while guarding against its ethical pitfalls. This involves developing comprehensive frameworks for bias testing, establishing clear chains of accountability, and ensuring that the complexity of rule systems doesn’t undermine their intended transparency.
Looking ahead, the ethical deployment of symbolic AI systems will require ongoing collaboration between technologists, ethicists, and domain experts to develop guidelines that promote fairness while maintaining the powerful reasoning capabilities these systems offer. The challenge lies not just in creating effective AI but in ensuring it serves society equitably and transparently.
Hybrid AI Approaches
Hybrid AI represents a fusion of symbolic AI’s rule-based reasoning with machine learning’s adaptive capabilities. By combining these approaches, organizations can develop more robust AI systems that overcome the limitations of using either method alone. Research indicates this synergy delivers superior results across various applications, from medical diagnosis to financial analysis.
The power of hybrid AI lies in its ability to leverage symbolic AI’s explicit rules and logical reasoning while incorporating machine learning’s pattern recognition and adaptability. For example, in a medical diagnosis system, symbolic AI can apply established medical guidelines and protocols, while machine learning algorithms analyze patient data to identify subtle patterns that might escape traditional rule-based systems. This combination enables more accurate and reliable diagnoses than either approach could achieve independently.
One of the most compelling advantages of hybrid AI is its enhanced explainability. While pure machine learning models often operate as black boxes, making it difficult to understand their decision-making process, hybrid systems maintain transparency through their symbolic AI components. This transparency is crucial in regulated industries like healthcare and finance, where understanding how AI reaches its conclusions is essential for compliance and trust.
The flexibility of hybrid AI systems also sets them apart. Machine learning components can continuously adapt and improve based on new data, while symbolic AI ensures adherence to fundamental rules and constraints. This dynamic interplay creates systems that can evolve while maintaining reliable performance boundaries. For instance, in autonomous vehicles, symbolic AI handles traffic rules and safety protocols, while machine learning manages complex real-time driving decisions.
Efficiency gains represent another significant benefit of hybrid approaches. By combining rule-based processing with machine learning, systems can optimize resource utilization – applying computationally intensive machine learning only when needed while using faster symbolic processing for straightforward tasks. This strategic resource allocation leads to more cost-effective and responsive AI solutions.
Advantages and Applications of Hybrid AI
Applications in Healthcare and Legal Systems
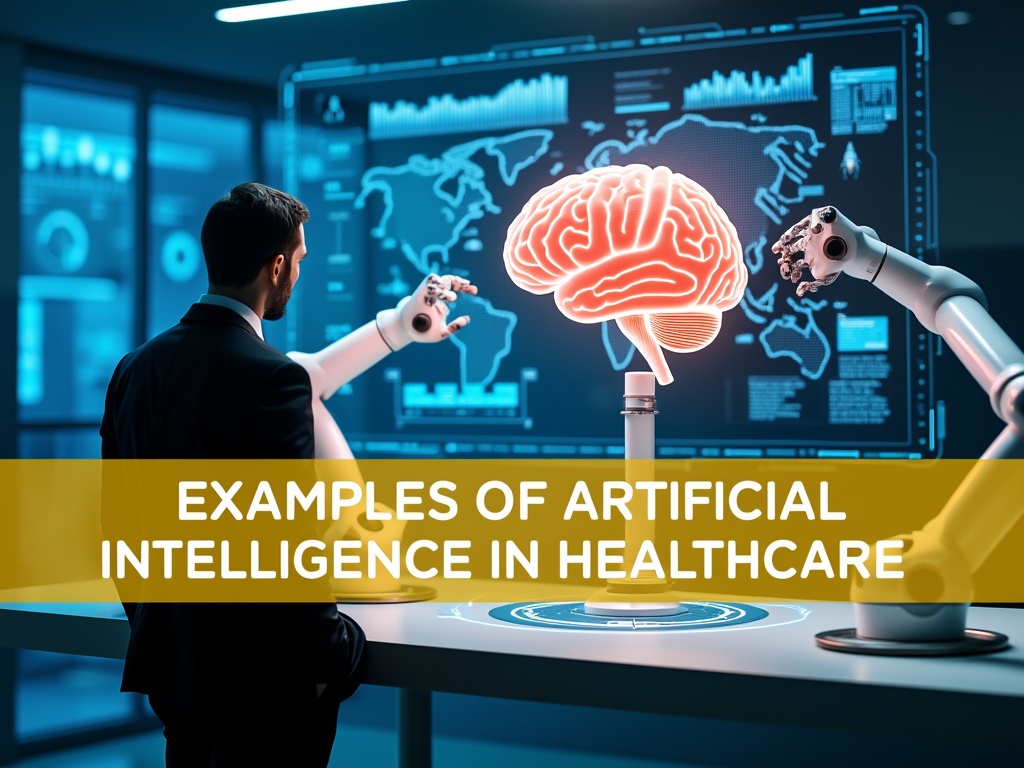
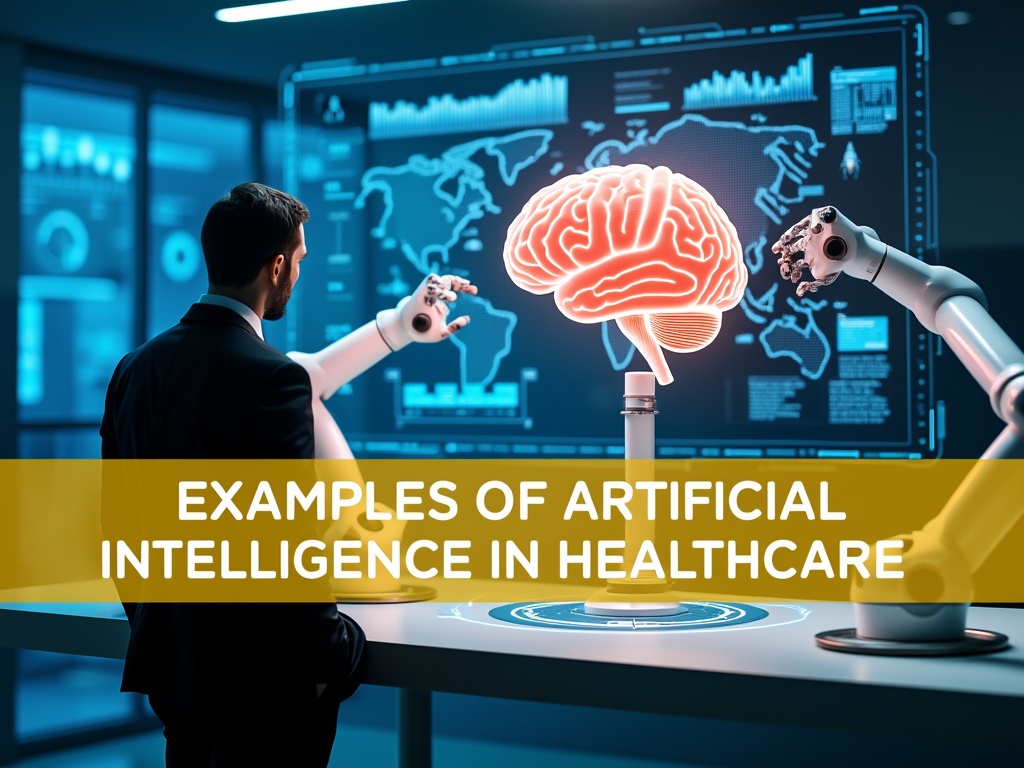
AI in healthcare: professional with digital brain.
Symbolic AI has emerged as a transformative force in both healthcare and legal sectors, fundamentally changing how medical diagnoses are made and legal cases are analyzed. These rule-based systems are bringing unprecedented precision and efficiency to critical decision-making processes.
In healthcare settings, symbolic AI serves as a powerful diagnostic assistant to medical professionals. For example, according to Cleveland Clinic research, diagnostic AI systems can now analyze medical imaging with remarkable accuracy, helping radiologists identify potential cancers and other conditions with greater precision. These systems work by applying rule-based logic to match patient symptoms and test results against vast databases of medical knowledge, significantly reducing the risk of misdiagnosis.
The impact of these AI systems extends beyond simple pattern matching. Modern healthcare AI can process complex combinations of symptoms, patient history, and diagnostic test results simultaneously—something that would take human doctors considerable time to analyze manually. This capability is particularly valuable in emergency medicine, where quick and accurate diagnoses can make the difference between life and death.
In the legal domain, symbolic AI is revolutionizing how lawyers and legal professionals analyze cases and predict outcomes. These systems can process thousands of legal documents and previous case decisions to identify relevant precedents and potential case outcomes. Studies have shown that AI systems can predict Supreme Court decisions with up to 70% accuracy by analyzing patterns in historical case data and legal precedents.
Legal AI systems excel at identifying subtle patterns across vast amounts of case law that might escape human notice. They can analyze decades of legal decisions to identify trends in judicial reasoning, helping lawyers build stronger arguments and make more informed decisions about litigation strategy. This capability is particularly valuable in complex areas of law where precedents may span multiple jurisdictions and decades.
AI is no longer just an interesting idea, but it’s being used in a real-life setting. Today, there’s a decent chance a computer can read an MRI or an X-ray better than a human.
Dr. Rohit Chandra, Chief Digital Officer, Cleveland Clinic
While these AI systems are powerful tools, they are designed to augment rather than replace human expertise. In both healthcare and legal applications, human professionals remain essential for interpreting AI insights, considering contextual factors, and making final decisions. The combination of human expertise and AI capabilities is creating more efficient and accurate systems in both fields.
Leveraging SmythOS for Enhanced AI Development
The landscape of artificial intelligence development demands powerful yet accessible tools that simplify complex processes. SmythOS emerges as a transformative platform, particularly in the realm of symbolic AI development, where precision and clarity are paramount.
At its core, SmythOS enhances the development process through its intuitive visual design interface. Instead of dealing with abstract code, developers can map out logical flows and reasoning patterns visually, similar to crafting a flowchart. This visual approach reduces the learning curve while enabling more sophisticated AI architectures. The platform’s drag-and-drop functionality allows even those new to symbolic AI to start building functional systems quickly.
One of SmythOS’s standout features is its comprehensive debugging environment. Traditional AI development often struggles with identifying and fixing logical errors, but SmythOS’s built-in debugging tools illuminate the entire reasoning process. Developers can trace the exact path of logic flows, pinpoint where rules might conflict, and optimize decision pathways with unprecedented clarity.
Integration capabilities set SmythOS apart in the crowded AI development space. The platform seamlessly connects with existing rule systems, allowing organizations to preserve their current investments while expanding their AI capabilities. This interoperability means businesses can gradually transition to more advanced symbolic AI without disrupting their operations.
SmythOS excels in scaling AI solutions through its efficient resource management and deployment options. The platform handles the complex task of resource allocation automatically, ensuring that symbolic AI systems remain responsive even as they grow in complexity. Whether scaling up to handle increased workloads or expanding to new use cases, SmythOS maintains consistent performance without requiring extensive manual optimization.
SmythOS is not just a tool; it’s a catalyst for innovation in multi-agent systems. Its visual builder and intuitive features empower developers to bring their most ambitious AI projects to life.
AI Development Expert at SmythOS
For enterprises looking to harness the power of symbolic AI, SmythOS provides the ideal balance of sophistication and accessibility. Its combination of visual tools, robust debugging capabilities, and seamless integration options makes it possible to develop and deploy AI solutions that drive real business value. As organizations continue to explore the possibilities of symbolic AI, SmythOS stands ready to transform complex concepts into practical, scalable solutions.
Conclusion on Symbolic AI and Ethics
The ethical dimensions of symbolic AI development require proactive measures to ensure responsible implementation. As recent research demonstrates, ethical principles must be integrated into AI systems from their inception rather than as an afterthought. The industry faces challenges in maintaining transparency, mitigating biases, and upholding ethical standards throughout the development process.
The path forward demands a focus on enhancing system transparency, allowing stakeholders to understand and validate AI decision-making processes. This transparency builds trust and enables the identification and correction of potential biases before they impact real-world applications. By making AI systems more interpretable, developers can ensure their creations align with human values and societal expectations.
SmythOS emerges as a valuable platform in this landscape, offering tools specifically designed to address these ethical considerations. Through its comprehensive framework, developers can implement robust safeguards while maintaining the flexibility needed for innovation. The platform’s approach to ethical AI development demonstrates how technological advancement and moral responsibility can coexist harmoniously.
As we look to the future of symbolic AI, the emphasis must remain on creating systems that perform effectively while operating within clear ethical boundaries. By leveraging platforms like SmythOS and maintaining a commitment to ethical principles, the industry can foster the development of AI systems that benefit society while minimizing potential harms.
The journey toward truly ethical AI systems is ongoing, but with dedicated focus on transparency, bias reduction, and integrated ethical frameworks, we can build a future where artificial intelligence serves humanity’s best interests while respecting our fundamental values and rights.
Last updated:
Disclaimer: The information presented in this article is for general informational purposes only and is provided as is. While we strive to keep the content up-to-date and accurate, we make no representations or warranties of any kind, express or implied, about the completeness, accuracy, reliability, suitability, or availability of the information contained in this article.
Any reliance you place on such information is strictly at your own risk. We reserve the right to make additions, deletions, or modifications to the contents of this article at any time without prior notice.
In no event will we be liable for any loss or damage including without limitation, indirect or consequential loss or damage, or any loss or damage whatsoever arising from loss of data, profits, or any other loss not specified herein arising out of, or in connection with, the use of this article.
Despite our best efforts, this article may contain oversights, errors, or omissions. If you notice any inaccuracies or have concerns about the content, please report them through our content feedback form. Your input helps us maintain the quality and reliability of our information.