Explainable AI in Finance: Driving Transparency and Accountability in Financial Services
What if you were denied a loan and couldn’t understand why? This scenario highlights a critical challenge in modern finance: the ‘black box’ problem of artificial intelligence.
Explainable AI (XAI) has emerged as a crucial solution for financial institutions grappling with the opacity of machine learning models. As recent research indicates, understanding the reasoning behind specific outcomes of complex financial models is essential for improving risk assessment and building a more resilient financial ecosystem.
Picture a traditional black-box AI model as a sealed vault—data goes in, decisions come out, but the process remains hidden. This lack of transparency poses significant challenges for banks, regulators, and customers alike. How can we trust decisions affecting millions of people’s financial lives without understanding their underlying logic?
XAI provides crucial insights into how AI systems evaluate risks and make determinations. For example, instead of simply rejecting a loan application, an XAI-powered system can explain which specific factors influenced the decision, making the process transparent and actionable.
The stakes are high. With financial institutions increasingly relying on AI for critical decisions—from risk management to fraud detection—the ability to explain and justify these decisions is becoming essential for regulatory compliance and maintaining customer trust. More than a technological advancement, XAI represents a fundamental shift toward more accountable and transparent financial services.
Challenges of Implementing XAI in Financial Systems
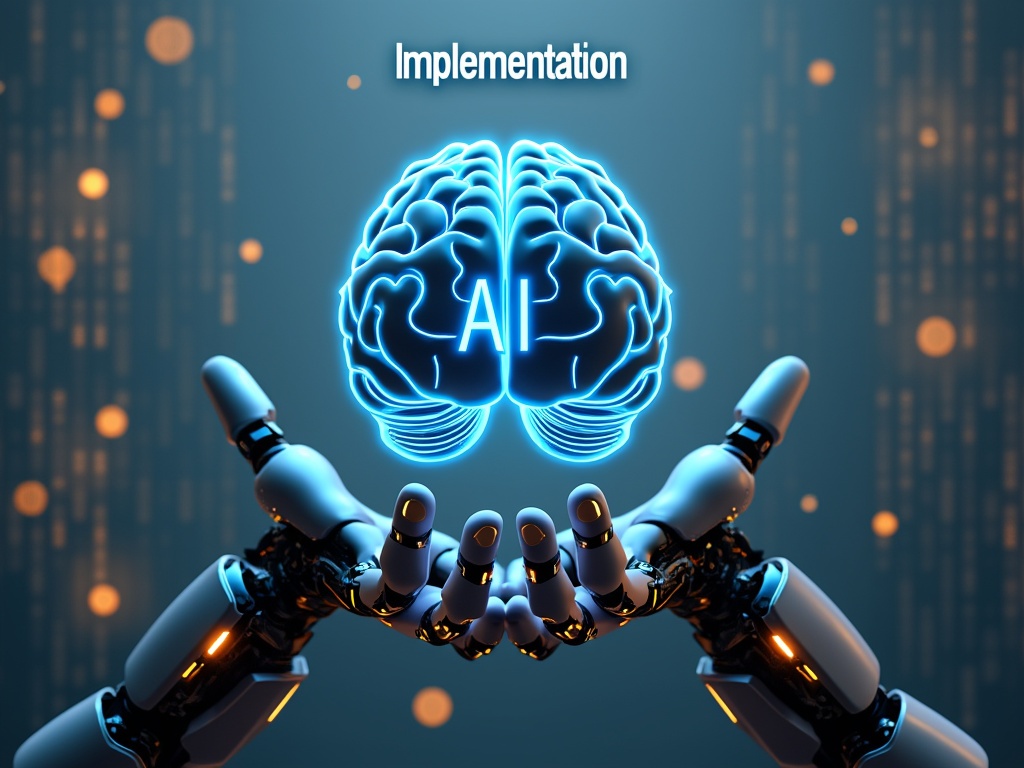
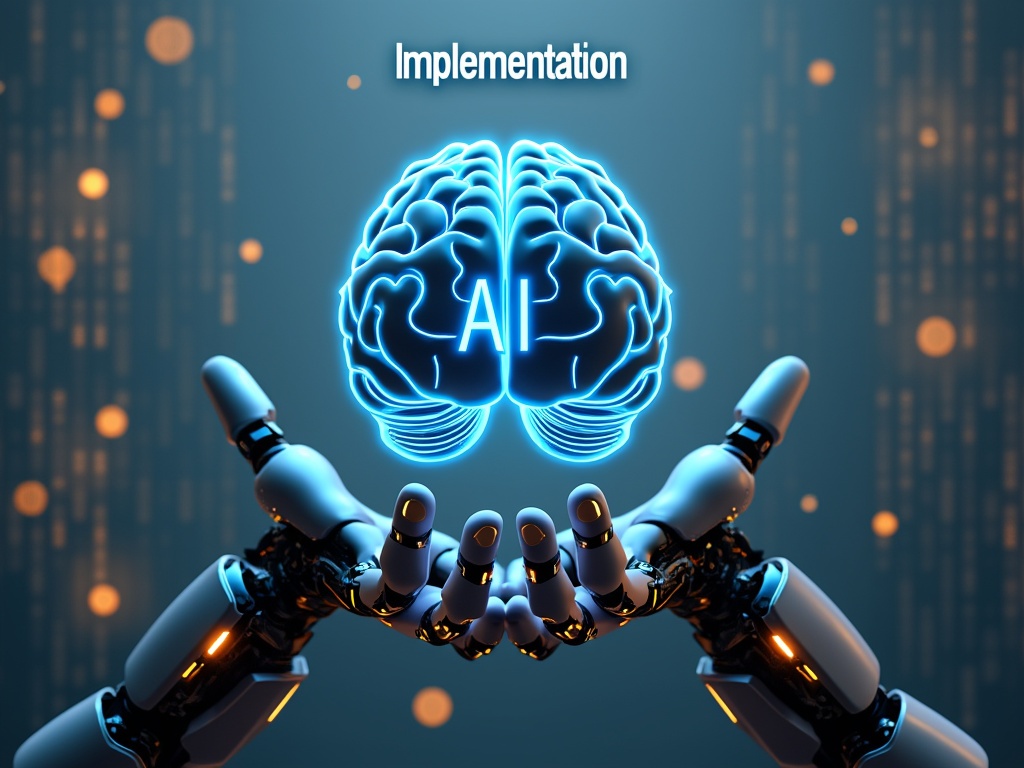
Financial institutions confront significant hurdles when implementing explainable AI (XAI) systems, as they must navigate the intricate balance between algorithmic sophistication and interpretability. At the core of these challenges lies the complexity of financial data—from diverse transaction patterns to intricate market indicators—which demands advanced modeling techniques while maintaining transparency.
One of the primary obstacles financial organizations face is managing the inherent tension between model performance and explainability. Research indicates that more sophisticated AI models often deliver superior accuracy but become increasingly opaque in their decision-making processes. This creates a paradox where the most powerful models may be the least explainable, forcing institutions to carefully weigh the tradeoffs between predictive power and transparency.
Regulatory compliance presents another formidable challenge. Financial institutions must ensure their XAI systems align with various regulatory frameworks while providing clear justification for automated decisions. This is particularly crucial in critical areas such as credit scoring, risk assessment, and fraud detection, where algorithmic decisions can significantly impact customers’ lives.
Data quality and bias mitigation are additional critical concerns. Financial institutions must ensure their XAI models are trained on representative, unbiased datasets while maintaining the ability to explain how these models handle potential biases. This requires robust data governance frameworks and continuous monitoring of model outputs to detect and address any emergent biases.
Technical integration challenges also pose significant obstacles. Legacy systems, which many financial institutions rely upon, often struggle to accommodate modern XAI solutions. This necessitates careful architectural planning to ensure seamless integration while maintaining system stability and performance.
The complexity of implementing XAI in financial systems goes beyond technical challenges—it requires a delicate balance between innovation and responsibility, ensuring that AI systems remain both powerful and accountable.
To address these challenges effectively, financial institutions must adopt a holistic approach that combines technical expertise with ethical considerations. This includes investing in specialized talent, developing robust governance frameworks, and maintaining open dialogue with regulators and stakeholders to ensure XAI implementations serve their intended purpose while meeting all necessary requirements.
Benefits of XAI for Financial Institutions
Financial institutions leveraging artificial intelligence face mounting pressure for transparency in their decision-making processes. XAI presents a powerful solution, offering several critical advantages for banks and financial organizations in an increasingly AI-driven landscape.
XAI dramatically improves transparency in AI-powered financial decisions. By providing clear explanations for model outputs, financial institutions can help stakeholders understand exactly how and why specific lending, investment, or risk assessment decisions are made. For example, when a loan application is denied, XAI can identify the key factors that influenced that outcome, allowing both customers and regulators to comprehend the reasoning.
Enhanced stakeholder trust represents another crucial benefit of implementing XAI. According to Deloitte research, financial institutions that provide clear explanations for AI decisions build stronger relationships with customers, investors, and regulatory bodies. This transparency helps establish credibility and confidence in AI systems, particularly for high-stakes financial decisions like credit scoring or fraud detection.
XAI also significantly strengthens regulatory compliance capabilities. As financial regulators increasingly scrutinize AI applications, the ability to explain model decisions becomes paramount. XAI enables institutions to demonstrate fairness in lending practices, justify risk assessments, and prove adherence to regulatory requirements. This enhanced compliance positioning helps financial organizations avoid potential penalties while maintaining innovative AI implementations.
Beyond compliance, XAI contributes to more effective risk management by allowing financial institutions to better understand and validate their AI models. Risk managers can identify potential biases, assess model performance, and make necessary adjustments with greater precision. This improved oversight leads to more reliable risk assessments and better-informed decision-making across the organization.
In fraud detection, XAI proves particularly valuable by helping analysts understand why specific transactions are flagged as suspicious. Rather than relying on black-box decisions, security teams can examine the specific patterns and anomalies that trigger fraud alerts. This deeper understanding enables more accurate fraud prevention and reduces false positives that could otherwise impact legitimate customer transactions.
XAI transforms AI from a black box into a transparent decision-making partner, enabling financial institutions to harness the full potential of artificial intelligence while maintaining the trust of their stakeholders.
For financial institutions seeking to balance innovation with responsibility, XAI represents a crucial bridge between advanced AI capabilities and the fundamental need for transparency in financial services. By making AI decisions more understandable and accountable, XAI helps build a stronger foundation for the future of AI-powered finance.
Key Techniques for Explainable AI in Finance
Financial institutions increasingly rely on artificial intelligence to make critical decisions about loans, investments, and risk assessments. However, these AI systems often operate as black boxes, making decisions that are difficult to understand or explain. This is where explainable AI (XAI) techniques come in, offering powerful tools to peek inside these complex systems.
One of the most widely adopted XAI methods is SHAP (SHapley Additive exPlanations), which draws from game theory to explain how each variable contributes to an AI model’s decisions. For example, when a bank uses AI to evaluate loan applications, SHAP can show exactly how factors like credit score, income, and employment history influenced the final decision. Recent research has shown that SHAP is particularly effective for analyzing complex financial models because it can provide both detailed individual explanations and broader insights about how the model works overall.
Another valuable technique is LIME (Local Interpretable Model-agnostic Explanations), which creates simplified explanations of AI decisions by examining how the model behaves around specific data points. Think of LIME as creating a magnifying glass that focuses on individual cases. When an AI system flags a credit card transaction as potentially fraudulent, LIME can highlight which specific aspects of that transaction – such as its location, amount, or timing – triggered the alert.
Counterfactual explanations offer yet another approach, answering questions like “What would need to change for this loan application to be approved?” This makes them especially valuable in financial services, where customers and regulators often want to understand not just why a decision was made, but what could be done differently to achieve a different outcome.
Each of these techniques brings unique strengths to the table. SHAP excels at providing comprehensive explanations across an entire model, while LIME shines when detailed explanations of specific cases are needed. Counterfactual explanations bridge the gap between understanding decisions and taking action to change them.
While these methods have revolutionized financial AI interpretability, they each have their limitations. SHAP calculations can be computationally intensive for complex models, LIME’s explanations are sometimes oversimplified, and counterfactual explanations may not always suggest realistic or achievable changes. Understanding these trade-offs is crucial for financial institutions as they work to build more transparent and accountable AI systems.
Regulatory Landscape and XAI
The financial sector’s adoption of explainable AI (XAI) occurs within an increasingly complex regulatory framework. The EU’s landmark AI Act, reaching political agreement in December 2023, exemplifies how regulators are taking decisive steps to ensure AI systems remain transparent and accountable, particularly in high-stakes sectors like finance. Financial institutions deploying XAI must navigate multiple regulatory considerations.
At the core is the requirement for AI systems to provide clear explanations for their decisions – whether approving loans, detecting fraud, or assessing credit risk. This transparency isn’t merely a technical preference; it’s becoming a legal necessity as regulators demand insights into AI decision-making processes.
The regulatory emphasis on explainability serves several critical functions. First, it enables financial institutions to validate that their AI systems aren’t perpetuating biases or making discriminatory decisions. Second, it helps satisfy regulators’ growing demands for documented evidence that AI systems operate within acceptable parameters. Finally, it provides customers with clear explanations when AI systems make decisions affecting their financial lives.
Compliance teams at financial institutions face particular challenges in this evolving landscape. They must ensure AI systems meet current regulatory requirements while remaining flexible enough to adapt to new regulations. This includes implementing robust governance frameworks, regular auditing processes, and clear documentation of AI decision-making pathways.
Regulatory Framework | Key Requirements |
---|---|
General Data Protection Regulation (GDPR) | Transparency and accountability in automated decision-making processes; right to know the logic involved |
Artificial Intelligence Act (EU) | Regulates AI systems based on risk levels; high-risk systems require transparency, risk assessment, and post-market monitoring |
National Institute of Standards and Technology (NIST) | Framework for AI risk management; encourages assessment of ethical implications |
Organization for Economic Co-operation and Development (OECD) | Principles emphasizing transparency, accountability, and inclusiveness in AI development |
Federal Financial Supervisory Authority (Germany) | Advises on weighing benefits of complex models against interpretability; documentation required |
The integration of XAI into financial technologies not only enhances regulatory compliance and consumer trust but also contributes to the ethical development of AI, paving the way for a more transparent and responsible financial landscape. The regulatory focus on transparency extends beyond simple compliance. It represents a fundamental shift in how financial institutions must approach AI implementation – moving from black-box solutions to explainable systems that can withstand regulatory scrutiny and maintain public trust. This shift makes XAI not just a technical solution, but a strategic necessity for financial institutions navigating an increasingly regulated AI landscape.
Risk management teams must now consider both traditional financial risks and new AI-specific regulatory risks. This includes ensuring AI systems can demonstrate fairness in lending decisions, show compliance with anti-money laundering regulations, and provide clear audit trails for regulatory examinations. The stakes are high – non-compliance can result in significant fines and reputational damage.
Future Trends in Explainable AI for Finance
The financial sector is undergoing a transformative era as Explainable AI (XAI) becomes essential for financial decision-making. Regulatory bodies worldwide are focusing on AI transparency, pushing financial institutions to advance their XAI implementations to meet these demands.
Intrinsic explainability is a game-changing approach in financial AI systems. Rather than retrofitting explanations onto existing black-box models, financial institutions are designing AI systems with built-in transparency from the start. This shift promises more reliable and trustworthy AI systems that better serve regulatory requirements and customer needs.
The integration of XAI into core financial processes is accelerating, especially in areas like credit risk assessment and fraud detection. Financial institutions are moving beyond simple feature importance explanations toward sophisticated approaches that provide contextual, real-time insights into AI decisions. This evolution enables faster, more accurate decision-making while maintaining the high standards of transparency required in financial services.
Regulatory advancements are shaping XAI’s future. The European Union’s AI Act and similar initiatives worldwide are establishing stringent requirements for AI transparency in financial services. These regulations are driving innovation in XAI methodologies, pushing financial institutions to develop more robust and comprehensive explanation frameworks.
The financial sector is witnessing a paradigm shift where explainability is no longer an afterthought but a fundamental requirement in AI system design. This transformation is essential for maintaining trust and accountability in an increasingly automated financial landscape.
Deloitte Insights, 2023
Looking ahead, standardized XAI frameworks specifically tailored for financial applications are expected to emerge. These frameworks will likely incorporate multiple explanation methods, from simple rule-based approaches to sophisticated counterfactual explanations, providing a comprehensive understanding of AI decisions across various financial contexts.
The convergence of XAI with other emerging technologies, such as federated learning and privacy-preserving AI, will create new possibilities for secure, transparent financial services. This synthesis will enable financial institutions to balance the demands of privacy, performance, and explainability in their AI systems.
Conclusion and Recommendations
The integration of explainable AI (XAI) into financial systems goes beyond a mere technological upgrade; it has become essential for institutions aiming to maintain trust, ensure compliance, and provide transparent AI-driven services. Financial organizations that adopt XAI gain significant advantages in risk management, fraud detection, and regulatory compliance while fostering deeper trust with stakeholders.
The financial sector is currently at a crucial point where the complexity of AI models must be balanced with their interpretability and transparency. Forward-thinking institutions are recognizing that XAI is not only about explaining model decisions; it is about establishing a foundation of trust that facilitates broader adoption of AI technologies. By implementing XAI solutions, banks and financial institutions can offer clear explanations for automated decisions, meet regulatory requirements, and uphold accountability within their AI systems.
SmythOS stands out as a valuable platform in this context, providing comprehensive tools that support transparent AI development at all stages. Its built-in monitoring capabilities and visual workflow builder allow financial institutions to create and deploy explainable AI solutions without compromising performance or accuracy. The platform’s robust integration capabilities ensure smooth incorporation into existing financial infrastructures while maintaining the high standards of transparency required in the industry.
For financial institutions looking to implement XAI effectively, several actionable steps are recommended. First, establish clear governance frameworks that define explainability requirements for different types of AI applications. Second, invest in training programs to develop internal expertise in XAI methodologies and best practices. Finally, regularly assess and update XAI implementations to ensure they continue to meet evolving regulatory requirements and stakeholder expectations.
As the financial sector progresses through its digital transformation, strategically implementing XAI will become increasingly crucial for maintaining a competitive advantage. Institutions that proactively adopt transparent AI development platforms and establish robust XAI practices will be better positioned to navigate regulatory challenges, build customer trust, and lead in an AI-driven financial landscape.
Last updated:
Disclaimer: The information presented in this article is for general informational purposes only and is provided as is. While we strive to keep the content up-to-date and accurate, we make no representations or warranties of any kind, express or implied, about the completeness, accuracy, reliability, suitability, or availability of the information contained in this article.
Any reliance you place on such information is strictly at your own risk. We reserve the right to make additions, deletions, or modifications to the contents of this article at any time without prior notice.
In no event will we be liable for any loss or damage including without limitation, indirect or consequential loss or damage, or any loss or damage whatsoever arising from loss of data, profits, or any other loss not specified herein arising out of, or in connection with, the use of this article.
Despite our best efforts, this article may contain oversights, errors, or omissions. If you notice any inaccuracies or have concerns about the content, please report them through our content feedback form. Your input helps us maintain the quality and reliability of our information.